Figures & data
Figure 1. Map of the bioregions for this analysis. Areas that have been cleared in the last 30 years are shown in red (as defined by the Statewide Land and Trees Study (SLATS, Department of Science Citation1988-2016)). Protected areas as of 2019 are shown in green.
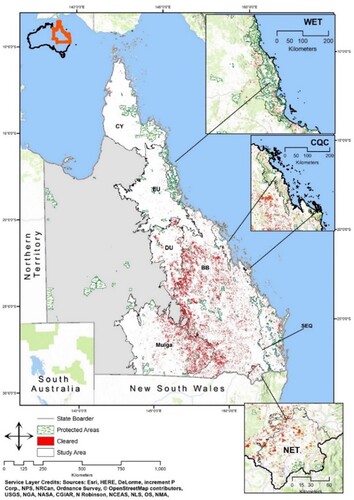
Table 1. Description of each independent predictor variable, the logic behind its inclusion, the data source, year published, and the data type. Datasets by the Queensland Department of Environment and Science, Department of Agriculture and Fisheries, or Department of Natural Resources and Mines were retrieved from http://qldspatial.information.qld.gov.au/catalogue/custom/index.page. We created Rainfall and Temperature data using ANUCLIM http://fennerschool.anu.edu.au/research/products/anuclim-vrsn-61.
Figure 2. Schematic representation of the modelling procedure. Predictor variables listed in were rasterised to a 250*250 m resolution and then stacked into a single dataset per bioregion. We used a generalised estimating equation to predict deforestation probability.
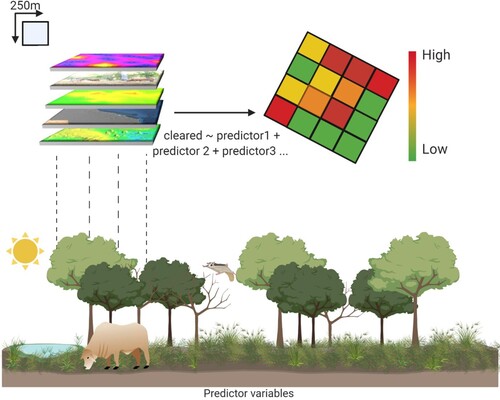
Table 2. A description of the variables included in the final model (p < 0.05) for each bioregion. ‘Built’ refers to Euclidean distance to built-up areas, ‘graze’ refers to grass biomass, ‘rain’ refers to average annual rainfall, ‘roads’ refers to Euclidean distance to State-controlled roads, ‘slope’ refers to slope in per cent rise, ‘temp’ refers to yearly average temperature, ‘wc’ refers to Euclidean distance to major watercourses. The third column shows the mean (M) of simulated Pearson’s Chi-Squared goodness-of-fit values. Chi-square tests whether or not the observed data are consistent with the values imputed from the models (Alsadik Citation2019). ^ indicates that there is no significant difference between the predicted and observed clearing data and the models have performed well. The final column is the mean (M) confidence interval (upper and lower 95 per cent of values) for each bioregion (see methods: model confidence).
Figure 3. Map of the likelihood that a pixel will be cleared given the relevant biophysical characteristics of the pixel. The predicted values for each bioregion are shown in a combined raster format ‘predicted values.’BB = Brigalow Belt, CY = Cape York, CQC = Central Queensland Coast, DU = Desert Uplands, EU = Einasleigh Uplands, Mulga = Mulga Lands, NET = New England Tablelands, SEQ = Southeast Queensland, WET = Wet Tropics.

Table 3. Summary table of the number of regional ecosystems affected in each bioregion per scenario. Number is the number of regional ecosystems affected under each of the scenarios. 1 status change is the number of regional ecosystems that will change status once and 2 status changes are those that will change status twice (i.e. from Least Concern to to Endangered) under each scenario.