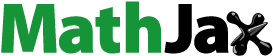
Abstract
Objectives
To compare metal artifacts and evaluation of metal artifact reduction algorithms during probe positioning in computed tomography (CT)-guided microwave ablation (MWA), cryoablation (CRYO), and radiofrequency ablation (RFA).
Materials and methods
Using CT guidance, individual MWA, CRYO, and RFA ablation probes were placed into the livers of 15 pigs. CT imaging was then performed to determine the probe’s position within the test subject’s liver. Filtered back projection (B30f) and iterative reconstructions (I30-1) were both used with and without dedicated iterative metal artifact reduction (iMAR) to generate images from the initial data sets. Semi-automatic segmentation-based quantitative evaluation was conducted to estimate artifact percentage within the liver, while qualitative evaluation of metal artifact extent and overall image quality was performed by two observers using a 5-point Likert scale: 1-none, 2-mild, 3-moderate, 4-severe, 5-non-diagnostic.
Results
Among MWA, RFA, and CRYO, compared with non-iMAR in B30f reconstruction, the largest extent of artifact volume percentages were observed for CRYO (11.5–17.9%), followed by MWA (4.7–6.6%) and lastly in RFA (5.5–6.2%). iMAR significantly reduces metal artifacts for CRYO and MWA quantitatively (p = 0.0020; p = 0.0036, respectively) and qualitatively (p = 0.0001, p = 0.0005), but not for RFA. No significant reduction in metal artifact percentage was seen after applying iterative reconstructions (p > 0.05). Noise, contrast-to-noise-ratio, or overall image quality did not differ between probe types, irrespective of the application of iterative reconstruction and iMAR.
Conclusion
A dedicated metal artifact algorithm may decrease metal artifacts and improves image quality significantly for MWA and CRYO probes. Their application alongside with dedicated metal artifact algorithm should be considered during CT-guided positioning.
Introduction
Percutaneous image-guided thermal therapy is an established therapy for local tumor control, especially in hepatocellular carcinoma and liver metastasis [Citation1–5]. Thermal ablation has also been applied for the ablation of benign tumors such as hepatic adenomas to prevent malignant transformation and hemorrhage [Citation6,Citation7]. Heat-based ablation such as radiofrequency ablation (RFA) or microwave ablation (MWA) ideally induces irreversible coagulation necrosis within the ablation zone. In contrast, the tissue outside the ablation zone should be left unaffected by the treatment as the diffusion thermal energy in this tissue could lead to damage of neighboring structures, e.g., vessels and bile ducts [Citation2]. Cryoablation (CRYO) leads to direct damage in the cell structures and also causes cell dehydration. The importance of the probe position for obtaining an adequate ablation zone has been shown in clinical settings. A homogeneous safety margin >5 mm is proposed to achieve the best local tumor control after CT-guided radiofrequency ablation of colorectal cancer liver metastases [Citation8]. Also, a safety margin ≥6 mm has been demonstrated to be crucial in patients with KRAS mutation [Citation9]. Therefore, the exact positioning of the probe is required, even more for overlapping ablations and for better control of the induced coagulation area due to image artifact reduction.
Computed tomography guidance is a common method for monitoring procedures that require probe positioning within the body. CT guidance is especially useful for large lesions needing overlapping ablations, those with long puncture tracts, or lesions in close proximity to critical structures. Metal artifacts induced by the probe itself can obscure puncture pathways and result in an insufficient visualization of small liver lesions, increasing false-negative biopsy by as much as 12.8% compared to lesions that were clearly visible [Citation10]. Metal artifacts consisting of dark and bright streaks occur around the probe tip and are the results of photon starvation as alloys of thermal ablation probes with higher atomic numbers absorb X-ray photons leading to loss of image data. The extent of metal artifacts in CT-guided biopsy may be influenced by, e.g., needle alloy, needle configuration, and needle thickness [Citation11]. Especially artifacts at the probe tip hamper the visualization of the liver parenchyma and vessel structures in the direction of the puncture pathway. Dedicated iterative metal artifact reduction algorithms have been shown to significantly reduce the artifact extent and improve the depiction of anatomical and pathological structures in musculoskeletal radiology and neuroradiology [Citation12–15]. These algorithms have also shown to be applicable for CT-guided interventions [Citation16]. One method is the sinogram in-painting method, in which a threshold-based algorithm segments the metal objects and removes them from the sinogram. The missing data is then replaced with the help of linear interpolation. The corrected sinogram and image are obtained via a weighted combination of filtering and multiple repetitions of these algorithms. Thus, the study aims to evaluate metal artifact extent in different ablation probes and whether postprocessing algorithms would sufficiently reduce metal artifacts during CT-guided ablation.
Materials and methods
The study was conducted with the approval of the local and governmental animal care committee instituted by the German government. The ethics committee approved this study as a part of other trials.
Animal preparation
General anesthesia and intubation were performed in 15 landrace pigs for breath control using azaperone, ketamine, and midazolam. Isoflurane was used for the maintenance of anesthesia. An ablation probe was positioned into the right liver lobe under CT guidance in the axial plane and at a depth of 5 cm. The probe placement was performed in the end-expiratory phase during a pause in breathing to minimize motion artifacts. Commercially available MWA probe (Emprint MWA System Probe, Medtronic Covidien, Mansfield, MA, USA), RFA probe (Covidien Cool tip, Medtronic Covidien, Mansfield, MA, USA), and CRYO (IceEdge 2.4, Boston Scientific, Marlborough, Massachusetts, USA) were used. Each probe type was placed in 5 different animals.
Image acquisition and reconstruction
An initial non-contrast CT series was performed for the orientation of the animal’s anatomy and the planning of the probe placement. After the first puncture procedure, several non-contrast CT acquisitions were performed to evaluate the probe placement and the effects of metal artifact caused by the probe. Non-contrast CT scans were performed using a dual-energy, dual-source 64-MDCT scanner (Somatom Definition Flash, Siemens Healthineers, Forchheim, Germany). CT acquisitions were conducted at a tube voltage of 120 kVp with a fixed tube current of 200 mA resulting in a CTDIvol of 13.40 mGy. Other parameters were as follows: pitch 0.6, collimation 64 × 0.6 mm, gantry rotation time 0.5 s, and scan length (5 cm) were identical for all acquisitions. Four different reconstruction algorithms were conducted: standard filtered back projection with soft tissue kernel B30f and iterative reconstruction with ADMIRE I30-1 (Siemens Healthineers, Erlangen, Germany) and each with and without the application of a dedicated iterative metal artifact reduction algorithm (iMAR, Siemens Healthineers, Erlangen, Germany) spine screw at level 1 out of 3. Images were reconstructed with a slice thickness of 2 mm and an increment of 1 mm.
Quantitative image analysis
For the objective and comprehensive quantification of artifacts, a threshold based, 3-dimensional, semiautomatic segmentation was performed using the free available software Medical Imaging Interaction Toolkit [Citation17,Citation18]. Manual segmentation of the liver parenchyma was performed in 10 axial slices to determine the reference values of the reference liver parenchyma for threshold calculation for each animal (). The reference liver volume was at least 20 slices from the probe tip and did not contain any visible artifacts.
Table 1. Mean CT number of normal liver tissue, standard deviation, and calculated upper and lower thresholds in Hounsfield units.
Upper and lower thresholds were defined as three standard deviations from the mean attenuation value of liver density. Within these thresholds, the cumulative distribution should comprise 99.73% of liver tissue without metal artifacts with individually calibrated density. The remaining tissue 0.27% with CT numbers outside the thresholds is considered as artifact. The volume of interest of the liver was set with 20 axial slices with the probe tip in the middle axial slice, which contains the greatest extent of photon starvation artifacts presented as dark streaks obscuring the liver tissue in the direction of the puncture pathway and hamper correct probe positioning. Artifacts within the volume of interest were automatically segmented by applying the calculated lower and upper threshold (). Voxels containing attenuation values beyond the thresholds were identified as artifacts. A correction factor was calculated for each reconstruction for each animal separately to compensate for tissue misclassification and subtracted from the artifact volume to obtain the final artifact volumes. The correction factor calculation avoids a bias due to the reconstruction algorithm. For each animal, liver reference volume and liver volume of interest was transferred to all reconstructed images of the same animal to maintain consistency among the different reconstructions. This results in an added benefit of reducing workload as each individual reconstruction did not need to be segmented.
Figure 1. Semiautomatic segmentation steps. The volume of liver tissue without artifacts shown in red (A); The volume of interest encompassing ablation probe and artifacts within the liver in black (B); Voxels below the lower threshold in blue (C); Voxels above the upper threshold in brown (D); Union of voxels beyond the thresholds (E); Intersection of the volume of interest of the liver with the union of voxels beyond the thresholds resulting in artifact volume shown in yellow (F). Thresholds were calculated for each animal separately.

The final artifact percentage for each animal was calculated as follows, as suggested in previous work [Citation16]
The noise was calculated using the standard deviation of the liver reference volume.
The contrast-to-noise ratio was calculated (CNR) as follows:
Qualitative image analysis
Two independent observers with 8 and 9 years of experience in diagnostic and interventional radiology performed the analyses. Observers were blinded for acquisition and reconstruction techniques using randomized images. Qualitative analysis were evaluated on a standard diagnostic workstation (Picture Archive and Communication System Centricity, GE, Boston, MA, USA). Image evaluation criteria that were taken into consideration were overall image quality, artifacts at the probe tip, artifact extent in the liver parenchyma in the puncture direction of the probe, and noise using a 5-point Likert scale [Citation13]. Metal artifacts were classified as 0 - no artifacts, 1- marginal artifacts, 2-minor dark streaks < 2 cm, 3- distinct dark streaks > 2cm, 4- extensive artifacts hampering further puncture pathway planning. Examples of the evaluation of the metal artifact extent in the liver parenchyma are shown in . Secondary artifacts generated by the dedicated iterative metal artifact reduction algorithm were classified as none − 1, mild − 2 either around the probe tip or at the image periphery, moderate − 3 around the probe tip and at the image periphery, and severe − 4 distinct artifacts with non-diagnostic quality. The default for the evaluation was a standard abdomen window center of 40 HU and window width of 400 HU.
Statistical analysis
Statistical analysis was performed using Prism (Prism, 7.0b, GraphPad Software, USA). A non-normal distribution of the data was assumed. The ANOVA Friedman test was used to compare different reconstructions, and different probe types. For correction of multiple comparisons, a post hoc Dunn–Bonferroni test was additionally applied. For the pair-wise comparison of iMAR vs. non-iMAR images, Wilcoxon-matched-pairs signed rank test was used. Statistical significance was considered at p < 0.05. For the inter-reader agreement of qualitative analysis, Cohen’s kappa and Landis and Koch classification were applied [Citation19].
Results
Quantitative image analysis
Artifact volumes for the RFA probes were between 3663.4–4162.9 mm3, for MWA probes 4031.4–5627.3 mm3, and CRYO probes 112286.9–17860.3 mm3. Correction factors were calculated ranging from 1.53E-3% to 0.85% based on misclassified liver tissue fraction within the liver volume of interest.
The highest artifact volume percentages were observed for the CRYO probes (11.5–17.9%). MWA probes in comparison, had an artifact volume percentage of 4.7– 6.6%, while RFA probes had a volume percentage of 5.5–6.2% ().
Table 2. Mean values of artifact volumes, correction factors, and final artifact volume percentages of quantitative analysis.
Using iMAR, a significant reduction of artifact volumes was seen for the MWA probe (p = 0.0036; yellow boxes) and the CRYO probe (p = 0.0020) ( green boxes; ). No significant artifact reduction was observed for RFA using iMAR ( blue boxes).
Figure 3. Metal artifact shown in yellow in different ablation probes MWA (A,B), RFA (C,D) and CRYO (E,F) without iMAR (upper row) and with iMAR (bottom row).

Table 3. Comparisons of metal artifact extent in different ablation probes are given in p-values with significant reduction of artifact volumes for the MWA probe (yellow boxes) and CRYO probe (green boxes). No significant artifact reduction was observed for RFA (blue boxes).
Image noise and contrast-to-noise ratio
For all three ablation probes, the noise was on average higher for iMAR than non-iMAR images regardless of the iterative reconstruction. Additionally, with the exception of RFA probe B30 iMAR vs. I30-1, the difference in noise amongst the different probes and iterative reconstruction types did not reach significance levels (). No significant difference was seen for CNR between the different probe types, iterative reconstruction, and iMAR application ()
Figure 4. Box plots of noise (A) and contrast-to-noise (B) with median and upper and lower quartiles. No significant difference was seen between the ablation probes for noise and CNR. iMAR did not show significantly higher noise than non-iMAR images. No significant difference in CNR was found between probes, iterative reconstruction, or iMAR application.

Qualitative image analysis
Metal artifacts
The subjective estimation of metal artifacts shows that the extent of overall metal artifacts was significantly greater in images without iMAR when compared to those with iMAR (p < 0.0001) for all types of ablation probes. The reduction of metal artifacts using iMAR was significantly higher for CRYO and MWA probes in comparison to the RFA probe (p = 0.0001, p = 0.0005) (). No significant differences in the metal artifact extent were observed after the application of iterative reconstruction i.e., B30 vs. I30-1 and B30 iMAR vs. I30-1 iMAR for all ablation probes.
Table 4. Mean of subjective evaluation of overall metal artifacts and standard deviation to the 1st and 3rd percentiles in parentheses.
Overall image quality
Taking delineation of liver parenchyma, liver vessels, aorta, and noise without metal artifacts into consideration, the overall image quality did not significantly differ between reconstruction type iterative vs. non-iterative, iMAR vs. non-iMAR images and type of ablation probe (0.16≤p ≤ 1).
Secondary artifacts generated by iMAR
Secondary artifacts were observed in all iMAR images regardless of probe type without any significant difference amongst the probe types (0.13≤p ≤ 1). Blooming artifacts along the ablation probe and streak artifacts at the image periphery were noted ().
Interreader-agreement
Cohen’s kappa of the inter-reader agreement for the qualitative analysis was substantial, with 0.68, according to Landis and Koch [Citation18].
Discussion
The results of this study showed that iMAR reduces metal artifacts for MWA and CRYO probes in the quantitative and qualitative evaluation. No significant metal artifact reduction effects were seen for RFA. iMAR did not show any significant influence on image noise or CNR.
To the best of our knowledge, there are no previous studies in the literature evaluating the extent to which metal artifacts affect ablation probe placement. When choosing the proper ablation probe, specific parameters need to be taken into consideration. This choice depends on aspects such as tumor size, type, location, ablation device availability, proper insertion pathway, and secure positioning of the ablation probe itself [Citation2,Citation20]. However, different ablation probes cause a varying degree of metal artifacts which has yet not been evaluated. Dedicated metal artifact reduction algorithms have been shown to effectively reduce artifacts in the ablation of hepatic and pulmonary metastases in human and animal models [Citation16,Citation21,Citation22]. The artifact reduction algorithms have shown to be feasible for MWA, which was also confirmed in this study [Citation23]. MWA probe types were also specifically taken into account due to their increasingly preferential use in comparison to RFA, due to their shorter ablation time and their larger resulting ablation zone [Citation24]. Therefore, the use of metal artifact reduction algorithm is worth considering when performing CT-guided positioning of an ablation probe. This study shows effective metal artifact reduction for CRYO and MWA probes but not for the RFA probe. This might be explained by the observation that CRYO and MWA probes produce more metal artifacts on CT images as the surfaces of these two probe types differ from that of RFA as they both contain cooling systems.
Interestingly, this study also showed that model-based iterative reconstruction neither reduced metal artifacts, nor noise, nor improved CNR. These findings are in accordance with data published in a previous study [Citation25]. Optimization of noise and CNR would be essential to ensure better delineation of a liver lesion but was not the main object of the study. Other ways to mitigate metal artifacts are reducing the collimation and decreasing the detector spacing, while the tube peak voltage and current should be increased. However, the effect of e.g., increasing tube voltage is less effective than iMAR in reducing metal artifacts [Citation16]. The radiation dose and decreasing soft tissue contrast limit an arbitrary increase in tube voltage.
In contrast to other methods, the approach of metal artifact evaluation shown in this study is a more comprehensive evaluation of metal artifacts as it captures them in three dimensions. Thus, the quantitative assessment allows metal artifacts to be assessed independently of their puncture angle. Puncture pathways coplanar to the axial orientation are easier to perform, but streak artifacts usually spread along the projection axis of the most enormous metal mass.
This study does have certain limitations. Firstly, no thermal ablation was performed before CT-imaging, and thus, no ablation is available for image quality evaluation. Additionally, the tests were performed in an animal model and not on the human objects. Further studies should include the artifact reduction post thermal ablation application and in a larger group of people. Moreover, the dedicated metal artifact reduction algorithm used in this work was from one individual vendor. Currently, similar dedicated metal artifact reduction algorithms can be purchased from many CT vendors. Finally, one drawback of the method for semiautomatic quantitative metal artifact evaluation is the missing differentiation between dark streak artifacts and blooming artifacts. In the case of these specific artifact types, further development of the artifact segmentation method is required. It is conceivable to apply the technique of metal artifact quantification to any other organs e.g., bone. Still, this study has focused on metal artifacts within the liver since the consideration of other organs seems subordinate in liver ablation procedures.
Conclusion
In summary, a dedicated iterative metal artifact reduction algorithm showed the benefits of MWA and cryoablation, reducing the extent of metal artifact and improving image quality CT images during CT-guided thermal ablations.
Ethical approval
The study was performed according to rules outlined by the local and governmental animal care committee instituted by the German government. The ethics committee approved this study as a part of other trials.
Author contributions
The corresponding author declares that all authors have read the manuscript and have given permission to submit the work in its current version. All authors have made substantial contributions to conception and design of the study, acquisition data, or analysis and interpretation of data; drafting the article or revising it.
Disclosure statement
No potential conflict of interest was reported by the author(s).
Additional information
Funding
References
- Ahmed M, R. Technology Assessment Committee of the Society of Interventional. Image-guided tumor ablation: standardization of terminology and reporting criteria – a 10-year update: supplement to the consensus document. J Vasc Interv Radiol. 2014;25(11):1706–1708.
- Hinshaw JL, Lubner MG, Ziemlewicz TJ, et al. Percutaneous tumor ablation tools: microwave, radiofrequency, or cryoablation–what should you use and why? Radiographics. 2014;34(5):1344–1362.
- Vogl TJ, Farshid P, Naguib NNN, et al. Thermal ablation of liver metastases from colorectal cancer: radiofrequency, microwave and laser ablation therapies. Radiol Med. 2014;119(7):451–461.
- Vogl TJ, Dommermuth A, Heinle B, et al. Colorectal cancer liver metastases: long-term survival and progression-free survival after thermal ablation using magnetic resonance-guided laser-induced interstitial thermotherapy in 594 patients: analysis of prognostic factors. Invest Radiol. 2014;49(1):48–56.
- Yin X-Y, Xie X-Y, Lu M-D, et al. Percutaneous thermal ablation of medium and large hepatocellular carcinoma: long-term outcome and prognostic factors. Cancer. 2009;115(9):1914–1923.
- Carnevale A, Pellegrino F, Cossu A, et al. Current concepts in ablative procedures for primary benign liver lesions: a step forward to minimize the invasiveness of treatment when deemed necessary. Med Oncol. 2020;37(4):31.
- Cheng Z, Liang P, Yu X, et al. Percutaneous microwave ablation for benign focal liver lesions: initial clinical results. Oncol Lett. 2017;13(1):429–434.
- Wang X, Sofocleous CT, Erinjeri JP, et al. Margin size is an independent predictor of local tumor progression after ablation of Colon cancer liver metastases. Cardiovasc Intervent Radiol. 2013;36(1):166–175.
- Shady W, Petre EN, Vakiani E, et al. Kras mutation is a marker of worse oncologic outcomes after percutaneous radiofrequency ablation of colorectal liver metastases. Oncotarget. 2017;8(39):66117–66127.
- Stattaus J, Kuehl H, Ladd S, et al. CT-guided biopsy of small liver lesions: visibility, artifacts, and corresponding diagnostic accuracy. Cardiovasc Intervent Radiol. 2007;30(5):928–935.
- McWilliams SR, Murphy KP, Golestaneh S, et al. Reduction of guide needle streak artifact in CT-guided biopsy. J Vasc Interv Radiol. 2014;25(12):1929–1935.
- Kotsenas AL, Michalak GJ, DeLone DR, et al. CT metal artifact reduction in the spine: can an iterative reconstruction technique improve visualization? AJNR Am J Neuroradiol. 2015;36(11):2184–2190.
- Morsbach F, Bickelhaupt S, Wanner GA, et al. Reduction of metal artifacts from hip prostheses on CT images of the pelvis: value of iterative reconstructions. Radiology. 2013;268(1):237–244.
- Morsbach F, Wurnig M, Kunz DM, et al. Metal artefact reduction from dental hardware in carotid CT angiography using iterative reconstructions. Eur Radiol. 2013;23(10):2687–2694.
- Bier G, Bongers MN, Hempel J-M, et al. Follow-up CT and CT angiography after intracranial aneurysm clipping and coiling-improved image quality by iterative metal artifact reduction. Neuroradiology. 2017;59(7):649–654.
- Do TD, Heim J, Skornitzke S, et al. Single-energy versus dual-energy imaging during CT-guided biopsy using dedicated metal artifact reduction algorithm in an in vivo pig model. PLoS One. 2021;16(4):e0249921.
- Wolf I, Vetter M, Wegner I, et al. The medical imaging interaction toolkit. Med Image Anal. 2005;9(6):594–604.
- Do TD, Sommer CM, Melzig C, et al. A novel method for segmentation-based semiautomatic quantitative evaluation of metal artifact reduction algorithms. Invest Radiol. 2019;54(6):365–373.
- Landis JR, Koch GG. The measurement of observer agreement for categorical data. biometrics. 1977;33(1):159–174.
- Vogl TJ, Farshid P, Naguib NNN, et al. Ablation therapy of hepatocellular carcinoma: a comparative study between radiofrequency and microwave ablation. Abdom Imaging. 2015;40(6):1829–1837.
- Do TD, Heim J, Melzig C, et al. Virtual monochromatic spectral imaging versus linearly blended dual-energy and single-energy imaging during CT-guided biopsy needle positioning: optimization of keV settings and impact on image quality. PLoS One. 2020;15(2):e0228578.
- Wang Y, Qian B, Li B, et al. Metal artifacts reduction using monochromatic images from spectral CT: evaluation of pedicle screws in patients with scoliosis. Eur J Radiol. 2013;82(8):e360-6–e366.
- Do TD, et al. The value of iterative metal artifact reduction algorithms during antenna positioning for CT-guided microwave ablation. Int J Hyperthermia. 2019;36(1):1223–1232.
- Wright AS, Sampson LA, Warner TF, et al. Radiofrequency versus microwave ablation in a hepatic porcine model. Radiology. 2005;236(1):132–139.
- Nattenmüller J, Hosch W, Nguyen T-T, et al. Hypodense liver lesions in patients with hepatic steatosis: do we profit from dual-energy computed tomography? Eur Radiol. 2015;25(12):3567–3576.