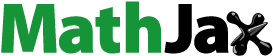
ABSTRACT
Climate extremes are threatening transportation infrastructures and hence require new methods to address their vulnerability and improve their resilience. However, existing studies have yet to examine the climate impacts on transportation networks systematically rather than independently assessing the infrastructures at a component level. Therefore, it is crucial to configure alternative shipping routes from a systematic perspective to reduce climate vulnerabilities and optimise the resilience of the whole shipping network. This paper aims to assess the global shipping network focusing on climate resilience by a methodology that combines climate risk indicators, centrality analysis and ship routing optimisation. The methodology is designed for overviewing the climate vulnerability of the current and future scenarios for comparison. First, a multi-centrality assessment defines the global shipping hubs and network vulnerabilities. Secondly, a shipping model is built for finding the optimal shipping route between ports, considering the port disruption days caused by climate change (e.g. extreme weather) based on the climate vulnerability analysis result from the first step. It contributes to a new framework combining the global and local seaport climate vulnerabilities. Furthermore, it recommends changing shipping routes by a foreseeable increase in port disruptions caused by extreme weather for climate adaptation.
1. Introduction
The global awareness for climate change impact in urbanisation is growing as their consequences become increasingly apparent. Seaports, which are crucial for human activities and lives, are exposed to different climate impacts, and they are commonly susceptible to storms and sea-level rise (Becker et al. Citation2013). In addition, Emergency Events Database (EM-DAT) has revealed that climate disasters have become more severe and frequent. From the 1970s to the 2010s, the damages caused by extreme hot weather has been increased by more than eight times, and those by storm and flooding have been increased by more than five times and eleven times, respectively (Panwar and Sen Citation2020). For example, during the 2017 Atlantic hurricane season, more than nine hurricanes were threatening North American and Caribbean areas. Until October, hurricanes, including the most powerful Maria, brought more than GBP 150 billion lost and 103 death toll in the US (Poo et al. Citation2018).
Therefore, researchers and practitioners have been interested in mitigating the climate change effects by reducing the carbon footprint of maritime transport. The footprints are managed by designing decision-making tools for speed control, berth scheduling, routing control for managing CO2 emission. Also, such pollutants as SOx and NOx from ships are policed to be reduced (Kim and Seo Citation2019). On the other hand, some scholars analyse climate change adaptation by investigating suitable adaptation measures for specific ports or regions. More than 100 relevant journal papers were featured in 65 internationally recognised journals between 2005 and 2018 (Wang et al. Citation2020), and it is necessary to implement decision-making tools for integrating the knowledge from different studies (Poo et al. Citation2018).
Port cities, including seaports and city zones, are risky to climate change as they lie along the coastline or other low-lying areas. Forty per cent of the global population are settling within 100 km along coastlines, and port cities, such as Shanghai, Rotterdam, and Singapore, concentrate the populations. Thirteen of the 20 most populated world cities are along the coasts. Extreme weather events, supercharged by climate change, affected approximately 62 million people worldwide in 2018. In addition, these port cities are crucial components for global economies as the total world seaborne trade has been tripled in the last 30 years (Becker et al. Citation2013).
Furthermore, extreme weather events can disrupt different ports, and hence a cascading breakdown of the involved shipping network(s) because the port cities mentioned above play essential roles for transhipments (Liu et al. Citation2018). For example, the high-risk level of marginalised ports will have less impact on the global network than the low-level risk of centralised nodes and vice versa.
It is undeniable that climate change can bring new opportunities for regional development (e.g. arctic shipping and coastal trade growth) and financial prosperity (e.g. the growth of red wine production towards Southern Australia regions). However, it has been witnessed with solid evidence that more extreme weather and climate risks have occurred with an increasing occurrence likelihood and more severe consequences in the past 50 years.
Therefore, climate impact or vulnerability assessments are not enough to independently focus on seaports, known as nodes of an integrated shipping network. To address this demand, network vulnerability studies from a global shipping system perspective are needed to test the network resilience from the failures in different seaports (Laxe, Seoane, and Montes Citation2012).
The upcoming part of the paper is organised as follows. In Section 2, the literature review takes place, and it includes vulnerability, resilience, centrality assessment in maritime transportation, and global in-port climate vulnerability and adaptation indices. Section 3 presents the centrality assessment for a global shipping network to calculate regional seaport vulnerability index, and an overall seaport vulnerability index is presented in Section 4 presents a resilience-based shipping routing optimisation modelling, and Section 5 includes a discussion on reducing climate vulnerabilities with in-port and inter-port perspectives by analysing the findings in Sections 3 and 4 holistically. Finally, Section 6 concludes the manuscript by highlighting its limitations and contributions.
2. Literature review
The literature review is split into three parts. First, vulnerability and resilience are defined for climate risks on maritime transportation. Second, a review on centrality assessment on maritime transportation is presented. Third, climate vulnerability assessments for port cities are presented.
2.1. Vulnerability and resilience
Transportation systems, including global shipping ones, usually have a network character. Therefore, other networks can refer to the resilience and vulnerability properties. Vulnerability is the adverse reaction of the system when they are facing hazardous events (Liu et al. Citation2018). So, it is an empirically proven concept for setting indices and measuring the risks faced by port cities in different aspects, including physical, social, and economic factors (Wang et al. Citation2020). By integrating all the factors, the levels of climate resilience can be obtained. Resilience can be split into two forms: the resistance strength of the whole system to threats and the recovery ability from disruptions (Proag Citation2014).
The increasing focus on vulnerability and resilience studies is ignited by the boost of adverse climate extreme events on transportation systems. shows the number of articles with the keyword string of ‘(transport or transportation) resilience’ and ‘(transport or transportation) vulnerability’, collected from keyword string search results of articles on the Web of Science year by year from 1996 to 2021 on 25 March 2022 by the authors using the analysis approach of Zhu and Liu (Citation2020). Both trends are steadily increased in the last two decades (Poo et al. Citation2018). While vulnerability-related articles increased about 50% in 2016 compared to 2015, resilience papers grow faster than vulnerability research. However, there is still no complete concept on the detailed definitions of resilience and vulnerability at the conceptual level (Mattsson and Jenelius Citation2015; Liu et al. Citation2018). Therefore, the relevant concepts from climate disaster management, transportation system, and engineering are collected to formulate the definitions of climate resilience and vulnerability of global shipping networks in this paper.
Figure 1. Trend of the transport vulnerability-related and resilience-related articles [Source: Web of science].
![Figure 1. Trend of the transport vulnerability-related and resilience-related articles [Source: Web of science].](/cms/asset/c52ac728-6b2f-48b7-be72-ad0621e7f5f8/tmpm_a_2094488_f0001_oc.jpg)
The Intergovernmental Panel on Climate Change (IPCC) focuses on climate change issues and solutions. It presents the dimensions of climate vulnerability and climate resilience. In the climate change context, vulnerability is the state or general quality of being harmed by extreme weather events (IPCC Citation2014). Dispositions take place as an internal nature of the affected regions. Resilience is described as the ability of the state or a part of the system to expect, engage with, and recover from the impacts of extreme weather events efficiently and effectively, including the possibilities in improving and restoring the existing system (Reggiani, Nijkamp, and Lanzi Citation2015). Then, resilience is coping with one more component, a fundamental sense of adaptive capacity, than vulnerability. It includes the positive features of reducing climate change and the risk posed by a particular hazard (IPCC Citation2012). The relation between the fundamental concepts of climate vulnerability and resilience is demonstrated in by IPCC.
Figure 2. Key concepts of climate vulnerability and resilience by intergovernmental panel on climate change [Source: IPCC].
![Figure 2. Key concepts of climate vulnerability and resilience by intergovernmental panel on climate change [Source: IPCC].](/cms/asset/1d78a568-19fc-4ca2-86bc-52ac5b62a234/tmpm_a_2094488_f0002_oc.jpg)
Scholars on transport systems have more and broader discussions on vulnerability and resilience compared to climate disaster management. Mattsson and Jenelius (Citation2015) and Reggiani, Nijkamp, and Lanzi (Citation2015) show comprehensive related articles and provide various definitions of vulnerability and resilience in transportation systems. It is necessary to reduce the vulnerability and strengthen the transport system’s resilience to obtain a better reputation and mitigate risk transfer costs as a source of competitive advantage (Kwak, Seo, and Mason Citation2018). Topological-based and system-based studies of transport networks are different fundamental types of related studies. The main advantage of topological-based studies is that they require less data input. However, the straightforwardness of the method makes the result less realistic. Then, a system-based approach is an alternative way for climate disaster management. Even though this approach provides comprehensive findings, this method is hungry for more data input such as travelling flows and related costs (Mattsson and Jenelius Citation2015). Furthermore, resilience requires including some socio-technical perspectives representing adaptation abilities (Becker et al. Citation2013). Furthermore, Reggiani, Nijkamp, and Lanzi (Citation2015) prove that connectivity is another core concept as transportation systems are similar to other networking systems.
The network vulnerability is also assessed for a shipping network, in which the analysis is driven by network robustness (Liu et al. Citation2018). It can also be described as the network robustness as the first resilience approach, known as resistance. Therefore, two terms for seaports or port cities in a global shipping network are described, ‘regional vulnerability’ (Taylor Citation2012) and ‘local vulnerability’ (Knoop et al. Citation2012). ‘Regional vulnerability’ is inversely proportional to the high centrality of a network (Laxe, Seoane, and Montes Citation2012). ‘Local vulnerability’ refers to the climate vulnerability experienced by the port infrastructures and operation stability. It can be known as the stability of the node facing extreme weather events (Zhang et al. Citation2016).
2.2. Centrality assessment in maritime transportation
Centrality assessment is commonly used for assessing the connectivity of shipping networks to provide a comprehensive reference for promoting balanced and sustainable development (Liu et al. Citation2018; Wu et al. Citation2019). Mccalla, Slack, and Comtois (Citation2005) provide a case study for container ports in Caribbean areas focusing on transhipment hubs. In 2009, Hu and Zhu (Citation2009) investigated the global shipping system by different measurements with network perspectives: links mean container liners and nodes mean ports. Ducruet et al. analyse the changes of possible hub ports in the Atlantic and Northeast Asia regions (Ducruet, Lee, and NG Citation2010), and then the results provide the evolution of hub ports and global shipping lines. In the same year, Kaluza et al. (Citation2010) analyse the shipping networks in three categories: container liner ships, dry bulk carriers, and others. Then, Ducruet and Zaidi (Citation2012) use the centrality assessment to define the relative position for the global network by different centralities in 2012. In the same year, Laxe, Seoane, and Montes (Citation2012) assess variations in the maritime transportation network upon the crisis, and Montes, Seoane, and Laxe (Citation2012) compare how general and containerised traffic has been added from 2008 to 2011. In 2015, Li, Xu, and Shi (Citation2015) define 25 geographical areas for analysing global shipping networks based on the new dividing areas. Then, Tovar, Hernández, and Rodríguez-déniz (Citation2015) make a case study to evaluate main Canarian ports on accessibility and connectivity. In 2016 and 2019, two case studies analyse the centrality and connectivity of the Maritime Silk Road (Zong and Hu Citation2016; Wu et al. Citation2019). Liu et al. (Citation2018) pioneer the analysis of vulnerabilities in shipping networks by centrality assessment. There are various tailored regional centrality assessments for specific regions, and therefore, it witnesses an increasing demand for a standardised framework for the systematic centrality assessment of global shipping networks. More importantly, the use of centrality indicators for the vulnerability analysis of maritime transport systems are still largely scanty in the current literature (Mansouri, Lee, and Aluko Citation2015).
2.3. Climate vulnerability assessment for port cities
Local vulnerability analysis is a more common topic compared to the regional one. Climate change vulnerability assessment and adaptation planning are trendy research topics. There are multiple focuses, including planning and operation, on transportation analyses (Sierra et al. Citation2017) and coastal areas analyses (Monioudi et al. Citation2018) They are different in terms of scales and dynamics to reflect different extreme weather events and social-economic factors. Most of them use a case study method for assessment and constrain the relevant studies to a single port or region assessment (Wan et al. Citation2018).
Hanson et al. (Citation2011) and Briguglio (Citation2010) provide an international insight for climate vulnerability studies, and they analyse the 136 port cities selected by the United Nations (UN). City selection is constrained to coastal cities with more than a million population. The global distribution of the selected port cities is centralised in Asia (38% or 52 ports). For the country level, China (10% or 14 ports) and the USA (13% or 17 ports) are the most concentrated countries.
The outcomes of the previous studies provide a comparative analysis on vulnerability and adaptation to assess climate risk by finalising the four categories of port cities. After mentioning the diversity in climate risks from different ports, it is necessary to provide a global shipping network analysis for assessing global climate resilience. The centrality assessment is used for the global vulnerability measurement, and the optimisation model is developed for estimating the new shipping routes based on the climate forecast.
The manuscript provides a method to foresee the future climate vulnerability on global shipping and forecasts future routing changes to fill the research gap. Therefore, a centrality assessment for regional seaport vulnerability analysis and a shipping routing optimisation model are provided in Section 3 and Section 4, respectively
3. Centrality assessment for regional seaport vulnerability analysis
Based on the graph theory, a network has two key components, which are nodes and links. The indices and categories set up by Briguglio (Citation2010) define a regional vulnerability index for each of the investigated seaport cities (Liu et al. Citation2018). To identify the regional vulnerability index for each port city, an advanced multi-centrality indicator which is designed by Wu et al. (Citation2019) to measure the importance, as known as hub nature, of ports by a more comprehensive analysis is applied in this paper to provide a global shipping network analysis for each of the 136 selected ports by UN for the first time as the following steps designed by Poo and Yang (Citation2020):
Data collection of global liner shipping
Modelling the global shipping network
Multiple centrality assessment
Validation of the results
Comparative analysis for regional vulnerability and local vulnerability
Providing seaport climate vulnerability index
3.1. Data collection of global liner shipping
Structuring a suitable global maritime transportation network is crucial for evaluating the shipping network’s climate vulnerability (Pape Citation2017). Therefore, it is necessary to set up a criterion to match seaports and their port cities before further investigation. The criterion is that a seaport within a 2-hour circle (i.e. about 200 km travelling distance) from a city can represent a city’s shipping demand. Then, the related information for the seaport is checked on Google Maps. For example, Tema Harbour is close to Accra in , and Thilawa Port is close to Yangon in (Google Citation2019).
After linking some rural ports to the associated cities, there are two mismatched port pairs. Using the above criterion, Hangzhou and Rabat can be represented by Ningbo and Casablanca ports, respectively, by the 2-hour circle rule. The 136 designated port cities are therefore reduced to 134 agglomerations.
The existing shipping route is used to construct the global shipping network, as shipping accounts for 80% of total exports and imports by volume globally (Mansouri, Lee, and Aluko Citation2015). Maersk shipping line data, in July 2019 from the official website, is chosen for sketching the unique shipping network. Maersk is chosen because it is the largest liner shipping company in the world, and it is sufficient to provide a worldwide shipping network for constructing the network. July data is chosen as Baltic Dry Index (BDI), which reveals that it presents more average traffic values than other months. The chosen port cities are undertaken for throughput data collection, and twenty transit ports are also selected by Poo and Yang (Citation2020) for utilising the network. It is because they are essential transhipment ports for the whole global shipping network. Six agglomerations do not link with routes, including Sapporo, Nampo, Natal, Maceio, Dhaka, and Belem. 2397 linkages are in the networks between all the chosen 134 ports and the other 20 transit ports. Thus, the network involving 154 ports needs to be formed and modelled.
3.2. Modelling the global shipping network
For assessing different centralities, UCINET 6, which is commonly used in Window, is chosen for analysing social network data (Borgatti, Everett, and Freeman Citation2002), and it is used in the coming analysis. For creating a network by using the tool, an adjacency matrix is created,
is defined by the route from port i to port j.
means no linkage, and
means otherwise. After inputting all
, the network is constructued as shown in . Due to the large number of nodes involved in the network, the centrality distribution of the nodes is visualised in , while the corresponding centralities of all the nodes are provided in and in Section 3.3.
Table 1. Degree rank, closeness rank, betweenness rank for the global shipping network.
Table 2. Multi-centrality ranking for the global shipping network.
3.3. Multiple centrality assessment
The port centrality analyses in this section are firstly investigated different centralities, and they represent the modelled global shipping network. The results are used to analyse the relationship between the 154 port cities. Degree centrality is defined as the total amount of linkages directly connecting to a port. The lesser the degree value of a port, the further away it is from other ports, and vice versa. The degree centrality of port i can be computed by EquationEquation (1)(1)
(1) :
where n represents the total ports within the network and presents the corresponding links between port i and j.
Closeness centrality means the shortest distances from all different ports to a target port, which indicates the centralised level of the target port in the network. The lesser the closeness centrality value, the more challenging it is to reach other port destinations within the network, and vice versa. The closeness centrality of port i is between zero and one and can be calculated by EquationEquation (2)(2)
(2) :
where n−1 represents the total numbers of ports in the system except the target port and presents the number of links between port i and j. In other words, it is the shortest distance between port i and j. Betweenness centrality describes whether the target port is in the middle of port s and t, which can reflect the ability of the node to be a hub in the network which can be calculated by using EquationEquation 3
(3)
(3) :
where is the number of times the port s and t passes the port i with the shortest distance and
is the total count of the shortest paths for port s and t.
To measure the overall impact of the 154 ports, a scoring system is designed by combining all three different indicators for further investigation and the Borda Count method I being used for such integration (Liu et al. Citation2018). The Borda Count method can accumulate different centrality properties into one rank while embedding easiness and visibility into the calculation process. The three different scores are firstly given by the three different centralities as shown in EquationEquations (4)(4)
(4) to (6):
The ranks of degree and closeness consider two directions together, and then two sets of results are collected based on two directions, inbound and outbound. The three independent scores have equal weights. By obtaining the overall rank, the importance of a port to the global shipping network can be presented, and the overall rank score can be obtained by EquationEquation (7)(7)
(7) :
Network efficiency and network average clustering coefficient are the chosen indicators for evaluating the finding of the multi-centrality indicator (Ducruet and Zaidi Citation2012; Liu et al. Citation2018; Wu et al. Citation2019). The clustering coefficient of an indicator is the density of its open neighbourhood. A graph consists of a set of vertices P and a set of edges E between them. An edge
connects two vertices,
and
. The neighbourhood
for a vertex
is assumed as its directly connected neighbours, as shown in EquationEquation (8)
(8)
(8) .
is the number of nodes,
as a vertex and
as a neighbour. Therefore, the local clustering coefficient for corresponding graphs is stated as EquationEquation (9)
(9)
(9) , and the network average clustering coefficient is presented as EquationEquation (10)
(10)
(10) .
Network efficiency is defined average distance or degree between two nodes. is the distance from node i to j, and the network efficiency is shown as EquationEquation (11)
(11)
(11) .
The ranks based on EquationEquations (4)(4)
(4) to (6) are shown in .
Degree Rank, Closeness Rank, Betweenness Rank for the global shipping network
Shanghai scores the highest in both degree rank and closeness rank, and Ningbo and Singapore are second and third, respectively. Singapore is at the top of the betweenness rank, and Shanghai and Ningbo score the second and third. Also, there are some important ports, including Rotterdam, Hong Kong, Guangzhou, and Busan, and they are both top 10 in three different ranks. By integrating all three ranks in , the multi-centrality ranking is shown in .
Multi-centrality
All the top 20 ports are ranked and categorised regarding their locations in , and there are half of them in East Asia. As a result, the obtained centrality scores can be used to present the regional vulnerabilities of all chosen agglomerations as described in Section 2. These regional vulnerability scores will be analysed with local vulnerability data set jointly in Section 4 for rationalising ship routing to configure the optimal climate resilience of the whole shipping network.
3.4. Validation of the centrality analysis results
For verifying the port ranking in , the port nodes are removed one by one for a time from the network. By eliminating a node within the network and observing its effect on the network efficiency, the global influence of the port can be obtained. Network efficiency and network clustering coefficient are chosen to validate the result of the multi-centrality ranking in Section 3.3. The top 20 agglomerations are taken away from the network accordingly and independently to observe the changes, as shown in . The agglomerations are listed from left to right according to their rank. The declines of both indicators are significant for Shanghai, Singapore, and Ningbo. The drops in network cluster coefficients are from 2.090% to 3.284%, and those of network efficiencies are from 0.832% to 1.313%. After that, the network cluster coefficient is reduced gradually from the fourth to the twentieth, and network efficiency changes are less significant as any individual impact is not more than 0.788%.
Figure 6. Drop of network efficiency and network clustering coefficient by removing an agglomeration.

Even the sequences of network efficiency and network cluster coefficient have shown slight variation, and they still witness the same result that the higher the multi-centrality rank, the higher the importance/impact the port has to the global shipping network. Therefore, the multi-centrality ranking is validated, and the result is used as the regional vulnerabilities to combine with local seaport vulnerability data for optimal shipping network resilience in the next section.
3.5. Comparative analysis for regional vulnerability and local vulnerability
To systematically understand the climate change influences on the global shipping network, regional vulnerability and local vulnerability are introduced to observe the difference between the influences inside and outside a port city. By assessing the centrality of seaports, the regional vulnerability of each seaport is assessed. Therefore, the local vulnerabilities of all 154 port cities are assessed in this section. The indices and categories set up by Briguglio are used for defining the local vulnerability index for each agglomeration (Briguglio Citation2010). He refers to the exposed population and assets from Nicholls et al. (Citation2008) on climate vulnerability and the UN Conference on Trade and Development data on the GDP per capita index, assumed to proxy adaptation abilities. By juxtaposing them, the extent to which climate change impacts each seaport can be assessed comprehensively.
Based on Briguglio (Citation2010) finding, port cities can be defined into four categories, ‘lowest-risk’ countries, ‘managed-risk’ countries, ‘mismanaged-risk’ countries and ‘highest-risk’ countries. Multi-centrality ranking is implied to all agglomerations. For the six-port cities without any connection, Dhaka, Belem, Maceio, Natal, Nampo, and Sapporo, rank the lowest in every single-centrality ranking, and thus the final one. The complete comparative analysis is shown in . The total score is the sum of all seaports scores with the same category, and the total rank is the sum of ranks of all seaports with the same category. Average scores and ranks of four categories are defined as dividing total scores and ranks by the number of seaports, and they are used to describe the different distribution patterns between local and regional vulnerability.
Table 3. Multi-centrality scoring and ranking of four local vulnerability categories.
Multi-centrality scoring and ranking of four local vulnerability categories
There is just one port city of Category 1 in the top 20. The average rank of Category 1 port cities is the lowest in terms of climate vulnerability throughout all four categories. The only top 20 agglomeration of Category 1 is Singapore. On the other hand, there are eight Category 4 in the top 20, and the average rank value of Category 4 port cities is the second highest. The top 20 agglomerations of Category 4 are from China and India. Therefore, regional vulnerabilities are not related to local vulnerabilities. However, some critical highest-risk port cities should put more effort into climate change adaptation. On the other hand, some lowest-risk port cities can take more essential positions of the global shipping network. Therefore, a vulnerability assessment, accompanying both local and regional vulnerabilities, is needed to observe the potential climate vulnerabilities of seaports.
3.6. Seaport climate vulnerability index
A new index, seaport climate vulnerability index-V, is designed for measuring the climate vulnerability by integrating local vulnerability index-X and regional vulnerability index-Y. In addition, V is developed by jointly connecting X and Y in EquationEquation (12)(12)
(12) . In terms of the two datasets, one is from multi-centrality assessment in Section 3 and the other from Briguglio (Citation2010). X is referred from the adaptation score
and vulnerability score
defined by Briguglio (Citation2010). The original local adaptability index
is defined by
subtracting by
in EquationEquation (13)
(13)
(13) . The lowest local adaptability index
among 134 seaports, excluding 20 transhipment ports from total 154 ports, is used to adjust all
to positive values and transform the data to X in EquationEquation (14)
(14)
(14) . The overall rank score
, defined in EquationEquation (7)
(7)
(7) , is used in EquationEquation (15)
(15)
(15) . The maximum overall rank score among 134 seaports is used to normalise the scores into Y.
After defining the seaport climate vulnerability index, all 134 seaports can be assessed by EquationEquations (12)(12)
(12) to (15). The result is shown in . The order of agglomeration in is different from that in . The cities in Category 4 have occupied the top 10 from China, India, Vietnam, and Thailand. On the other hand, the cities with high centrality in Category 1 and Category 2, such as Rotterdam, Hong Kong, and New York, rank out of the top 10.
Table 4. Seaport climate vulnerability index scoring and ranking
Seaport climate vulnerability index scoring and ranking
4. Shipping routing optimisation model
A shipping network model with all 154 seaports has been designed to find the optimum shipping route between ports based on their climate vulnerability indices and categories. Changes in route selections are obtained upon more port disruption days caused by extreme weather by referencing the data from Briguglio (Citation2010). The top 20 ports, known as hubs, are found in the centrality assessment are exclusively tested on changes to look at the sensitivity on shipping networks between continents.
4.1. Problem formulation
The formulas are listed by the adoption of notations in Section 4.1.1. The equations are listed in Section 4.1.2, and the assumptions are presented in Section 4.1.3.
4.1.1. Notations
The following notations are adopted in the following mathematical model.
Sets:
N Set of ports;
K Set of transhipments, including no transhipment;
Indices:
i, j Set of transhipments, including no transhipment;
k Indices of transhipment stages;
a Indices of starting port;
Indices of transhipment port;
c Indices of ending port;
Parameters:
Z Total time;
Total time from port i;
Travel time from port i to port j;
TT Total travel time;
Service time at port i;
ST Total service time;
Service time at port i;
Basic service time at port i;
Climate risk (CR) of a territory;
M Very large positive constant;
Decision variables
1 if directly travels from port i to port j; 0 otherwise;
Auxiliary variable associated with port i used for the sub-tour elimination constraint;
4.1.2. Equations
subject to
EquationEquation (16)(16)
(16) is the objective function, which describes the total time of all delivery routes between the set of ports. EquationEquation (17)
(17)
(17) represents the objective function of every single route, and the two components are travel time and service time. Constraint (18) defines the total travel time between the starting node, the k transhipment nodes, and the ending node, and Constraint (19) represents the whole service time between the starting node and the k transhipment nodes, and ending node. Constraints (20) define the service time of a node based on the climate performance index. Constraints (21) and (22) limit all nodes being visited only once or none in each period. Constraint (23) states the decision variables in the binary system for routing, and Constraint (24) ensures no internal movement within the same port. Constraint (25) is the sub-tour elimination constraint. Constraint (26) controls all the auxiliary variables larger than zero.
4.1.3. Assumptions
Some possible solutions can minimise Z, known as the accumulated shortest paths between all nodes. Thus, several assumptions take place for setting up the model:
The service time in the transhipment node is fixed, independent of cargo loading and unloading times;
The travel time between the starting node, transhipment nodes, and ending node is fixed;
The minimum service time is one day;
Port disruption implies a static delay and is represented in basic service time.
4.2. Results
Two types of assessments are used to present the results. Hub assessment is used to investigate the busy port cities of the whole global shipping system, and global overall network assessment is used to overview the changes of the global shipping networks.
4.2.1. Hub assessment
The busiest port cities, mentioned in , are further investigated as they contribute as key routes for the whole global shipping system. Then, the changes among all origin-destination (OD) pairs of 20 ports listed in are recorded. First, is given one day and runs the programme as the baseline. Then,
is given the values of three days in the short future and five days in the long future climate projection scenarios, and the positive and negative changes are recorded. It is set to be three and five days because, for example, Shanghai gives more than 2.5 disruption days per year in August (Zhang and Lam Citation2015). Thus, for assessing the global shipping hub changes, it is possible to investigate the changes of main shipping routes rather than just counting up the changes of transhipment hubs. 190 OD pairs, with two directions, drive the hub assessment, and the result is listed in . First, it is noticed that some nodes remain unchanged, including Shanghai, Shenzhen, Santos, and Houston. Then, some nodes have provided higher port calls in the near and long future, including Panama City, Rotterdam, Mumbai, and Tianjin. Except for Singapore Hong Kong, the port calls of remaining agglomerations have slightly dropped less than 12%. Hong Kong has a significant drop of 15.6% for the near future, and the counts of Singapore have a significant drop in 31.5% and 16.4%, respectively. The assessment provided an insight that the hub calls may not be affected by climate changes as there is no correlation between climate risk and changes. Therefore, it is concluded that some key routes are essential even if there are more disruptions in the future. Furthermore, it is necessary to investigate the whole shipping network changes by another assessment.
Table 5. Hub assessment summary.
Hub assessment summary
4.2.2. Global overall network assessment
11,935 OD pairs in between 154 port cities are assessed, and the program evaluates the whole network. Basic service time is altered to observe the possible route changes for the whole network by the same method used in the previous section, as shown in and . The total number of transhipments in the network is dropped by 14.22% in the near future and 19.12% in the long future. Then, some agglomerations with higher climate risks, such as Kuala Lumpur and Shanghai. Alternatively, some agglomerations with lower climate risks, such as Tokyo and Singapore, obtain more transhipment passing through them. It presents that the climate change risks affect the influences of the ports in the future.
Table 6. Rank of agglomerations with less port calls.
Table 7. Rank of agglomerations with higher port calls.
5. Discussion
The discussion can be split into two directions by defining global and local climate resilience. Local vulnerability has attracted more research interests in the past decade. More tailor-made adaptation plans for port cities should be designed for high-income and low-income port cities. Also, climate change will profoundly impact urban infrastructure systems, ecosystem services, and built environment. Hence, it could exacerbate existing social, economic, and environmental drivers. If the vulnerability assessment is considered in the scale mentioned by IPCC, agglomerations need to experience different extreme weathers, including extreme temperatures and extreme precipitation. Therefore, a more comprehensive international vulnerability assessment can be designed to assess the local vulnerability through the global shipping network.
From the analysis, it is evident that regional vulnerability is as crucial as local vulnerability. It is possible to reduce local vulnerability by new adaptation technologies. However, it is essential to enhance the resilience of the network by tackling regional vulnerability involving alternative routes. Weather-based routing optimisation, based on the methodology in this study, can provide recommendations for sailing under changing global weather forecasts. In other words, while each port can use their own resources to tackle their climate vulnerability at a local level, the transport authorities in the countries can use the global network resilience results to guide their investment being more rational. By evaluating network efficiency and network clustering coefficient properties in Section 3.4, decentralisation can increase system reliability, scale, and privacy. Therefore, the lowest-risk agglomerations mentioned in Section 4.1 should provide more routes to the global shipping network. However, geography is destiny as some port cities are necessary because of high populations and trades, such as Shanghai and New York. Also, straits and canals are crucial for cargo transhipments, and Singapore and Panama City are important port cities for global shipping and world trading. Therefore, the changes in ‘geography’ may be possible to diminish the vulnerability of the shipping network.
Also, new strategic shipping routes can be planned to reduce regional vulnerability, based on constructing new branch in the global shipping network model provided in the study. Furthermore, it has made more strategic contributions by being able to take into account future weather forecasting into the model to realise optimal shipping routes for today and future. Shipping companies can effectively use the model to proactively arrange their shipping routes and schedule to gain the best climate resilience for ensure their operation safety against climate change. For example, Arctic shipping routes, which are still mostly covered by sea ice, may be available for sailing during a part of the year, and lowest-risk port cities include some high latitude port cities, Montreal, Helsinki, Sapporo, Ulsan, Stockholm and Glasgow. Therefore, new shipping routes can be more critical and decentralise the world shipping network. Also, building up a new canal is a way to reduce the reliance on the existing straits and canals. For example, the Nicaragua canal can bear the workload and importance of the Panama Canal, and the Kra Canal can connect the Gulf of Thailand and Bay of Bengal. In addition, some new crucial port cities will take shape if the canals are constructed. Increasing the connections within the global shipping network can also be considered decentralisation to reduce the pressure of existing transhipment ports. Furthermore, the seaport climate vulnerability index can simulate the comprehensive decisions on shipping routing problems by combining the impact of both local and regional vulnerability.
6. Conclusion
This paper presents new ideas (i.e. simultaneous consideration of local level vulnerability and global network level resilience in Sections 3 and 4) and methods (i.e. climate risk based ship optimisation model in Section 4) for measuring the maritime transportation system’s recent and future climate risks by integrating climate risk indicators, centrality assessment, and a shipping routing model. Also, it shows the possible changes in shipping routing. Finally, except for reducing local vulnerability, some further regional assessments can be done as a new concept, climate-adapted decentralisation.
However, it is not enough to assess the climate resilience for seaports as climate sensitivity and adaptability are not assessed due to the lack of data. This manuscript uses secondary datasets holistically to form a new database for analysis, including shipping data from a shipping company and climate vulnerability data from other studies. For comprehensive assessment in future, if secondary data becomes unavailable, new climate vulnerability data can be collected through the interviews involving seaports and liner companies worldwide. Also, local vulnerability includes just climate threats from storming and sea-level rise in this article. Therefore, climate exposure is not fully assessed, and a more comprehensive global climate resilience framework is needed. The local climate vulnerability can be calculated by taking into account exposure, sensitivity, and vulnerability holistically. Hence, the vulnerability of each port can be more precisely expressed. Furthermore, regional vulnerability assessment with shipping routing modelling can focus on different regions and shipping companies, including fleet capacity and economic profit. Therefore, this paper pioneers a solution to integrating climate adaptation and routing modelling to enhance climate resilience in global shipping networks.
The proposed shipping network models can be tailored to tackle emerging risks of high impact on global shipping networks (e.g. COVID-19), in which both local node level risk and global network level resilience are required to be tackled simultaneously. More specifically, in terms of the methodology innovation, apart from assessing the climate risks for the shipping network, it can also be used to evaluate other issues or influences, such as COVID-19, if we can find related datasets or indicators for the nodes of a network being assessed. Therefore, their applications within the climate risk and resilience areas should not constrain their generality in other contexts.
Disclosure statement
No potential conflict of interest was reported by the authors.
Additional information
Funding
References
- Becker, A., M. Acciaro, R. Asariotis, E. Cabrera, L. Cretegny, P. Crist, M. Esteban, et al. 2013. “A Note on Climate Change Adaptation for Seaports: A Challenge for Global Ports, A Challenge for Global Society.” Climatic Change 120 (4): 683–696. doi:10.1007/s10584-013-0843-z.
- Borgatti, S. P., M. G. Everett, and L. C. Freeman. 2002. Ucinet for Windows: Software for Social Network Analysis, 6. Harvard, MA: analytic technologies.
- Briguglio, L. P. 2010. “Defining and Assessing the Risk of Being Harmed by Climate Change.” International Journal of Climate Change Strategies and Management 2 (1): 23–34. doi:10.1108/17568691011020238.
- Ducruet, C., S.-W. Lee, and A. K. Y. NG. 2010. “Centrality and Vulnerability in Liner shipping Networks: Revisiting the Northeast Asian Port Hierarchy.” Maritime Policy & Management 37 (1): 17–36. doi:10.1080/03088830903461175.
- Ducruet, C., and F. Zaidi. 2012. “Maritime Constellations: A Complex Network Approach to shipping and Ports.” Maritime Policy & Management 39 (2): 151–168. doi:10.1080/03088839.2011.650718.
- Google Maps. 2019. “Google Maps [Online]. Google Maps.” Available: https://www.google.co.uk/maps/dir/accra/tema+harbour/@5.6434247,-0.1548833,12z/data=!3m1!4b1!4m13!4m12!1m5!1m1!1s0xfdf9084b2b7a773:0xbed14ed8650e2dd3!2m2!1d-0.1869644!2d5.6037168!1m5!1m1!1s0x102078b84e1be8eb:0x51be0a7fbca0d94a!2m2!1d0.0166667!2d5.6333333?hl=en [Accessed 26 August 2019].
- Hanson, S., R. Nicholls, N. Ranger, S. Hallegatte, J. Corfee-morlot, C. Herweijer, and J. Chateau. 2011. “A Global Ranking of Port Cities with High Exposure to Climate Extremes.” An Interdisciplinary, International Journal Devoted to the Description, Causes and Implications of Climatic Change 104: 89–111.
- Hu, Y., and D. Zhu. 2009. “Empirical Analysis of the Worldwide Maritime Transportation Network.” Physica A: Statistical Mechanics and Its Applications 388 (10): 2061–2071. doi:10.1016/j.physa.2008.12.016.
- IPCC 2012. “Managing the Risks of Extreme Events and Disasters to Advance Climate Change Adaptation. Special Report of the Intergovernmental Panel on Climate Change (IPCC).” Cambridge University Press, New York, NY (United States).
- IPCC. 2014. Impacts, Adaptation, and Vulnerability. Part A: Global and Sectoral Aspects. Summary for Policymakers. C. B. Field, V. R. Barros, D. J. Dokken, K. J. Mach, M. D. Mastrandrea, T. E. Bilir, M. Chatterjee, et al.eds. Cambridge, United Kingdom and New York, NY, USA: Cambridge University Press.
- Kaluza, P., A. Kölzsch, M. T. Gastner, and B. Blasius. 2010. “The Complex Network of Global Cargo Ship Movements.” Journal of the Royal Society Interface 7 (48): 1093–1103. doi:10.1098/rsif.2009.0495.
- Kim, A. R., and Y.-J. Seo. 2019. “The Reduction of SOx Emissions in the Shipping Industry: The Case of Korean Companies.” Marine Policy 100: 98–106. doi:10.1016/j.marpol.2018.11.024.
- Knoop, V. L., M. Snelder, H. J. Van Zuylen, and S. P. Hoogendoorn. 2012. “Link-level Vulnerability Indicators for real-world Networks.” Transportation Research Part A: Policy and Practice 46: 843–854.
- Kwak, D. W., Y. J. Seo, and R. Mason. 2018. “Investigating the Relationship between Supply Chain Innovation, Risk Management Capabilities and Competitive Advantage in Global Supply Chains.” International Journal of Operations & Production Management 38 (1): 2–21. doi:10.1108/IJOPM-06-2015-0390.
- Laxe, F. G., M. J. F. Seoane, and C. P. Montes. 2012. “Maritime Degree, Centrality and Vulnerability: Port Hierarchies and Emerging Areas in Containerised Transport (2008–2010).” Journal of Transport Geography 24: 33–44. doi:10.1016/j.jtrangeo.2012.06.005.
- Li, Z., M. Xu, and Y. Shi. 2015. “Centrality in Global Shipping Network Basing on Worldwide Shipping Areas.” GeoJournal 80 (1): 47–60. doi:10.1007/s10708-014-9524-3.
- Liu, H., Z. Tian, A. Huang, and Z. Yang. 2018. “Analysis of Vulnerabilities in Maritime Supply Chains.” Reliability Engineering & System Safety 169: 475. doi:10.1016/j.ress.2017.09.018.
- Mansouri, S. A., H. Lee, and O. Aluko. 2015. “Multi-objective Decision Support to Enhance Environmental Sustainability in Maritime Shipping: A Review and Future Directions.” Transportation Research Part E: Logistics and Transportation Review 78: 3–18. doi:10.1016/j.tre.2015.01.012.
- Mattsson, L.-G., and E. Jenelius. 2015. “Vulnerability and Resilience of Transport Systems – A Discussion of Recent Research.” Transportation Research Part A: Policy & Practice 81: 16–35.
- Mccalla, R., B. Slack, and C. Comtois. 2005. “The Caribbean Basin: Adjusting to Global Trends in Containerisation.” Maritime Policy & Management 32 (3): 245–261. doi:10.1080/03088830500139729.
- Monioudi, I. N., R. Asariotis, A. Becker, C. Bhat, D. Dowding-Gooden, M. Esteban, L. Feyen, et al. 2018. “Climate Change Impacts on Critical International Transportation Assets of Caribbean Small Island Developing States (SIDS): The Case of Jamaica and Saint Lucia.” Regional Environmental Change 18 (8): 2211–2225. doi:10.1007/s10113-018-1360-4.
- Montes, C. P., M. J. F. Seoane, and F. G. Laxe. 2012. “General Cargo and Containership Emergent Routes: A Complex Networks Description.” Transport Policy 24: 126–140. doi:10.1016/j.tranpol.2012.06.022.
- Nicholls, R., S. Hanson, C. Herweijer, N. Patmore, S. Hallegatte, J. Corfee-morlot, J. Château, and R. Muir-wood 2008. “Ranking Port Cities with High Exposure and Vulnerability to Climate Extremes: Exposure Estimates.” IDEAS Working Paper Series from RePEc.
- Panwar, V., and S. Sen. 2020. “Disaster Damage Records of EM-DAT and DesInventar: A Systematic Comparison.” Economics of Disasters and Climate Change 4 (2): 295–317. doi:10.1007/s41885-019-00052-0.
- Pape, M. 2017. EU Port Cities and Port Area Regeneration . E. P. T. Tank ed.https://www.europarl.europa.eu/thinktank/en/document/EPRS_BRI(2017)603889
- Poo, M. C. P., Z. Yang, D. Dimitriu, and Z. Qu. 2018. “Review on Seaport and Airport Adaptation to Climate Change: A Case on Sea Level Rise and Flooding.” Marine Technology Society Journal 52 (2): 23–33. doi:10.4031/MTSJ.52.2.4.
- Poo, M. C. P., and Z. Yang, 2020, June. “Optimising the Climate Resilience of Shipping Networks.” In IAME 2020 Conference. Hong Kong.
- Proag, V. 2014. “The Concept of Vulnerability and Resilience.” Procedia Economics and Finance 18: 369–376. doi:10.1016/S2212-5671(14)00952-6.
- Reggiani, A., P. Nijkamp, and D. Lanzi. 2015. “Transport Resilience and Vulnerability: The Role of Connectivity.” Transportation Research Part A: Policy and Practice 81: 4–15.
- Sierra, J. P., A. Genius, P. Lionello, M. Mestres, C. Mösso, and L. Marzo. 2017. “Modelling the Impact of Climate Change on Harbour Operability: The Barcelona Port Case Study.” Ocean Engineering 141: 64–78. doi:10.1016/j.oceaneng.2017.06.002.
- Taylor, M. A. 2012. “Remoteness and Accessibility in the Vulnerability Analysis of Regional Road Networks.” Transportation Research Part A: Policy and Practice 46: 761–771.
- Tovar, B., R. Hernández, and H. Rodríguez-déniz. 2015. “Container Port Competitiveness and Connectivity: The Canary Islands Main Ports Case.” Transport Policy 38: 40–51. doi:10.1016/j.tranpol.2014.11.001.
- Wan, C., Z. Yang, D. Zhang, X. Yan, and S. Fan. 2018. “Resilience in Transportation Systems: A Systematic Review and Future Directions.” Transport Review 38 (4): 479–498. doi:10.1080/01441647.2017.1383532.
- Wang, T., Z. Qu, Z. Yang, T. Nichol, D. Dimitriu, and G. Clarke. 2020. “Climate Change Research on Transportation Systems: Climate Risks, Adaptation and Planning.” Transportation Research Part D: Transport and Environment 88: 202553. doi:10.1016/j.trd.2020.102553.
- Wu, J., D. Zhang, C. Wan, J. Zhang, and M. Zhang. 2019. Novel Approach for Comprehensive Centrality Assessment of Ports along the Maritime Silk Road, 0361198119847469.Transportation Research Record.2673(9): 461–470. doi:10.1177/0361198119847469
- Zhang, Y., and J. S. L. Lam. 2015. “Estimating the Economic Losses of Port Disruption Due to Extreme Wind Events.” Ocean and Coastal Management 116: 300–310. doi:10.1016/j.ocecoaman.2015.08.009.
- Zhang, R., X. Ran, C. Wang, and Y. Deng. 2016. “Fuzzy Evaluation of Network Vulnerability.” Quality and Reliability Engineering International 32 (5): 1715–1730. doi:10.1002/qre.1905.
- Zhu, J., and W. Liu 2020. “A Tale of Two Databases: The Use of Web of Science and Scopus in Academic Papers.” Scientometrics, pp.1–15.
- Zong, K., and Z. H. Hu. 2016. “Analysis of the Port Connectivity in Maritime Silk Road.” Journal of Guangxi University 41: 1423–1431.