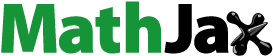
Abstract
Purpose
To evaluate numerical simulations of focused ultrasound (FUS) with a rabbit model, comparing simulated heating characteristics with magnetic resonance temperature imaging (MRTI) data collected during in vivo treatment.
Methods
A rabbit model was treated with FUS sonications in the biceps femoris with 3D MRTI collected. Acoustic and thermal properties of the rabbit muscle were determined experimentally. Numerical models of the rabbits were created, and tissue-type-specific properties were assigned. FUS simulations were performed using both the hybrid angular spectrum (HAS) method and k-Wave. Simulated power deposition patterns were converted to temperature maps using a Pennes’ bioheat equation-based thermal solver. Agreement of pressure between the simulation techniques and temperature between the simulation and experimental heating was evaluated. Contributions of scattering and absorption attenuation were considered.
Results
Simulated peak pressures derived using the HAS method exceeded the simulated peak pressures from k-Wave by 1.6 ± 2.7%. The location and FWHM of the peak pressure calculated from HAS and k-Wave showed good agreement. When muscle acoustic absorption value in the simulations was adjusted to approximately 54% of the measured attenuation, the average root-mean-squared error between simulated and experimental spatial-average temperature profiles was 0.046 ± 0.019 °C/W. Mean distance between simulated and experimental COTMs was 3.25 ± 1.37 mm. Transverse FWHMs of simulated sonications were smaller than in in vivo sonications. Longitudinal FWHMs were similar.
Conclusions
Presented results demonstrate agreement between HAS and k-Wave simulations and that FUS simulations can accurately predict focal position and heating for in vivo applications in soft tissue.
Introduction
High-intensity focused ultrasound therapy is a noninvasive treatment that uses focused ultrasound (FUS) to deposit acoustic energy at a specific target site in the body. This procedure has shown promise in the treatment of various medical conditions, including women’s health, oncology, and neurology [Citation1]. Thermal ablation in tissue can be induced using FUS, where the absorbed acoustic energy leads to localized temperature rise. Protein denaturation and eventual cell necrosis of the target tissue is achievable by reaching a thermal dose threshold. This thermal dose is a measure of thermal energy deposition, where a threshold of 240 min at 43 °C is commonly used to predict tissue necrosis [Citation2]. In addition to thermal ablation, FUS can also generate nonthermal mechanical forces that are useful for other therapeutic applications, such as histotripsy [Citation3], blood–brain barrier opening [Citation4], and neuromodulation [Citation5]. FUS has the potential to offer a safe and effective treatment option for a wide range of medical conditions, with ongoing research and clinical trials exploring its potential applications [Citation6].
Numerical simulations of FUS are used by researchers and clinicians in various ways. For instance, simulation of the focused beam informs the design of phased-array transducers, e.g., by determining element numbers [Citation7], size [Citation8], and positions [Citation9] to minimize grating lobes and increase efficiency. FUS simulation has also been used in model-based treatment planning and control [Citation10–12]. Retrospective simulation studies have been conducted to examine the effects of tissue acoustic and thermal properties on FUS energy deposition. For example, Hyvärinen et al. [Citation13] retrospectively simulated FUS for uterine fibroid treatment, determining that the acoustic absorption coefficient of fibroids had a substantial impact on predicted temperature rise, while the effect of realistic perfusion rates on peak temperature rise was minimal.
Pretreatment planning for ablative therapies has been shown to increase treatment efficacy [Citation14,Citation15] and pretreatment simulated therapies could be used to evaluate patient-specific confounding factors [Citation16]. In particular, pretreatment planning for FUS therapies can help determine the optimal parameters for successful treatment, such as the acoustic window, sonication power, and duration. FUS pretreatment planning can also predict levels of near- and far-field pressure and/or heating [Citation17], which can increase patient safety by informing parameters likely to lead to treatment performance within safe limits. While thermally ablative FUS has the advantage of using real-time magnetic resonance thermal imaging (MRTI) feedback to monitor temperature and heating location in certain tissues, FUS simulation can be useful in predicting safety constraints for treatment in and around tissues that are unsuitable for MRTI monitoring, such as adipose and bone tissue.
Simulation of thermally ablative FUS requires both acoustic and thermal modeling algorithms. Several acoustic simulation methods have been developed, all with tradeoffs in accuracy, model complexity, and computational efficiency [Citation18]. One computationally efficient method for acoustic simulation in heterogeneous media is the hybrid angular spectrum (HAS) method. As described by Vyas et al. [Citation19] the HAS method employs a three-dimensional numerical model of the targeted tissue volume composed of small rectangular voxels, each of which is assigned a specific tissue type with unique acoustic attenuation, speed of sound, and density coefficients. Briefly, the HAS algorithm utilizes the Rayleigh–Sommerfeld integral to initially determine the pressure field from the transducer through a water path to the front face of the model. The acoustic field is propagated plane-by-plane through the model in a split-step manner within each plane, alternating between the space and spatial-frequency domains. In the space domain, the effects of both attenuation and phase difference from the average phase shift are implemented by multiplying the complex pressure pattern by a pattern specific to each voxel’s acoustic properties. Using the Fast Fourier Transform, the pressure pattern is translated to the spatial-frequency (angular-spectrum) domain, where the planar-average phase shift is used in a transfer function to propagate to the next plane. The pressure pattern is then returned to the spatial domain using the inverse Fast Fourier Transform. This process is repeated for each plane of the model until the pressure pattern has been simulated for the entire model. As currently implemented [Citation19], several key assumptions are made in the algorithm, including linear and steady-state conditions, non-varying acoustic tissue properties in time, no tissue movement within the model, and that all acoustic attenuation is due to absorption.
The resultant pressure pattern, along with the tissue absorption coefficients, allows computation of the volumetric power deposition pattern, which can then be used to obtain the four-dimensional thermal rise in the tissue with a finite-difference time-domain (FDTD) implementation of the Pennes’ bioheat equation (PBHE) [Citation20,Citation21].
Recently, the ITRUSST consortium compared output characteristics of eleven different FUS modeling tools, including HAS, in nine benchmark tests simulated with two transducers [Citation18]. Resultant maximum pressures, focal positions, and focal size showed close agreement among the modeling tools. When comparing the resultant pressure patterns derived from the HAS algorithm with pressures patterns derived from all other simulation methods, the median difference in peak pressure was within 10%, the median difference in focal position was 0.5 mm, and the median differences in axial and lateral focal size were within 0.6 and 0.2 mm, respectively. While no comparisons were made on computational efficiency in this study, it is noted that HAS trades some measure of accuracy for increased calculation speed.
Previous work [Citation22,Citation23] has also shown good agreement between HAS-simulated FUS and experimental MR thermometry data in soft tissue-mimicking phantoms. For transcranial FUS treatments [Citation24], positional differences between the HAS-simulated heating location and the experimental heating location were lower than the MR thermometry resolution in both the frequency- and phase-encoding directions. The HAS-simulated temperature rise varied significantly compared with the experimental temperature rise, with the simulated temperatures ranging from greatly overestimating heating to greatly underestimating heating, depending on the patient.
For many applications of FUS simulation, accurate prediction of the temperature rise, focal position, and focal size is necessary. The aim of this study is to experimentally evaluate the accuracy of current FUS modeling tools in predicting these metrics for soft-tissue applications using an in vivo animal model, utilizing rigorously characterized tissue properties to compare simulated temperature profiles in a heterogeneous soft-tissue rabbit model with those obtained experimentally. HAS was chosen as the FUS modeling method due to its simulation speed in comparison to full-wave and FDTD approaches, with the modeling tool k-Wave [Citation25] used as a comparison for one sonication per animal. As in previous work [Citation22,Citation23], 3D magnetic resonance temperature imaging data obtained during in vivo FUS are used as a clinical comparison metric.
Materials and methods
In vivo focused ultrasound exposures
All animal studies were carried out under the guidance of the local Institutional Animal Care and Use Committee. MR-guided FUS was performed using female and male New Zealand white rabbits (n = 4, 2.7–3.1 kg), targeting regions in the quadricep muscle (biceps femoris). A rabbit muscle model was chosen because a heterogeneous animal model with a large amount of homogeneous tissue was required to ensure ex vivo tissue properties could be accurately measured. It emulates the range in MRI relaxometry and diffusion values, tissue acoustic and thermal properties, and acute edematous response as found in MRgFUS treatments. Muscle provides an excellent tissue for accurate MR thermometry and the biceps femoris is an accessible muscle target where bone is not in the attenuation path of the ultrasound beam. The tissue was also able to be excised, allowing for acoustic and thermal property measurement. Each animal’s leg was shaved prior to treatment and a depilatory agent (NairTM, Church & Dwight) was applied to remove any remaining hair. Experiments were performed in a 3 T MRI scanner (PrismaFIT, Siemens Healthcare, Erlangen, Germany) and a 256-element phased-array focused ultrasound transducer (10 cm radius of curvature, f = 940 kHz, pressure full width half maximum in water = 1.60 × 2.39 × 9.74 mm3, 2 mm element radius with semi-random placement, Image Guided Therapy, Pessac, France, and Imasonic, Voray-sur-l’Ognon, France) was used to perform the FUS ablations. Animals were anesthetized with ketamine and xylazine (25 and 5 mg/kg, intramuscular injection, respectively), intubated, and maintained with isoflurane (1–3%, inhaled, 100% oxygen). Animals were placed in lateral decubitus position on an MR-compatible FUS system (), with the leg submerged in degassed and deionized water (33–37 °C) above the transducer. One to six single-point sonications were performed (8.8–48.3 acoustic watts, 30.2–33.6 s each) in the biceps femoris, as indicated in . Approximately five minutes elapsed between sonications, during which the transducer was mechanically moved laterally several millimeters to target untreated tissue. Sonications were monitored in real time with MRTI (3D GRE with segmented EPI readout) to measure temperature changes via the proton resonance frequency shift method [Citation27] within the ultrasound focal region for the duration of each sonication, with post-sonication cooling temperature data recorded for approximately 60 s. An updated thermometry baseline measurement was acquired between sonications. T1-weighted and T2-weighted MR images were captured before and after treatment, with additional contrast-enhanced T1-weighted imaging (gadoteridol, IV 0.1 mmol/kg) post-ablation. MR sequence parameters are listed in . Animals were euthanized immediately following post-ablation imaging and tissues were harvested for further analysis, as described below.
Figure 1. Rabbit focused ultrasound system. Experimental structure includes a single-channel loop RF imaging coil (A), degassed, deionized water coupling reservoir (B), ultrasound transducer housing (C) with MRI positioning radiofrequency coils [Citation26] and multi-axis adjustable positioning (two translational and one rotational degree of freedom), (D). Animals (E) were positioned with a saline bag (F) between the rear legs such that the focal zone of the transducer (G) was located in the quadriceps. Also indicated are the transducer face (green), approximate ultrasound beam path (blue), and MRTI volumetric coverage (yellow). 2D projection of semirandom transducer element locations (H).
![Figure 1. Rabbit focused ultrasound system. Experimental structure includes a single-channel loop RF imaging coil (A), degassed, deionized water coupling reservoir (B), ultrasound transducer housing (C) with MRI positioning radiofrequency coils [Citation26] and multi-axis adjustable positioning (two translational and one rotational degree of freedom), (D). Animals (E) were positioned with a saline bag (F) between the rear legs such that the focal zone of the transducer (G) was located in the quadriceps. Also indicated are the transducer face (green), approximate ultrasound beam path (blue), and MRTI volumetric coverage (yellow). 2D projection of semirandom transducer element locations (H).](/cms/asset/244b6865-785a-45c4-8696-a81b01197ca7/ihyt_a_2301489_f0001_c.jpg)
Table 1. Ultrasound exposure conditions. Average heating reported for a 2.5 × 2.5 × 9.5-mm3 volume at the time of peak heating.
Table 2. MRI sequence parameters.
Muscle property measurement
Subject-specific acoustic and thermal properties of the rabbit muscle were measured for use in HAS simulations after animal euthanasia. For each animal, a section of each hamstring was excised and encased in a saline-filled cylindrical tissue holder for property measurement. The speed of sound of the tissue was measured using the through-transmission method described by Farrer et al. [Citation28] Briefly, the encased muscle section was placed in a degassed water-filled tank between a single-element broadband transducer centered at 1 MHz (Panametrics-NDT, V314, Watham, MA, USA) and a receiver hydrophone (ONDA, HNR-0500, Sunnyvale, CA, USA) such that the ultrasound beam passed through the sample. The transducer was driven in bursts of 10 cycles at four frequencies: 600 kHz, 1.0, 1.8, and 3.0 MHz, and the corresponding oscilloscope signals from the hydrophone recorded. A reference measurement using a saline-filled tissue holder (i.e., with no tissue sample in place) was acquired for the same frequencies. Time-of-flight differences between the sample and water reference signals were measured, resulting in speed-of-sound calculations for the tissue at each frequency considering the measured thickness of the sample, which in turn were used to determine the speed of sound with respect to frequency.
Acoustic attenuation of each tissue sample was determined using the radiation force balance method [Citation29]. An ultrasound absorbing target was suspended underneath a balance (Fischer Science EducationTM Portable Balance, Model SLF103, Thermo Fisher Scientific, Watham, MA, USA) at the top of a water column with the same 256-element ultrasound transducer from the in vivo experiments positioned at the bottom. The same hamstring samples used for the speed of sound measurements encased in the acoustically transparent holder were placed between the ultrasound transducer and the target. Measurements were taken with the tissue in three different positions (laterally translated a few millimeters and rotated approximately 120° respective to the transducer/target axis to partially average over local tissue inhomogeneities) and with the transducer driven at two different power levels (1.7 and 3.4 acoustic watts). The resulting weight of the hanging target was recorded at a sampling rate of 1 Hz and converted to absorbed power, according to EquationEq. (1)(1)
(1) :
(1)
(1)
where P is the calculated acoustic power, m is the measured mass of the target while sonicating, g is the Earth’s gravitational acceleration constant 9.81 m/s2, and cw is the speed of sound in water.
Measurements were also taken at these conditions, with no tissue in the holder (saline only). The pressure attenuation coefficient at the 940 kHz frequency of the transducer was calculated according to EquationEq. (2)
(2)
(2) :
(2)
(2)
where PS and PW are the measured acoustic powers in watts after passing through the sample and saline only, respectively, d is the thickness of the tissue sample in cm, and sf is a transducer-specific scaling factor that accounts for the spread of the incident angles of the ultrasound beam (0.915 for the transducer in this study), accounting for the average path length variation over the transducer elements. The calculated attenuation values for all positions and transducer powers were averaged to determine subject-specific attenuation values.
Heat capacity and thermal conductivity of each tissue sample were measured using the transient-line heat source method with a KD2 Pro thermal probe (Decagon Devices, Inc., Washington, USA) as previously described [Citation30].
Segmented digital model creation
T1- and T2-weighted images of each animal taken prior to treatment were used to create 3D digital models of each rabbit leg. A semi-autonomous process using Seg3D (NIH Center for Integrative Biomedical Computing, Salt Lake City, UT, USA) was used to segment each digital model into muscle, fat, bone, and bowel through a combination of thresholding, edge detection tools, and manual segmentation. A skin layer of uniform thickness (1 mm) was created exterior to the segmented tissue. Voxels exterior to the skin layer were treated as water. The resolution of all models was 0.5 mm isotropic. To evaluate the effect of variability of the segmentation process, Rabbit 1 was separately segmented by three trained individuals. Each resultant segmented model was used to simulate all four sonications conducted experimentally using HAS and a validated PBHE thermal solver [Citation31] via the process described below. The similarity of the three segmented models was evaluated using the Dice similarity coefficient (DSC), with multi-class DSC used to determine overall similarity and single-class DSC used to determine specific tissue-type similarities. Differences in peak temperatures and peak temperature locations were calculated. An example of the segmented numerical model for Rabbit 1 is shown in .
Figure 2. Representative segmented digital model and simulated pressure pattern. Segmented model (A) coronal, (B) sagittal, and (C) transverse views. Each rabbit was segmented with a semi-autonomous procedure into five tissues: muscle (red), fat (yellow), bone (blue), skin (green), and bowel (dark red), with all non-segmented voxels assigned as water. Focus location denoted with yellow “F.” Simulated ultrasound pressure pattern (D) coronal, (E) sagittal, and (F) transverse views superimposed on model outline. Pressure pattern was simulated using the hybrid angular spectrum technique. Pressure simulations used a radiating rear boundary and totally reflecting edges. Typical attenuation paths include 3 to 5 total pre-focus interfaces (water/skin, skin/muscle or skin/fat, and 0 to 4 amounts of muscle/fat interfaces), and general path lengths of 1 mm skin and 10 to 20 mm of ∼90–100% muscle and ∼0–10% fat.

HAS and PBHE simulations
Pressure patterns for all sonications were computed using the HAS algorithm. The transducer location was registered to MR coordinate space using in-house tracker coils on the transducer housing, with a triangulation algorithm of the tracker coil locations used to identify the geometric focus. The segmented discrete models of each animal were registered to the transducer location for each sonication using the MR positioning-coil data [Citation32] recorded during each sonication. Because the bulk of the FUS focal energy was deposited in the target muscle, muscle tissue properties were modeled on a subject-specific basis using measured values, as described above. The muscle voxels were assigned subject-specific measured attenuation, speed of sound, heat capacity, and thermal conductivity values. In addition, density and perfusion values for the muscle, along with all properties for the non-muscle tissue types, were assigned values from the literature [Citation33–35], as listed in . Pressures were derived using a Dirichlet boundary condition at the array surface, with the surface pressure of each element made consistent with the assumed radiated beam power. Back propagation can be modeled using the HAS method [Citation19], and a single reflection was modeled for all sonications. An example pressure pattern is shown in . Pressure patterns obtained by the HAS simulations were used to calculate power deposition maps according to EquationEq. (3)(3)
(3) :
(3)
(3)
where Q is the power deposition for a specific voxel, α is the acoustic absorption coefficient, p is the calculated pressure from the simulation, and ρ and c are the density and speed of sound of the voxel’s assigned tissue type, respectively. These power deposition maps were used to create dynamic temperature maps across all voxels of the model using a PBHE thermal solver (0.084 s temporal resolution, 0.5 mm isotropic spatial resolution, uniform initial temperature condition, adiabatic boundary temperature). Spatial and peak temperature convergence testing was performed using calculated 3D pressure and power deposition maps at voxel sizes of 0.5, 0.25, and 0.1 mm3.
Table 3. Tissue property values used in the acoustic and thermal modeling.
HAS benchmarking with k-wave simulations
Linear acoustic propagation modeling with HAS was compared to a well-established standard for full-wave acoustic simulation in heterogeneous tissues, known as k-Wave [Citation18] in a subset of sonications. Additionally, effects of nonlinear acoustic propagation were investigated and quantified with nonlinear k-Wave simulations. The segmented tissue models were interpolated to a 0.39 mm isometric voxel size in order to be below a quarter of the ultrasound wavelength as required for the full-wave implementation of k-Wave. The first treatment sonication of each rabbit was simulated using k-Wave and HAS at the 0.39 mm isometric voxel size with identical acoustic parameters as used in the previous HAS simulation. Pressures were calculated in k-Wave using both linear and nonlinear propagation. Maximum pressure values, their location, and the full width at half maximum (FWHM) of the pressure were compared between simulation outputs of HAS and both linear and nonlinear k-Wave. To compare the effects that nonlinearities may have on temperature, linear HAS and nonlinear k-Wave derived pressures were converted to temperature using PBHE as outlined above. Spatially averaged temperatures, the center of thermal mass (COTM), and the FWHM of the simulated heating were compared between the HAS and nonlinear k-Wave models.
Data analysis
The experimental MRTI data were zero-fill interpolated to 0.5 mm isotropic voxels, the same resolution as the simulated HAS model for voxel-wise comparison. For each sonication, the 3D position of the COTM was calculated using a temperature-weighted spatial average of a volume containing the ultrasound focal zone at the time of maximum heating, both for the experimental and simulated sonications. Differences in location between the experimental and simulated COTMs were calculated, and ANOVA with Tukey honestly significant difference (HSD) was used to determine the statistical similarity of COTM differences between Rabbits 1-3. Rabbit 4 was not included because it underwent only one sonication. Temperature rise and peak temperatures were calculated over a 2.5 × 2.5 × 9.5 mm3 volume centered at the focus to quantify heating over the approximate FWHM of the predicted beam profile. Temperatures were normalized by sonication power for comparison. The root-mean-square error between the spatially averaged experimental and simulated temperatures was calculated.
The full-width half-maximum of the focal spot at the peak temperature rise for each sonication, both experimental and simulated, was determined by thresholding a contiguous volume around the COTM for all voxels reaching at least 50% of the peak temperature. The FWHM values in the direction of propagation (longitudinal) and orthogonal (transverse) directions of the volume were calculated.
Estimate of absorption coefficient
The attenuation coefficient is a combination of scattering and absorption, but only the absorption component contributes to focal heating. Scattering is difficult to measure directly; therefore, while the total attenuation coefficient of the targeted muscle was determined as described above, the percentage due to absorption is unknown. However, it can be roughly estimated from the observed experimental temperature rise by running an optimization simulation to find the fraction of total attenuation that could be attributed to absorption in determining the power absorption pattern. HAS uses attenuation for pressure calculations and a separate absorption value to calculate power deposition; therefore, an iterative process was employed to find this optimized subject-specific absorption value as follows: Each sonication was simulated using the HAS algorithm over a range of absorption values for muscle (40–100% of the measured attenuation value). Then, the RMSE was calculated between temporally interpolated simulated and experimental MRTI temperatures at the peak time point over a 2.5 × 2.5 × 9.5 mm3 volume centered at the focus. For each rabbit, the absorption value with the lowest average error across all sonications was selected as the optimized absorption value used to calculate the power deposition pattern and resulting simulated temperatures for that animal.
Tissue edema assessment
Evaluation of treatment-related tissue changes was performed by calculating post-treatment edema volume in T2-weighted images acquired after all sonications were completed. Segmentation of the edematous region was achieved using a signal intensity thresholding technique (Seg3D) similar to the methods used in the model segmentation.
All image reconstruction, simulations, and computations were performed in MATLAB 2021a (MathWorks, Natick, MA, USA) on an Intel® Xeon® Silver 4210 CPU (2.20 GHz).
Results
The measured subject-specific thermal and acoustic properties of the rabbit muscles are summarized in . Due to measurement equipment failure during the analysis of Rabbit 3’s tissue, the attenuation value for Rabbit 3 is the mean attenuation coefficient of the other three animals.
Table 4. Measured subject-specific tissue properties used in simulations.
To compare the simulation results using the HAS method to those of k-Wave, four cases were considered using the parameters of the first sonication for each of the four rabbits. The simulated peak pressures derived with the HAS method exceeded the simulated peak pressures from linear k-Wave simulations by 1.6 ± 2.7%, while the simulated peak pressures from the HAS method were lower than the simulated peak pressures from nonlinear k-Wave by 1.1 ± 6.2%, as shown in . The location of the peak pressure calculated from HAS and both linear and nonlinear k-Wave differed by less than 1 mm in the transverse and longitudinal planes. The FWHM for the pressure was 2.54 ± 0.23 × 1.90 ± 0.10 × 10.56 ± 0.28 mm3 when calculated with HAS, 2.58 ± 0.19 × 1.80 ± 0.19 × 11.32 ± 0.85 mm3 when calculated with linear k-Wave, and 2.05 ± 0.20 × 1.37 ± 0.23 × 10.34 ± 0.50 when calculated with nonlinear k-Wave.
Figure 3. Representative pressure patterns derived from HAS and k-Wave. The HAS method assumes linear wave propagation through all tissues. To quantify the effect that nonlinearities may have on simulations, the first simulated sonication from each animal using HAS was compared to simulations of the same sonications using k-Wave with nonlinear propagation. Shown are the transverse views of the pressure patterns derived with both the HAS method and k-Wave with nonlinearities. The simulated peak pressures derived with the HAS method were lower than the pressures from nonlinearly propagating k-Wave by 1.1 ± 6.2%.

Simulated peak temperatures averaged over a 2.5 × 2.5 × 9.5 mm3 volume using HAS were 32.3, 28.0, 4.5, and 23.4 °C for animals 1 to 4, respectively. Simulated peak temperatures over the same spatially averaged volume were 30.1, 28.6, 4.4, and 24.0 °C using k-Wave with nonlinear propagation (). The temperature FWHM was 3.02 ± 0.20 × 2.73 ± 0.32 × 11.80 ± 1.25 for the HAS model and 3.02 ± 0.20 × 2.93 ± 0.23 × 11.80 ± 1.51 for the nonlinear k-Wave model.
Figure 4. Spatially averaged simulated temperature profiles. Temperature profiles of the first sonication for each animal were simulated using HAS (blue) and with nonlinear propagating k-Wave (orange). Temperature profiles are for a 2.5 × 2.5 × 9.5 mm volume centered at the center of thermal mass. Temperatures shown (A–D) are simulations of the first sonication applied to Rabbits 1–4, respectively.

Inter-user variability in model segmentations was evaluated by performing a multi-class similarity analysis (Dice Similarity Coefficient) between the three segmentations of Rabbit 1 performed by different segmenters. This analysis demonstrated an overall similarity coefficient of 0.852 ± 0.006 in assigned voxels between pairs of segmentations, with specific tissue type coefficients of 0.944 ± 0.001 for water voxels, 0.367 ± 0.007 for fat, 0.796 ± 0.001 for muscle, 0.531 ± 0.060 for bowel, 0.783 ± 0.003 for bone, and 0.271 ± 0.010 for skin. Acoustic and thermal simulations performed with the three different models resulted in maximum temperature rise of 0.382 ± 0.013, 0.427 ± 0.030, and 0.427 ± 0.075 °C/W with no change in the location of the peak temperature.
Spatial-mean experimental and simulated temperatures normalized to applied acoustic power over all sonications for Rabbit 1 (N = 4), Rabbit 2 (N = 6), Rabbit 3 (N = 5), and Rabbit 4 (N = 1) are shown in . The normalized experimental peak temperatures averaged over a 2.5 × 2.5 × 9.5 mm3 volume positioned at the center of thermal mass were 0.379 ± 0.070 °C/W for individual sonications (N = 16, blue curves). The normalized simulated peak temperature average was 0.637 ± 0.098 °C/W for the same volume (green curves). Average RMSE between the experimental and simulated heating curves was 0.152 ± 0.068 °C/W.
Figure 5. Normalized mean spatial experimental and simulated temperatures. Experimental (blue), simulated with absorption equal to attenuation (green), and simulated with scaled absorption value (orange) average (mean ± standard deviation) temperature profiles for a 2.5 × 2.5 × 9.5 mm volume centered at the center of thermal mass for all sonications of (A) Rabbit 1 (N = 4), (B) Rabbit 2 (N = 6), and (C) Rabbit 3 (N = 5). A single sonication is shown for (D) Rabbit 4. All temperature profiles are normalized to applied acoustic power.

Using the optimization procedure described in the Methods section, the percentage of muscle attenuation due to absorption was found to be 54.1, 54.0, 52.7, and 56.1%, respectively, for Rabbits 1 to 4, leading to an average absorption coefficient of 2.53 ± 0.3 Np/m. When these absorption values were input into the HAS model (while still assuming the measured attenuation values to obtain the pressures), the normalized simulated peak temperatures averaged across the 2.5 × 2.5 × 9.5 mm3 volume was 0.340 ± 0.050 °C/W for individual sonications (orange curves). The average RMSE between the experimental and simulated spatial average temperature data using the optimized absorption coefficient for each sonication (N = 16) was 0.046 ± 0.019 °C/W.
The experimental and simulated temperatures overlaid on T1-weighted images of the targeted tissue are shown in for a single sonication in Rabbit 1, qualitatively indicating the position and shape of the focal heating. Positional differences between the experimental and simulated COTM varied across subjects and sonications, as shown in and . ANOVA determined statistically significant differences in the location of the COTM between Rabbits 1 and 3 (p = 0.0030), with Tukey HSD determining statistically significant differences between the COTMs of Rabbits 2 and 3 (p = 0.0027) and Rabbits 1 and 3 (p-value = 0.0297), but not between Rabbits 1 and 2 (p-value = 0.2438). The location of the peak temperature data was stable with no spatial drift.
Figure 6. Experimental and simulated focal zone thermal profile. Peak temperature rise in focal zone of (A) experimental and (B) simulated FUS treatments for single sonication in Rabbit 1. Temperature values shown are greater than 20% of peak temperature rise. Edge of MRTI volume proximal to transducer indicated by arrows in experimental images.

Figure 7. Distance between experimental and simulated centers of thermal mass. Mean transverse and longitudinal location differences between experimental and simulated centers of thermal mass (COTM). Error bars denote standard deviation for Rabbits 1 (blue), 2 (red), and 3 (orange). A single point (purple *) is shown for Rabbit 4. COTM calculated at time of maximum temperature rise for each sonication.

Table 5. Distance between experimental and simulated centers of thermal mass.
The calculated FWHM of the heated volume for the experimental and simulated conditions in three axes are shown in . Along the two orthogonal transverse axes of the transducer, the FWHM values averaged over all rabbits and sonications were 3.81 ± 0.60 mm and 3.28 ± 0.71 mm in experimental treatments and 2.53 ± 0.13 mm and 3.00 ± 0.18 mm in simulated treatments. The FWHM in the longitudinal direction was 11.38 ± 3.43 mm and 11.81 ± 2.10 mm for the experimental and simulated treatments, respectively.
Figure 8. Full width at half maximum length of focal spot. Mean ± standard deviation of the FWHM length of the heated volume for simulated (blue) and experimental (orange) FUS in (A) animal head-foot direction normal to the direction of sonication, (B) animal anterior-posterior direction normal to the direction of the sonication, and (C) animal left-right direction along the direction of ultrasound propagation.

shows representative pre- and post-treatment T2-weighted images for Rabbit 2. The post-treatment image for each rabbit showed a large, hyperintense region not present in pretreatment images. The edematous volume present in Rabbits 1 to 3 at the conclusion of all sonications was determined to be 936, 2376, and 1402 mm3. Edematous volume was not calculated for Rabbit 4, as additional sonications unrelated to this study were performed following the treatment.
Figure 9. Pre- and post-FUS T2-weighted images. Image taken prior (A) and post (B) treatment. Arrow demonstrates areas of significant hyperintensity associated with edema. Total post-treatment hyperintense volumes were 936, 2376, and 1402-mm3 for Rabbits 1–3, respectively. Volume was not calculated for Rabbit 4.

Discussion
Previous work has quantitatively evaluated the accuracy of the HAS algorithm and FDTD implementation of the PBHE to predict the temperature rise achieved during FUS sonications in both homogeneous and heterogeneous phantom environments. Johnson et al. [Citation22] reported a 6.9 ± 5.0% overestimation of FUS heating in tissue-mimicking homogenous gelatin phantoms when using the HAS method in conjunction with the PBHE and utilized a linear regression and local sensitivity analysis to determine that uncertainty in attenuation had a substantial impact on the uncertainty in peak temperature in comparison with other acoustic and thermal tissue properties. That work also demonstrated that the HAS algorithm had a 0.94 ± 0.18 mm error toward the transducer in predicted focal spot location. Hansen et al. [Citation23] expanded this work, evaluating HAS in strongly aberrating heterogeneous phantoms with known acoustic and thermal properties. They demonstrated a 10.6% overestimation of the heating and a 2.1 mm shift in the location of the center of the thermal mass when comparing HAS-simulated and experimental MR thermometry data.
The HAS method has also been used to retrospectively analyze transcranial FUS treatments. Leung et al. [Citation24] evaluated transcranial magnetic resonance-guided FUS treatments in essential tremor patients. In through-skull heating, mean positional error between MR thermometry and simulated focal spots was 0.78 mm in the frequency direction and 1.48 mm in the phase encode direction. Statistically significant correlation between simulated and experimental temperature rise was shown, although the agreement between the two was highly variable, with an approximately four-fold difference in simulated temperatures when comparing simulations with two different realistic skull attenuation values. Changes in thermal conductivity and perfusion had minimal effects on temperature rise. It was hypothesized that the peak temperature differences were most likely caused by errors in the simulated skull acoustic attenuation.
In this study, we evaluated the accuracy of predicted temperature change and focal spot accuracy in simulated FUS in comparison with MR thermometry obtained during in vivo FUS.
Simulated maximum pressures from HAS and k-Wave with linear and nonlinear propagation showed good agreement, with HAS predicting maximum pressures an average of 1.6 ± 2.7% higher than linear k-Wave and 1.1 ±.6.2% lower than nonlinear k-Wave. Differences in the location of peak pressure were less than 1 mm between simulations of the same sonication. This agreement between FUS treatments simulated with HAS, linear k-Wave, and nonlinear k-Wave demonstrates that differences between in vivo and simulated temperature change are unlikely to be specific to the underlying assumptions of the HAS algorithm and are more likely to be due to errors in segmentation, inaccurate acoustic and thermal properties (in particular, the muscle absorption coefficient), uncertainties in the applied power levels, and changing physiological conditions during treatment.
Nonlinear wave propagation can have substantial effects on the pressure and deposited energy in FUS, depending on the strength of the incident field; however, the current version of HAS assumes linear wave propagation. Generally, nonlinear effects result in higher focal temperatures in FUS applications. To quantity this effect, HAS-derived pressures and temperatures were compared to nonlinear wave propagation simulations in k-Wave for the modest incident pressure levels used in this study. Simulated pressures from k-Wave with nonlinear propagation closely matched HAS pressures, with k-Wave pressures slightly exceeding those in HAS in two simulations and pressures in HAS slightly exceeding k-Wave in the other two. Normalized spatially averaged peak temperatures derived from the calculated pressures also followed the same trend, with an average difference of 0.01 ± 0.03 °C/W or 1.2 ± 4.6% in normalized peak temperature. (The choice to use spatially averaged temperatures was made to provide thermal information across the focal zone, as opposed to peak temperatures which only provide a quantitative analysis of a single voxel.) This analysis suggests that nonlinearities are most likely not responsible for the difference between the HAS-simulated temperatures and the measured in vivo temperatures.
The results in this work agree with those of other studies [Citation22,Citation23] that have demonstrated an overestimation of simulated temperature rise when compared with experimental values and a high degree of sensitivity to the absorption and attenuation values of sonicated tissues. When the muscle absorption coefficient was assumed to be equal to the measured muscle attenuation value, average temperatures of the 2.5 × 2.5 × 9.5 mm3 volume centered at the focus were 69.5 ± 12.7% higher in simulations than in the recorded MRTI temperature measurements at the peak-temperature time step. When muscle absorption was scaled to be only an optimized fraction of attenuation, simulated temperature profiles agreed (RMSE of 0.046 ± 0.019 °C/W) with the in vivo MRTI measurements. The problem of parsing acoustic attenuation into absorption and scattering components is difficult. Literature is sparse and demonstrates the difficulty of measurement and estimation. As an example, values for absorption in liver have been reported to range from 31% [Citation36] of attenuation to 94% [Citation37]. Similarly, measured absorption fraction in bovine muscle were also shown to have high variation when measured at different fiber orientations; the results in Lyons et al. [Citation37] reported three conflicting cases in their findings: absorption and attenuation were found to be identical, absorption was found to be half that of attenuation (at 1 MHz), and the impractical condition of absorption being higher than attenuation. In a study exploring the effect of ultrasound scattering on thermal dose, Cortella et al. [Citation38] simulated heating in ex vivo bovine muscle using a finite-difference forward-time centered-space method. In that study, when including only the measured attenuation value of the muscle in the PBHE, simulated temperatures overestimated experimental temperatures, and when the measured absorption value was used in place of the attenuation value in the PBHE, simulated temperatures underestimated the experimental temperatures. In our work, the estimated absorption components were similar across subjects, which is expected for singular tissue type in a specific animal model. While simulations using the estimated absorption coefficient did more closely reflect experimental temperatures, differences in the temperatures during cooling suggest that other parameters would also need to be adjusted in order to accurately reflect the in vivo condition.
Creating representative segmented models of the treatment area is more difficult with in vivo studies than phantoms and has higher potential to increase error between simulated and experimental data but is nevertheless necessary for patient-specific simulations. The segmentation performed for this work was semi-autonomous, requiring user input. Precise delineation between tissue types is often not possible due to partial-volume voxels that contain multiple tissues. Additionally, segmentation often looks to assign a simplified number of tissue types compared to the number of types actually present. This work evaluated this source of variability by creating numerical models by three individuals. While DSC showed overall segmentation varied in Rabbit 1 by 15% depending on the individual performing segmentation, heating location was identical for each sonication (in a 0.5 mm isotropic model). However, temperature rises from the simulated sonications differed by 11.7 ± 10.5% between the three different models. The lack of variation in heating positions across segmentations demonstrated that positional accuracy may be independent of the segmenting individual, but the larger variability in spatial average temperatures may indicate that precise segmentation is necessary for accurate simulated temperature increases. Segmentation accuracy may be improved through the use of improved input images, an increased number of contrasts, or the use of U-net or other AI-based techniques [Citation39].
The HAS algorithm, like many other simulation methods, assumes constant acoustic and thermal tissue properties throughout an individual sonication, and this study assumed constant properties over all FUS sonications for each animal. However, the complex environment of the treated region may change substantially over the course of treatment. FUS-induced edema, indicated by hyperintense volumes in T2-weighted images of the sonicated tissue, demonstrates that the accumulated thermal dose likely was sufficient for ablation of tissue. This hyperintensity may indicate substantial vasculature and cellular destruction [Citation40], leading to changes in both thermal and acoustic tissue characteristics. While this study attempted to minimize these tissue property changes by mechanically moving the transducer between sonications, the relative size of the FUS focal spot size to the rabbit quadriceps limited this strategy, as demonstrated by the edematous tissue response seen in . Therefore, even if patient-specific thermal and acoustic tissue properties are known at the beginning of FUS sonications, these properties will likely change during the course of successive FUS sonications and could introduce some level of inaccuracy in treatment simulations.
While focal spot position error was larger than in previous work in phantoms [Citation22,Citation23], agreement between the experimental and simulated COTMs was good (mean error of 3.25 ± 1.37 mm), with a bias toward overestimating the depth of the COTM. The mean distance between the experimental and simulated COTM was 0.92 ± 0.57 mm in the transverse direction and 3.08 ± 1.34 mm in the longitudinal direction. The differences in COTM location were also highly animal dependent, with Rabbit 3 having a statistically significant smaller error in simulated position compared to Rabbits 1 and 2. The COTM of Rabbit 4, with only one sonication, was unable to be statistically compared to the others. The transverse focal spot FWHMs for in vivo sonications were generally larger than the simulated FWHMs (3.81 vs. 2.53 mm and 3.28 vs. 3.00 mm) and had higher standard deviations (0.60 vs. 0.13 mm and 0.71 vs 0.18 mm). Average longitudinal FWHM were similar (11.38 mm experimental and 11.81 mm simulated), with again higher standard deviations in the experimental data (3.43 mm experimental and 2.10 mm simulated). This could be due to a number of factors, including scattering effects, aberration of the ultrasound beam, variability of thermal properties in the target tissues, or partial volume effects of MRTI.
The utility of FUS simulation methods such as HAS as treatment planning tools remains conditional on several factors. Initially, creating a segmented model of the treatment target volume mandates pretreatment imaging of the patient and adequate time between the imaging and treatment for segmentation. However, this study does demonstrate that some differences in models may only lead to small overall changes in simulated heating and position; thus, increasing the accuracy of segmented models may have diminishing returns. However, these effects will likely change for more patient-specific heterogeneous tissue environments. Acoustic and thermal properties of all segmented tissues also must be known prior to simulation, which can create a significant challenge. Measurement of these properties, especially acoustic absorption, would need to be undertaken for all tissues in the acoustic window, as well any temperature-dependent changes. For tissues with large interpatient variability, subject-specific properties would need to be measured or estimated, some of which may be possible utilizing MRTI with low-grade heating [Citation41]. Although modeling in vivo treatments is currently limited by challenges in measuring tissue characteristics, HAS demonstrated accurate focal positioning and good agreement with experimental temperature rises after adjusting the acoustic attenuation to account for estimated absorption.
As a fast method for simulating high-intensity FUS sonications, HAS may be more easily adapted for clinical workflows than alternative full-wave numerical methods such as k-Wave. While this work is not specific to any one clinical indication, its finding can be applied to any soft tissue FUS treatment, such as the treatment of uterine fibroids or breast cancer. These results do show that simulated pressure patterns generated using the HAS method may be useful in spatial positioning of the ultrasound transducer and patient by accurately predicting focal location. One application of this work in breast cancer would be to apply fast phase-aberration correction for targeting heterogeneous breast tissue [Citation42]. However, accurate simulations of in vivo temperatures will require incorporation of acoustic scattering in existing models and in vivo measurements of attenuation fractions. More work should be done to accurately simulate in vivo temperature change with the evaluated methods.
Conclusion
The HAS method for simulating FUS was evaluated against MRTI data taken from in vivo MRgFUS using a rabbit model. The HAS predicted temperatures greatly exceeded those measured with MRTI, possibly due to acoustic scattering in the tissue, but the focal position and size showed good agreement. Simulated temperatures can be brought into alignment with the in vivo temperatures by scaling the acoustic absorption to be less than the acoustic attenuation. Pressures derived using the HAS method agreed with k-Wave simulations, both with linear and nonlinear wave propagation modeled. Future work to quantify acoustic scattering in biological tissues, incorporate scattering and nonlinear wave propagation in HAS, and simulating FUS in other tissues would further inform FUS simulation efforts.
Acknowledgments
Mark H. Huntsman Endowed Chair. The authors appreciate help from Dr. Rock J Hadley and Robb Merrill for designing and building the rabbit setup and RF coil used in this study.
Disclosure statement
No potential conflict of interest was reported by the author(s).
Data availability
The data that support the findings of this study are available from the corresponding author, AP, upon reasonable request.
Additional information
Funding
References
- Bachu VS, Kedda J, Suk I, et al. High-intensity focused ultrasound: a review of mechanisms and clinical applications. Ann Biomed Eng. 2021;49(9):1975–1991. doi: 10.1007/s10439-021-02833-9.
- Sapareto SA, Dewey WC. Thermal dose determination in cancer therapy. Int J Radiat Oncol Biol Phys. 1984;10(6):787–800. doi: 10.1016/0360-3016(84)90379-1.
- Roberts WW, Hall TL, Ives K, et al. Pulsed cavitational ultrasound: a noninvasive technology for controlled tissue ablation (histotripsy) in the rabbit kidney. J Urol. 2006;175(2):734–738. doi: 10.1016/S0022-5347(05)00141-2.
- Hynynen K, McDannold N, Sheikov NA, et al. Local and reversible blood–brain barrier disruption by noninvasive focused ultrasound at frequencies suitable for trans-skull sonications. Neuroimage. 2005;24(1):12–20. doi: 10.1016/j.neuroimage.2004.06.046.
- Legon W, Bansal P, Tyshynsky R, et al. Transcranial focused ultrasound neuromodulation of the human primary motor cortex. Sci Rep. 2018;8(1):10007. doi: 10.1038/s41598-018-28320-1.
- 2022 State of the Field Report. Focused Ultrasound Foundation. 2022.
- Pajek D, Hynynen K. The design of a focused ultrasound transducer array for the treatment of stroke: a simulation study. Phys Med Biol. 2012;57(15):4951–4968. doi: 10.1088/0031-9155/57/15/4951.
- Goss SA, Frizzell LA, Kouzmanoff JT, et al. Sparse random ultrasound phased array for focal surgery. IEEE Trans Ultrason, Ferroelect, Freq Contr. 1996;43(6):1111–1121. doi: 10.1109/58.542054.
- Gavrilov LR, Hand JW. A theoretical assessment of the relative performance of spherical phased arrays for ultrasound surgery. IEEE Trans Ultrason Ferroelectr Freq Control. 2000;47(1):125–139. doi: 10.1109/58.818755.
- De Bever J, Todd N, Payne A, et al. Adaptive model-predictive controller for magnetic resonance guided focused ultrasound therapy. Int J Hyperthermia. 2014;30(7):456–470. doi: 10.3109/02656736.2014.968223.
- Prakash P, Diederich CJ. Considerations for theoretical modelling of thermal ablation with catheter-based ultrasonic sources: implications for treatment planning, monitoring and control. Int J Hyperthermia. 2012;28(1):69–86. doi: 10.3109/02656736.2011.630337.
- Chen W, Zhu H, Zhang L, et al. Primary bone malignancy: effective treatment with high-intensity focused ultrasound ablation. Radiology. 2010;255(3):967–978. doi: 10.1148/radiol.10090374.
- Hyvärinen M, Huang Y, David E, et al. Comparison of computer simulations and clinical treatment results of magnetic resonance-guided focused ultrasound surgery (MRgFUS) of uterine fibroids. Med Phys. 2022;49(4):2101–2119. doi: 10.1002/mp.15263.
- Heydari M, Jahed M. Prediction of temperature distribution and volume of lesion during HIFU therapy. In: ITNG 2009—6th International Conference on Information Technology: New Generations; 2009; p. 1468–1473. doi: 10.1109/ITNG.2009.234.
- Meaney PM, Clarke RL, Ter Haar GR, et al. A 3-D finite-element model for computation of temperature profiles and regions of thermal damage during focused ultrasound surgery exposures. Ultrasound Med Biol. 1998;24(9):1489–1499. doi: 10.1016/S0301-5629(98)00102-1.
- Vyas U, Webb T, Bitton R, et al. Acoustic and thermal simulations of tcMRgFUS in patient specific models: validation with experiments. J Ther Ultrasound. 2015;3(S1):35. doi: 10.1186/2050-5736-3-S1-P35.
- Ellens N, Hynynen K. Simulation study of the effects of near- and far-field heating during focused ultrasound uterine fibroid ablation using an electronically focused phased array: a theoretical analysis of patient safety. Med Phys. 2014;41(7):072902. doi: 10.1118/1.4883777.
- Aubry J-F, Bates O, Boehm C, et al. Benchmark problems for transcranial ultrasound simulation: intercomparison of compressional wave models. J Acoust Soc Am. 2022;152(2):1003–1019. doi: 10.1121/10.0013426.
- Vyas U, Christensen D. Ultrasound beam simulations in inhomogeneous tissue geometries using the hybrid angular spectrum method. IEEE Trans Ultrason Ferroelectr Freq Control. 2012;59(6):1093–1100. doi: 10.1109/TUFFC.2012.2300.
- Pennes HH. Analysis of tissue and arterial blood temperatures in the resting human forearm. J Appl Physiol (1985). 1998;85(1):5–34. doi: 10.1152/jappl.1998.85.1.5.
- Todd N, Payne A, Parker DL. Model predictive filtering for improved temporal resolution in MRI temperature imaging. Magn Reson Med. 2010;63(5):1269–1279. doi: 10.1002/mrm.22321.
- Johnson SL, Christensen DA, Dillon CR, et al. Validation of hybrid angular spectrum acoustic and thermal modelling in phantoms. Int J Hyperthermia. 2018;35(1):578–590. doi: 10.1080/02656736.2018.1513168.
- Hansen M, Christensen D, Payne A. Experimental validation of acoustic and thermal modeling in heterogeneous phantoms using the hybrid angular spectrum method. Int J Hyperthermia. 2021;38(1):1617–1626. doi: 10.1080/02656736.2021.2000046.
- Leung SA, Webb TD, Bitton RR, et al. A rapid beam simulation framework for transcranial focused ultrasound. Sci Rep. 2019;9(1):7965. doi: 10.1038/s41598-019-43775-6.
- Treeby BE, Cox BT. k-wave: MATLAB toolbox for the simulation and reconstruction of photoacoustic wave fields. J Biomed Opt. 2010;15(2):021314. doi: 10.1117/1.3360308.
- Payne A, Merrill R, Minalga E, et al. A breast-specific MR guided focused ultrasound platform and treatment protocol: first-in-Human technical evaluation. IEEE Trans Biomed Eng. 2021;68(3):893–904. doi: 10.1109/TBME.2020.3016206.
- Adams-Tew SI, Johnson S, Odéen H, et al. Validation of a drift-corrected 3D MR temperature imaging sequence for breast MR-guided focused ultrasound treatments. Magn Reson Imaging. 2023;96:126–134. doi: 10.1016/j.mri.2022.12.006.
- Farrer AI, Odéen H, de Bever J, et al. Characterization and evaluation of tissue-mimicking gelatin phantoms for use with MRgFUS. J Ther Ultrasound. 2015;3(1):126. doi: 10.1186/s40349-015-0030-y.
- Maruvada S, Harris GR, Herman BA, et al. Acoustic power calibration of high-intensity focused ultrasound transducers using a radiation force technique. J Acoust Soc Am. 2007;121(3):1434–1439. doi: 10.1121/1.2431332.
- Dillon CR, Payne A, Christensen DA, et al. The accuracy and precision of two non-invasive, magnetic resonance-guided focused ultrasound-based thermal diffusivity estimation methods. Int J Hyperthermia. 2014;30(6):362–371. doi: 10.3109/02656736.2014.945497.
- Dillon CR, Borasi G, Payne A. Analytical estimation of ultrasound properties, thermal diffusivity, and perfusion using magnetic resonance-guided focused ultrasound temperature data. Phys Med Biol. 2016;61(2):923–936. doi: 10.1088/0031-9155/61/2/923.
- Svedin BT, Beck MJ, Hadley JR, et al. Focal point determination in magnetic resonance-guided focused ultrasound using tracking coils. Magn Reson Med. 2017;77(6):2424–2430. doi: 10.1002/mrm.26294.
- Hasgall PA, Di Gennaro F, Baumgartner C, et al. IT’IS database for thermal and electromagnetic parameters of biological tissues. Version 4.1, Feb 22, 2022, doi: 10.13099/VIP21000-04-1.itis.swiss/database
- International Commission on Radiation Units and Measurements. ICRU report 61: tissue substitutes, phantoms and computational modelling in medical ultrasound (ICRU Publications, Bethesda, MD); 1998.
- Renaud G, Callé S, Remenieras J-P, et al. Non-linear acoustic measurements to assess crack density in trabecular bone. Int J Non Linear Mech. 2008;43(3):194–200. doi: 10.1016/j.ijnonlinmec.2007.12.007.
- Goss SA, Frizzell LA, Dunn F. Ultrasonic absorption and attenuation in mammalian tissues. Ultrasound Med Biol. 1979;5(2):181–186. doi: 10.1016/0301-5629(79)90086-3.
- Lyons ME, Parker KJ. Absorption and attenuation in soft tissues II-experimental results. IEEE Trans Ultrason Ferroelectr Freq Control. 1988;35(4):511–521. doi: 10.1109/58.4189.
- Cortela GA, Von Krüger MA, Negreira CA, et al. Influence of ultrasonic scattering in the calculation of thermal dose in ex-vivo bovine muscular tissues. Ultrasonics. 2016;65:121–130. doi: 10.1016/j.ultras.2015.10.011.
- Tseng KK, Zhang R, Chen CM, et al. DNetUnet: a semi-supervised CNN of medical image segmentation for super-computing AI service. J Supercomput. 2021;77(4):3594–3615. doi: 10.1007/s11227-020-03407-7.
- Hectors SJCG, Jacobs I, Moonen CTW, et al. MRI methods for the evaluation of high intensity focused ultrasound tumor treatment: current status and future needs. Magn Reson Med. 2016;75(1):302–317. doi: 10.1002/mrm.25758.
- Johnson SL, Dillon C, Odéen H, et al. Development and validation of a MRgHIFU non-invasive tissue acoustic property estimation technique. Int J Hyperthermia. 2016;32(7):723–734. doi: 10.1080/02656736.2016.1216184.
- Farrer AI, Almquist S, Dillon CR, et al. Phase aberration simulation study of MRgFUS breast treatments. Med Phys. 2016;43(3):1374–1384. doi: 10.1118/1.4941013.