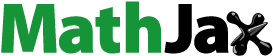
Abstract
This research presents a novel fault-tolerant predictive power control method for a Doubly-fed induction generator (DFIG) used in wind turbine control systems. Due to the proposed control mechanism, the system can continue to function effectively despite open-circuit or short-circuit faults in the insulated-gate bipolar transistors (IGBTs) of the MPC controller. Depending on the type of problem and its location, the tolerant IGBT overcomes power oscillations and limits the power converter's potential switching states. By monitoring the optimal generator speed, wind turbine control systems strive to maximize power output. For wind turbines operating in the partial-load area, a fault-tolerant model predictive control strategy is recommended in order to achieve control goals despite disturbances, uncertainties, sensor, and actuator difficulties. A high order sliding mode observer (HOSMO) is used to evaluate both the actual states and sensor-faults at the same time. A high order sliding mode (HOSM) control strategy based on the MPC controller is used to regulate the speed of wind turbines in order to harness the wind's maximum power.
1. Introduction
For more dependable and effective electrical energy production, wind turbines are being created. Integral sliding surface and control strategy are changed in a full-order compensation by output feedback. To reduce faults and disturbances, by using the full-order compensator [Citation1]. A turbine generator can be affected by a variety of problems, including Inter-Turn Short-Circuit (ITSC), which can also quickly deteriorate the quality of the energy produced. The control methods can be developed using the Indirect Rotor Flux Oriented Control and the Maximum Power Point Tracking [Citation2].
A variable speed wind turbine pitch angle management method uses an adaptive fractional-based Terminal Back-stepping with Sliding Mode controller. Time delay estimating is utilized as an active fault estimate algorithm to locate, recognize, and correct the faults. The use of a higher twisting sliding mode method, which also provides convergence to finite time and high precision, prevents traditional sliding mode chattering [Citation3]. The regulation of doubly-fed induction generator (DFIG) driven WECS was tested using model errors and rotor current sensor-faults.
The speed profiles will be shown by the two fractional-order non-singular terminal sliding mode controllers. A state observer is also included into the control strategy in order to determine the dynamics of the rotor current in the event of sensor failures [Citation4]. Nonlinear dynamics, uncertainties, and outside disturbances are constant in a typical grid-connected wind energy system. Additionally, the wind energy system must maintain a predetermined level of grid connectivity for a predetermined period of time in accordance with grid regulations and in the event of certain faults [Citation5].
Unexpected defects thus increase the requirement for maintenance, which raises energy costs and decreases the reliability of power supply. The wind turbine power generating system's dependability, availability and safety features are also improved [Citation6]. Due to nonlinearities in WECS dynamics, the unpredictable nature of wind and unavoidable occurrence of faults in reality. Long regarded a prerequisite for WECS's maximum power production, fault-tolerant control mechanisms must be developed. For wind turbines with actuator problems, fractional-order non-singular terminal sliding mode control (FNTSMC) strategy has been developed [Citation7].
A reliable aerodynamic torque observer based on the super twisting algorithm is introduced to the control approach. The provided robust control technique minimizes the chattering problem that could occur in conventional sliding mode control systems [Citation8] ensuring acceptable performance under system uncertainties. a wind turbine (WT) with variable speed and adaptive fault tolerance control (FTC) operating in an area with high wind speeds. It reduces mechanical stress in the region of huge wind speeds by restricting the generator output to its rated value and addressing pitch actuator issues. The sliding mode control (SMC) technique is used in FTC design through the application of adaptation law which determines the upper bounds of uncertainty. A number of pitch actuator problems and irregular wind conditions were used to evaluate the controller's effectiveness [Citation9].
WECS fault-tolerant control fundamental problem with a potential actuator fault. An enhanced conditional value at risk (CVAR) stochastic model predictive control (SMPC) controller (CVaR). The WECS dynamics are modelled using a Markov jump linear model and are influenced by a number of random factors, including the wind. The CVaR object function enhances the SMPC controller's fault-tolerant control capabilities. CVa can balance the performance of the system with the risk of random failure [Citation10]. A fault-free and disturbance-free TS fuzzy observer is created for state subsystem by resolving linear matrix inequalities (LMIs). Second, utilizing the other subsystem models, determinations of faults and disturbance are obtained. Third, to mitigate the effects of disruption and actuator problems, an active FTC programme is created [Citation11]. A controller and a disturbance compensator, both are formulated in the discrete time domain, can be combined to perform any operation. The goals of a disturbance compensator include identifying actuator failures and creating the DTC to result in an FTC [Citation12].
A second-order sliding mode with MPPT for managing the DFIG of the wind turbine. The primary error is inadequate power extraction, even though some errors are also brought on by the estimates used to establish current references. To maximize power extraction and follow the DFIG torque directly, use a dependable control, such as second-order sliding mode [Citation13]. The version of the Lyapunov theorem also guarantees the general stability of a closed control system. The control system's performance and robustness are evaluated by addressing three faults between the interface buses [Citation14]. The position sensor-fault-tolerant control for a wind turbine system based on permanent magnet synchronous generators uses a sliding mode observer and back-stepping controller system profiles in order to prevent degradation brought on by sensor-fault [Citation15].
The major contributions of the proposed research work are listed below,
In order to maximize wind power, the research presented here controls the wind turbine speed using high order sliding mode (HOSMO) control method based on the MPC controller.
The Model Predictive Controller was designed as a nominal controller to track the high wind power, whereas the High Order Sliding Mode Observer was developed to show the actual states and sensor-faults.
In order to meet the regulations in the occurrence of uncertainties and actuator problems, a fault-tolerant model predictive control technique is designed for wind turbine in the partial-load region.
A simulation results are demonstrate the suggested MPC controller with High Order Sliding Mode effective performance.
The fault-tolerant control method based on sliding mode observer will be briefly introduced in section 1. The system modelling for wind turbines and DFIG is covered in Section 2. A high order sliding mode (HOSM) control strategy based on the MPC controller is described in Section 3 for controlling the wind turbine speed. Section 4 explains the fault descriptions. The results of the simulation can be found in section 5. Section 6 contains the conclusion of the paper.
2. Methodology
The WECS system uses steady state operation while utilizing aerodynamics and a wind turbine control model to safeguard against transient or unstable circumstances. Figure depicts the design for the DFIG-based wind turbine system. The real and reactive power flow from the generator to the grid is managed by a mechanical and electrical model control system, which also has a safety mechanism in case of an abnormal condition. The system creates signals for power, speed, and voltage. The electrical model system guides the generator to provide the necessary real power and reactive power as well as to maintain synchronism under all operating conditions, while the mechanical model system directs the turbine to extract the maximum mechanical power for a given wind speed. The actual and reactive power from the DFIG is managed by rotor and grid side controllers.
Reactive power flow is controlled by altering the rotor current direction while the DFIG rotor is maintained at its optimal speed using the RSC controller. To quickly deliver leading or lagging reactive power without significantly varying from generator real power, the controller controls a stable DC link voltage across the capacitor at back-to-back terminals. The functioning and stability of the closed-loop system might be affected by other problems, sensor and actuator faults in a partial-load zone must be considered equally when developing a fault control system. The uncertainty of the power coefficients is one of the difficulties. The uncertainty of the power coefficients and the limitations of the wind turbine are some of the difficulties.
2.1 High order sliding mode observer (HOSMO)
The wind turbine's power output will be increased by the control strategy. In order to enforce the ideal tip-speed ratio value, the controller modifies the wind turbine rotational speed in response to changes in wind speed. The energy extraction potential of DFIG wind turbines is maximized even at low wind speeds. However, the shaft's speed must be set in line with an ideal tip-speed ratio, which produces the highest power coefficient, to maximize energy extraction. This suggests that the wind turbine should be accelerated to a specific and special value in order to maximize wind energy extraction depending on wind speed.
HOSMO, which is involved in both fault detection and isolation and fault-tolerant control methods, predicts the stator currents under defective conditions. The system equations are derived using the Park transformation matrix with stator (αβ)-stationary reference ([P θp] = 0).
The equations of the SMO-based system in the (αβ) is
(1)
(1) where the input, output and state vectors
(2)
(2)
Stator currents and rotor flux dynamics error is
(3)
(3)
(4)
(4) The observer errors are
(5)
(5) Lyapunov condition is
(6)
(6) sliding surface is expressed by
(7)
(7) Q is a regular matrix.
Lyapunov function is expressed by
(8)
(8) Replacing
and
by their expression,
(9)
(9) Supposing that
(10)
(10) The derivative of Lyapunov function becomes
(11)
(11)
(12)
(12) If we select
and
verifying
>
and
where
and
denote the maximum values of
and
.
(13)
(13)
Vector sgn is expressed by
(14)
(14) The dynamic of errors become
(15)
(15) It can be then selected
(16)
(16) The parametric vector
is,
(17)
(17) By using Park matrix transformation, the reconstructed three-phase current
in the (abc)-real frame is established.
(18)
(18)
2.2 MPC design for the wind turbine
A wind turbine control system is designed for partial loads. Maximizing extracted aerodynamic power while taking into account all system limits is the aim of the control in this area. According to has a unique maximum at
.
(19)
(19) The optimal generator speed is
(20)
(20)
Therefore, when the generator tracks the optimal generating speed, the greatest amount of electricity is extracted.
3. System modelling
3.1 Wind turbine system
The main components of the nacelle are aerodynamic rotor, drive system and generator are on top of the tower. In a nacelle, the wind energy is turned into electricity. The drive-train rotates at high speed when the generator is powered by wind. The pitch angle of the blades is regulated by the control system mounted on the generator. The pitch angle is determined by the tower speed and the generator speed. A top of the nacelle also has an anemometer formeasuring wind speed and direction. The rotor blades, gear box, and generator comprise the three primary parts of a wind turbines power train. A wind turbine collects and transforms wind energy into mechanical energy. The DFIG rotates as a result of the torque created. The wind energy input is expressed as
(21)
(21) The wind turbine's mechanical output is represented by the following formula:
(22)
(22)
The aerodynamic power consumed by the wind is shown below and is obtained by combining equations 21 and 22.
(23)
(23) Where
and A represent air density, area of turbine blades.
denotes the coefficient of power
Tip ratio is expressed by
(24)
(24) Where,
is the shaft speed
(25)
(25) In above equation, C1, C2 and C3 are constants. The wind turbine's output power will always be at its maximum speed. The maximum speed is indicated by the tip-speed ratio, which is denoted by symbol
. When
the power coefficient value is high
and the wind may provide the most power possible. The Parameters of the 5MW baseline wind turbine is shown in Table .
(26)
(26) Where
Table 1. Parameters of 5MW baseline wind turbine.
and
represent the generator and aerodynamic torque
G is represent the gear ratio
By combining eqns. 23, 24 and 26 we get,
(27)
(27)
(28)
(28) Where,
is represents the wind turbine efficiency
3.2 DFIG model
The following equations can be used to define the dynamic model of a wind turbine based on a DFIG in a synchronously rotating dq reference frame
(29)
(29)
(30)
(30)
(31)
(31)
(32)
(32)
Flux equations of stator and rotor expressed as,
(33)
(33)
(34)
(34)
(35)
(35)
(36)
(36) Where,
{,
}, {
;
} indicate the d and q-axis voltage components
{,
}, {
;
} represent the current components.
The rotating parts mechanical dynamics are
(37)
(37) Electromagnetic torque
can be expressed with stator fluxes and currents as
(38)
(38) stator flux
leads to
=
and
= 0
(39)
(39) The stator active and reactive powers can be expressed as
(40)
(40)
(41)
(41)
(42)
(42)
(43)
(43) where σ =
and the generator slip is defined as s =
.
4. Fault description
Control systems that can withstand potential component failures while preserving desirable performance and stability characteristics are known as fault-tolerant control (FTC) designs. The FTC design is to stop local faults from spreading and growing into system failures, which in turn create safety risks for people and the environment. Since sensor, actuator, and component problems frequently result in rising operating costs, unexpected shutdowns, and potential negative environmental effects, FTC is an important duty that must be completed. A well-designed FTC system can be used to improve the performance of wind turbine by adjusting the maintenance plan and schedule based on the Fault Detection and Diagnosis information. As it provides the essential performance, it also inspires a certain amount of confidence in the planning of the maintenance. FTC approaches frequently use both active and passive schemes.
A fault is defined as an improper deviation from the nominal state of the system's parameters or structure. Examples include sensor reading scaling errors, trapped sensors or actuators, biassed actuator faults, fixed sensors, decreased actuator efficacy, sensor loss and disconnected system components. These flaws have the potential to degrade the closed-loop system's performance and even lead to the system's eventual loss of functionality. Sensor, actuator, and plant faults are the three types of faults. Actuator problems can affect the function of the controller and either limit or stop it from having an impact on the plant. The performance of the controller might be seriously affected by incorrect sensor readings or their entire absence.
An actuator position is controlled by a servo valve, which receives a control signal from a controller based on the difference between the actual and reference rotor speeds. There are many potential sources of pitch system faults, including control and hydraulic subsystems. Sensor failures affect the hydraulic subsystem whereas pump degradation, hydraulic leakage, and high oil air content are issues with the control subsystem. The controller's effectiveness is hampered by pitch sensor bias and gain, making it challenging for the system to follow the proper input. When the pitch sensor fails, the blades are ruffled. The pitch angle can be affected by the generator speed sensor. The sluggish pitch control action can be caused by high oil air content..
4.1 Pitch sensor-fault
A faulty pitch sensor or improper system calibration can allow the bias problem, which is common in wind turbines, to enter the system. The rate and range of a biassed signal can be differentiated from a sensor error, which is referred to as an emerging fault. The performance of the closed-loop system is negatively impacted by biassed output, which also affects how pitch angle is measured.
4.2 Pitch actuator faults
The three problems with hydraulic pitch actuators are excessive oil air content, hydraulic leakage, and pump wear. The pitch system's natural frequency and damping ratio both reflect these defects, which have various implications on the system's dynamics. Table provides the actuator dynamic parameters for the reference wind turbine model.
Table 2. Various faults effect on pitch system dynamics.
4.3 Performance measure
Sensor-Faults:
All forms of scaling, trapped sensor failures, and bias failures are modelled as additive faults. The following is a representation of the sensor-fault system's output:
(44)
(44) Actuator Faults:
Actuator additive and actuator loss effectiveness faults are two forms of actuator faults that are taken into consideration in the WT system. These two forms of actuator defects can be modelled as follows:
(45)
(45)
(46)
(46)
5. Simulation result and discussions
A wind turbine is used to generate power. Whether a wind turbine operates depends on where the primary indicator power changes with wind speed. A wind turbine can output all of its rated power if the wind speed is rated power. The recommended control technique reduces the output power of the wind energy conversion system if the wind speed exceeds the rated wind speed.
5.1 Faulty actuator
When combined the high order sliding mode observer and MPC controller. The rotor speed, generator speed, drive-train torsion angle, and output power are reliably responsive with reduced fluctuation and stress. In Figure , this degradation is shown with significant variations and attrition. In HOSMO tolerates the actuator defect very well and does not exhibit any significant alterations or variations. In faulty circuit simulations, the generator power tracking, voltage, and current are shown in Figure .
5.2 Healthy actuator
The HOSMO controller's performance was determined by applying the wind profile to the turbine without any errors or uncertainties. The generator power tracking, voltage, and current in healthy actuator simulations are shown in Figure . Performance of the reconstruction in the presence of faulty sensors and combined uncertainty. It is clear that the estimation is fulfilled quickly and with little error, highlighting its impressive performance.
The designed fault-tolerant control performance of the wind turbine system controlled by a DFIG is shown in Figure and Figure with 30% three-phase at t = 0.5s, a 50% rise in both stator and rotor resistive values at time, t = 0.5s and a sudden change in wind speed at 0.14s. The effectiveness of fault-tolerant control in a wind turbine system powered by DFIG with 30% three-phase at t = 0.3s under 30% rise in stator and rotor resistive values at t = 0.3s and a sudden change in wind speed. The reaction of the generator speed is seen in Figure .
It is necessary to analyze the time variation of the wind turbine system under reactance fluctuations of the stator and rotor phases. The established fault technique is further investigated under fault tolerance control when the wind speed suddenly increases in order to be as realistic as possible. Figure illustrates the estimate and reconstruction performance of the state observer's rotor current dynamics in the presence of malfunctioning sensors and lumped uncertainty. It is clear that the estimation is fulfilled quickly and with little error, highlighting its impressive performance. Figure illustrates as the estimated status of the plant.
The MPC control technique is intended to diminish the effect of disturbance on the WT. In the case of actuator faults, this method uses the optimal control strategy to generate a full state feedback gain. The numerical results show that the suggested control strategy has the best robustness against the accumulated uncertainty and the lowest tracking error. Figure of the proposed control signal ensures the least tracking errors according to the numerical results that have been presented.
The saturating period has minimized the chattering of the HOSMO control signal. sliding surface convergence almost at zero and HOSMO adaptation gain oscillations. The sliding surfaces attached to the proposed high order sliding mode observers are shown in Figure . In a suggested controller offers the most reliable performance, the quickest convergence time, and the least amount of chattering in the control signal and sliding surfaces, according to the results.
The proposed technique HOSMO was compared to the AOFSMC (Adaptive Output Feedback Sliding Mode Controller) and SMO methods. When compared to the existing methods, the fault duration time is reduced and also performance is increased are shown in table in Table . Higher desirable chattering mitigation performs better than the suggested HOSMO. The aforementioned results indicate that the proposed fault-tolerant control technique describes rotor currents as well as how superior it is at tracking speed and power.
Table 3. Comparison table of fault duration in various methods.
6. Conclusion
In this research, a DFIG employed in a variable speed wind turbine system was given a HOSMO control scheme. In spite of system uncertainties, the aforementioned method Sproduced an optimal power efficiency of the turbine operating over a broad range of wind speeds. The proposed method results in more consistent behaviour with fewer changes. A fault-tolerant control is provided to ensure that the wind turbine system will perform well in the partial-load region. To capture the highest power while accepting parameter constraints, an MPC is presented. To manage uncertainty, estimate the system's actual states, and identify and isolate sensor issues, a high order sliding mode observer is utilized. Stress on the torsion angle of the drive-train, output power, generator speed and rotor speed. In the case of a faulty actuator, HOSMO now has more fault-tolerant capability. The proposed HOSM observer and MPC controller provide both a precise prediction of the aerodynamic torque and assurance that the speed tracking target is met, enabling increased power extraction to be maintained with varying wind speeds and system uncertainties.
Disclosure statement
No potential conflict of interest was reported by the author(s).
References
- Azizi A, Nourisola H, Shoja-Majidabad S. Fault tolerant control of wind turbines with an adaptive output feedback sliding mode controller. Renew Energy. 2019;135:55–65. doi:10.1016/j.renene.2018.11.106
- Sellami T, Berriri H, Jelassi S, et al. Short-circuit fault tolerant control of a wind turbine driven induction generator based on sliding mode observers. Energies. 2017;10(10):1611. doi:10.3390/en10101611
- Mazare M, Taghizadeh M, Ghaf-Ghanbari P. Fault tolerant control of wind turbines with simultaneous actuator and sensor faults using adaptive time delay control. Renew Energy. 2021;174:86–101. doi:10.1016/j.renene.2021.04.077
- Mousavi, Yashar, Geraint Bevan, Ibrahim Beklan Kucukdemiral, and Afef Fekih. “Active fault-tolerant fractional-order terminal sliding mode control for DFIG-based wind turbines subjected to sensor faults.” In IEEE IAS global conference on emerging technologies (GlobConET'22). IEEE, 2022.
- Asghar M. Performance comparison of wind turbine based doubly fed induction generator system using fault tolerant fractional and integer order controllers. Renew Energy. 2018;116:244–264. doi:10.1016/j.renene.2017.01.008
- Habibi H, Howard I, Simani S. Reliability improvement of wind turbine power generation using model-based fault detection and fault tolerant control: A review. Renew energy. 2019;135:877–896. doi:10.1016/j.renene.2018.12.066
- Mousavi Y, Bevan G, Küçükdemiral IB, et al. Maximum power extraction from wind turbines using a fault-tolerant fractional-order nonsingular terminal sliding mode controller. Energies. 2021;14(18):5887. doi:10.3390/en14185887
- Barambones O, Cortajarena JA, Calvo I, et al. Real time observer and control scheme for a wind turbine system based on a high order sliding modes. J Franklin Inst. 2021;358(11):5795–5819. doi:10.1016/j.jfranklin.2021.05.022
- Fekih A, Mobayen S, Chen C-C. Adaptive robust fault-tolerant control design for wind turbines subject to pitch actuator faults. Energies. 2021;14(6):1791. doi:10.3390/en14061791
- Shi Y-T, Xiang X, Wang L, et al. Stochastic model predictive fault tolerant control based on conditional value at risk for wind energy conversion system. Energies. 2018;11(1):193. doi:10.3390/en11010193
- Li S, Wang H, Aitouche A, et al. Active fault tolerant control of wind turbine systems based on DFIG with actuator fault and disturbance using Takagi–Sugeno fuzzy model. J Franklin Inst. 2018;355(16):8194–8212. doi:10.1016/j.jfranklin.2018.08.021
- Vidal Y, Tutivén C, Rodellar J, et al. Fault diagnosis and fault-tolerant control of wind turbines via a discrete time controller with a disturbance compensator. Energies. 2015;8(5):4300–4316. doi:10.3390/en8054300
- Beltran B, El Hachemi Benbouzid M, Ahmed-Ali T. Second-order sliding mode control of a doubly fed induction generator driven wind turbine.”. IEEE Trans Energy Convers. 2012;27(2):261–269. doi:10.1109/TEC.2011.2181515
- Ullah N, Sami I, Babqi AJ, et al. Processor in the loop verification of fault tolerant control for a three phase inverter in grid connected PV system. Energy Sour Part A Recov Utiliz Environ Effects. 2023;45(2):3760–3776.
- Tahri A, Moreau S, Hassaine S. Experimental Validation of Mechanical Sensor Fault Diagnosis And Fault-Tolerant Control For Wind Turbine.