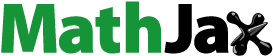
ABSTRACT
The attractiveness of the equities of the Islamic faith-compliant companies as a hedge or possible diversifier has been underscored; however, there is a lack of empirical research on their safe-haven and hedge attributes against changes in the level of cryptocurrency environmental attention (ICEA). We examine whether various distributions of the ICEA possess a significant predictive power on various quantiles of Islamic sectoral stock returns by employing weekly data on the ICEA and Shariah-compliant stocks from 10 sectors of economic activity and base their multi-scale analysis on the complete ensemble empirical mode decomposition (CEEMDAN) approach. We present the asymmetric causality-in-means and quantile-on-quantile regression between the ICEA and Islamic stocks. The empirical results show a significant predictive power of the ICEA on various quantiles of Islamic sectoral stocks in the medium- and long term. We find that the safe-haven and hedging attributes of investments in Islamic stocks are sector-dependent across the medium- and long-term scales. Hence, our findings emphasize that based on market states, possible safe-haven attributes, diversification opportunities, and hedges for cross-sectoral investments with Islamic stocks are viable along various investment horizons for diverse levels of cryptocurrency environmental attention. These findings provide original valuable insights for portfolio management and improving financial stability.
I. Introduction
The exponential growth of the pivotal role of digital assets, particularly cryptocurrencies, in global markets, has been accompanied by widespread interest from market participants, practitioners, regulators, and mass media (Umar et al., Citation2022a; Umar et al., Citation2022; Yousaf et al., Citation2022; Yousaf & Yarovaya, Citation2022). The mining of some cryptocurrencies requires intense use of energy, which is accompanied by negative externalities leading to climate change (Bejan, Bucerzan, and Craciun Citation2022). These externalities of cryptocurrencies have been recently captured in the news-based index of cryptocurrency environmental attention (ICEA) developed by Wang et al. (Citation2022). The originators of the index emphasize that the ICEA is responsive to significant market events and could be used as a control metric for financial markets.
Given the influence of media coverage on investor sentiments, which affect asset prices (Bossman, Teplova, and Umar Citation2022), a section of undeveloped literature has empirically examined the relationship between cryptocurrency environmental attention and various assets. To deepen the evidence provided by the above strand of works, we analyse the safe-haven attributes of Islamic sectoral stocks, in the presence of cryptocurrency environmental attention. Islamic stocks, by their nature, represent a class of sustainable assets, as their Shariah-compliant features assure anti-phoniness, anti-Ponziness, and, hence, foster sustainability. On the other hand, crypto assets are potentially prone to Ponzi arrangements and highly damaging to the environment due to inefficient energy employment (Taleb, Citation2021; Bejan et al., Citation2022). Hence, it would be expectable that the cryptocurrency environmental attention level would be interrelated with the performance of sustainable assets, in general, and Islamic stocks, in particular. We distinguish between several sectors of economic activity that, in our study, are represented by Islamic stocks because previous research by Umar and Gubareva (Citation2021) showed that these sectors react differently to panic and media attitudes during the COVID-19 pandemic.
At this point, it is worth providing a succinct discussion on the nature of Islamic stocks and the differences they have from conventional stocks. We base our description on different previous studies such as Jawadi, Jawadi, and Cheffou (Citation2015), Alhomaidi et al. (Citation2018), Jawadi et al. (Citation2021), Umar and Gubareva (Citation2021), Adekoya, Oliyide, and Tiwari (Citation2022), Asutay, Wang, and Avdukic (Citation2022), Bossman et al. (Citation2022), Godil et al. (Citation2022), Jabeen and Kausar (Citation2022), Shear and Ashraf (Citation2022), Umar et al. (Citation2022c), Aslam et al. (Citation2023), and Umar et al. (Citation2023). A comprehensive review of issues in Islamic equities, addressing comparative performances of Islamic equities vis-à-vis their conventional counterparts, could be found in Masih, Kamil, and Bacha (Citation2018). The nature of Islamic equities is defined by Shariah law, which implies that Islamic equity investments must be scrutinized by Shariah-compliant screening procedures (Asutay, Wang, and Avdukic Citation2022). This process consists of two parts: qualitative compliance and quantitative screening. The former assesses compliance with Islamic investment guidelines ensuring the exclusion of businesses involved in alcohol, tobacco, pork, gambling, weapons, interest-rate-based finance, and non-Shariah-compatible entertainment. The latter is quantitative evaluation, which uses a scrupulous analysis of certain financial ratios, which result in the removal, from the eligible stocks, those companies, which being in Shariah-law compliant businesses, still obtain a large portion of their revenues out of non-Shariah-compliant activities. These activities include, for instance, holding a large proportion of assets in liquid form, as well as lending money on interest. Most of the research on the Islamic equity markets is based on a comparative analysis of the Islamic and conventional equities and it is motivated by the decoupling hypothesis, according to which Islamic stocks are different from conventional stocks due to their distinct risk-return profiles and, thus, provide diversifying opportunities for investors. The decoupling hypothesis has its roots in the assumption that Shariah-compliant stocks, because of their more conservative nature and the sector and financial ratio screening, can be seen as an alternative investment class that has its own distinctive features and does not move in tandem with conventional stocks (Masih, Kamil, and Bacha Citation2018).
From a theoretical perspective, the links between the news-based index of cryptocurrency environmental attention (ICEA) and Islamic stocks are aligned with previous studies. Such studies address asymmetric connectedness between the ICEA, conventional stocks (S&P500), gold, and green assets (Kamal and Hassan Citation2022). Their quantile regression results suggest that ICEA exerts positive effects on S&P500 stocks in bearish market conditions. Moreover, quantile connectedness results show that there is a potential for diversification with clean energy stocks and green bonds in the portfolio against the ICEA in bearish market conditions. Gold has a positive relationship with the ICEA. In addition, clean energy stocks have a consistently positive relationship with the ICEA, specifically during the period of high spikes of the ICEA in 2017–2021, but green bonds failed to maintain consistent positive correlations with ICEA during such a period. In this context, the Shariah-compliant equities represent an extremely important class of sustainable financial assets, which, in line with other sustainable assets, is expected to be influenced by the ICEA, and, hence, herein we investigate this relationship.
The present study contributes to the literature in four ways. First, following the ICEA’s development, this is the first study to examine its influence on Islamic sectoral stocks. Second, we employ the nonparametric quantile-on-quantile regression (QQR) technique, which allows us to determine the possible effects of ICEA on Islamic stocks not only across the various distributions of the sectoral stocks’ returns but also across different states of ICEA. Third, we utilize the complete form of the ensemble empirical mode decomposition (CEEMDAN) technique to delineate the underlying data, into intramode functions (IMFs) representative of investment horizons particularly relevant for market participants, whose preferences vary across the short-, medium-, and long-term investments inline with Owusu Junior et al. (Citation2020) and Bossman et al. (Citation2022). Fourth, our analysis is instrumental for investors and policymakers in their quest to make effective judgements across the realms of high, normal, and low environmental attention.
The rest of the study is designed as follows. Section II presents the literature review. We elaborate on the data and methodological steps in Section III. Then, we delineate and discuss the empirical results and robustness test in Section IV. Finally, we summarize the study and present some notable policy implications in Section V.
II. Literature review
Given the importance of Islamic equity markets, numerous studies have been targeting Sharia-compliant equities and presenting a comparative analysis of their performance vis-à-vis conventional stocks. We recommend consulting the works of Alhomaidi et al. (Citation2018), Hammami (Citation2020), Jawadi et al. (Citation2021), Umar and Gubareva (Citation2021), Adekoya, Oliyide, and Tiwari (Citation2022), Asutay, Wang, and Avdukic (Citation2022), Bossman et al. (Citation2022), Godil et al. (Citation2022), Jabeen and Kausar (Citation2022), Shear and Ashraf (Citation2022), Umar et al. (Citation2022c), Aslam et al. (Citation2023). A vast body of research on the Islamic equity markets focuses on a comparison between the dynamics of the Shariah-compliant and conventional equity markets (Jawadi, Jawadi, and Cheffou Citation2015; Jawadi et al. Citation2021; Aziz et al. Citation2021; Shear and Ashraf Citation2022; Aslam et al. Citation2023). We highlight a literature survey on Islamic equities by Masih, Kamil, and Bacha (Citation2018). Hence, we focus on the recent works, from 2018 onwards.
Investigating liquidity issues, Alhomaidi et al. (Citation2018) try to answer the question of whether Islamic label causes stock price comovements and commonality in liquidity. The authors study how shared beliefs and the personal preferences of individual investors affect their trading and investment decisions. They argue that classifying stocks into Sharia-compliant (Islamic) and non-Sharia-compliant (conventional) has an effect on the investment attractiveness and acceptance of the stocks, especially by individual investors. Their results show that stock classification has an effect on share price comovements through increased stock trading correlation among the groups of Islamic investors. The authors demonstrate that the commonality in preferences among investors in Islamic equities generates commonality in trading activity and stock liquidity. They conclude that classifying an asset as a Shariah-compliant stock increases its price comovement with other Islamic stocks and also increases its commonality in liquidity.
Asset pricing in an Islamic economy is investigated by Hammami (Citation2020). The author defines an Islamic economy as one with borrowing restrictions, no leverage, and no risk-free assets. Then, a consumption-based asset pricing model for this economy under standard preferences is derived. The results provide evidence that news to consumption growth is the main driver of Islamic financial markets, whereas the degree of borrowing constraints affects the pricing of Islamic assets too. The author’s calculations, based on the Saudi Arabian dataset, demonstrate that the proposed model does exhibit a fair adherence in matching the observed equity premium and the volatility of the market return. The model implies that the price-dividend ratio predicts dividend growth, and, hence, that prices are mainly driven by cash-flow news rather than by discount rate news. Empirical tests indicate that the developed model is consistent with the observed data.
Another important strand of the research in what concerns the comparative behaviour of Islamic versus conventional stocks has been triggered by the COVID-19 pandemic. For instance, Aziz et al. (Citation2021) study how Shariah compliance affects investor behaviour during the COVID-19 times. In particular, the authors investigate investor behaviour in herding across firms in the global energy market. Using closing price data for more than two and a half thousand selected firms, the authors reveal herding in both Shariah-compliant and conventional energy firms, especially on the days of a market downtrend. The cross-sectional tests demonstrate higher herding in larger and more-profitable Shariah firms, and those with positive analyst forecasts for the future, which is consistent with pressure-driven behaviour to maintain performance. The authors conclude that COVID-19 does not significantly alter the herding behaviour of the analysed firms.
In their turn, Jawadi et al. (Citation2021) investigate Shariah-compliant and conventional stock market volatility and liquidity during COVID-19 and observe that both liquidity and volatility exhibit an important time variation. This outcome is based on different stock market data, namely, stock prices and trading volumes and diverse types of COVID-19 statistics. The effect of COVID-19 on the liquidity and volatility of the US conventional and Islamic equity indices is assessed by evaluating their efficiency in both linear and nonlinear frameworks. The authors conclude that Islamic stocks are not more resilient than conventional vis-à-vis the COVID-19 pandemic. Following these previous studies, we consider the liquidity issue to be an important strand for further research.
Adekoya, Oliyide, and Tiwari (Citation2022) study risk transmissions between sectoral Islamic and conventional stock markets during the COVID-19 pandemic, trying to answer the question of what matters more: COVID-19 or speculative and sentiment factors? The authors provide novel insights into the volatility connectedness between conventional and Islamic stock markets. They conduct their analysis at the sectoral level and conclude that, on average, in what concerns volatility, Islamic markets tend to be more immune to the pandemic than conventional markets. Finally, the authors demonstrate that the effect is stronger for the speculative/sentiment indicators than for COVID-19 statistics and argue that their findings cater to appropriate policy insights for policymakers and investors.
Asutay, Wang, and Avdukic (Citation2022) explore the performance of Shariah-compliant and conventional stock indices by means of comparative analysis. As a theoretical foundation for their study, the authors use the argument that the behaviour of Islamic indices is determined by different fundamental principles than those defining the dynamics of conventional equities, justifying an exploration of comparative financial performance. This paper offers a comprehensive performance comparison between Islamic indices and conventional indices, based on four main markets: worldwide, the US, Europe and Asia – Pacific for the period between 2007 and 2017. The authors employ the CAPM-EGARCH model. Their overall conclusion is that Islamic indices outperformed conventional indices during the global financial crisis period (2007–2009) and the latter post-crisis phase (2013–2017), especially in the European and Asia – Pacific geographies.
Bossman et al. (Citation2022) investigate dynamic connectedness and spillovers between Islamic and conventional stock markets during COVID-19 employing a time- and frequency-domain approach. The authors use daily stock market indices for G7 conventional and Shariah-compliant markets over the years 2015–2021. They demonstrate that the volatility spillovers across and within Islamic and/or G7 markets are time-varying and frequency-dependent. However, their results show that during market turmoil (Brexit, COVID-19), conventional stocks exhibit higher levels of volatility than Shariah-compliant equities.
Shear and Ashraf (Citation2022) study the performance of Shariah-compliant versus conventional stocks during COVID-19, proving empirical evidence from firm-level data. This work differs from a vast body of the existing literature, which mostly employs equity indices for the sake of comparative analysis. The authors provide robust empirical evidence that Islamic stocks outperform their conventional counterparts during the market meltdown during the initial stage of the pandemic. In particular, they show that the prices of Islamic equities are not affected so adversely by confirmed COVID-19 cases as the prices of conventional stocks. Their results demonstrate that Islamic equities performed better than non-Shariah-compliant stocks better during the pandemic expansion.
Umar et al. (Citation2022c) address spillover and risk transmission between the term structure of the US interest rates and Shariah-compliant stocks. The authors investigate spillovers between the US yield curve components and Shariah-compliant stocks. They provide empirical evidence of the strong connectedness between the US yield curve components and Islamic sectoral equity indices. In particular, it is demonstrated that the level component is the net transmitter, whereas the slope and curvature components are the net recipients. Overall, the results show that the decoupling hypothesis of the Shariah-compliant and conventional financial markets does not hold for the yield curve.
In a recently published paper, Aslam et al. (Citation2023), Shariah-compliant and conventional stock markets are studied by a multifractal cross-correlation analysis with economic policy uncertainty. The authors of this work explore the US economic policy uncertainty cross-correlation with Shariah-compliant and conventional equity markets from the perspective of multifractality. They employ daily stock market prices of five countries: Indonesia, India, Pakistan, Thailand, and the US. The multifractal detrended cross-correlation analysis is used. Their results show that all stock-uncertainty pairs exhibit varying levels of multifractal strength, with the US economic policy uncertainty and US conventional equity market revealing the strongest multifractal patterns. The authors claim that they provide empirical evidence that Shariah-compliant equities reveal behaviour that is different from conventional equities, especially when economic policy uncertainty or any other uncertainty such as geopolitical uncertainty is present.
Wrapping up. From this succinct literature review, it is possible to infer that there exist many research papers, addressing how Islamic stocks are influenced by diverse factors, such as economic policy uncertainty, market sentiment, COVID-19 cases, energy resources, precious metals, US yield curve, among many others. On the other hand, there is growing awareness of the environmental consequences and excessive carbon print of cryptocurrency mining due to elevated levels of energy consumption (Bejan et al., Citation2022). In what concerns investor sentiment across all the markets, including Islamic and conventional equities, it becomes increasingly affected by the positive and negative externalities of cryptocurrencies that are captured in the news-based index of cryptocurrency environmental attention (ICEA) developed by Wang et al. (Citation2022), indicating the existence of at least indirect relations between ICEA and Islamic finance. However, the direct nexus between ICEA and Islamic stocks, as far as we know has never been addressed in the literature. Our research represents the efforts to fill this gap.
III. Data and methods
Data
The data comprise the weekly index of cryptocurrency environmental attention (ICEA) and the stock returns from 10 Islamic economic sectors, namely, basic materials, consumer goods, consumer services, oil & gas, financials, healthcare, industrials, technology, telecoms, and utilities. Predicated by data availability, the data cover the period from 12 January 2014 to 10 July 2022. The ICEA index is gleaned from https://sites.google.com/view/cryptocurrency-indices and the data on Islamic sectoral stocks are sourced from Bloomberg. The natural log transformation is employed. The sample statistics and plots of the data are presented in and , respectively.
Figure 1. Plots of sectoral returns and the index of cryptocurrency environmental attention.

Table 1. Descriptive statistics and stationarity tests.
All the data series are stationary, reject normality, and are generally fat-tailed with negative skewness. From , the trajectories of the returns series exhibit several high volatility clusters, which are rather expectable given that the sample period is characterized by notable extreme events. We could see from the ICEA’s trajectory that the index is responsive to market events, as noted by Wang et al. (Citation2022).
Methods
The CEEMDAN algorithm
The CEEMDAN approach appeals to scholars due to its ability to delineate scales which are exclusive of predefined basis functions (Peng, Wen, and Gong Citation2021). CEEMDAN is robust to the deficiency of low decomposition efficiency and saves a substantial level of processing power and has been applied in recent finance literature (Asafo-Adjei et al. Citation2022).
Following Peng, Wen, and Gong (Citation2021) the CEEMDAN algorithm can be summarized as follows:
Stage I: For an imported data series , begin the number of realizations N for
.
Stage 2: Perform the EMD for N realizations:
Here, is the realization index;
represents the series of white noise added to the intended signal, and
is the noise parameter for the first stage.
Stage 3: The ensemble average IMFs are computed as:
Stage 4: An exclusive first residue is determined as:
Stage 5: Develop N realizations. is an operator that yields the
mode gotten from EMD.
Stage 6: By CEEMDAN, compute the mode as:
Stage 7: Finally, given that , estimate the
residue:
Following this approach, seven IMFs and a residual series are generated after decomposing the signal (original) data series. As per Bossman (Citation2021) and Owusu Junior et al. (Citation2020), we assign IMF1, IMF5, and Residual as the short-, medium, and long-term series, respectively.
Causality-in-quantile means
We ascertain the predictive ability of ICEA across different quantiles of Islamic sectoral stock returns using Citation2012) causality-in-quantiles test. The nonparametric-based Granger-quantile-causality framework is explained as follows:
Assume is stock returns from a given sector and
is ICEA. We test the hypothesis that in the
quantile, given the lag vector
,
has no causal influence on
if:
However, in the quantile, given the lag vector
,
causes
if:
where represents the
quantile of
. Here, note the conditional quantiles,
, of
are predicted by
within the range
.
Further, if have the vectors
and
, their conditional distribution functions can be noted as
and
, respectively, with
and
as conditional vectors. For almost all
, we assume
to be strictly continuous in
.
Then, if and
, we obtain
, with
as its probability.
As per EquationEquations (7)(7)
(7) and (Equation8
(8)
(8) ), every hypothesis for causality-in-quantile can be written as:
Note that causality at fat tails differs from its median counterpartFootnote1 The lag order, , is estimated using the SIC criterionFootnote2 under the vector-autoregression (VAR) that contains sectoral stock returns and the ICEA.
Quantile-on-quantile regression
To investigate relationships between ICEA and Islamic sectoral stock returns, we utilize the nonparametric QQR approach (Sim and Zhou Citation2015). The QQR model is a combination of nonparametric steps with the quantile regression (QR) model. It helps assess the effect of various ICEA quantiles on diverse distributions of Islamic sectoral stock returns.
The QQR model is given as:
where and
are sectoral stock returns and the cryptocurrency environmental attention index, respectively, at period
;
is an unknown parameter determined as the estimated slope coefficient between the observed values of
and
;
is the
quantile of Islamic sectoral stock returns’ conditional distribution, and
is the quantile residue, which is assumed to have a zero conditional
quantile.
In this nonparametric approach, the right specification of the bandwidth () is essential (Asafo-Adjei et al. Citation2022; Bossman et al. Citation2022,d). The wider the bandwidth, the larger the bias surrounding an estimate and the smaller the bandwidth, the larger the estimate’s variance. To maintain a balance between bias and variance in this study, we maintain Citation2015) recommended bandwidth value of
.
IV. Results and discussion
The empirical results are presented in this section in three steps: (i) first, results from the causality-in-means test; (ii) second, the quantile regression estimates; and (iii) the quantile-on-quantile regression estimates. Under each sub-section, the results are reported for the signal (original) data, the short-term scale (IMF1), the medium-term scale (IMF5), and the long-term (Residual IMF) scale.
Causality-in-quantile means analysis
The causality-in-quantiles test captures all distributional quantiles relative to the classical Granger-causality that focuses on the median only (Umar et al., Citation2022a). We employ an innovative technique that accounts for the nonhomogeneous characteristics of time series, focusing on the mutual information they share. Namely, we apply the CEEMDAN methodology to generate intramode functions (IMFs). CEEMDAN offers IMFs corresponding to short-, medium-, and long-term constituents. We follow the extant literature to represent the short term with IMF1, the medium term with IMF5, and the long term with the residual IMF (Owusu Junior and Tweneboah Citation2020, and the references therein). The degrees of correlation between each IMF component and the original data are calculated in accordance with the Pearson correlation coefficient method and are available upon request. After the Pearson correlation coefficient test, IMF1, IMF5 and the residual term are more correlated with the original series, which indicates that the IMF1 and IMF5 components and the residual term are more correlated with the original data. The rest of the IMF2, IMF3 and IMF4 components are less correlated that the three components mentioned above. Hence, we focus on the three components IMF1, IMF5 and the residual term, which are higher correlated with the original time series.
The test statistics from the causality-in-means tests for the signal data and IMFs are reported in , detailing the causal effect of crypto environmental attention on the returns of Islamic sectoral stocks. captures the plots for the signal (original) data series; for IMF1 (i.e. short term); for IMF5 (medium-term); and for the Residual IMF (long-term). For each plot from each figure, the solid horizontal line shows a crucial value of 1.96 at a 95% confidence interval. The null hypothesis holds that a change in ICEA does not Granger-cause a change in a named sectoral Islamic stock across quantiles with a bandwidth spanning between 0.05 and 0.95.
Figure 2. Plots of t-statistics from causality-in-means at the signal.

Figure 3. Plots of t-statistics from causality-in-means at IMF1.

Figure 4. Plots of t-statistics from causality-in-means at IMF5.

Figure 5. Plots of t-statistics from causality-in-means at Residual IMF.

The resulting test statistics from the causality-in-means analysis () present intriguing facts about the analysed Islamic economic sectors. First, specific to , which reveal the results for the signal data and the short-term (IMF1) series, the null hypothesis – that Islamic sectoral stock returns are not Granger-caused by the ICEA – is maintained across all quantiles for all Islamic sectoral stocks. However, from , which reveal the results for the medium- (IMF5) and long-term (Residual IMF) scale, the null hypothesis – that Islamic sectoral stock returns are not Granger-caused by the ICEA – is rejected
across most quantiles for all Islamic sectoral stocks. The conclusion to be drawn is that the ICEA does not Granger-cause Islamic sectoral stock returns across the signal and short-term scale but significantly Granger-causes Islamic sectoral stock returns across the medium- and long-term investment horizons. Impliedly, when assessing the impact of the ICEA on asset returns, focusing on the aggregated impact would lead to ineffective conclusions and this is attributable to the presence of fat tails in financial asset returns (Asafo-Adjei et al. Citation2022). Given that the sample period is filled with a series of market stresses, the findings emphasize the conclusions that noise and fat tails are prevalent in financial markets during turbulent trading periods (Bossman et al. Citation2022; Bossman & Agyei Citation2022).
Quantile regression analysis
Having assessed the predictive power of ICEA on Islamic sectoral stocks, we present the quantile regression (QR) estimates in this sub-section. The beta estimates from the QR are presented in four panels (A-D) of . Panel A contains the beta estimates for the signal (original) data series; Panel B for the short-term scale (IMF1); Panel C for the medium-term scale (IMF5) (); and Panel D for the long-term scale (Residual IMF). We maintain a bandwidth spanning from 0.05 to 0.95.
Table 2. Quantile regression beta estimates.
The following are noticeable from the results. First, exclusive to the signal data (, Panel A), i.e. the composite series, the effect of the ICEA on Islamic sectoral stock returns is negative across lower-to-medium quantiles (mainly 0.05–0.45) and positive across the median and upper quantiles (0.50–0.95). While these estimates are largely significant, some important relationships are shelved by the use of the composite series, which contain noise and fat tails. e.g. negative (positive) relationships that may prevail across upper (lower) quantiles are not detailed by the results at the composite level. This makes the multi-scale analysis, as we employ in this study, relevant for comparing the quantile relationships, which exist across the short-, medium-, and long-term horizons. Second, for the short-term scale (, Panel B), the results show that the direction of the ICEA’s effect on Islamic sectoral stocks is sector-dependent rather than quantile-dependent, as the composite (signal) data (, Panel A) suggest. However, it appears that most relationships lack statistical significance, substantiating insignificant causal influence revealed by the causality-in-means analysis.
Third, it is important to note that in the medium-term (, Panel C), the significance of the asymmetric effect of the ICEA on Islamic sectoral stocks is sector-dependent. Specifically, the ICEA has a significant asymmetric effect on Islamic stock returns from financials, industrials, technology, telecoms, and utility sectors, while it lacks significance for basic materials, consumer goods, consumer services, healthcare, and oil & gas in the medium term. The ICEA has a negative (positive) relationship with the returns from the industrials, technology, and utilities (financials and telecoms) sectors. Fourth, in the long term (, Panel D), the results suggest that the ICEA has a significant asymmetric effect on Islamic stock returns irrespective of the sector. However, note that the asymmetric effect of the ICEA on Islamic sectoral stocks is either mixed or strictly positive or negative based on the sector. By these findings, we emphasize that when assessing the impact of the ICEA on asset returns, in the presence of fat tails, a multi-scale analysis would offer a comprehensive view of how crypto-environmental attention influences returns on faith-based investments.
Quantile-on-quantile regression analysis
We use the QQR approach proposed by Sim and Zhou (Citation2015) to examine the comprehensive relationship between Islamic sectoral stock returns and ICEA. This model is a modification of a conventional quantile regression, which focuses on the influence of the quantiles of a single independent variable on the different quantiles of the dependent variable. The QQR method is primarily dependent on the use of nonparametric estimates and quantile regression (Adebayo et al. Citation2021; Agyei Citation2022). In this way, the benefits of the QQR model reside in the fact that it provides a more complete connection analysis, than, for example, the QR technique and least square approach. In other words, the main advantage of QQR methodology is that this method allows for comprehending relationships between variables outside of the mean of the data, making it useful in understanding outcomes that are non-normally distributed and that have nonlinear relationships with predictor variables (Bossman, Gubareva, and Teplova Citation2023; Bossman and Gubareva Citation2023). The attractiveness of the QQR technique is that it makes it possible to analyse the response of the entire conditional distribution Islamic sectoral stock returns to various levels of ICEA, captured by the quantiles of ICEA. Moreover, to the best of our knowledge, this is the first paper to analyse the ability of ICEA to predict Islamic sectoral stock returns using the QQR approach.
The results from the QQR analysis of how various quantiles of the ICEA affect the different distributions of Islamic sectoral stock returns are displayed in three-dimensional (3D) plots in Footnote3 contains the 3D plots for the signal (original) data series; for the short-term scale (IMF1); for the medium-term scale (IMF5); and for the long-term investment horizon (Residual IMF). For each plot from each figure, the QQR estimates are plotted against the z-axis and the various quantiles of ICEA and sectoral stock returns are plotted against the y- and x-axes, respectively. The colour bar shows the beta estimates, with lower estimates shown in green to blue and higher estimates in yellow to red. Returns refer to the returns on a named Islamic sectoral stock while ICEA is the index of cryptocurrency environmental attention.
Figure 6. 3D plots of QQR estimates at the signal.

Figure 7. 3D plots of QQR estimates at the short-term scale (IMF1).

Figure 8. 3D plots of QQR estimates at the medium-term scale (IMF5).

Figure 9. 3D plots of QQR estimates at the long-term scale (Residual IMF).

We start with a visual inspection of , which displays the 3D plots for the signal (original) data series. The results suggest that when analysed at the composite level, the relationship between the ICEA and Islamic sectoral stock returns is mainly negative across the lower quantiles but positive across the upper quantiles of both the ICEA and the returns from a named sector. This observation is consistent with all sectors – except for a few opposite relationships in the cases of basic materials and utilities – and also substantiates the QR results when the signal data is analysed. For a comprehensive assessment of the overall relationship between cryptocurrency environmental attention, measured by the ICEA, and Islamic sectoral stocks across various scales that represent the short-, medium-, and long-term trading horizons, we discuss the multi-scale QQR results.
presents the 3D plots for the short-term scale (IMF1). The results suggest that in the short term, the direction of the ICEA’s asymmetric effect on Islamic sectoral stock returns is sector-dependent. So, across all the ICEA quantiles and all distributions of Islamic stock returns from the basic materials, financials, industrials, and telecoms sectors, the beta estimates are zero or negative, suggesting a safe-haven role for the stock returns from these sectors against cryptocurrency environmental attention in the short term. Given that the slope coefficients are mainly negative, Islamic stock returns from the above sectors serve as strict hedges in cryptocurrency environmental attention. The direction of the ICEA’s influence on Islamic stock returns from the consumer goods, consumer services, healthcare, oil & gas, technology, and utility sectors is mixed across various quantiles of the ICEA and different distributions of Islamic stock returns from such sectors. However, we observe that the sign (positive/negative) of the slope coefficients varies across distinct quantiles of these sectors in function of the market conditions. This feature allows for possible diversification for cross-sectoral investments in the short term.
presents the 3D plots for the medium-term scale (IMF5). The results suggest that in the medium term, the direction of the ICEA’s asymmetric effect on Islamic sectoral stock returns is sector-dependent, as found for the short-term scale. Across all the ICEA quantiles and all distributions of Islamic stock returns from the healthcare, industrials, oil & gas, technology, and utility sectors, the beta estimates are zero or negative, suggesting safe-haven and hedging roles for the Islamic stock returns from these sectors against cryptocurrency environmental attention in the medium term. Given that the slope coefficients are mainly negative, Islamic stock returns from the above sectors serve as strict hedges in cryptocurrency environmental attention. While this observation is similar for the remaining sectors, the positive beta coefficients at median quantiles (0.35–0.60) of the financials and telecoms sectors and the positive coefficients at the upper quantiles of the basic materials, consumer goods, and consumer services sectors make them lose their attribute as a hedge in normal and bullish market conditions, respectively, in the medium term.
displays the 3D plots for the long-term scale (Residual IMF). Similar to the short- and medium-term scales, the results suggest that in the long term, the direction of the ICEA’s asymmetric effect on Islamic sectoral stock returns is sector-dependent. So, across all the ICEA quantiles and all distributions of Islamic stock returns from the basic materials and oil & gas sectors, the beta estimates are zero or negative, suggesting safe-haven and hedging roles for the Islamic stock returns from these sectors against cryptocurrency environmental attention in the long term. As the slope coefficients are mainly negative, Islamic stock returns from the above sectors serve as strict hedges in cryptocurrency environmental attention in the long term. Islamic stock returns from the consumer services and financials sectors are safe-havens across the lower quantiles but across the median-to-upper quantiles, particularly in the long term, they lose their hedging attribute. For all other sectors (consumer goods, healthcare, industrials, technology, telecoms, and utilities), the ICEA has a positive long-term effect across all quantiles. Nonetheless, it is worth reiterating that with the alternating (positive/negative) sign of the slope coefficients across distinct quantiles of various economic sectors, based on the market condition, possible diversification opportunities for cross-sectoral investments with Islamic stocks are viable in the long-term horizon amid cryptocurrency environmental attention.
V. Conclusion
This study explores the hedging and safe-haven attributes of Islamic sectoral stocks at variable degrees of cryptocurrency environmental attention. We analyse the asymmetric causality-in-means and quantile-on-quantile regression between ICEA and Islamic sectoral stocks. The empirical results show a significant predictive power of ICEA on various quantiles of Islamic stock returns in the medium- and long term. The quantile-on-quantile regression analysis reveals that Islamic stocks from the basic materials, financials, industrials, and telecoms sectors are strict safe-havens against environmental attention in the short term. The Islamic stock returns from the healthcare, industrials, and oil & gas, technology, and utility sectors are strictly safe-havens in the medium term, while those from the basic materials and oil & gas sectors are strictly safe-havens in the long term.
To provide more details about the reason for the priority of the mentioned sectors, which depends on the investment horizon, we evoke a pre-Islamic faith tradition, whose claim in respect to the portfolio theory could be expressed as follows. Build your investment portfolio house on the rock of brick-and-mortar industries as opposed to the sand of service-oriented sectors. Hence, it is wise to build one’s portfolio on a sure foundation, anchoring it to bedrock of industries active in extraction, production, and possession of physical goods, i.e. basic material and oil & gas. It makes one’s portfolio building capable of withstanding all types of weather over sufficiently long investment horizons. That is why we see the basic materials and oil & gas sectors are strict safe-havens in the long term. On the other hand, the more service-oriented exposures, - such as healthcare, technology, and utilities - may be profitable for certain time windows, especially during favourable economic conjectures. That is why we see them as strict safe-havens in the medium, but not in the long term. It is worth highlighting that this consideration is in line with the Shariah law, on which is based the screening procedures of Islamic equities and with the conclusion of Umar and Gubareva (Citation2021), stating that within the Shariah-law compliant companies, there are more asset-based brick-and-mortar stocks and less asset-based equities of services-driven businesses. Not surprisingly, their hedge properties differ from one another.
Therefore, based on economic sectors and the states of both environmental attention and stock markets, our results substantiate the employment of Islamic stocks by investors hunting diversification and downside risk hedging strategies. The empirical findings, thus, have imperative implications for investors, portfolio managers, policymakers, and future studies. To investors and portfolio managers, the results allow for crafting effective hedging strategies across market conditions, sectors, and investment horizons in stress periods where noise and fat tails are characteristic of financial markets. Impliedly, we can emphasize the usability of the findings for an improved delineation of more accurate risk profiles of Islamic stocks of diverse financial institutions with exposures to Islamic equity markets. Furthermore, policymakers could be guided by the findings of this study to fashion policies targeted at reducing asset volatility in periods of high environmental attention and negative externalities from the creation of digital assets. Finally, in terms of future studies, an extension of the findings from this study could be targeted by employing techniques that could capture time and/or time-frequency dynamics.
Acknowledgments
This work was supported by FCT, I.P., the Portuguese national funding agency for science, research and technology, under the Project UIDB/04521/2020. The article was prepared within the framework of the Basic Research Program at HSE University.
Disclosure statement
No potential conflict of interest was reported by the author(s).
Additional information
Funding
Notes
1 We set the bandwidth as . For
and
, Gaussian as used the kernel type.
2 Over-optimization with nonparametric techniques are mitigated by choosing the lag order based on the sparse Schwarz Information Criterion (SIC) under the VAR comprising of the ICEA and Islamic sectoral stock returns.
3 It is worth noting that the QQR estimations yield a 19 by 19 matrix for each analysed pair. To conserve space, we present them in 3D plots using default plotting tools in MATLAB. The numerical estimates of all QQR estimates are available upon request.
References
- Adebayo, T. S., H. Rjoub, G. D. Akinsola, and S. D. Oladipupo. 2021. “The Asymmetric Effects of Renewable Energy Consumption and Trade Openness on Carbon Emissions in Sweden: New Evidence from Quantile-On-Quantile Regression Approach.” Environmental Science and Pollution Research 29: 1875–1886. doi:10.1007/s11356-021-15706-4.
- Adekoya, O. B., J. A. Oliyide, and A. K. Tiwari. 2022. “Risk Transmissions Between Sectoral Islamic and Conventional Stock Markets During COVID-19 Pandemic: What Matters More Between Actual COVID-19 Occurrence and Speculative and Sentiment Factors?” Borsa Istanbul Review 22 (2): 363–376. doi:10.1016/j.bir.2021.06.002.
- Agyei, S. K. 2022. “Diversification Benefits Between Stock Returns from Ghana and Jamaica: Insights from Time-Frequency and VMD-Based Asymmetric Quantile-On-Quantile Analysis.” Mathematical Problems in Engineering 2022: 1–16. doi:10.1155/2022/9375170.
- Alhomaidi, A., M. K. Hassan, D. Zirek, and A. Alhassan. 2018. “Does an Islamic Label Cause Stock Price Comovements and Commonality in Liquidity?” Applied Economics 50 (59): 6444–6457. doi:10.1080/00036846.2018.1486023.
- Asafo-Adjei, E., A. M. Adam, P. Owusu Junior, P. K. Akorsu, and C. L. Arthur. 2022. “A CEEMDAN-Based Entropy Approach Measuring Multiscale Information Flow Between Macroeconomic Conditions and Stock Returns of BRICS.” Complexity 2022: 7871109. doi:10.1155/2022/7871109.
- Asafo-Adjei, E., S. K. Agyei, A. M. Adam, A. Bossman, and Ş. C. Gherghina. 2022. “On the Nexus Between Constituents and Global Energy Commodities: An Asymmetric Analysis.” Applied Economics Letters 1–9. doi:10.1080/13504851.2022.2140753.
- Asafo-Adjei, E., S. Frimpong, P. Owusu Junior, A. M. Adam, E. Boateng, R. O. Abosompim, and A. Dionisio. 2022. “Multi-Frequency Information Flows Between Global Commodities and Uncertainties: Evidence from COVID-19 Pandemic.” Complexity 2022: 1–32. doi:10.1155/2022/6499876.
- Aslam, F., P. Ferreira, H. Ali, Arifa, and M. Oliveira. 2023. “Islamic Vs. Conventional Equity Markets: A Multifractal Cross-Correlation Analysis with Economic Policy Uncertainty.” Economies 11 (1): 16. doi:10.3390/economies11010016.
- Asutay, M., Y. Wang, and A. Avdukic. 2022. “Examining the Performance of Islamic and Conventional Stock Indices: A Comparative Analysis.” Asia-Pacific Financial Markets 29: 327–355. doi:10.1007/s10690-021-09351-7.
- Aziz, S., A. Jalan, R. Matkovskyy, and T. Bouraoui. 2021. “Does Shariah Compliance Affect Investor Behaviour in the COVID-19 Times: Evidence from Herding in the Global Energy Market.” Applied Economics 54 (24): 2825–2836. doi:10.1080/00036846.2021.1999384.
- Bejan, C. A., D. Bucerzan, and M. D. Craciun. 2022. “Bitcoin Price Evolution versus Energy Consumption; Trend Analysis.” Applied Economics 55 (13): 1497–1511. doi:10.1080/00036846.2022.2097194.
- Bossman, A. 2021. “Information Flow from COVID-19 Pandemic to Islamic and Conventional Equities: An ICEEMDAN-Induced Transfer Entropy Analysis.” Complexity 2021: 4917051. doi:10.1155/2021/4917051.
- Bossman, A., and S. K. Agyei. 2022. “ICEEMDAN-Based Transfer Entropy Between Global Commodity Classes and African Equities.” Mathematical Problems in Engineering 2022: 1–28. doi:10.1155/2022/8964989.
- Bossman, A., S. K. Agyei, P. Owusu Junior, E. A. Agyei, P. K. Akorsu, E. Marfo-Yiadom, and G. Amfo-Antiri. 2022. “Flights-To-And-From-Quality with Islamic and Conventional Bonds in the COVID-19 Pandemic Era: ICEEMDAN-Based Transfer Entropy.” Complexity 2022: 1–25. doi:10.1155/2022/1027495.
- Bossman, A., Ş. C. Gherghina, E. Asafo-Adjei, A. M. Adam, and S. K. Agyei. 2022. “Exploring the Asymmetric Effects of Economic Policy Uncertainty and Implied Volatilities on Energy Futures Returns: Novel Insights from Quantile-On-Quantile Regression.” Journal of Business Economics and Management 23 (6): 1351–1376. doi:10.3846/jbem.2022.18282.
- Bossman, A., and M. Gubareva. 2023. “Asymmetric Impacts of Geopolitical Risk on Stock Markets: A Comparative Analysis of the E7 and G7 Equities During the Russian-Ukrainian Conflict.” Heliyon 9: e13626. doi:10.1016/j.heliyon.2023.e13626.
- Bossman, A., M. Gubareva, and T. Teplova. 2023. “Asymmetric Effects of Geopolitical Risk on Major Currencies: Russia-Ukraine Tensions.” Finance Research Letters 51: 103440. doi:10.1016/j.frl.2022.103440.
- Bossman, A., P. Owusu Junior, and A. K. Tiwari. 2022. “Dynamic Connectedness and Spillovers Between Islamic and Conventional Stock Markets: Time- and Frequency-Domain Approach in COVID-19 Era.” Heliyon 8 (4): e09215. doi:10.1016/j.heliyon.2022.e09215.
- Bossman, A., T. Teplova, and Z. Umar. 2022. “Do Local and World COVID-19 Media Coverage Drive Stock Markets? Time-Frequency Analysis of BRICS.” Complexity 2022: 2249581. doi:10.1155/2022/2249581.
- Bossman, A., Z. Umar, S. K. Agyei, and P. Owusu Junior. 2022. “A New ICEEMDAN-Based Transfer Entropy Quantifying Information Flow Between Real Estate and Policy Uncertainty.” Research in Economics 76: 189–205. doi:10.1016/j.rie.2022.07.002.
- Bossman, A., Z. Umar, and T. Teplova. 2022. “Modelling the Asymmetric Effect of COVID-19 on REIT Returns: A Quantile-On-Quantile Regression Analysis.” Journal of Economic Asymmetries 26: e00257. doi:10.1016/J.JECA.2022.E00257.
- Godil, D. I., S. Sarwat, M. K. Khan, M. S. Ashraf, A. Sharif, and I. Ozturk. 2022. “How the Price Dynamics of Energy Resources and Precious Metals Interact with Conventional and Islamic Stocks: Fresh Insight from Dynamic ARDL Approach.” Resources Policy 75: 102470. doi:10.1016/j.resourpol.2021.102470.
- Hammami, Y. 2020. “Asset Pricing in an Islamic Economy.” Applied Economics 52 (29): 3106–3122. doi:10.1080/00036846.2019.1706715.
- Jabeen, M., and S. Kausar. 2022. “Performance Comparison Between Islamic and Conventional Stocks: Evidence from Pakistan’s Equity Market.” ISRA International Journal of Islamic Finance 14 (1): 59–72. doi:10.1108/IJIF-07-2020-0150.
- Jawadi, F., A. I. Cheffou, N. Jawadi, and H. B. Ameur. 2021. “Conventional and Islamic Stock Market Liquidity and Volatility During COVID-19.” Applied Economics 53 (60): 6944–6963. doi:10.1080/00036846.2021.1954595.
- Jawadi, F., N. Jawadi, and A. I. Cheffou. 2015. “Are Islamic Stock Markets Efficient? A Time-Series Analysis.” Applied Economics 47 (16): 1686–1697. doi:10.1080/00036846.2014.1000535.
- Jeong, K., W. K. Härdle, and S. Song. 2012. “A Consistent Nonparametric Test for Causality in Quantile.” Econometric Theory 28 (4): 861–887. doi:10.1017/S0266466611000685.
- Kamal, J. B., and M. K. Hassan. 2022. “Asymmetric Connectedness Between Cryptocurrency Environment Attention Index and Green Assets.” Journal of Economic Asymmetries 25: e00240. doi:10.1016/j.jeca.2022.e00240.
- Masih, M., N. K. M. Kamil, and O. I. Bacha. 2018. “Issues in Islamic Equities: A Literature Survey.” Emerging Markets Finance and Trade 54 (1): 1–26. doi:10.1080/1540496X.2016.1234370.
- Owusu Junior, P., A. K. Tiwari, H. Padhan, and I. Alagidede. 2020. “Analysis of EEMD-Based Quantile-In-Quantile Approach on Spot-Futures Prices of Energy and Precious Metals in India.” Resources Policy 68: 101731. doi:10.1016/j.resourpol.2020.101731.
- Owusu Junior, P., and G. Tweneboah. 2020. “Are There Asymmetric Linkages Between African Stocks and Exchange Rates?” Research in International Business and Finance 54: 101245. doi:10.1016/j.ribaf.2020.101245.
- Peng, Q., F. Wen, and X. Gong. 2021. “Time‐dependent Intrinsic Correlation Analysis of Crude Oil and the US Dollar Based on CEEMDAN.” International Journal of Finance & Economics 26 (1): 834–848. doi:10.1002/ijfe.1823.
- Shear, F., and B. N. Ashraf. 2022. “The Performance of Islamic versus Conventional Stocks During the COVID-19 Shock: Evidence from Firm-Level Data.” Research in International Business and Finance 60: 101622. doi:10.1016/j.ribaf.2022.101622.
- Sim, N., and H. Zhou. 2015. “Oil Prices, US Stock Return, and the Dependence Between Their Quantiles.” Journal of Banking & Finance 55: 1–8. doi:10.1016/j.jbankfin.2015.01.013.
- Taleb, N. N. 2021. “Bitcoin, Currencies, and Fragility.” Quantitative Finance 21 (8): 1249–1255. doi:10.1080/14697688.2021.1952702.
- Umar, Z., A. Bossman, S. Choi, and T. Teplova. 2022. “Does Geopolitical Risk Matter for Global Asset Returns? Evidence from Quantile-On-Quantile Regression.” Finance Research Letters 48 (May): 102991. doi:10.1016/j.frl.2022.102991.
- Umar, Z., and M. Gubareva. 2021. “Faith-Based Investments and the COVID-19 Pandemic: Analyzing Equity Volatility and Media Coverage Time-Frequency Relations.” Pacific-Basin Finance Journal 67: 101571. doi:10.1016/j.pacfin.2021.101571.
- Umar, Z., M. Gubareva, and T. Sokolova. 2023. “Assessing the Impact of Media Sentiment on the Returns of Sukuks During the COVID-19 Crisis.” Applied Economics 55 (12): 1371–1387. doi:10.1080/00036846.2022.2097187.
- Umar, Z., M. Gubareva, T. Teplova, and D. K. Tran. 2022. “Covid-19 Impact on NFTs and Major Asset Classes Interrelations: Insights from the Wavelet Coherence Analysis.” Finance Research Letters 47-B: 102725. doi:10.1016/j.frl.2022.102725.
- Umar, Z., I. Yousaf, M. Gubareva, and X. V. Vo. 2022. “Spillover and Risk Transmission Between the Term Structure of the US Interest Rates and Islamic Equities.” Pacific-Basin Finance Journal 72: 101712. doi:10.1016/j.pacfin.2022.101712.
- Wang, Y., B. Lucey, S. A. Vigne, and L. Yarovaya. 2022. “An Index of Cryptocurrency Environmental Attention (ICEA).” China Finance Review International 12 (3): 378–414. doi:10.1108/CFRI-09-2021-0191.
- Yousaf, I., R. Nekhili, and M. Gubareva. 2022. “Linkages Between DeFi Assets and Conventional Currencies: Evidence from the COVID-19 Pandemic.” International Review of Financial Analysis 81: 102082. doi:10.1016/j.irfa.2022.102082.
- Yousaf, I., and L. Yarovaya. 2022. “Herding Behavior in Conventional Cryptocurrency Market, Non-Fungible Tokens, and DeFi Assets.” Finance Research Letters 103299. doi:10.1016/j.frl.2022.103299.