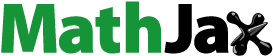
Abstract
Purpose
MR thermometry (MRT) enables noninvasive temperature monitoring during hyperthermia treatments. MRT is already clinically applied for hyperthermia treatments in the abdomen and extremities, and devices for the head are under development. In order to optimally exploit MRT in all anatomical regions, the best sequence setup and post-processing must be selected, and the accuracy needs to be demonstrated.
Methods
MRT performance of the traditionally used double-echo gradient-echo sequence (DE-GRE, 2 echoes, 2D) was compared to multi-echo sequences: a 2D fast gradient-echo (ME-FGRE, 11 echoes) and a 3D fast gradient-echo sequence (3D-ME-FGRE, 11 echoes). The different methods were assessed on a 1.5 T MR scanner (GE Healthcare) using a phantom cooling down from 59 °C to 34 °C and unheated brains of 10 volunteers. In-plane motion of volunteers was compensated by rigid body image registration. For the ME sequences, the off-resonance frequency was calculated using a multi-peak fitting tool. To correct for B0 drift, the internal body fat was selected automatically using water/fat density maps.
Results
The accuracy of the best performing 3D-ME-FGRE sequence was 0.20 °C in phantom (in the clinical temperature range) and 0.75 °C in volunteers, compared to DE-GRE values of 0.37 °C and 1.96 °C, respectively.
Conclusion
For hyperthermia applications, where accuracy is more important than resolution or scan-time, the 3D-ME-FGRE sequence is deemed the most promising candidate. Beyond its convincing MRT performance, the ME nature enables automatic selection of internal body fat for B0 drift correction, an important feature for clinical application.
1. Introduction
In hyperthermia (HT) treatments, tumor tissue temperature is increased to sensitize it for other forms of cancer treatment such as radio-, chemo- and immunotherapy. Real-time temperature feedback during HT treatments is essential, as clinical outcome depends heavily on the temperature reached [Citation1]. The target treatment temperature usually lies between 39 and 43 °C. The temperature achieved in the region of interest (ROI) is commonly measured using temperature probes that are placed intraluminally or punctured into tissue in, or close to, the target volume. These probes provide temperature information for a limited region, and may sometimes be difficult or even unfeasible to place [Citation2]. MR thermometry (MRT) promises to enable 3D noninvasive, real-time temperature measurement during HT treatments. These temperature maps could visualize the temperature in the target volume and identify hot- and cold-spots during treatments, hence enabling the optimization of treatment quality. Ultimately, good quality thermal dosimetry will facilitate enhanced understanding of the relationship between target temperature and treatment outcome. Hence, MRT carries the potential to make HT treatments more repeatable, safer and more effective for the patient.
In MR imaging, many temperature sensitive magnetic properties can be exploited for temperature measurement. Proton resonance frequency shift (PRFS) is the most frequently used, and considered the most accurate method [Citation3], as it varies linearly over a large temperature range and is independent of tissue type (with the exception of fat) [Citation4]. Measuring the temperature induced changes in the local resonance frequency by phase mapping may be confounded by other changes in the magnetic field occurring simultaneously, such as magnetic field drift [Citation5]. Hence, even though MRT is relatively straight forward and regularly used when performing HT in patients with static tumors such as sarcoma [Citation6], it’s much more challenging in body sites where more movement can be expected e.g., abdomen, thorax and head&neck [Citation7].
The scientific and clinical communities agree on the importance of having accurate and reliable MRT measurements [Citation8]. The desired performances have been clearly defined, but as explained above, successful MRT is largely dependent on the treatment location [Citation9,Citation10]. One way to improve MRT is by increasing the SNR, leading to better precision of the phase maps. This is largely made possible by novel MR HT devices that position multiple receiver coils close to the body [Citation11]. Other strategies to improve MRT include various motion correction techniques and faster imaging; these are comprehensively discussed in Yuan et al. [Citation12].
For the brain, motion is not a primary concern. Hence, in preparation of developing motion confounder corrections for other body sites, this location is ideal for testing which sequence will provide accurate temperature mapping. Consequently, the results can be of general value also when performing MRT in other (more challenging) anatomies, because the effect of the sequences are not being intermixed with motion effects. As such, any difference in error that will be encountered in other anatomies can be seen as advantages and drawbacks that can be expected in that particular anatomy, rather than a general trend of the sequence. High precision MRT in the brain has been demonstrated in the past [Citation13,Citation14], using the help of field monitoring to correct for non-temperature induced frequency shift. While this method gives good results, it is expensive and thus not an option for most institutions. Also, the MRT approaches presented don’t have sufficient spatiotemporal resolution to monitor temperature during thermal therapies [Citation14].
The only clinical standard pulse sequence available for MRT is the DE-GRE sequence [Citation15,Citation16], which hence is the baseline for comparison in this paper. DE-GRE has been compared to other pulse sequences before [Citation17,Citation18], but never on a broader scale. Also multi-echo sequences have been used to calculate MRT, but often rely on creating a library of images as a baseline [Citation19]. This takes additional time for imaging at the start of the experiment. Cheng et al. [Citation20] developed a dual-step iterative temperature estimation algorithm, that improved both accuracy and precision. However, this only applies to areas where fat fractions are between about 10% and 90%. In areas with homogeneous water and fat distributions, fat-referenced thermometry has been shown to work well [Citation21].
In this work, we compare the performance of different clinically available ME-GRE sequences for MRT. PRFS MRT performance, especially accuracy, is investigated in phantom and unheated volunteers. By investigating MRT in the brain, we aim to demonstrate the possible improvements also for more challenging treatment areas, whilst keeping the door open for further developments that could build on these results. The sequence used for clinical MRT monitoring during HT at most institutions (including ours) is double-echo gradient-echo, DE-GRE (two echoes, 2D). We hypothesize that alternative multi-echo gradient-echo sequences might allow better monitoring of HT treatments than DE-GRE, and therefore compare it to two other multi-echo sequences: ME-FGRE (11 echoes, 2D) and 3D-ME-FGRE (11 echoes, 3D) [Citation22]. MRT of the sequences having more than two echoes are being evaluated with a multi-peak and multi-echo fitting method developed previously: MMT-PRFS [Citation23] (see Appendix A.1).
2. Materials and methods
2.1. Phantom design
A phantom was created with different fat percentages, containing T1 and T2 ranges similar to what is found in human tissue [Citation24], following the recipe by Bush et al. [Citation25] (see ). The water mixture (0% fat), positioned in the center, is the only one being evaluated in this study. It consists of a mixture of distilled water, gadolinium-diethylenetriaminepentacetate (DTPA) contrast agent, water-soluble surfactant, agar, and sodium benzoate, and thus solidifies. The mixtures were filled in 50 ml Polypropylene conical vials (30 × 115 mm) and placed in a PVC pipe with a lid. The space between tube and pipe was filled with distilled water. Preliminary experiments of the different mixtures excluded susceptibility artifacts and evaluated SNR, and T1 and T2 values. Subsequently, catheters were added to facilitate easy insertion of temperature probes. The temperature probes are part of our PYREXAR BSD2000-3D-MRI deep HT system, and they serve as the ground truth temperature measurements. According to the vendor specification, the thermistor type sensors are non-perturbing and electromagnetically insensitive with an accuracy of ±0.2 °C over a range of 25 to 52 °C [Citation26].
2.2. Theory/method
Post-processing was conducted offline in MATLAB and SPSS. gives an overview of the post-processing pipeline that we developed, and the steps are described below in more detail. The code is publicly available on GitLab: https://gitlab.com/radiology/quantitative-mri/mr-thermometry.
Figure 2. Flow chart of post-processing of experimental data. More detailed information on the methods in general can be found in the text, and specifically on MMT-PRFS in Appendix A.1.

Figure 3. Example fat mask (of bone marrow) with volunteer 1, slice 1. The fat mask is overlaid on the magnitude image of echo 1 at time point 2.

2.2.1. Inputs
The acquisition of sequences was repeated at multiple time points and the input into the pipeline differed between the sequences. The ME acquisitions were automatically reconstructed by the scanner into DICOM images of the real and imaginary components. For the DE-GRE acquisition the raw multi-channel k-space data was imported into MATLAB. In order to combine the k-space data for the individual coils, for each time point, one set of coil sensitivity maps is obtained by applying the ESPIRiT method [Citation27]. The method was applied to each slice, with a calibration area spanning the center 40% of k-space in both in-plane dimensions and using all echoes in the calibration matrix. More details on the corrections applied for DE-GRE images from phantom experiments are described in Appendix A.2.
2.2.2. Image registration
For volunteers it was in hindsight deemed necessary to register the coil-combined complex valued data, to correct for subject motion along the time points. This was deemed especially important for performing the B0 drift correction (Section 2.2.4) using the thin fat layer, see the Supplementary Material for support of this. For our experiments subject motion was not restricted, however patients motion will be severely constrained by the water bolus of the HT device. To compensate motion, we performed a rigid pair-wise image registration of the magnitude images using Elastix [Citation28].Footnote1 Subsequently the found deformation was applied to the complex valued images. Since the 2D acquisitions had a slice gap, and phase consistency across slices was not ensured, image registration could only be reliably applied in in-plane directions. However, 3D registration was used to quantify the z-motion for all data; scans for which the motion in the z-direction exceeded 20% of the slice thickness were excluded.
2.2.3. Change in off-resonance
For the DE-GRE acquisitions in volunteers both echoes were used, to correct for other not-temperature-related changes such as the conductivity [Citation29]. The change in off-resonance of water only voxels, varies lineally with temperature and can be described by EquationEquation (1)
(1)
(1) :
(1)
(1)
where
is the phase at the time point in question,
is the phase at the reference time point r (here,
is used) and TE is the echo time of the acquisition.
For the ME-FGRE and 3D-ME-FGRE sequence MMT-PRFS was used to calculate the change in off-resonance frequency, previously introduced by Salim et al. [Citation23]. This proposed model is described in detail in Appendix A.1.
2.2.4. B0 drift correction
In order to ensure that the MR temperature reading is not influenced by the B0 field drift of the scanner, a drift correction needs to be applied to all acquired images. Fat experiences a much smaller temperature related off-resonance frequency shift than water and tissues [Citation30]; in the order of α=−1.8*10−10/°C [Citation31], compared to α = 1.0*10−8/°C of water [Citation32]. Therefore, as fat still experiences all other disturbances of the magnetic field, it can be used for field drift correction. Firstly, fat voxels need to be identified. For the phantom experiments, ROIs were drawn in the external fat tubes; For the volunteer data, we developed an automatic fat selection similarly to [Citation33], selecting the internal body fat based on the water- and fat- density maps at zero echo time obtained from MMT-PRFS, see . For DE-GRE, the fat mask could not be calculated with the same method and was hence imported from the ME-FGRE acquisition. The process is illustrated with example images and described in more detail in Appendix A.3.
2.2.5. MRT
Once is calculated, the change in temperature can be found by EquationEquation (2)
(2)
(2) :
(2)
(2)
where γ is the gyromagnetic ratio, α is the change coefficient for PRFS, and B0 is the magnetic field strength of the MRI scanner. The constants used were γ = 267.513*106 rad/s/T, α=−0.01 ppm/°C and B0=1.5T.
To mitigate a possible bias at higher temperatures, the phantom results are also calculated for the clinical temperature range of 37–43 °C, referencing to the time point in each sequence that was closest to and above 37 °C.
Since our method relies on the changes in the resonance frequency in the hydrogen of water molecules, we exclude voxels containing more than 20% fat as in these voxels the off-resonance frequency estimate might be biased. This selection was made using the fat and water density maps, which contain the signal at zero echo time:
Additionally voxels with low SNR were excluded; we defined those voxels with of the mean
of the three voxels with the highest
in that time point and slice.
2.2.6. MRT performance quantities
To give a complete comparison of MRT performance for different sequences, we followed the standard suggested in [Citation9] in order to calculate performance measures for the phantom experiments, i.e., reporting on bias (ME), spatial temperature standard deviation (spatial SD), temporal temperature standard deviation (temporal SD) and accuracy (MAE). The definitions with their respective formulas are shown in .
Table 1. MRT performance quantities used for comparison between sequences.
The acquired MRT measurements for the phantom experiment were compared to the ‘ground truth’ obtained from the temperature probes. The slice that was evaluated with MRT corresponded to the depth of the temperature probe. The ROI had a diameter of about 12 pixels (18.0 mm), resulting in 107 voxels selected. This was drawn as large as possible whilst excluding the wall of the vial ().
Figure 4. ROIs chosen for MRT evaluations. (A) shows the ROI for the phantom: only the vial in the center containing water mixture was selected; and (B) for the volunteers: encompassing an elliptical volume of brain tissue, as large as possible while constant among all volunteers.

For the MRT performance assessment in volunteers an elliptical ROI was drawn in the brain. The ROI was made as large as possible, while keeping it constant for all volunteers ().
Accuracy in volunteers was calculated similarly to the accuracy as described in : the mean deviation from zero of the apparent temperature change in the ROI for each slice. The mean absolute deviation of each slice was then averaged over all slices and all volunteers.
SD in the volunteer data was calculated as the standard deviation of temperatures measured in each ROI evaluated (one for each slice) and then averaged over all slices and volunteers, just as the spatial precision in the volunteer experiments, defined in .
The statistical significance of the differences among the sequences is calculated in SPSS, using a two-sided t-test (p < .05).
2.3. Experiments
All experiments were conducted using a 1.5 T MR scanner (GE Healthcare, Waukesha, WI, USA) and a 22-channel GE Head&Neck coil. For comparison between sequences, we aimed to match them as closely as possible to DE-GRE, with the settings used in the clinic for MRT in the pelvis, regarding the following properties: range of echo times, spatial resolution and the number of echoes for the ME sequences. Additionally, the SNR estimate for each set-up, as indicated by the command window before scanning, was matched as well. The sequences run for both experiments were DE-GRE (TR620), DE-GRE (TR200), ME-FGRE and 3D-ME-FGRE. Scans were run continuously and the FOVs were copied between the sequences so that they covered the same area. All MRT images were acquired with a spatial coverage of 192 × 192 mm2 and resolution 1.5 × 1.5 × 5 mm3.
The SNR of the sequences were measured to be 42 for DE-GRE (both TRs), 47 for MEFGRE, and 64 for 3D-ME-FGRE.
2.3.1. Phantom
The water in between the vials with the different mixtures was replaced with hot water (at 70 °C) and a temperature probe was inserted into the vial with the water mixture via the catheter. Two experiments were run, both spanning a time of about 205 min, where the MR sequences were played out continuously whilst the phantom was slowly cooling down. In the first experiment all sequences were run, but only the DE sequences produced usable results, due to an error in the reconstruction of the ME coil-combined images from the scanner. Hence, for the second experiment, ME-FGRE and 3D-ME-FGRE were run again leading to more measurement points.
The acquisition parameters for different sequences are presented in .
Table 2. Acquisition parameters and scan times for different sequences for the phantom experiment.
2.3.2. Volunteers
All volunteers signed an informed consent (protocol MEC-2014-096, approved by the Erasmus MC review board) and were screened prior to entering the MR room. For imaging, the volunteers were placed in the scanner in supine position with the head coil around the head. In order to investigate the MRT accuracy in-vivo, we acquired brain images from a total of ten volunteers. Detailed acquisition parameters are shown in .
Table 3. Parameters of all the sequences as well as their respective scan times for the volunteer experiment using the multi-channel GE head coil (22 channels).
Table 4. MRT performances from the multi-coil phantom experiment for the whole temperature range (top) and the clinically HT range 37–43 °C (bottom).
3. Results
3.1. Phantom cooling-down experiment
shows example MRT maps of the phantom cooling down over time. We excluded low SNR voxels as well as voxels with more than 20% fat, as temperature estimates are unreliable in those voxels with the current method. Note that some temperature errors at the edges of the phantom and the tubes with water-fat mixtures remain.
displays the temperature changes measured by MRT (as the mean of the ROI evaluated) against the temperature measured by the probe for each time point. The time points in A are referenced to the first time point acquired during the experiment at the highest temperature, and shows the entire temperature range of the experiment (205 min). B only presents the HT clinical temperature range of 37–43 °C, and the MRT measurements are referenced to the time point closest to 37 °C, as would be the case during treatment, spanning 65 min.
Figure 6. Temperature measured by MRT (mean of the ROI evaluated ± SD) vs. the temperature measured by the thermometer probe during the cooling down of the phantom experiment. Each dot represents a temperature reading at a distinct time point for which the acquisition time from the MRI scanner is taken. The dashed line illustrates the perfect scenario, where the temperature of the probe and the temperature measured with the MRI are matching. A shows the entire temperature range, referenced to the first time point acquired during the experiment; and B shows only the clinical temperature range of 37–43 °C, referenced to the time point closest to (and above) 37 °C. The bias, spatial SD, temporal SD and accuracy are presented in for both the extended range and the reduced treatment relevant range.

The performances from the phantom experiment are summarized in . Considering the whole temperature range of the experiment, ME-FGRE had the best accuracy and bias of 0.46 °C and −0.08 °C. Spatial SD was only sufficient for the DE-GRE sequences and the temporal SD was sufficient in all. Regarding the clinical temperature range of 37–43 °C, the 3D-ME-FGRE sequence performed best regarding bias, spatial SD and accuracy.
3.2. Volunteer experiment
shows example MRT estimations for one volunteer for all sequences. Also here, low SNR voxels as well as voxels with more than 20% fat were excluded, as temperature estimates are unreliable in those voxels with the current method. It can be seen, that 3D-ME-FGRE estimates the temperature change more homogenous across the slice, as well as closer to 0 °C than the other pulse sequences.
The MRT performances for the volunteer experiments in brain are presented in . One volunteer and one ME-FGRE acquisition of another volunteer was excluded because the motion in the slice direction was larger than 20% of the slice thickness. Excluding individual slices due to too little fat voxels being selected for a B0 drift correction was necessary in two volunteers and resulted in excluding a total of six slices for both DE-GRE sequences, five slices for ME-FGRE and four slices for 3D-ME-FGRE. The effect of the exclusion criteria is shown in .
Figure 8. Accuracy achieved for all sequences in the volunteer experiments, including all scans and excluding scans with too much motion and too few fat voxels (9/10 volunteers remaining). Accuracy was calculated as mean absolute deviation over all slices of the mean deviation from zero of the apparent temperature change in the ROI for each slice.

Table 5. MRT performances averages of 10 volunteers in brain over all slices, including all and excluding motion and too little fat voxels.
When testing for the statistical significance of the improvements of the accuracy after exclusion, DE-GRE TR200 and ME-FGRE were non-significant (p = .415 and p = .088, respectively). 3D-ME-FGRE, however, shows a significant improvement in accuracy compared to the original DE-GRE TR620 sequence, with p < .001. Regarding the SD over the investigated ROI, the multi-echo sequences achieved better values after exclusion, though these improvements were not significant.
An aspect that stood out, was that with 3D-ME-FGRE, only 5% of slices had to be excluded due to selecting too few fat voxels, compared to up to 30% for other sequences.
4. Discussion
In this work the MRT performance of different multi-echo gradient-echo sequences was compared, both in a phantom and in volunteers. This study was aimed to be a first step in improving PRFS-MRT to a more reliable and robust monitoring modality for HT treatments.
The analysis in volunteers presented here was performed only in brain, an ideal testing location due to little motion disturbances, although subject motion had to be compensated, see also the Supplementary Material for an illustration of this. However, we believe that the results can also be useful when applied to other anatomies. This is because when moving to more challenging areas in the future, we know that any difference in behavior and the likely increase in temperature errors will be caused by motion and other magnetic field disturbances, and not be due to the sequences and settings themselves. In other words: it is precisely because the effects of the sequences are not being intermixed with big adjustments in the analysis, that they will likely be helpful when considering different anatomical regions.
Accuracy is the most important measure investigated, enabling reliable detection of small temperature increases during the relatively long HT treatment times. ME-FGRE and 3D-ME-FGRE achieve better accuracy and bias than DE-GRE in phantom over the whole temperature range, consequently satisfying the minimum requirement for successful monitoring during a HT treatment. This improvement in performance was expected, due to the increased number of echoes, which provide more data to estimate the off-resonance frequency. The smaller echo spacing of ME-FGRE also means that we can have a larger bandwidth, which leads to less problems with wrap-around of the phase when dealing with large temperature changes (due to larger ranges being able to unambiguously be identified). This will also affect the B0 compensation positively, especially when fat is sparsely distributed.
Considering the (smaller) clinical temperature range, the errors decrease and all investigated sequences satisfy the minimum requirements. The best accuracy in the clinical temperature range was obtained with 3D-ME-FGRE – representing a clinically relevant and statistically significant improvement of 46% compared to DE-GRE TR620. The trends observed in phantoms is mirrored by the measurements in volunteers. We expected the multi-echo sequences to achieve better (lower) values of SD’s compared to the standard DE-GRE, and indeed observe up to a 22% improvement (not statistically significant). Most impressive is the 61.7% statistically significant increase in accuracy shown by 3D-ME-FGRE compared to the standard DE-GRE TR620 sequence. In general, the reduced range is more indicative of the thermometry performance that can be expected in the clinic – also because it spans a shorter cooling time (65 min, much closer to the HT treatment time) and comparable effects of B0 drift can be expected.
The possibility to implement automatic fat map selection is an advantage of using multi-echo sequences, making the addition of external fat references superfluous. In current clinical MR-compatible hyperthermia applicators, external fat references are attached to the wall of the applicator. As a result, the fat references are not places close to the subject, but often at the boundary of the imaging FOV. Internal body fat selection provides the opportunity to simplify treatment set-up and reduce the FOV for MRT, therefore shortening treatment time. Additionally it provides a reference as close as possible to the tissue of interest, likely improving accuracy of the field drift correction. Additional benefits include the saving the time of manual delineation of fat, as is common practice when using body fat as a fat reference [Citation16,Citation34], and which can be a very time consuming task [Citation15].
One of the explanations for the robustness of 3D-ME-FGRE is the sequence’s echo spacing, which is optimized for detecting and quantifying fat by the three-point Dixon method [Citation35], more so than the 2D implementation, making 3D-ME-FGRE superior when selecting the fat mask for reliable B0 drift corrections; something that is of particular importance for the clinic, where the scanner drift can be substantial during the long treatment times of >60 min. This robustness is reflected in the lower amount of slices that had to be excluded (5%) due to selecting too few fat voxels, compared to other sequences (up to 30%). Furthermore, 3D-ME-FGRE is acquiring in 3D, and hence has 3D phase consistency. This gives an advantage for the HT application, because it would enable estimating the field drift only once for the whole volume, rather than for every slice, which is the case for the 2D sequences.
In regards of the results of both phantoms and volunteers, it is noteworthy that DE-GRE TR200 performs as well as the original DE-GRE TR620, whilst the acquisition time is shortened by more than 65%. The accuracy over both temperature changes investigated are similar, and the difference in accuracy in volunteers is not significant. If there is a dependency in clinical scenarios on using a DE-GRE sequence, reducing the TR would provide an easy way to reduce scan time without compromising MRT performance.
Whilst this study presents a comprehensive comparison of different performance metrics, there are some limitations that need to be mentioned. The study only analyses four different sequences with particular acquisition settings, which were matched as closely as possible to the clinically used DE-GRE, but may not be optimized even for imaging the brain, as was done here.
The automatic fat selection method that we constructed is able to select reliable fat voxels for the B0 drift correction. However, sometimes there are not enough fat voxels selected toward the outer edges of the acquisition volume. It may not always be feasible or desirable to acquire a larger volume to avoid these effects, thus improvements in the fat selection method could benefit the HT application. Regarding this aspect, 3D acquisitions could prove beneficial due to the consistency of the off-resonance frequency among slices.
With some volunteers, we observed substantial motion in the z-direction. Since the 2D acquisitions had a slice gap, 3D image registration was not possible and sets of data were excluded where z-motion was exceeding our criteria. We expect that this exclusion of data due to prominent motion is not going to be an issue during HT treatments, as such motion will be restricted by the water bolus of the HT applicator. Of course, for 3D sequences, motion could be compensated in all three dimensions, potentially providing an additional benefit in HT.
Image processing has been performed offline, and is not yet optimized for performing temperature monitoring during HT treatments in real-time. When undertaking HT treatments with physical probes, the time for transferring the data and analysis takes 1–2 min. Thus, the response time for adjusting the heating is in the order of minutes and relying heavily on patient feedback, due to the point-like temperature readings of the probe. With MRT we can achieve a 3D temperature distribution and potentially react to hot spots before the discomfort of the patient, as well as improving temperature coverage.
5. Conclusion
Different multi-echo gradient-echo sequences were assessed for their MRT performance in a phantom and volunteers. 3D-ME-FGRE offers a statistically significant improvement in accuracy of 62% and 46% in unheated volunteers and temperature varying phantom (in the range of 37.0 °C–43.0 °C), respectively, compared to the clinical standard DE-GRE sequence. Thus, 3D-ME-FGRE presents a promising improvement to noninvasive MR temperature monitoring that can be implemented promptly. We believe this to be the first essential step toward improved MRT for HT treatments and anticipate to further investigate this 3D multi-echo sequence for MRT by optimizing the acquisition settings and corresponding post-processing method.
Ethical approval
The protocol used was approved by our institutional review board, protocol 'MRI technology healthy volunteers’ (MEC-2014-096).
Consent form
After having received an explanation of the study, all volunteers signed an informed consent.
Supplemental Material
Download PDF (170.9 KB)Acknowledgements
We would like to acknowledge Loes Priester for her help with making phantom, Samy Abo Seada for his code for automatic sorting DICOM images, Sergio Curto Ramos for his help setting up the phantom experiments and Iva Vilas Boas Ribeiro for her help setting up and conducting the volunteer experiments.
Disclosure statement
No potential conflict of interest was reported by the author(s).
Correction Statement
This article has been corrected with minor changes. These changes do not impact the academic content of the article.
Additional information
Funding
Notes
1 2D parameter file ‘parameters_rigid.txt’ and 3D parameter file ‘parameters_rigid_3D.txt’ at https://github.com/tfeddersen/ElastixModelZoo.
References
- Kroesen M, Mulder HT, van Holthe JML, et al. Confirmation of thermal dose as a predictor of local control in cervical carcinoma patients treated with state-of-the-art radiation therapy and hyperthermia. Radiother Oncol. 2019;140:150–158.
- Wust P, Cho CH, Hildebrandt B, et al. Thermal monitoring: invasive, minimal-invasive and non-invasive approaches. Int J Hyperthermia. 2006;22(3):255–262.
- Wlodarczyk W, Hentschel M, Wust P, et al. Comparison of four magnetic resonance methods for mapping small temperature changes. Phys Med Biol. 1999;44(2):607–624.
- Ishihara Y, Calderon A, Watanabe H, et al. A precise and fast temperature mapping using water proton chemical shift. Magn Reson Med. 1995;34(6):814–823.
- Bing C, Staruch RM, Tillander M, et al. Drift correction for accurate PRF-shift MR thermometry during mild hyperthermia treatments with MR-HIFU. Int J Hyperthermia. 2016;32(6):673–687.
- Unsoeld M, Lamprecht U, Traub F, et al. MR thermometry data correlate with pathological response for soft tissue sarcoma of the lower extremity in a single center analysis of prospectively registered patients. Cancers. 2020;12(4):959.
- VilasBoas-Ribeiro I, Curto S, van Rhoon GC, et al. MR thermometry accuracy and prospective imaging-based patient selection in MR-guided hyperthermia treatment for locally advanced cervical cancer. Cancers. 2021;13(14):3503.
- Rieke V, Pauly KB. MR thermometry. J Magn Reson Imaging. 2008;27(2):376–390.
- Feddersen TV, Hernandez-Tamames JA, Franckena M, et al. Clinical performance and future potential of magnetic resonance thermometry in hyperthermia. Cancers. 2020;13(1):31.
- Kothapalli SVVN, Altman MB, Zhu L, et al. Evaluation and selection of anatomic sites for magnetic resonance imaging-guided mild hyperthermia therapy: a healthy volunteer study. Int J Hyperthermia. 2018;34(8):1381–1389.
- Adibzadeh F, Sumser K, Curto S, et al. Systematic review of pre-clinical and clinical devices for magnetic resonance-guided radiofrequency hyperthermia. Int J Hyperthermia. 2020;37(1):15–27.
- Yuan J, Mei CS, Panych LP, et al. Towards fast and accurate temperature mapping with proton resonance frequency-based MR thermometry. Quant Imaging Med Surg. 2012;2(1):21–32.
- Le Ster C, Mauconduit F, Mirkes C, et al. RF heating measurement using MR thermometry and field monitoring: methodological considerations and first in vivo results. Magn Reson Med. 2021;85(3):1282–1293.
- Le Ster C, Mauconduit F, Mirkes C, et al. Measuring radiofrequency field‐induced temperature variations in brain MRI exams with motion compensated MR thermometry and field monitoring. Magn Reson Med. 2022;87(3):1390–1400.
- Gellermann J, Hildebrandt B, Issels R, et al. Noninvasive magnetic resonance thermography of soft tissue sarcomas during regional hyperthermia: correlation with response and direct thermometry. Cancer. 2006;107(6):1373–1382.
- Gellermann J, Wlodarczyk W, Hildebrandt B, et al. Noninvasive magnetic resonance thermography of recurrent rectal carcinoma in a 1.5 tesla hybrid system. Cancer Res. 2005;65(13):5872–5880.
- Wu M, Mulder HT, Zur Y, et al. A phase-cycled temperature-sensitive fast spin echo sequence with conductivity bias correction for monitoring of mild RF hyperthermia with PRFS. MAGMA. 2019;32(3):369–380.
- Dadakova T, Gellermann J, Voigt O, et al. Fast PRF-based MR thermometry using double-echo EPI: in vivo comparison in a clinical hyperthermia setting. MAGMA. 2015;28(4):305–314.
- Poorman ME, Braškutė I, Bartels LW, et al. Multi‐echo MR thermometry using iterative separation of baseline water and fat images. Magn Reson Med. 2019;81(4):2385–2398.
- Cheng C, Zou C, Wan Q, et al. Dual‐step iterative temperature estimation method for accurate and precise fat‐referenced PRFS temperature imaging. Magn Reson Med. 2019;81(2):1322–1334.
- Hofstetter LW, Yeo DTB, Dixon WT, et al. Fat‐referenced MR thermometry in the breast and prostate using IDEAL. J Magn Reson Imaging. 2012;36(3):722–732.
- Reeder SB, Pineda AR, Wen Z, et al. Iterative decomposition of water and fat with echo asymmetry and least‐squares estimation (IDEAL): application with fast spin‐echo imaging. Magn Reson Med. 2005;54(3):636–644.
- Salim G, Poot D, Numan W, et al., editors. Multipeak multiecho modeling for improved PRF-based thermometry. 32nd Annual Scientific Meeting of the ESMRMB; 1–3 October 2015; Edinburgh, UK.
- Ikemoto Y, Takao W, Yoshitomi K, et al. Development of a human‐tissue‐like phantom for 3.0‐T MRI. Med Phys. 2011;38(11):6336–6342.
- Bush EC, Gifford A, Coolbaugh CL, et al. Fat-water phantoms for magnetic resonance imaging validation: a flexible and scalable protocol. J Vis Exp. 2018;7(139):57704.
- Products & Services – An overview of Pyrexar’s line of hyperthermia medical devices for the treatment of cancer. Pyrexar Medical; [cited 2021 07-Dec]. Available from: https://www.pyrexar.com/hyperthermia/product-overview.
- Uecker M, Lai P, Murphy MJ, et al. ESPIRiT—an eigenvalue approach to autocalibrating parallel MRI: where SENSE meets GRAPPA. Magn Reson Med. 2014;71(3):990–1001.
- Klein S, Staring M, Murphy K, et al. Elastix: a toolbox for intensity-based medical image registration. IEEE Trans Med Imaging. 2010;29(1):196–205.
- Peters RD, Henkelman RM. Proton-resonance frequency shift MR thermometry is affected by changes in the electrical conductivity of tissue. Magn Reson Med. 2000;43(1):62–71.
- Hindman JC. Proton resonance shift of water in the gas and liquid states. J Chem Phys. 1966;44(12):4582–4592.
- Kuroda K, Oshio K, Mulkern RV, et al. Optimization of chemical shift selective suppression of fat. Magn Reson Med. 1998;40(4):505–510.
- Carter DL, MacFall JR, Clegg ST, et al. Magnetic resonance thermometry during hyperthermia for human high-grade sarcoma. Int J Radiat Oncol Biol Phys. 1998;40(4):815–822.
- Zhang L, Armstrong T, Li X, et al. A variable flip angle golden-angle-ordered 3D stack-of-radial MRI technique for simultaneous proton resonant frequency shift and T1-based thermometry. Magn Reson Med. 2019;82(6):2062–2076.
- Winter L, Oberacker E, Paul K, et al. Magnetic resonance thermometry: methodology, pitfalls and practical solutions. Int J Hyperthermia. 2016;32(1):63–75.
- Glover GH. Multipoint dixon technique for water and fat proton and susceptibility imaging. J Magn Reson Imaging. 1991;1(5):521–530.
- Yu H, Shimakawa A, McKenzie CA, et al. Multiecho water‐fat separation and simultaneous R estimation with multifrequency fat spectrum modeling. Magn Reson Med. 2008;60(5):1122–1134.
- Poot DHJ, Klein S. Detecting statistically significant differences in quantitative MRI experiments, applied to diffusion tensor imaging. IEEE Trans Med Imag. 2014;34(5):1164–1176.
Appendix A
A.1. MMT-PRFs
In this section, the multi-peak multi-echo thermometry with PRFS, referred to as MMT-PRFS, will be described in more detail.
A.1.1. Signal model
Yu et al. [Citation36] proposed a signal model to describe the measured signal of a voxel containing a mixture of water and fat in a multi-echo GRE sequence. However, this signal model doesn’t take the initial phase offset into account. We hypothesized that neglecting
may hinder accurate PRFS based thermometry, and thus use the following extended model to describe the signal of a voxel for MMT-PRFS:
(3)
(3)
where
and
are the density values of water and fat;
is the total number of fat frequency peaks;
is the relative amplitude of the kth fat peak with
is the frequency shift of the kth fat peak relative to that of water;
is the echo time;
is the off-resonance frequency; and
is the effective relaxation rate of the transverse magnetization. The values for
were taken from from [Citation36], as [0.62 0.15 0.10 0.06 0.03 0.04], corresponding to frequencies (at 1.5 T) of [210 159 ‒ 47 236 117 23] Hz.
A.1.2. Maximum likelihood estimator
Based on the work of Poot et al. [Citation37], we developed a maximum likelihood estimator (MLE) to simultaneously fit
and
to the complex-valued measurements of multiple echoes with arbitrary echo times.
Let denote a vector containing the tissue parameters to be estimated (
) at voxel
and let
represent an array containing the vectors
of all voxels. Let
denote the measured signal of voxel
in image
Since every image corresponds to a single echo time,
is substituted by
in the rest of this section.
To distinguish between the real and the estimated values we use the notation and
respectively. The estimation procedure uses the model
described in EquationEquation (4)
(4)
(4) to predict the intensity of a voxel
in an image
The MLE procedure is defined as the following minimization problem:
(4)
(4)
where
is the likelihood function of the data from a single measurement, for which we used a complex-valued Gaussian distribution, and
is the spatial domain (region of interest) in which the fit is performed.
For each voxel, the maximum likelihood estimation begins with a grid search, with 2 values of 101 values of
and 6 values of
For all combinations of
and
the least squares solution of
and
was implicitly obtained, and the combination with the lowest residual norm was used for subsequent non-linear optimization. The initialization range was determined based on the expected values of the five parameters. The outcome of the algorithm is not very sensitive to the initialization, but a reasonable initialization can improve the processing time.
A.2. Coil sensitivity correction details
This section described the coil sensitivity corrections that were put in place for the phantom DE-GRE experiments, when only using the second echo.
The individual coil sensitivity maps at every time point maximize SNR of the images (and would reduce artifacts in accelerated scans). However, they can differ (slightly) in phase so that the phase of the reconstructed images may vary spatially – within the smoothness constraints on the coil sensitivity maps. This is because the measurements depend on the product of coil sensitivity maps with the images and not on the phase images directly. However, as the temperature change measurements are based on phase changes, it is relevant to minimize the phase differences among the coil sensitivity maps of different time points.
EquationEquation (5)(5)
(5) shows how this is accomplished: by adjusting the phase of the maps to be as close as possible to the phase of the coil sensitivity map of the reference time point. Specifically, for each voxel x and time point j:
(5)
(5)
where
is a column vector with the uncorrected coil sensitivity maps (for every channel) of a location
and time point
is a column vector with the coil sensitivity maps at the reference time point r (here,
=1 is used), H is the complex conjugate transpose and
is a column vector with the corrected coil sensitivity maps.
In order to obtain the complex valued images for all time points, firstly we multiply the complex conjugate of the corrected coil sensitivity maps with the Fourier transform of the fully sampled k-space data. The summation over all channels of that multiplication is equal to the coil-combined complex valued images.
A.3. Internal body fat selection
This section describes in more detail how the internal body fat was selected for the volunteers:
Initial mask: An initial fat mask selects voxels in which the apparent density of fat
is at least four times higher than that of water
and where the fat density is larger than 10% of the maximum:
(6)
(6)
Elimination of water-fat swapped voxels: Sometimes, especially around tissue boundaries, the water and fat components can be swapped. These voxels can visually easily be spotted, as they vary greatly from their correctly identified neighbors. In order to identify these swapped voxels and eliminate them from the fat mask, we applied Gaussian blurring with a sigma of 4 voxels in-plane to the change in off-resonance frequency map
The swapped voxels are then identified as where the absolute difference between the filtered off-resonance frequency and the unfiltered off-resonance frequency is bigger than 200 rad/s (31Hz). These voxels are subsequently excluded from the fat selection.
Remove low SNR voxels: A signal constraint was also added in order to avoid selecting nonfat voxels in regions of very low signal, that can be missed in step 1. It’s effect can be seen in , going from subplot 2 to subplot 3. For the good fat-water contrast in the magnitude image, the second echo was chosen for DE-GRE (19.2 ms). For the other two sequences the echo closest to 19.2ms was selected. For every time point and every slice, the signal is taken as the mean of the three voxels with the highest signal. Voxels that are less than 10% of that signal threshold, are excluded from the fat mask.
Eliminating residual outliers & B0 drift field: A linear bias field is fitted within the fat pixels of the current mask of the change in off-resonance frequency map
This method is described in detail in Glover et al. [Citation35]. The difference between
and the predicted regression on the fat voxels in the current fat mask should not be very large and abruptly changing. This property can be exploited to identify and exclude outliers in the fat mask by applying a threshold to the absolute difference (chosen to be 100 rad/s (16 Hz)). These steps are iterated over all time points and slices until all the outliers have been removed. The resulting fat mask is updated and used to calculate the linear bias field. To obtain the field-drift corrected change in off-resonance maps the bias field is subtracted from
Figure A1. Example fat mask selection (of bone marrow) with volunteer 1, slice 1, (echo 1 of magnitude image), at time point 2. (1) Shows the initial mask, (2) eliminating fat-water swapped voxels, (3) including a signal threshold and (4) eliminating the residual outliers which is at the same time also the final fat mask. For this particular volunteer there were no residual outliers, so step 3 to 4 was superfluous.
