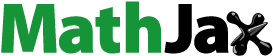
Abstract
Purpose
Radiomics may aid in predicting prognosis in patients with colorectal liver metastases (CLM). Consistent data is available on CT, yet limited data is available on MRI. This study assesses the capability of MRI-derived radiomic features (RFs) to predict local tumor progression-free survival (LTPFS) in patients with CLMs treated with microwave ablation (MWA).
Methods
All CLM patients with pre-operative Gadoxetic acid-MRI treated with MWA in a single institution between September 2015 and February 2022 were evaluated. Pre-procedural information was retrieved retrospectively. Two observers manually segmented CLMs on T2 and T1-Hepatobiliary phase (T1-HBP) scans. After inter-observer variability testing, 148/182 RFs showed robustness on T1-HBP, and 141/182 on T2 (ICC > 0.7).
Cox multivariate analysis was run to establish clinical (CLIN-mod), radiomic (RAD-T1, RAD-T2), and combined (COMB-T1, COMB-T2) models for LTPFS prediction.
Results
Seventy-six CLMs (43 patients) were assessed. Median follow-up was 14 months. LTP occurred in 19 lesions (25%).
CLIN-mod was composed of minimal ablation margins (MAMs), intra-segment progression and primary tumor grade and exhibited moderately high discriminatory power in predicting LTPFS (AUC = 0.89, p = 0.0001). Both RAD-T1 and RAD-T2 were able to predict LTPFS: (RAD-T1: AUC = 0.83, p = 0.0003; RAD-T2: AUC = 0.79, p = 0.001). Combined models yielded the strongest performance (COMB-T1: AUC = 0.98, p = 0.0001; COMB-T2: AUC = 0.95, p = 0.0003). Both combined models included MAMs and tumor regression grade; COMB-T1 also featured 10th percentile of signal intensity, while tumor flatness was present in COMB-T2.
Conclusion
MRI-based radiomic evaluation of CLMs is feasible and potentially useful for LTP prediction. Combined models outperformed clinical or radiomic models alone for LTPFS prediction.
Introduction
Colorectal liver metastases (CLM) strongly affect prognosis in colorectal cancer and are frequently diagnosed along the course of disease history [Citation1,Citation2]. Thermal ablation by either radiofrequency (RFA) or microwaves (MWA) has been endorsed by international guidelines in the management of CLM, along with other loco-regional treatments (LRTs) [Citation3]. In this scenario, thermal ablation is selected for curative treatment of CLMs up to 3 cm. Despite its widespread application and its uprising as a possible non-inferior alternative to surgical resection, oncological efficacy is still limited by the occurrence of local tumor progression, i.e., the disease recurrence abutting the ablation zone after evidence of a complete coverage of the tumor area [Citation4]. Numerous attempts have been made for elaborating predictive models of local tumor progression-free survival, whose main consistent predictors are tumor size and minimal ablation margins [Citation5,Citation6]; other factors such as mutational status and the impact of pre-ablation systemic chemotherapy have shown non-univocal results [Citation7,Citation8]; the net result is that local tumor progression cannot be fully explained by pure clinical approaches.
Radiomics, i.e., the quantitative analysis of volumetric imaging data, offers a potential means by which the composition of tumors may be explored, including possible microscopic features of tumor aggressivity [Citation9–11].
As precision medicine approaches in oncology progress, the application of radiomic-derived data on prognostic models have been implemented in several solid tumors, yielding interesting results when integrated with traditional clinical data [Citation12–14].
In the context of CLM ablation, application of radiomic-derived models has offered interesting data regarding prediction of LTPFS when studied on CT images [Citation15].
As MRI has higher sensitivity than CT for detection of CLM as well as a multiparametric characterization of lesions [Citation16,Citation17], radiomic analysis of MRI images may have a greater potential also for their prognostic characterization.
The objective of this research is to assess the capability of MRI-derived radiomic features (RFs) to predict local tumor progression-free survival (LTPFS) in patients with CLMs treated with microwave ablation (MWA).
Materials and methods
Patient population and study design
An institutional prospectively collected database of all patients who received microwave ablation of CLMs (September 2015–February 2022) was retrospectively reviewed. Inclusion criteria for analysis were the following: oligometastatic colorectal cancer, ≤ 10 CLMs, ablation performed alone or in combination with surgical resection to achieve liver disease eradication, availability of preoperative (<30 days) MRI with gadoxetic acid. Patient with a follow-up shorter than 6 months or with evidence of residual disease at first follow-up imaging (30–40 days) were excluded.
Since the objective of the study was strictly related to LTPFS, which is a CLM-related endpoint, each CLM was analyzed independently in patients with multifocal disease.
Clinical variables
Preoperative clinical and radiological data were recovered for all patients in the cohort. Clinical data included primary tumor TNM staging, RAS mutational status, localization along the lower gastrointestinal tract, clinical risk score [Citation18], history of previous CLM-directed therapies, history of systemic therapy. In patients undergoing concomitant resection and ablation, tumor regression grade (TRG) on histological specimens of resected metastases was also collected.
For each CLM, collected data included size, localization, synchronicity vs metachronicity relative to the diagnosis of primary CRC, radiomic-derived data on T1 and T2 images. Ablation time and power were recorded for each procedure and expressed as energy over tumor size (J/mm)
Image acquisition
MRI scans were acquired using a 1.5-T MRI (Achieva DStream, Philips Medical Systems, Best, The Netherlands) equipped with a multichannel coil.
The protocol included but was not limited to axial T2 weighted Tubo Spin Echo (TSE) Multivane XD images (2000 ms repetition time (TR), 100 ms echo time (TE), 400 × 400 mm field of view (FOV), 560 × 560 matrix, 5 mm section thickness, 0.5 mm gap, turbo factor = 39 and hepatobiliary-phase T1-weighted Fast Field Echo (FFE) fat sat DIXON (5,5 ms repetition time (TR), 3,8 ms echo time (TE),375 × 310 mm field of view (FOV), 400 × 400 matrix, 3 mm section thickness, obtained 20 min after injection of Gadoxetic acid disodium (Primovist, Bayer Schering Pharma, Berlin, Germany, 0.1 ml/kg) and 0.9% saline chaser (35 ml) administered as a bolus at a flow rate of 0.8 ml/s.
Ablation technique
All ablations were performed by experienced interventional radiologists either by the percutaneous approach or intraoperatively, as described previously by De Cobelli et al. [Citation8]. A 2450 MHz/100W Microwave EmprintTM ablation system with ThermosphereTM Technology (Medtronic, Minneapolis, MN, USA) was used. The MWA protocol was tailored to tumor size to achieve complete tumor coverage seeking at least 5 mm minimal ablation margins.
Tumor recurrence and follow-up
As per institutional protocol, a contrast-enhanced CT was acquired 1 month after ablation to define technique efficacy; subsequently, follow-up consisted in cross-sectional imaging (CT/MRI) every 3 months for the first year, then every 6 months.
Minimal ablation margins were measured according to the methodology provided by Wang et al. [Citation5]. First, the distance between the tumor and the nearest reliable landmark (including vessel bifurcations, benign lesions, capsula, surgical staples) was measured on preoperative imaging on all directions; then, the distance between the same landmark and the ablation zone was measured on the portal phase images of the CT obtained one month post-ablation.
The difference between the two distances was termed ‘Ablation Margin’; the smallest value among all the ablation margins calculated on all directions was termed ‘minimal ablation margin’ (MAM). MAM cutoffs of 5 and 10 mm were used in the analysis.
The definition of Local Tumor Progression (LTP) followed the standard terminology by Ahmed et al., i.e., appearance of disease foci abutting the ablation zone at follow-up [Citation4].
Texture analysis
Segmentation – The segmentation of CLMs was performed by two independent observers (A.D.C, diagnostic and interventional radiologist, and F.C., senior resident in Diagnostic Radiology; the two readers had respectively 7 and 5 years of experience in interpretation of abdominal MRI images) on T2 and T1-Hepatobiliary phase (T1-HBP) sequences. Segmentations were performed manually with a dedicated software (MIM Software Inc., Cleveland, OH, USA). The dual manual segmentation was performed to allow for subsequent quantification of inter-observer variability. Images and segmentations were exported as DICOM files to proceed with subsequent analysis.
Radiomic features extraction – Import of DICOM files exported to Matlab platform (MathWorks, Natick, MA, USA)was performed through the Computational Environment for Radiological Research (CERR) [Citation19,Citation20]. Radiomic features were extracted with SPAARC Pipeline for Automated Analysis and Radiomics Computing (SPAARC) [Citation21,Citation22], following the International Biomarker Standardization Initiative (IBSI) guidelines [Citation23]. Resampling of all images at cubic voxels of 1.5 × 1.5 × 1.5 mm3 with bilinear interpolation was performed directly from SPAARC settings pre-processing tools to minimize directional bias, according to IBSI recommendations. Image rebinning was implemented to optimize the RFs extraction process, as well as to minimize noise. Sixty-four bins were selected, in accordance with previous literature [Citation24,Citation25]. SPAARC allowed extraction of 182 first and higher order RFs: morphology, Statistical, Intensity Histogram (IG), Grey Level Run Length 3D_average (GLRL3D_avg), Grey Level Run Length 3D_combined (GLRL3D_comb), Grey Level Co-occurrence Matrix 3D_average (GLCM3D_avg), Grey Level Co-occurrence Matrix 3D_combined (GLCM3D_comb), Grey Level Size Zone Matrix 3D (GLSZM3D), Neighbors Grey Tone Difference Matrix 3D (NGTDM3D), Grey Level Distance Zone Matrix 3D (GLDZM3D).
Inter-observer variability – To test the robustness and reliability among features extracted from different contours the Intra-Class Correlation Coefficient was used (ICC) in accordance with other similar studies [Citation26,Citation27]. ICC is a metric based on the analysis of variance (ANOVA), ranging between 0 and 1 (which indicates, null and perfect reproducibility between observers, respectively). The ICC of a single feature was calculated from the feature values obtained from all contours and all patients, using a one-way random single-measure model. The threshold of ICC > 0.70 was considered as an indicator of ‘very good’ inter-observer agreement between, so that each radiomic feature with ICC <0.70 was discarded for subsequent analysis.
RFs redundancy limitation – A correlation-based filter was implemented in order to minimize the risk of redundancy: a Spearman coefficient threshold was set at 0.80 to discriminate redundant (≥0.80) and independent RFs (<0.80). The latter were included in further analysis; among the former, only the one with the lowest Univariate Logistic Regression p-value associated to LTPFS was selected.
Signal analysis– In this study all the scanner and the acquisition/reconstruction protocols used are the same for all patients. Consequently, it is likely that the signal normalization, which is a well-known issue in MRI images, can be overlooked. However, to exactly quantify the possible impact of a bias due to the signal variations on the radiomic features, two different ROIs were selected of both images’ series T1 and T2 representing a normal tissue as reference and a target. The first ROI was placed out of the tumor in the abdominal aorta, at the level of the L2 vertebra, where the signal is expected to be the same from each patient supposing there are not effects due to MRI scanner. Then another ROI was segmented in the lesion to investigate the impact of scanner also in the lesion. In addition, these structures have different range of gray-level intensities, covering different regions of the whole image histogram. Radiomic features were extracted from both regions and their variations around the mean value were quantified looking at the relative standard deviation. Subsequently, the changes of the values of individual features were analyzed in relation to fluctuations of the median intensity signal of the aorta. These trends were depicted in dispersion graphs because in cases of signal instability or other effects, a similar variation in the median signal from the aorta should also be reflected in the radiomic features extracted from the lesion itself, thus indicating an observable trend.
Statistical analysis
Standard descriptive statistics were utilized to describe patients and CLMs characteristics. Categorical variables were expressed as absolute number and presented as percentages; continuous variables were presented as mean ± standard deviation.
Machine learning-based bootstrap ranking was employed for variable selection, as previously described [Citation9,Citation13], to identify the best possible models among all possible variables combinations excluding variables that were found to be redundant and radiomic features not robust to segmentation, i.e., with ICC < 0.70. The Bootstrap was run 1000 times on the original dataset to obtain different populations with the same information content as the original. A maximum of three covariates were included in the Cox regression, in accordance with the number of events [Citation28–30]. All the possible combinations of three variables were tested with Cox Logistic Regression on each bootstrapped sample, and for each triplet, the frequency with which the overall model P-value was found < 0.05 out of 1000 was calculated. A ranking of those appearing most frequently with overall model Pvalue <0.05 was done, and the combination with higher frequency was chosen as the most ‘robust’ model. The first combination in the ranking including only clinical variables was retained to develop the most reliable clinical model (CLIN-mod). The same was done selecting two models including only radiomic variables for the T2 and T1-HBP MRI sequences (RAD-T1; RAD-T2) and two combined models including radiomic and clinical variables together (COMB-T1; COMB-T2). In this way, the variables for the 7 models were selected showing the best robustness and performance in terms of p-value and AUC among all available combinations. Therefore, for each model the Cox Regression was finally applied on the variables selected on the original dataset. A prognostic index was calculated according to the formula of risk of Multivariate Cox Regression:
Where Bi are the coefficients estimated by Cox Regression and Xi are the variables included in the model, which range from 1 to N. It is represented by the linear combination of each variable included, weighted with its Cox Regression coefficient. To evaluate the capability of Pindex to discriminate CLMs according to LTPFS, a threshold value was calculated as the Youden criterion value of ROC analysis, then all metastases were dichotomized in two risk classes. Finally, the Kaplan-Meier test was run to compare the two risk categories. Models’ performances were quantified in terms of: C-index (that is the AUC value of the overall fit model), Sensitivity, Specificity, Kaplan-Meier p-value and hazard ratio. Analyses were performed using homemade Matlab codes.
To test the models given the small sample size, the ‘internal validation’ criterion suggested by the TRIPOD guidelines was followed [Citation31]. The original sample was bootstrapped 1000 times again and subsequently, the P-indexes of the 7 models were calculated directly on the new populations and were tested with COX and ROC analysis on the 1000 samples to determine the median value and interquartile ranges of the p-value, C-index, and HR to assess the robustness of the results obtained in the original sample. It is worth noting that in this second bootstrap made for testing, the coefficients of the 7 models were fixed at those values obtained by the COX regression of the training. Therefore, they were simply tested on the 1000 samples to obtain the confidence intervals for the performance parameters obtained on the original dataset. However, in the first bootstrap used for selecting the best models, the coefficients of the Cox regressions were free.
Results
The study cohort included 43 patients with 76 CLM, consisting of 25 males (58%) and 18 females (42%). Mean age was 69 years (range 46 – 88); N = 6 patients were treated percutaneously (14%), whereas 37 (86%) were treated by a combined surgical-ablative approach during laparoscopy or laparotomy. Regarding notable CLM-related characteristics, mean tumor size was 12 mm, and minimal ablation margins (MAMs) were <5 mm in 23 nodules (30.3%). Population characteristics are summarized in .
Table 1. Population characteristics.
After a median follow-up of 14 months, local tumor progression was observed in 19/76 CLM (25%).
After Inter-observer variability testing, 148/182 radiomic features (81.3%) proved to be robust on T1-HBP and 141/182 radiomic features (77.5%) on T2 (ICC > 0.7).
Concerining signal analysis, the relative standard deviation of the radiomic features included in the model extracted from the aorta was around 2%, indicating signal stability in blood. As expected, the relative standard deviation of the radiomic features included in the model extracted from the lesion was larger, up to 14%, as suggested by lesion heterogeneity by itself.
The changes of the values of individual features were analyzed in relation to fluctuations of the median intensity signal of the aorta. In cases of signal instability, a similar variation in the median signal from the aorta and the lesions is expected. However, no correlation trends were detected between the variation of radiomic features and those of the signal in the aorta, thus excluding signal instability. Dispersion graphs depicting these trends were reported in the Supplementary Materials.
Multivariate analysis
Following ranking procedure, the best clinical model (CLIN-mod) was identified and was composed of MAMs, intra-segment progression and primary tumor grade (). CLIN-mod exhibited moderately high discriminatory power in predicting LTPFS (AUC = 0.89, p = 0.0001), . Upon Cox multivariate regression and Kaplan Meyer curve analysis, CLIN-mod categorized the risk of local tumor progression with a good performance [HR = 7.56 (95% CI = 2.42 − 23.61; p = 0.0005] ().
Figure 1. ROC curves for prediction of local tumor progression according to clinical model (green), pure radiomic model (red), and combined model (blue) on T1-HBP images (figure a) and T2 images (figure b), respectively.

Figure 2. Kaplan Meyer curve for low-risk (green) and high risk group (red) as identified by the clinical model on local tumor progression.

Looking at the ranking procedure applied to imaging-derived data, two pure radiomic models (RAD-T1, RAD-T2) and two mixed models (COMB-T1 and COMB-T2) for T1-HPB and T2 sequence images were obtained, respectively.
RAD-T1 included IH10, NGTDM3D-busyness and Morphology-COMshift () and predicted LTPFS with quite good discriminatory power (AUC = 0.83, p = 0.0003), , as well as a good performance in categorizing the risk of LTP on Cox multivariate regression and Kaplan-Meyer curve analysis [HR = 4.29 (95%CL = 1.54–11.96) p = 0.005, ].
COMB-T1 included minimal ablation margins, TRG and IH-percentile10 () with a very strong discriminatory power for predicting local progression (AUC = 0.98, p = 0.0001, ). The Kaplan Meier analysis confirmed significant separation of survival curves in the groups identified by COMB-T1 (, p = 0.0152)
Figure 3. Kaplan Meyer curve for low-risk (green) and high risk group (red) as identified by the T1-HBP-based (figure a) and T2-based (figure b) radiomic model on local tumor progression.

Figure 4. Kaplan Meyer curve for low-risk (green) and high risk group (red) as identified by the T1-HBP-based (figure a) and T2-based (figure b) combined model on local tumor progression.

RAD-T2 included GLSZM3D-smallZoneEmphasis, GLCM3D-sumEntropy, IH-entropy () and showed a good discriminatory power for LTPFS prediction (AUC = 0.79, p = 0.001, ). Cox multivariate regression and Kaplan Meier analysis confirmed its ability in stratifying the risk of LTP [HR = 4.14 (95%CL = 1.52–11.23) p = 0.005, ]. COMB-T2 comprised MAMs, TRG and flatness (); it demonstrated a very high performance in discrimination of local progression (AUC = 0.95, p = 0.0003). The Kaplan Meier analysis confirmed significant separation of survival curves in the groups identified by COMB-T2. (, p = 0.0078)
Regarding the internal validation, the bootstrap procedure confirmed the robustness of results as reported by Table S1, S2 and S3 of Supplementary Materials.
Table 2. Characteristics of the clinical model.
Table 3. Characteristics of T1-based models.
Table 4. Characteristics of T2-based models.
Discussion
In the present study, MRI-derived radiomic data showed a prognostic value when integrated to traditional predictive factors for local recurrence of colorectal liver metastases after microwave ablation.
After semi-manual contouring by two observers, more than 80% of extracted radiomic features exhibited a high concordance rate (>0.7) on both T2 and HBP-T1 sequences. This finding highlights the technical feasibility of MRI-radiomic analysis for colorectal liver metastases, since a large amount of radiomic features were retained as potential robust predictors. Most radiomic studies on CLM mainly focus on CT scan, mainly due to its higher availability. However, given the increasing role of locoregional treatments in management of CLM, clinical application of MRI in this setting has become predominant, therefore assessing prognosis from MRI-derived radiomics might have a stronger clinical impact. In particular, the relatively high concordance among observers may have been positively affected by the higher sensitivity of MRI in detection and characterization of CLMs, even when dealing with smaller lesions.
Furthermore, the possibility to assess different imaging sequences allows to achieve multiparametric tumor characterization also in the domain of texture features.
The potential superiority of MRI over CT has been partially demonstrated by Shur et al. when applied to tumor recurrence after surgical resection [Citation32].
Mixed models were the best at predicting local tumor progression-free survival: in particular, COMB-T1 comprised MAMs, TRG and IH10; in COMB-T2, the clinical covariates were retained, while IH10 was replaced by flatness.
Minimal Ablation Margins, in particular below 5 mm, are a known to predict local tumor progression [Citation6,Citation33]. In our study, we followed the methodology described by Wang et al. to calculate MAMs [Citation5]. Recent studies have emphasized the critical role of 3D softwares in the accurate assessment of ablation zones and margins post-procedure. These techniques could be implemented in future studies since they have been demonstrated to enhance the accuracy of these fundamental assessments, accounting for organ motion and deformation [Citation34–37].
TRG is an interesting addition to the local prognostic model. Tumor regression grade reflects the degree of pathological response to systemic chemotherapy and was calculated on resected CLMs in all those patients who underwent a concomitant surgical and ablative intervention. Lower TRG indicates higher degree of response and has demonstrated to be associated with better clinical outcome in patients resected for colorectal liver metastases [Citation38]. In our study cohort, the role of TRG in local tumor progression of concomitantly ablated CLMs may suggest a generally higher degree of local aggressivity of the oligometastatic disease.
In the combined model derived from T1-HBP images, IH10 was negatively related to the event; in other words, a tendency toward lower signal intensity is associated to a metastasis more susceptible to local progression. Heterogeneous enhancement on HBP images has been described in colorectal liver metastases [Citation39] and its occurrence may be at least partially explained by expression of organic anionic transporting polypeptide 1B3 (OATP1B3) negatively impacting prognosis [Citation40]. In our study cohort, metastases did not qualitatively exhibit enhancement given their relatively small size (mean 1.2 cm), but this result may unveil a tumor microstructure more prone to local aggressiveness.
In the T2 scenario, flatness is the most predictive radiomic feature. This radiomic feature, ranging from 0 to 1, expresses the ratio between maximal and minimal CLM diameter. A perfectly spherical volume has flatness equal to 1, whereas smaller values of flatness express increasingly flatter objects. As a result, since this study showed the flatness negatively correlated to the events, it suggests that the flatter the volume the higher the risk of local progression .This result is sound considering the utilization of thermosphere technology which produces a spherical ablation zone and may result in better covering of more spherical tumors, regardless of their size [Citation41].
Pure radiomic models achieved a good prognostic performance, even though they did not perform as well as the pure clinical one. This could be explained by the presence in the clinical model of intra-segment progression, i.e., appearance of tumor foci in the same liver segment but not abutting the ablation zone. Since intra-segment progression is observed during follow-up, it cannot function as an actual predictor, but its relationship with LTP, as proposed by a previous study [Citation8], may be a sign of a locally aggressive behavior of the tumor.
This study has several limitations. Despite the internal validation reported, the monocentric nature of the study, with limited sample size, requires replication in other data sets for external validity. Furthermore, the retrospective nature of the study implied high degree of variability in potentially confounding variables (e.g., previous chemotherapeutic regimens), as well as inconsistent availability of potentially prognostic variables (e.g., RAS mutational status, carcino-embronic antigen (CEA) at the moment of ablation).
Conclusion
The application of T1-HBP and T2-derived radiomic data for local prognosis of CLM after microwave ablation is a feasible and has a potential to provide an added value to a clinical prognostic model. An external validation in a larger cohort is warranted to confirm consistency of the results. If validated, a perspective application of the mixed model could be an interesting add-on to address therapeutic options when considering patients for a local therapy of oligometastatic disease. The large amount of data coming from CLM texture characterization may potentially be the object of correlation studies with other data coming from novel technologies, including characterization of tumor genome, immune landscape and the application of metabolic imaging biomarkers, particularly through the use of 18 F-FDG PET/CT, which has already demonstrated added value for evaluation of ablation efficacy and treatment guidance [Citation42–45]. Multi-level integration of such diverse data may eventually allow the extraction of even more complex and informative prognostic models.
Ethical approval
This study was performed in line with the principles of the Declaration of Helsinki. Approval was granted by the Ethics Committee of IRCCS Ospedale San Raffaele (Date: 12/09/2019; No: 137/INT/2019).
Consent to participate
Informed consent was obtained from all individual participants included in the study.
Author’s contributions
All authors contributed to study conceptualization. Methodology, data curation and formal analysis were performed by Angelo Della Corte, Martina Mori, Francesca Calabrese, Domenico Santangelo and Alessandro Pellegrini. The first draft of the manuscript was written by Angelo Della Corte and Martina Mori and all authors commented on previous versions of the manuscript. All authors read and approved the final manuscript.
Supplemental Material
Download MS Word (385.6 KB)Acknowledgments
Stephanie Steidler contributed to language editing.
Disclosure statement
No potential conflict of interest was reported by the author(s).
Data availability statement
The data that support the findings of this study are available from the corresponding author, A.D.C, upon reasonable request.
Additional information
Funding
References
- Khattak MA, Martin HL, Beeke C, et al. Survival differences in patients with metastatic colorectal cancer and with single site metastatic disease at initial presentation: results from South Australian clinical registry for advanced colorectal cancer. Clin Colorectal Cancer. 2012;11(4):1–11. doi: 10.1016/j.clcc.2012.06.004.
- Engstrand J, Nilsson H, Strömberg C, et al. Colorectal cancer liver metastases - a population-based study on incidence, management and survival. BMC Cancer. 2018;18(1):78. doi: 10.1186/s12885-017-3925-x.
- Cervantes A, Adam R, Roselló S, et al. Metastatic colorectal cancer: ESMO clinical practice guideline for diagnosis, treatment and follow-up. Ann Oncol. 2023;34(1):10–32. doi: 10.1016/j.annonc.2022.10.003.
- Ahmed M, Solbiati L, Brace CL, et al. Image-guided tumor ablation: standardization of terminology and reporting criteria-A 10-year update. Radiology. 2014;273(1):241–260. doi: 10.1148/radiol.14132958.
- Wang X, Sofocleous CT, Erinjeri JP, et al. Margin size is an independent predictor of local tumor progression after ablation of Colon cancer liver metastases. Cardiovasc Intervent Radiol. 2013;36(1):166–175. doi: 10.1007/s00270-012-0377-1.
- Han K, Kim JH, Yang SG, et al. A single-center retrospective analysis of periprocedural variables affecting local tumor progression after radiofrequency ablation of colorectal cancer liver metastases. Radiology. 2020;298(1):212–218. doi: 10.1148/RADIOL.2020200109.
- Calandri M, Yamashita S, Gazzera C, et al. Ablation of colorectal liver metastasis: interaction of ablation margins and RAS mutation profiling on local tumour progression-free survival. Eur Radiol. 2018;28(7):2727–2734. doi: 10.1007/s00330-017-5273-2.
- De Cobelli F, Calandri M, Della Corte A, et al. Multi-institutional analysis of outcomes for thermosphere microwave ablation treatment of colorectal liver metastases: the SMAC study. Eur Radiol. 2022;32(6):4147–4159. doi: 10.1007/s00330-021-08497-2.
- Mori M, Palumbo D, Muffatti F, et al. Prediction of the characteristics of aggressiveness of pancreatic neuroendocrine neoplasms (PanNENs) based on CT radiomic features. Eur Radiol. 2022;33(6):4412–4421. doi: 10.1007/s00330-022-09351-9.
- Gillies RJ, Kinahan PE, Hricak H. Radiomics: images are more than pictures, they are data. Radiology. 2016;278(2):563–577. doi: 10.1148/radiol.2015151169.
- Lambin P, Rios-Velazquez E, Leijenaar R, et al. Radiomics: extracting more information from medical images using advanced feature analysis. Eur J Cancer. 2012;48(4):441–446. doi: 10.1016/j.ejca.2011.11.036.
- Altazi BA, Fernandez DC, Zhang GG, et al. Investigating multi-radiomic models for enhancing prediction power of cervical cancer treatment outcomes. Phys Med. 2018;46:180–188. doi: 10.1016/j.ejmp.2017.10.009.
- Palumbo D, Mori M, Prato F, et al. Prediction of early distant recurrence in upfront resectable pancreatic adenocarcinoma: a multidisciplinary, machine learning-based approach. Cancers. 2021;13(19):4938. doi: 10.3390/cancers13194938.
- Panzeri MM, Losio C, Della Corte A, et al. Prediction of chemoresistance in women undergoing neo-adjuvant chemotherapy for locally advanced breast cancer: volumetric analysis of first-order textural features extracted from multiparametric MRI. Contrast Media Mol Imaging. 2018;2018:8329041–8329047. doi: 10.1155/2018/8329041.
- Taghavi M, Staal F, Gomez Munoz F, et al. CT-based radiomics analysis before thermal ablation to predict local tumor progression for colorectal liver metastases. Cardiovasc Intervent Radiol. 2021;44(6):913–920. doi: 10.1007/s00270-020-02735-8.
- Zech CJ, Korpraphong P, Huppertz A, et al. Randomized multicentre trial of gadoxetic acid-enhanced MRI versus conventional MRI or CT in the staging of colorectal cancer liver metastases. Br J Surg. 2014;101(6):613–621. doi: 10.1002/bjs.9465.
- Kim C, Kim SY, Kim M-J, et al. Clinical impact of preoperative liver MRI in the evaluation of synchronous liver metastasis of colon cancer. Eur Radiol. 2018;28(10):4234–4242. doi: 10.1007/s00330-018-5422-2.
- Fong Y, Fortner J, Sun RL, et al. Clinical score for predicting recurrence after hepatic resection for metastatic colorectal cancer: analysis of 1001 consecutive cases. Ann Surg. 1999;230(3):309–321. doi: 10.1097/00000658-199909000-00004.
- Deasy JO, Blanco AI, Clark VH. CERR: a computational environment for radiotherapy research. Med Phys. 2003;30(5):979–985. doi: 10.1118/1.1568978.
- Apte AP, Iyer A, Crispin-Ortuzar M, et al. Technical note: Extension of CERR for computational radiomics: a comprehensive MATLAB platform for reproducible radiomics research. Med Phys. 2018;45(8):3713–3720. doi: 10.1002/mp.13046.
- Whybra P, Parkinson C, Foley K, et al. Assessing radiomic feature robustness to interpolation in 18F-FDG PET imaging. Sci Rep. 2019;9(1):9649. doi: 10.1038/s41598-019-46030-0.
- Piazzese C, Foley K, Whybra P, et al. Discovery of stable and prognostic CT-based radiomic features independent of contrast administration and dimensionality in oesophageal cancer. PLOS One. 2019;14(11):e0225550. doi: 10.1371/journal.pone.0225550.
- Zwanenburg A, Vallières M, Abdalah MA, et al. The image biomarker standardization initiative: standardized quantitative radiomics for high-throughput image-based phenotyping. Radiology. 2020;295(2):328–338. doi: 10.1148/radiol.2020191145.
- Desseroit M-C, Tixier F, Weber WA, et al. Reliability of PET/CT shape and heterogeneity features in functional and morphologic components of non-small cell lung cancer tumors: a repeatability analysis in a prospective multicenter cohort. J Nucl Med. 2017;58(3):406–411. doi: 10.2967/jnumed.116.180919.
- Loi S, Mori M, Benedetti G, et al. Robustness of CT radiomic features against image discretization and interpolation in characterizing pancreatic neuroendocrine neoplasms. Phys Med. 2020;76:125–133. doi: 10.1016/j.ejmp.2020.06.025.
- Mori M, Benedetti G, Partelli S, et al. Ct radiomic features of pancreatic neuroendocrine neoplasms (panNEN) are robust against delineation uncertainty. Phys Med. 2019;57:41–46. doi: 10.1016/j.ejmp.2018.12.005.
- Belli ML, Mori M, Broggi S, et al. Quantifying the robustness of [18F]FDG-PET/CT radiomic features with respect to tumor delineation in head and neck and pancreatic cancer patients. Phys Med. 2018;49:105–111. doi: 10.1016/j.ejmp.2018.05.013.
- Peduzzi P, Concato J, Kemper E, et al. A simulation study of the number of events per variable in logistic regression analysis. J Clin Epidemiol. 1996;49(12):1373–1379. doi: 10.1016/S0895-4356(96)00236-3.
- Vittinghoff E, McCulloch CE. Relaxing the rule of ten events per variable in logistic and cox regression. Am J Epidemiol. 2007;165(6):710–718. doi: 10.1093/aje/kwk052.
- Steyerberg EW. Clinical prediction models: a practical approach to development, validation, and updating. Newyork (NY): Springer Science + Business Media, LLC; 2009.
- Collins GS, Moons KGM, Dhiman P, et al. Transparent reporting of a multivariable prediction model for individual prognosis or diagnosis (TRIPOD): the TRIPOD statement. BMJ. 2015;385(j 4):e078378–g7594. doi: 10.1136/bmj.g7594.
- Shur J, Orton M, Connor A, et al. A clinical-radiomic model for improved prognostication of surgical candidates with colorectal liver metastases. J Surg Oncol. 2020;121(2):357–364. doi: 10.1002/jso.25783.
- Shady W, Petre EN, Gonen M, et al. Percutaneous radiofrequency ablation of colorectal cancer liver metastases: factors affecting outcomes-a 10-year experience at a single center. Radiology. 2016;278(2):601–611. doi: 10.1148/radiol.2015142489.
- Zirakchian Zadeh M, Sotirchos VS, Kirov A, et al. Three-dimensional margin as a predictor of local tumor progression after microwave ablation: intraprocedural versus 4–8-week postablation assessment. J Vasc Interv Radiol. 2024;35(4):523–532.e1. doi: 10.1016/j.jvir.2024.01.001.
- Chlorogiannis D-D, Sotirchos VS, Georgiades C, et al. The importance of optimal thermal ablation margins in colorectal liver metastases: a systematic review and meta-analysis of 21 studies. Cancers . 2023;15(24):5806. doi: 10.3390/cancers15245806.
- Lin Y-M, Paolucci I, O’Connor CS, et al. Ablative margins of colorectal liver metastases using deformable CT image registration and autosegmentation. Radiology. 2023;307(2):e221373. doi: 10.1148/RADIOL.221373.
- Vasiniotis Kamarinos N, Gonen M, Sotirchos V, et al. 3D margin assessment predicts local tumor progression after ablation of colorectal cancer liver metastases. Int J Hyperthermia. 2022;39(1):880–887. doi: 10.1080/02656736.2022.2055795.
- Rubbia-Brandt L, Giostra E, Brezault C, et al. Importance of histological tumor response assessment in predicting the outcome in patients with colorectal liver metastases treated with neo-adjuvant chemotherapy followed by liver surgery. Ann Oncol. 2007;18(2):299–304. doi: 10.1093/annonc/mdl386.
- Bhayana R, Baliyan V, Kordbacheh H, et al. Hepatobiliary phase enhancement of liver metastases on gadoxetic acid MRI: assessment of frequency and patterns. Eur Radiol. 2021;31(3):1359–1366. doi: 10.1007/s00330-020-07228-3.
- Park SH, Kim H, Kim EK, et al. Aberrant expression of OATP1B3 in colorectal cancer liver metastases and its clinical implication on gadoxetic acid-enhanced MRI. Oncotarget. 2017;8(41):71012–71023. doi: 10.18632/oncotarget.20295.
- Alonzo M, Bos A, Bennett S, et al. The EmprintTM ablation system with ThermosphereTM technology: one of the newer next-generation microwave ablation technologies. Semin Intervent Radiol. 2015;32(4):335–338. doi: 10.1055/s-0035-1564811.
- Cornelis FH, Petre EN, Vakiani E, et al. Immediate postablation 18 F-FDG injection and corresponding SUV are surrogate biomarkers of local tumor progression after thermal ablation of colorectal carcinoma liver metastases. J Nucl Med. 2018;59(9):1360–1365. doi: 10.2967/jnumed.117.194506.
- Shyn PB, Cubre AJ, Catalano PJ, et al. F-18 FDG perfusion PET: intraprocedural assessment of the liver tumor ablation margin. Abdom Radiol. 2021;46(7):3437–3447. doi: 10.1007/s00261-021-02970-8.
- Zirakchian Zadeh M, Yeh R, Kunin HS, et al. Real-time split-dose PET/CT-guided ablation improves colorectal liver metastasis detection and ablation zone margin assessments without the need for repeated contrast injection. Cancers. 2022;14(24):6253. doi: 10.3390/cancers14246253.
- Zirakchian Zadeh M, Yeh R, Kirov AS, et al. Gradient-based volumetric PET parameters on immediate pre-ablation FDG-PET predict local tumor progression in patients with colorectal liver metastasis treated by microwave ablation. Cardiovasc Intervent Radiol. 2023;46(7):911–920. doi: 10.1007/s00270-023-03470-6.