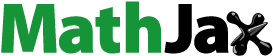
Abstract
Objectives
To determine the value of dual-sequence magnetic resonance imaging (MRI)-based radiomics in predicting the efficacy of high-intensity focused ultrasound (HIFU) ablation for hysteromyoma.
Methods
A total of 142 patients with 172 hysteromyomas (95 hysteromyomas from the sufficient ablation group, and 77 hysteromyomas from the insufficient ablation group) were enrolled in the study. The clinical–radiological model was constructed with independent clinical–radiological risk factors, the radiomics model was constructed based on the optimal radiomics features of hysteromyoma from dual sequences, and the two groups of features were incorporated to construct the combined model. A fivefold cross validation procedure was adopted to validate these models. A nomogram was constructed, applying the combined model in the training cohort. The models were assessed with receiver operating characteristic (ROC) curves and integrated discrimination improvement (IDI). An independent test cohort comprising 40 patients was used to evaluate the performance of the optimal model.
Results
Among the three models, the average areas under the ROC curves (AUC) of the radiomics model and combined model were 0.803 (95% confidence interval (CI): 0.726–0.881) and 0.841 (95% CI: 0.772–0.909), which were better than the clinical–radiological model in the training cohort. The IDI showed that the combined model had the best prediction accuracy. The combined model also showed good discrimination in both the validation cohort (AUC = 0.834) and the independent test cohort (AUC = 0.801).
Conclusion
The combined model based on the dual-sequence MRI radiomics is the most promising tool from our study to assist clinicians in predicting HIFU ablation efficacy.
Introduction
Hysteromyoma is a common benign tumor in women of reproductive age, with an incidence rate of more than 70% [Citation1]. Myoma originates from uterine smooth muscle tissue, and its growth depends on estrogen and progesterone [Citation2]. Twenty to 50% of patients have corresponding symptoms, such as menstrual disorders, abdominal pain and loss of fertility, which seriously reduce the quality of life of patients [Citation3,Citation4].
Common treatment options for hysteromyoma include hysterectomy, drug therapy and minimally invasive surgery [Citation5]. Even though hysterectomy remains a recognized treatment [Citation6], it has certain limitations. For example, the patient age must be considered when determining whether to perform this procedure. For patients who want to retain fertility, the radical surgery should be avoided. Due to the lack of sufficiently large sample sizes and high-quality studies, uncertainty persists in drug treatment and the possibility of adverse reactions after drug withdrawal [Citation4]. In minimally invasive surgery, myomectomy is currently the gold standard for the treatment of hysteromyoma in women who desire pregnancy. However, this surgery has a long convalescence. High-intensity focused ultrasound (HIFU) has the potential to become an alternative to myomectomy [Citation7]. The HIFU principle is to ablate the target tissue under the guidance of monitoring images by relying on the thermal and mechanical energy of ultrasonic waves [Citation8]. The purpose of this method to cause cell coagulation necrosis only at the focus within the target volume and minimize the damage to the surrounding and covered tissues [Citation9]. Numerous clinical trials have confirmed that HIFU has the advantages of safety, effectiveness and rapid recovery after treatment, especially for the patients who want to preserve the uterus or wish to remain fertile [Citation7,Citation10,Citation11]. The non-perfusion volume ratio (NPVR) is an important means to evaluate ablation efficacy. NPVR was associated with reduced myoma volume and improvement of symptoms during medium-long term follow-up [Citation12]. Therefore, the preoperative prediction of NPVR has great significance for accurately selecting patients and improving the success rate of HIFU treatment.
Previous studies have found that when myoma presents high T2-weighted imaging (T2) signal intensity and a high apparent diffusion coefficient (ADC) value, the NPVR is poor [Citation13,Citation14]. However, these traditional imaging parameters can be disturbed by many factors. Even for hysteromyoma with the same signal before operation, great differences in the NPVR persist after operation. As an imaging-based technology, radiomics uses computer algorithms to extract and analyze many quantitative features that cannot be recognized by the naked eye and provides spatial coding information about normal and abnormal tissue structure and function, which can be used in clinical decision support systems to improve the accuracy of diagnosis and prognosis [Citation15–17]. Recently, some studies have assessed the value of radiomics models based on nonenhanced magnetic resonance imaging (MRI) in predicting the efficacy of HIFU ablation for hysteromyoma [Citation18,Citation19].
Therefore, in this study, we aimed to develop and validate a combined model based on contrast-enhanced T1-weighted imaging (T1C) and T2 sequences for predicting the efficacy of HIFU ablation for hysteromyoma.
Materials and methods
Patients
This retrospective study was approved by the Institutional Review Board, and the requirement for informed consent was waived (IRB no. 2022ER101-1). From November 2019 to August 2021, 258 patients underwent hysteromyoma ablation at the HIFU center. The inclusion criteria were as follows: (I) premenopausal or perimenopausal women above 18 years old diagnosed with hysteromyoma by clinical and radiological examination; (II) patients undergoing their first HIFU ablation of hysteromyoma and having 10 or fewer hysteromyomas; (III) MRI examination within three days before and after ablation treatment; and (IV) no previous history of surgery or drug treatment. The exclusion criteria were as follows: (I) other uterine or accessory diseases; (II) lack of some MRI sequences or images; (III) poor MR image quality; and (IV) hysteromyomas with diameter ≤3 cm or diameter ≥10 cm [Citation20]. According to the inclusion and exclusion criteria, 142 eligible patients (with 172 hysteromyomas; 45 (42–48) years) were enrolled in the study cohort. From January 2020 to July 2022, 40 patients (with 40 hysteromyomas; 43 (34–46) years) in another group were screened according to the same criteria. The patient recruitment process is shown in .
MRI examination
The MR images of the training and validation cohorts were from a GE Healthcare 3T MRI scanner, and the MR images of the independent test cohort were from a Unite Imaging Healthcare 3T MRI scanner. All patients underwent both T1C and T2 examinations before surgery. The MRI sequences and parameters are presented in Supplementary Table S1. The contrast agent was injected intravenously at a dose of 0.1 mmol/kg and a flow rate of 2.0 ml/s. Early, middle and late arterial images were collected approximately 15 s, 30 s and 45 s after the contrast agent was injected.
Patient grouping
The volume (V) and non-perfusion volume (NPV) (NPVR = NPV/V × 100%) of hysteromyomas were calculated on preoperative and postoperative T1C images using the ellipsoid formula (V = 0.5233 × D1 × D2 × D3 [Citation21], where D1, D2 and D3 are the maximal longitudinal, anteroposterior and transverse diameters, respectively). Some studies have shown that the efficacy of HIFU ablation of hysteromyomas is related to the NPVR. The volume of hysteromyomas with an NPVR ≥70% is significantly reduced within one year after operation, and the cumulative clinical recurrence rate in two years is <10% (24, 25). Therefore, with NPVR = 70% as the boundary, hysteromyomas in the training and validation cohorts were divided into a sufficient ablation group (NPVR ≥70%, n = 95) and an insufficient ablation group (NPVR <70%, n = 77), and hysteromyomas in the test cohort were divided into a sufficient ablation group (NPVR ≥70%, n = 27) and an insufficient ablation group (NPVR <70%, n = 13).
Clinical–radiological features
Clinical–radiological features that might affect the NPVR of hysteromyomas were analyzed, including age, volume of hysteromyoma, type of hysteromyoma, T2 signal intensity of hysteromyoma (hypointensity: signal intensity equal to that of the skeletal muscle; isointensity: higher than that of the skeletal muscle but lower than that of the myometrium; hyperintensity: similar to or higher than that of the myometrium [Citation22]), enhancement degree of hysteromyoma (mild: lower than that of the myometrium; moderate: similar to that of the myometrium; obvious: higher than that of the myometrium [Citation22]), enhancement signal homogeneity of hysteromyoma and color Doppler flow imaging (CDFI) signal (small amount: Adler grade I, meaning that one or two short rods or punctate flow signals are observed; slightly abundant: Adler grade II or Adler grade III. Adler grade II implies that three to four punctate vessels are observed; in Adler grade III, more than four blood vessels, or the blood vessels are intertwined into a network are observed [Citation23]).
Tumor segmentation and radiomics feature extraction
3D Slicer (version 4.10.2, https://www.slicer.org) was used for tumor region of interest (ROI) segmentation and feature extraction. In the case of unknown hysteromyoma information, one radiologist manually segmented the preoperative T1C (late arterial) sequence images, and another radiologist manually segmented the preoperative T2 sequence images and delineated the hysteromyoma area ROI layer by layer, generating a 3D volume of interest (VOI) for hysteromyomas by fusing the ROIs of each layer of images. To evaluate the repeatability of radiomics features, approximately 1/3 of the images from the T1C and T2 sequences of all the hysteromyomas were randomly selected one month later, and the hysteromyomas were again delineated layer by layer by above two radiologists, respectively. The intergroup correlation coefficient (ICC) was used to evaluate the consistency between the measures. An ICC >0.75 was considered to indicate good consistency of radiomics features. The original images were transformed by Laplacian Gaussian filtering and wavelet transform filtering, and radiomic features of T1C and T2 were extracted from VOIT1C and VOIT2, respectively. A total of 1223 radiomic features were extracted from both VOIT1C and VOIT2, including first-order features, shape features, texture features (grey level cooccurrence matrix (GLCM), grey level dependence matrix (GLDM), grey level run length matrix (GLRLM), grey level size zone matrix (GLSZM) and neighboring grey tone difference matrix (NGTDM)). A total of 2446 features were extracted from the T1C and T2 images.
Feature selection
The R software (version 4.1.1, https://www.r-project.org) and uAI Research Portal (version 730) were used for feature screening. For the clinical–radiological features, univariate and multivariate logistic regression (LR) analyses were performed to test the correlation between clinical–radiological risk factors for hysteromyoma and the NPVR, and the features with the strongest correlation were screened as independent clinical–radiological risk factors. For the radiomics features of T1C and T2 images, Z score normalization was used to eliminate the dimensional effects of different features. Then, the variance threshold method was used to remove the features with variance lower than 0.8. The Select K Best method was then used to remove the features that did not significantly differ between the two groups. Finally, the least absolute shrinkage and selection operator (LASSO) was used to select the features highly correlated with the NPVR as the optimal radiomic features.
Model construction
A LR algorithm was used to establish three models: independent clinical–radiological risk factors were used to construct the clinical–radiological model, the optimal T1C and T2 features were used to construct the radiomics model, and three groups of features were incorporated to construct the combined model. These models comprehensively reflected the factors that influence the NPVR from different perspectives and compared and analyzed the ability of different models to predict the efficacy of HIFU ablation for hysteromyomas.
Model performance evaluation
A fivefold cross validation procedure was adopted to validate the model. The procedure randomly divided all hysteromyomas into five subgroups, including a validation cohort and a training cohort with four subgroups. The experiment was repeated five times. The receiver operating characteristic (ROC) curve was drawn, and the area under the ROC curve (AUC), sensitivity, specificity, accuracy and F1 score were calculated to quantify the predictive performance of each model for HIFU ablation of hysteromyomas in the training cohort and validation cohort. Integrated discrimination improvement (IDI) was used to compare the improvement degree of the prediction accuracy of the different models. A nomogram was constructed using the combined model based on LR in the training cohort. The Hosmer–Lemeshow (H–L) test evaluated the consistency between the actual HIFU ablation efficacy and the predicted HIFU ablation efficacy and was used to draw the calibration curves of the training cohort and validation cohort. Decision curve analysis (DCA) was performed to evaluate the clinical usefulness of the model by quantifying the net benefits at different threshold probabilities (). An independent test cohort was applied to test the performance of the optimal model.
Statistical analysis
The R language package (R Foundation for Statistical Computing, Vienna, Austria) and IBM SPSS Statistics 26.0 (Armonk, NY) were used for statistical analysis. Quantitative data are expressed as ( ± ѕ) or M (Q25 – Q75) and were compared with the independent sample t-test or Wilcoxon’s rank sum test, and qualitative data are expressed as percentages and compared with the Chi-square test or Fisher’s exact test. A two-sided p value of 0.05 was used to determine statistical significance.
Results
Clinical–radiological features
The clinical–radiological features of the study patients are shown in . In the training and validation cohorts, univariate analysis indicated that T2 signal intensity, CDFI signal and enhancement degree were significantly different between the sufficient ablation group and insufficient ablation group. Multivariate analysis indicated that T2 signal intensity (odds ratio, OR = 1.064; p = 0.044) and CDFI signal (OR = 0.045; p = 0.007) were independent clinical–radiological risk factors for predicting the NPVR ().
Table 1. Clinical–radiological features of patients in the training, validation and independent test datasets.
Table 2. Univariate and multivariate analyses results of clinical–radiological features.
Selection of radiomics features
In total, 2446 radiomics features were extracted for each patient. From these features, we selected 854 and 805 features with high consistency (both interobserver ICCs >0.75) for T1C and T2 images, respectively. After screening by the variance threshold method, selecting the best k method and the LASSO algorithm, 6 and 3 features remained in the T1C and T2 sequences, respectively (Supplementary Table S2).
Performance validation
The combined model yielded preferable predictive performance in both the training cohort (an average AUC = 0.841, 95% confidence interval (CI), 0.772–0.909) and validation cohort (an average AUC = 0.834, 95% CI, 0.691–0.971) compared with the clinical–radiological model (training cohort: an average AUC = 0.693, 95% CI, 0.611–0.775; validation cohort: an average AUC = 0.689, 95% CI, 0.525–0.853). The radiomics model also showed greater predictive performance than the clinical–radiological model in the training cohort (an average AUC = 0.803, 95% CI, 0.726–0.881), but not in the validation cohort (an average AUC = 0.794, 95% CI, 0.631–0.954).The IDI results showed that the combined model improved the prediction accuracy by 10.40% (training cohort, p < 0.001) and 14.1% (validation cohort, p < 0.001) compared with the clinical–radiological model, and it also improved the prediction accuracy by 2.9% (training cohort, p < 0.001) and 5.3% (validation cohort, p = 0.004) compared with the radiomics model (, and , Supplementary Table S3).
Figure 3. ROC curves of clinical–radiological models, radiomics models and combined models in the training (a, c, e) and validation (b, d, f) cohorts.

Table 3. Performance of clinical–radiological, radiomics and combined models in the training and validation cohorts.
Table 4. Comparison of models in the training and validation cohorts.
Development and verification of the nomogram
The nomogram was constructed with optimal radiomics features and clinical–radiological variables as a convenient visible tool to estimate the risk of HIFU (). The calibration curves of the combined model demonstrated good consistency between the predictive outcome and observation in the training and validation cohorts (p = 0.251) (). The DCA plot indicated that the combined model had the highest clinical net benefit with wider threshold probabilities compared with the other models (. If the threshold probability is between 0.06 and 0.8, the application of the combined model to predict the successful clinical outcome of HIFU treatment provides more net benefits than the ‘treat-all patients’ or the ‘treat-none’ method.
Figure 4. The combined nomogram for predicting the efficacy of HIFU for hysteromyoma (a). The developed nomogram based on the combined model to predict the risk of insufficient HIFU ablation. T2WI signal intensity: 1, hyperintensity; 2, isointensity; 3, hypointensity; CDFI signal: 1, small amount; 2, slightly abundant. Calibration curves for the nomogram in the training and validation cohorts (b, c). The black dashed line represents the ideal prediction, and the green, red and purple lines represent the predictive ability of clinical–radiological models, radiomics models and combined models. The closer they fit to the dashed green line, the greater the prediction accuracy of the model. Decision curve analysis for the nomogram (d). The black line represents the net benefit of assuming no hysteromyoma patients receive HIFU ablation. The grey line is the net benefit of assuming that all hysteromyoma patients receive HIFU ablation. The green line, red line and purple line represent the expected net benefit of predicting stroke outcome using the clinical–radiological models, radiomics models and combined models, respectively.

Independent test
The proposed preoperative combined model was tested with independent data, and the model performed well, with an AUC of 0.801 (sensitivity: 81.5%; specificity: 61.5%; accuracy: 75.0%).
Discussion
In this study, we used the LR classifier to develop and validate three models: the clinical–radiological model, radiomics model and combined model. According to the results, the predictive performance of the combined model outperformed those of the radiomics model and clinical–radiological model. To our knowledge, this study is the first to predict the efficacy of HIFU ablation for hysteromyoma based on T1C combined with T2 radiomics, which will help clinicians judge the difficulty of ablation before surgery, select an appropriate treatment plan for patients, and promote the development of personalized medicine.
Predicting the efficacy of HIFU based on traditional imaging parameters remains challenging. In our study, CDFI signal and T2 signal intensity were independent clinical–radiological risk factors for predicting the efficacy of HIFU ablation. However, the prediction efficiency of the clinical–radiological model based only on CDFI and T2 signal intensity was low. Although CDFI can reflect the vascular distribution and blood flow velocity of hysteromyoma and T2 signal intensity can reflect the density of hysteromyoma cells [Citation24], the qualitative features were greatly affected by the subjective factors of the observer in the evaluation, which increased the uncertainty of the prediction results. At the same time, these signals were also affected by tissue type, main magnetic field intensity and magnetic field uniformity.
Therefore, a quantitative and reliable model needs to be found to predict the ablation efficacy of HIFU. Our study found that the combined model showed greater predictive performance than the clinical–radiological model. We propose three reasons for this improvement. First, radiomics can reveal the heterogeneity of tumor spatial distribution, and texture features reflect the pathological changes of tissues to an extent [Citation25]. The small tissue differences caused by the pathological changes of a hysteromyoma in the image signal, shape and texture can be quantified by radiomics to overcome the subjectivity of image interpretation. Some studies have shown that radiomics may become a biomarker based on artificial intelligence to noninvasively capture the heterogeneity of hysteromyomas [Citation26]. Second, T2 sequencing is also an important basic sequence for the evaluation of hysteromyoma because its signal changes are related to the pathological classification of myomas [Citation27,Citation28]. Previous studies [Citation18] have confirmed the value of radiomics models based on T2 sequences in predicting the efficacy of HIFU. However, the T2 sequence could not directly reflect the blood flow of hysteromyoma and could not distinguish between degenerative myoma and cellular myoma-both growths showed high signals in the T2 sequence [Citation29]. Cellular myoma was resistant to HIFU, which made HIFU energy difficult to deposit, and the NPVR was consequently low. In contrast, the number and blood supply of degenerative myoma cells decreased, making them easier to ablate. In contrast-enhanced MRI (CE-MRI), the blood supply of cellular myoma was rich; therefore, the degree of enhancement was high, while the blood supply of degenerative fibroids was reduced, resulting in a low degree of enhancement. Due to the different textural features, cellular myoma and degenerative fibroids are easy to distinguish. According to Penne’s bioheat transfer equation, focused ultrasound energy is lost with blood flow, causing the target temperature to be lower than the ablation set temperature [Citation30,Citation31]. CE-MRI can directly reflect the perfusion and permeability of myoma vessels and has potential clinical value in predicting and evaluating the NPVR [Citation32]. Therefore, we included T1C sequences in this study to improve the characterization of myoma by the model, which might also be one of the reasons for the high efficiency of the combined model. Previous studies have also confirmed that the combination of sequences could fully reflect the information of tumors [Citation33,Citation34].
Although the predictive performance did not significantly differ between the combined model and the radiomics model, the IDI results showed that the prediction accuracy of the combined model was significantly improved. The IDI value reflects the overall improvement of the model. The nomogram based on the combined model showed good performance, calibration and clinical utility, with potential clinical significance in predicting the efficacy of HIFU ablation for hysteromyoma.
Several limitations to our study should be noted. First, this study had a single-center retrospective design with a small sample size. Before clinical application, we need to analyze a larger external dataset for further validation. Second, the laboratory index of patients was not recorded and analyzed with regard to clinical–radiological features. Finally, this study only extracted the features of late arterial images, which may limit the type and number of features. In the future, we intend to develop a multisequence radiomics model based on multicenter imaging and more clinical information.
Conclusion
The combined model based on dual-sequence MRI radiomics has the potential to predict the efficacy of HIFU ablation for hysteromyoma. In the future, further developing and verifying the model will help clinicians better screen patients who will benefit the most from HIFU treatment, provide a reference for treatment decision-making and allow for the formulation of accurate treatment plans.
Supplementary Tables
Download PDF (156.9 KB)Disclosure statement
No potential conflict of interest was reported by the author(s).
Data availability statement
The data used to support the findings of this study are available from the corresponding author upon request
Additional information
Funding
References
- Stewart EA, Cookson CL, Gandolfo RA, et al. Epidemiology of uterine fibroids: a systematic review. BJOG. 2017;124(10):1501–1512.
- Donnez J, Dolmans MM. Uterine fibroid management: from the present to the future. Hum Reprod Update. 2016;22(6):665–686.
- Giuliani E, As-Sanie S, Marsh EE. Epidemiology and management of uterine fibroids. Int J Gynaecol Obstet. 2020;149(1):3–9.
- De La Cruz MS, Buchanan EM. Uterine fibroids: diagnosis and treatment. Am Fam Physician. 2017;95(2):100–107.
- Wang Y, Zhang S, Li C, et al. Minimally invasive surgery for uterine fibroids. Ginekol Pol. 2020;91(3):149–157.
- Islam MS, Ciavattini A, Petraglia F, et al. Extracellular matrix in uterine leiomyoma pathogenesis: a potential target for future therapeutics. Hum Reprod Update. 2018;24(1):59–85.
- Anneveldt KJ, van 't Oever HJ, Nijholt IM, et al. Systematic review of reproductive outcomes after high intensity focused ultrasound treatment of uterine fibroids. Eur J Radiol. 2021;141:109801.
- Napoli A, Alfieri G, Andrani F, et al. Uterine myomas: focused ultrasound surgery. Semin Ultrasound CT MR. 2021;42(1):25–36.
- Lee KW. The Asian perspective on HIFU. Int J Hyperthermia. 2021;38(2):5–8.
- Jeng CJ, Ou KY, Long CY, et al. 500 cases of high-intensity focused ultrasound (HIFU) ablated uterine fibroids and adenomyosis. Taiwan J Obstet Gynecol. 2020;59(6):865–871.
- Marinova M, Ghaei S, Recker F, et al. Efficacy of ultrasound-guided high-intensity focused ultrasound (USgHIFU) for uterine fibroids: an observational single-center study. Int J Hyperthermia. 2021;38(2):30–38.
- Kim YS. Clinical application of high-intensity focused ultrasound ablation for uterine fibroids. Biomed Eng Lett. 2017;7(2):99–105.
- Sainio T, Saunavaara J, Komar G, et al. Feasibility of apparent diffusion coefficient in predicting the technical outcome of MR-guided high-intensity focused ultrasound treatment of uterine fibroids – a comparison with the Funaki classification. Int J Hyperthermia. 2021;38(1):85–94.
- Liao D, Xiao Z, Lv F, et al. Non-contrast enhanced MRI for assessment of uterine fibroids’ early response to ultrasound-guided high-intensity focused ultrasound thermal ablation. Eur J Radiol. 2020;122:108670.
- Wu G, Jochems A, Refaee T, et al. Structural and functional radiomics for lung cancer. Eur J Nucl Med Mol Imaging. 2021;48(12):3961–3974.
- Lafata KJ, Wang Y, Konkel B, et al. Radiomics: a primer on high-throughput image phenotyping. Abdom Radiol. 2021;47(9):2986–3002.
- Lambin P, Leijenaar R, Deist TM, et al. Radiomics: the bridge between medical imaging and personalized medicine. Nat Rev Clin Oncol. 2017;14(12):749–762.
- Zheng Y, Chen L, Liu M, et al. Nonenhanced MRI-based radiomics model for preoperative prediction of nonperfused volume ratio for high-intensity focused ultrasound ablation of uterine leiomyomas. Int J Hyperthermia. 2021;38(1):1349–1358.
- Zheng Y, Chen L, Liu M, et al. Prediction of clinical outcome for high-intensity focused ultrasound ablation of uterine leiomyomas using multiparametric MRI radiomics-based machine leaning model. Front Oncol. 2021;11:618604.
- Keserci B, Duc NM, Nadarajan C, et al. Volumetric MRI-guided, high-intensity focused ultrasound ablation of uterine leiomyomas: ASEAN preliminary experience. Diagn Interv Radiol. 2020;26(3):207–215.
- Orsini LF, Salardi S, Pilu G, et al. Pelvic organs in premenarcheal girls: real-time ultrasonography. Radiology. 1984;153(1):113–116.
- Wei C, Li N, Shi B, et al. The predictive value of conventional MRI combined with radiomics in the immediate ablation rate of HIFU treatment for uterine fibroids. Int J Hyperthermia. 2022;39(1):475–484.
- Che D, Yang Z, Wei H, et al. The Adler grade by Doppler ultrasound is associated with clinical pathology of cervical cancer: implication for clinical management. PLOS One. 2020;15(8):e236725.
- Zhao WP, Chen JY, Chen WZ. Effect of biological characteristics of different types of uterine fibroids, as assessed with T2-weighted magnetic resonance imaging, on ultrasound-guided high-intensity focused ultrasound ablation. Ultrasound Med Biol. 2015;41(2):423–431.
- Mayerhoefer ME, Materka A, Langs G, et al. Introduction to radiomics. J Nucl Med. 2020;61(4):488–495.
- Bortolotto C, Lancia A, Stelitano C, et al. Radiomics features as predictive and prognostic biomarkers in NSCLC. Expert Rev Anticancer Ther. 2021;21(3):257–266.
- Huang H, Ran J, Xiao Z, et al. Reasons for different therapeutic effects of high-intensity focused ultrasound ablation on excised uterine fibroids with different signal intensities on T2-weighted MRI: a study of histopathological characteristics. Int J Hyperthermia. 2019;36(1):477–484.
- Oguchi O, Mori A, Kobayashi Y, et al. Prediction of histopathologic features and proliferative activity of uterine leiomyoma by magnetic resonance imaging prior to GnRH analogue therapy: correlation between T2-weighted images and effect of GnRH analogue. J Obstet Gynaecol (Tokyo 1995). 1995;21(2):107–117.
- Zhao WP, Chen JY, Chen WZ. Dynamic contrast-enhanced MRI serves as a predictor of HIFU treatment outcome for uterine fibroids with hyperintensity in T2-weighted images. Exp Ther Med. 2016;11(1):328–334.
- Andreozzi A, Brunese L, Iasiello M, et al. Modeling heat transfer in tumors: a review of thermal therapies. Ann Biomed Eng. 2019;47(3):676–693.
- Pennes HH. Analysis of tissue and arterial blood temperatures in the resting human forearm. 1948. J Appl Physiol (1985). 1998;85(1):5–34.
- Keserci B, Duc NM. The role of T1 perfusion-based classification in magnetic resonance-guided high-intensity focused ultrasound ablation of uterine fibroids. Eur Radiol. 2017;27(12):5299–5308.
- Hou L, Zhou W, Ren J, et al. Radiomics analysis of multiparametric MRI for the preoperative prediction of lymph node metastasis in cervical cancer. Front Oncol. 2020;10:1393.
- Zhao Y, Wang N, Wu J, et al. Radiomics analysis based on contrast-enhanced MRI for prediction of therapeutic response to transarterial chemoembolization in hepatocellular carcinoma. Front Oncol. 2021;11:582788.