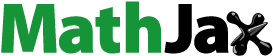
ABSTRACT
Residual methane emission (RME) is a trait that has previously been identified as being independent of animal production traits. The objective of this study was to investigate the effect of ranking grazing dairy cows by RME on animal productivity and enteric methane (CH4) emissions. Milk production, dry matter intake (DMI), liveweight (LWT) and CH4 were recorded on grazing late lactation dairy cows at Teagasc Moorepark, Ireland. The dairy cows were producing 352 g CH4/day, while consuming 16.6 kg DM. The mean methane yield was 20.79 g CH4/kg DMI. Residual methane emission was calculated as the difference between measured CH4 yield and New Zealand emission factor (21.6 g CH4/kg DMI). These dairy cows were ranked based on their RME and classified into groups. The low RME group produced 15% less CH4 than the high RME group while maintaining milk production and feed conversion efficiency. The low RME group had lower methane yield, and methane intensity. There are no significant phenotypic correlations between RME and animal production traits such as energy corrected milk yield, or LWT. These results indicate that RME has the ability to select and rank low emitting grazing dairy cows while being independent from animal productivity traits.
Introduction
As the global population increases and is predicted to reach 9.1 billion by 2050, food demand is expected to also increase by 60–70% (FAO Citation2009; van Dijk et al. Citation2021). This is a huge challenge that the world is facing and with climate change altering growing seasons this becomes more difficult to achieve (Ray et al. Citation2019). Along with these challenges, reducing emissions is also a key target area for international and national governments. Emission reduction targets have been set in order to lower the effect that human activity is having on the climate. Within these targets sectors such as agriculture have been given specific reduction targets to strive towards (United Nations Citation2016). Enteric CH4 is the major gas that is contributing to agricultural emissions in New Zealand and Ireland (Environmental Protection Agency Citation2022; Ministry for the Environment Citation2022). Enteric CH4 emissions are produced as a by-production from fermentation and digestion in ruminants (Ministry for the Environment Citation2022; Environmental Protection Agency, 2022). Within the agricultural sector, enteric CH4 is the largest contributor to greenhouse gases (GHG) in both Ireland and New Zealand (Ministry for the Environment Citation2022; Environmental Protection Agency Citation2022).
Residual CH4 emissions (RME) is a relatively new trait that has been identified as a potential selection index trait for animal breeding programs (Herd et al. Citation2014; Smith et al. Citation2021). It has been reported that RME is independent of production traits and therefore is able to rank and select animals with low enteric CH4 emissions with the same level of productivity. This trait has not been assessed on grazing dairy cows and therefore this study’s objective is to investigate the potential for RME to rank and select animals that are low enteric CH4 emitting animals while not effecting productivity, i.e. milk production, dry matter intake, feed conversion efficiency.
Materials and methods
This study was conducted in Teagasc Moorepark, Co Cork, Ireland between 3rd August and 18th October 2020 (10 week period). Enteric CH4 emissions and animal production traits were measured on forty-five late lactation grazing dairy cows. The mean lactation number was 3.02 ± 1.67. There were twenty-seven Holstein-Friesian and eighteen Holstein-Friesian Jersey crossbred cows ranging from first to eighth lactation. The average stocking rate was 2.6 cows per hectare and these cows were managed in a rotational grazing system. The sward composition consisted of mainly perennial ryegrass (Lolium perenne; > 85%), and the remainder consisted of white clover and meadow grasses (Poa, Festuca pratensis and Trifolium repens L., cv. Chieftain). All procedures were approved by the Teagasc Animal Ethics Committee and the Health Products Regulatory Authority (HPRA).
Animal measurements
Individual cow milk yield and composition was measured throughout the study. Milk yield was measured at each milking (Dairymaster, Causeway, Co. Kerry, Ireland), typically between 07.00 and 09.30 in the morning and again between 14.30 and 16.30 in the afternoon. Milk composition samples were taken twice weekly on Tuesday afternoon and Wednesday morning milkings using Dairymaster milk sampling equipment (Dairymaster, Causeway, Co. Kerry, Ireland). These milk samples were analysed using the Pro-Foss FT 600 (MilkoScan TM FT) for protein, fat and lactose yields, as well as somatic cell count and milk urea.
Liveweight (LWT) and body condition score (BCS) were also measured weekly. Weighing and BCS took place the same morning every week after milking. LWT was measured using electronic weighing scales (Tru-test, Auckland, New Zealand). Body condition scoring was scored by a trained individual on a scale of one to five with 0.25 increments (Lowman and Scott Citation1976).
Individual dry matter intake (DMI) was estimated using the n-alkane technique (Mayes et al. Citation1986) as modified by Dillon and Stakelum (Citation1989). This occurred twice during the 10 week study (week 1 and week 10). All cows were orally dosed twice a day for 12 days with a paper bullet (Carl Roth GmbH, Karlesruhe, Germany) containing 500 mg of dotriacontane (C32- alkane). After 7 days of oral dosing of C32, faecal collection begins. Faeces were collected twice daily before each milking for 5 days. These faecal samples were bulked (12 g of each sample), dried for 48 h at 60°C and milled through a 2 mm screen then stored for chemical analysis. Two herbage samples of approximately fifteen individual grass snips were manually collected with hand shears (Gardena, UK). The herbage selected mimics the grazing pattern observed on previously grazed swards. These herbage samples were frozen at −20°C and then bowl chopped (Muller, Type MKT 204 Special, Saabrucken, Germany). Once chopped the samples were freeze-dried at −50°C for 120 h and milled through a 2 mm screen. Concentrate samples that were fed through the GreenFeed were also sampled. These samples were dried at 60°C and milled through a 2 mm screen then stored for chemical analysis. The herbage, concentrate and faecal samples were analysed for alkane content. The ratio of C31 and C32 alkane present in these samples along with C32 dosage rates were used to establish the estimated DMI using the equation stated by Mayes et al. (Citation1986).
Enteric CH4 emissions were measured using two outdoor GreenFeed systems (C-Lock Inc, Rapid City, SD, USA). The cows had constant access to the GreenFeed’s allowing them to visit at any time. Before the experimental period there was a training period to ensure each cow had an adequate visitation frequency. Concentrate (Dairy pride concentrate, Dairygold, Ireland) was dispensed as 34 grams every 20 s for a total of 200 s. During the study, cows were able to visit the GreenFeed and receive concentrate at a minimum of every 4 h. The cows visited on average 2.21 times per day throughout this study. Standard gas calibrations were carried out using span (20% oxygen and 80% nitrogen) and zero (10 ppm hydrogen, 500 ppm CH4, 5000 ppm carbon dioxide and 21% oxygen and the balance nitrogen) gases. The calibrations occurred automatically every 3 days at 04.00. Manual carbon dioxide calibrations were done monthly to ensure the sensors did not drift away from the baseline concentrations over time.
Individual estimated enteric CH4 production values were excluded from the study if the average visits frequency was ≤1 visit per day for each 7-day period. The diurnal pattern for the 10-week period was evenly distributed throughout the day and therefore no adjustments had to be made. All data was averaged per week of the experiment.
Herbage mass measurements
Pre-grazing herbage mass was determined by cutting two strips 1.2 m wide by a known length (approximately 10 m) using an Etesia mower (Etesia UK Ltd., Warwick, UK) in the sward prior to grazing. The herbage from each strip was weighed and a sample of approximately 300 g was collected. A sub-sample of 100 g was weighed, dried for 16 h at 90°C to determine dry matter content.
Compressed sward height was recorded pre and post cutting on each strip by taking 10 measurement per strip using a rising plate metre (Jenquip, Fielding, New Zealand). This was used to calculate the sward density (cut herbage mass [kgDM/ha]/[pre-cutting height – post cutting height]). Post-grazing herbage heights were also recorded using a rising plate metre (Jenquip, Fielding, New Zealand). Herbage mass was then corrected for post-grazing sward height using the following equation described by Delaby et al. (Citation1998).
Corrected herbage mass = (pre-cutting compressed sward height – targeted residual)/sward density.
Calculations
Energy corrected milk yield (ECMY) was calculated through the following formula (Sjaunja et al. Citation1991):
Percentage of gross energy (GE) intake consumed through methane production (Ym) was calculated using the following formula (Herron et al. Citation2021):
Methane yield = g CH4 / kg DMI.
Methane intensity = g CH4 / kg milk solids.
Methane production = g CH4.
Residual methane emissions (RME) = measured CH4 yield – CH4 yield estimated.
The estimated CH4 yield is the same emission factor (21.6g CH4/kg DMI) that is used in New Zealand GHG inventory calculations (Ministry for Primary Industries Citation2013).
The highest RME animals were classified as high, the lowest RME animals were ranked low and the 15 animals that were in the middle were ranked medium. The herd was split in 3 groups with 15 cows in each group. This resulted in the range for each rank group being:
Statistical analysis
All statistical analysis was carried out using SAS software version 9.4 (SAS Institute Inc, Cary, NC, USA). MEANS procedure was used to calculate the descriptive statistics (mean, standard deviation and coefficient of variation). Least squares means and standard errors for methane, milk production and composition traits, and live weight, dry matter and feed efficiency traits for each for the RME groups were obtained with a linear model that included the fixed effect of RME group and residual error. The least squares means were used for multiple mean comparison using the Fisher’s least significant difference test. Significant differences were declared at P < 0.05.
Simple linear regressions of CH4, energy corrected milk yield, LWT, and DMI on RME were plotted using Excel (Microsoft Corporation, 2018). Partial correlations of energy corrected milk yield, LWT, and DMI with RME were estimated with the GLM procedure with a linear model that included the fixed effects of parity and breed.
Results
Herbage chemical analysis
The mean chemical quantities such as organic matter digestibility are shown in . Herbage mass measured pre and post-grazing is also included in these graphs which were sampled during the two intake measurement periods (week 1 and week 10). There is a difference between DMI offered and measured (± 0.27 kg). This is due to the sampling periods as DMI was measured only during week 1 and 10 of the study period. The mean daily herbage allowance and mean GreenFeed concentrate intake are from every day throughout the study.
Table 1. Pre and post-grazing herbage masses and herbage chemical composition of pasture eaten during the study period.
Descriptive statistics
Mean, standard deviation and coefficient of variation values for the different traits are presented in . The average CH4 produced was 352 g per day. When comparing the CH4 emitted against production traits such as milk solids (MS) or DMI, cow efficiencies can be identified. The methane yield (g CH4/kg DMI) is 20.79 g/kg, and methane intensity (g CH4/kg MS) is 224g/kg. The coefficient of variation is relatively low for enteric CH4 production at 13%, in comparison to other animal production traits such as milk yield and composition, and comparative to DMI, LWT, and BCS.
Table 2. Number of observations (N) Mean, standard deviation (SD) and coefficient of variation (CV) for enteric methane, milk production and composition traits, and live weight traits measured in late lactation dairy cows in Ireland.
Residual methane classification
shows the least squares mean, standard error and p-values for each residual methane classification and different traits. The cows were ranked as high, medium, or low through their residual methane yield. The low group produced 15% less enteric CH4 than the high group. While producing less enteric CH4 these animals in the low group have equivalent milk yield, MS, milk urea, and BCS. There was no significant difference between visits per day between each RME group; low 2.21, medium 2.20 and high 2.19 (low-high p-value = 0.171; low-medium p-value = 0.839, medium-high p-value = 0.222). The overall mean visits per day was 2.21 ± 0.248, with a mean animal to animal coefficient of variation of 11%. The low RME group have lower LWT (−42kg) and higher DMI (1.5kg) in comparison to the high RME group. These groups had the same proportions of Holstein-Friesian - Jersey crossbred animals (5-6 crossbred animals per group). However, low RME group has a significantly greater mean parity of 2.65, in comparison to the high RME group of 2.02 (p-value = 0.036).
Table 3. Least squares mean and standard errors (SE) for methane, milk production, live weight, dry matter intake (DMI) measured in late lactation dairy cows classified into three groups of residual enteric methane production.
These high, medium, and low groups were analysed for measures of efficiency as shown in . These results show that there is no difference in feed efficiency between the groups, however there is a significant difference between enteric CH4 efficiency traits. The low group produced 17% less enteric CH4 per kg MS produced and 22% less enteric CH4 per kg DMI. Both the medium and low groups exhibit a significant difference between the high group for these CH4 efficiency traits. This indicates that the low group were producing significantly less enteric CH4 without any effect on feed efficiency, or milk productivity.
Relationships between residual enteric methane emissions and animal productivity traits
Dry matter intake has a negative linear regression trendline and a significant partial phenotypic correlation with RME. This means that as DMI increases it would be expected that the RME decreases. demonstrates that there is a tendency for daily enteric CH4 production and ECMY to have a negative relationship with RME, however both traits had insignificant partial phenotypic correlations with RME. Liveweight has a positive trendline with RME, however an insignificant partial correlation.
Discussion
Reducing enteric CH4 emissions from dairy cows within the agricultural sector has the potential to play a large role in achieving the emission reduction targets set nationally and internationally (Environmental Protection Agency Citation2022; Ministry for the Environment Citation2022). However, with reducing daily enteric CH4 emissions it is likely that there may also be a reduction in productivity as shown by a number of studies (Molano and Clark Citation2008; Shibata and Terada Citation2010; Bird-Gardiner et al. Citation2017). Starsmore et al. (CitationIn Review) reported that it would be expected that as CH4 reduced, milk production, liveweight and dry matter intake would also be expected to decrease. Therefore, when reducing CH4 it is important that other traits and consequences are investigated to ensure continued economic prosperity of farmers, sectors, and nations. Some authors have proposed residual CH4 production (RME) as a potential trait to select for when aiming to lower CH4, while not affecting productivity (Herd et al. Citation2014; Smith et al. Citation2021). Therefore, the objective of this study was to investigate the potential for RME to select grazing cows with low enteric CH4 production, without impacting productivity.
Enteric CH4 emission traits were evaluated in the current study. The average CH4 in this study was 352 g/day measured through the GreenFeed monitoring system. This is comparable (278–384 g/day) to other late lactation grazing perennial ryegrass pasture studies (Wims et al. Citation2010; O'Niell et al. Citation2012; Jonker et al. Citation2018). The daily visitation frequency in this study (2.21 ± 0.64) is comparative to other studies (2.4–1.6 times per day; Manafiazer et al. Citation2016; Waghorn et al. Citation2016) in both indoor and outdoor systems. The coefficient of variation reported in this study for CH4 (13%) is lower than milk production and composition traits and within 2% of DMI, LWT, and BCS. These two factors indicate that the enteric CH4 data has the same variation between and within animals in comparison to other traits and also there is sufficient data points in comparison to other studies.
Residual methane in this study is the difference between measured CH4 yield (g CH4/kg DMI) and estimated CH4 yield. When classifying animals by RME it is evident that there is a large variation of RME between animals ranging from 8 to −4 g CH4/kg DMI. The cohort of dairy cows in this study were split into 3 groups, according to their RME: high, medium, and low. These RME groups did not exhibit any significant difference in any milk production and composition traits. However, there were significant differences in LWT and DMI. The animals in the low RME group were 42kg lighter and consumed 1.49kg more than the high RME group. This is showing that these low RME animals are able to maintain productivity, reduced CH4 production while being lighter and consuming more DMI. These RME groups did show a significant difference between CH4, with the low and medium groups both having significantly lower CH4 than the high group. These same trends (CH4 differences but no performance differences) were also reported by Smith et al. (Citation2021) in beef cattle. Methane efficiency traits also indicated that animals in the low group produced less CH4 per unit of milk solids produced and per unit of DMI. Therefore, these animals identified in the low group are more enteric CH4 efficient. In previous years, feed efficiency (kg MS/kg DM; kg ECMY/kg DM) has been a key metric in breeding systems to maximise utilisation of feed into outputs (Waghorn and Hegarty Citation2011). It has been thought that animals with improved feed efficiency may have a reduced CH4 (Yan et al. Citation2010; Waghorn and Hegarty Citation2011; Løvendahl et al. Citation2018). Within the current study, there was no significant difference between each RME group for feed efficiency. This finding is consistent with Flay et al. (Citation2019) study where residual feed intake and enteric CH4 production were not correlated in dairy heifers. This indicates there are other factors that influence low emitting animals to be producing less enteric CH4 than expected.
As RME was calculated as the difference between measured CH4 yield and NZ inventory emission factor (21.6 g CH4/kg DMI), this study has highlighted the difference between animal efficiencies. There were animals producing as little as 16 g CH4/kg DMI and as high as 30 g CH4/kg DMI. The overall mean CH4 yield is 20.79 g CH4/kg DMI, indicating that the NZ inventory emission factor may be overestimating national enteric CH4 emissions. The NZ inventory factor is established by the International Panel of Climate Change using Tier 2 methodology. Studies that have been carried out during late lactation also indicate that the 21.6 g CH4/kg DMI emission factor used in the inventory calculations is too high and reducing this value would better reflect what is occurring (Wims et al. Citation2010; Waghorn et al. Citation2016). Based on the current NZ inventory equation it would be expected that these animals are producing 359 g CH4 per day from 16.60kg DMI. This formula is resulting in a slight overestimation of enteric CH4 production. Therefore, this emissions factor should also be assessed for its accuracy as other studies also indicate that dairy cows are producing less than 21.6 g CH4/kg DMI throughout lactation (O'Niell et al. Citation2011).
The results in this study showed tendencies for lower RME animals to have lower LWT, greater ECMY and greater DMI. There is a significant partial correlation between RME and DMI, therefore as DMI increases it is expected that RME will decrease. Smith et al. (Citation2021) reported no significant correlations between RME and production traits. These results are indicating that RME yield is highly related to DMI as expected, but independent of milk production, LWT and enteric CH4 production. Therefore, if RME yield was used to rank and select animals for breeding schemes, these results indicate that there would be little effect on overall animal productivity as LWT and milk production, are not correlated with RME. However, the results in this study only capture phenotypic associations and therefore further work need to be done to establish genetic relationships between these factors and RME yield.
Conclusion
Ranking dairy cows based on RME resulted in a 15% reduction in CH4 between the high and low RME groups. The results in this study show that the RME trait is phenotypically independent of milk production, and liveweight, however genetic associations have not been established in this study. The cows that are classified as low RME also are producing less enteric CH4 per kg milksolids and per kg DMI. These are two key metrics that milk processing companies and governments will be interested in going forward to market products and calculate national emissions. The explanation behind the differences in RME is not identified in this study. Additional analysis should be conducted to discover what is causing this difference in RME between animals.
Acknowledgements
The authors acknowledge the contribution of the farm staff at the Teagasc Moorepark research farm as well as a research grant from Science Foundation Ireland and the Department of Agriculture, Food and Marine on behalf of the Government of Ireland under the grant 16/RC/3835 (VistaMilk). The authors have not stated any conflicts of interest.
Disclosure statement
No potential conflict of interest was reported by the author(s).
Additional information
Funding
References
- Bird-Gardiner T, Arthur PF, Barchia IM, Donoghue KA, Herd RM. 2017. Phenotypic relationships among methane production traits assessed under ad libitum feeding of beef cattle. Journal of Animal Science. 95:4391–4398. doi:10.2527/jas2017.1477.
- Delaby L, Peyraud J-L, Bouttier A, Peccatte J-R. 1998. Effet d’une réduction simultanée de la fertilisation azotée et du chargement sur les performances des vaches laitières et la valorisation du pâturage. Annales de Zootechnie. 47:17–39. doi:10.1051/animres:19980102.
- Dillon P, Stakelum G. 1989. Herbage and dosed alkanes as a grass measurement technique for dairy cows. Irish Journal of Agricultural Research. 28:104.
- Environmental Protection Agency. 2022. Ireland's national inventory report, 2022. Dublin, Ireland: Environmental Protection Agency.
- FAO. 2009. How to feed the world in 2050 (High-Level Expert Forum, 2009).
- Flay HE, Kuhn-Sherlock B, Macdonald KA, Camara M, Lopez-Villalobos N, Donaghy DJ, Roche JR. 2019. Hot topic: selecting cattle for low residual feed intake did not affect daily methane production but increased methane yield. Journal of Dairy Science. 102:2708–2713. doi:10.3168/jds.2018-15234.
- Herd RM, Arthur PF, Donoghue KA, Bird SH, Bird-Gardiner T, Hegarty RS. 2014. Measures of methane production and their phenotypic relationships with dry matter intake, growth and body composition traits in beef cattle. Journal of Animal Science. 92:5267–5274. doi:10.2527/jas.2014-8273.
- Herron J, O’Brien D, Shalloo L. 2021. Life cycle assessment of pasture-based dairy production systems: current and future performance. Journal of Dairy Science. 105:5849–5869. doi:10.3168/jds.2021-21499.
- Jonker A, Green P, Waghorn GC, van der Weerden T, Pacheco D, de Klein C. 2018. A meta-analysis comparing four measurement methods to determine the relationship between methane emissions and dry matter intake in New Zealand dairy cattle. Animal Production Science. 60:96–101. doi:10.1071/AN18573.
- Løvendahl P, Difford GF, Li B, Chagunda MGG, Huhtanen P, Lidauer MH, Lassen J, Lund P. 2018. Review: selecting for improved feed efficiency and reduced methane emissions in dairy cattle. Animal. 12:336–349. doi:10.1017/S1751731118002276.
- Lowman BG, Scott NA. 1976. Condition scoring for cattle. Edinburgh, UK: East of Scotland College of Agriculture.
- Manafiazer G, Zimmerman S, Basarab J. 2016. Repeatability and variability of short-term spot measurement of methane and carbon dioxide emissions from beef cattle using GreenFeed emissions monitoring system. Canadian Journal of Animal Science. 97(1):118–126.
- Mayes RW, Lamb CS, Colgrove PM. 1986. The use of dosed and herbage n-alkanes as markers for the determination of herbage intake. Journal of Agricultural Science. 107:161–170. doi:10.1017/S0021859600066910.
- Ministry for Primary Industries. 2013. Evaluation of the energy equations used by the National Enteric Methane Inventory. Ministry for Primary Industries, Wellington, New Zealand.
- Ministry for the Environment. 2022. New Zealand's Greenhuse Gas Inventory 1990-2020. Ministry for the Environment, Wellington, New Zealand.
- Molano G, Clark H. 2008. The effect of level of intake and forage quality on methane production in sheep. Australian Journal of Experimental Agriculture. 48:219–222. doi:10.1071/EA07253.
- O'Niell BF, Deighton MH, O'Loughlin BM, Galvin N, O'Donovan M, Lewis E. 2012. The effects of supplementing grazing dairy cows with partial mixed ration on enteric methane emissions and milk production during mid to late lactation. Journal of Dairy Science. 95:6582–6590. doi:10.3168/jds.2011-5257.
- O'Niell BF, Deighton MH, O'Loughlin BM, Mulligan FJ, Boland TM, O'Donovan M, Lewis E. 2011. Effects of a perennial rygrass diet or total mixed ration diet offered to spring-calving Holstein Friesian dairy cows on methane emissions, dry matter intake, and milk production. Journal of Dairy Science. 94:1941–1951. doi:10.3168/jds.2010-3361.
- Ray DK, West PC, Clark M, Gerber JS, Prishchepov AV, Chatterjee S. 2019. Climate change has likely already affected global food production. PLOS. 14:e0217148.
- Shibata M, Terada F. 2010. Factors affecting methane production and mitigation in ruminants. Animal Science Journal. 81:2–10. doi:10.1111/j.1740-0929.2009.00687.x.
- Sjaunja LO, Baevre L, Junkkarinen L, Pederson J, Setala J. 1991. A Nordic proposal for an energy correlated milk (ECM) formula, 156–157 in Proceedings of Performance Recording of Animals: State of the art. EAAP publication.
- Smith PE, Waters SM, Kenny DA, Kirwan SE, Conroy S, Kelly AK. 2021. Effect of divergence in residual methane emissions on feed intake and efficiency, growth and cascass performance, and incides of rumen fermentation and methane emissions in finishing beef cattle. Journal of Animal Science. 99:1–13.
- Starsmore K, Lopez-Villalobos N, Lahart B, Egan M, Burke J, Shalloo L. In Review. Animal factors that affect enteric methane production measured using the GreenFeed monitoring system in grazing dairy cows. Journal of Dairy Science (In Review).
- United Nations. 2016. Paris Agreement to the United Nations Framework Convention on Climate Change. Paris, France: United Nations.
- van Dijk M, Morley T, Rau ML, Saghai Y. 2021. A meta-analysis of projected global food demand and population at risk of hunger for the period 2010–2050. Nature Food. 2(7):494–501. doi:10.1038/s43016-021-00322-9.
- Waghorn GC, Hegarty RS. 2011. Lowering ruminant methane emissions through improved feed conversion efficiency. Animal Feed Science and Technology. 166:291–301. doi:10.1016/j.anifeedsci.2011.04.019.
- Waghorn GC, Jonker A, Macdonald KA. 2016. Measuring methane from grazing dairy cows using GreenFeed. Animal Production Science. 56(3):252. doi:10.1071/AN15491.
- Wiggins GR, Shook GE. 1987. A lactation measure of somatic cell count. Journal of Dairy Science. 70:266–267.
- Wims CM, Deighton MH, Lewis E, O'Loughlin BM, Delaby L, Boland TM, O'Donovan M. 2010. Effect of pregrazing herbage mass on methane production, dry matter intake and milk production of grazing dairy cows during mid season period. Journal of Dairy Science. 93:4976–4985. doi:10.3168/jds.2010-3245.
- Yan T, Mayne CS, Gordon FG, Porter MG, Agnew RE, Patterson DC, Ferris CP, Kilpatrick DJ. 2010. Mitigation of enteric methane emissions through improved efficiency of energy utilisation and productivity in lactating dairy cows. Journal of Dairy Science. 93:2630–2638. doi:10.3168/jds.2009-2929.