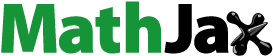
Abstract
Run orders in factorial designs have been a topic of interest in recent decades because the basic principle of randomization does not necessarily eliminate the bias caused by unknown factors and also generates many level changes, making experimentation more expensive. Therefore, the literature in this area has addressed the construction of preestablished run orders to eliminate the bias produced by unknown factors and/or minimize the cost of the experiment. This paper presents the results of a systematic literature review (SLR) and a taxonomical classification of studies about run orders for factorial designs published between 1952 and 2021. The objective here is to describe the findings and main and future research directions in this field. The main components considered in each study and the methodologies they used to obtain run sequences are also highlighted, allowing professionals to select an appropriate ordering for their problem. This review shows that obtaining orderings with good properties for an experimental design with any number of factors and levels is still an unresolved issue.
1 Introduction
Factorial experiments have numerous applications in many engineering, biology, chemistry, and industrial fields, among others. A basic principle of these experiments is carrying out experimental runs randomly so that possible effects generated by unknown factors affect not only some levels of the factors of interest but that they are distributed among all of them, thus avoiding errors in the analysis of their statistical significance (Box, Hunter, and Hunter Citation1978). However, conducting an experiment randomly can be very difficult and expensive due to the number of changes that must be made to factor levels. Additionally, random run orders may not be the most appropriate option to isolate the bias in the estimation of the effects generated by uncontrollable factors (Correa, Grima, and Tort-Martorell, Citation2012). Nevertheless, this issue can be resolved by generating run orders that guarantee low costs and good protection against the effects of unknown factors.
In this regard, Joiner and Campbell (Citation1976) presented examples that show the influence of unknown factors and the difficulty of making many level changes. One of these examples is a study by Joiner, where he needed to conduct an experiment to evaluate the accuracy of an installation that calibrated meters to sell cryogenic fluids (liquid nitrogen, liquid oxygen, etc.). In that context, it was known that meters deteriorated with use and the factors to be studied were temperature, pressure, flow rate, and total weight of the pumped liquid. In that case, a random order was discarded because changing the temperature and even pressure was too time-consuming; and, although flow speed and weight could be changed easily, the optimal ordering was not clear. A second example presented by Joiner and Campbell (Citation1976), who conducted an experiment to evaluate the effect of three variables on the amount of vitamin B2 in turnips. When they analyzed the results, they obtained randomly, they found that the data presented a 90% correlation with the time order in which they were collected, showing that a random ordering was not appropriate because the amount of vitamin B2 was affected by an unknown factor, i.e., time.
The search for run orders that provide a minimum number of level changes per factor and minimize the influence of unknown factors has been a topic of great interest in recent literature. The idea of not randomizing and including systematic orders in experimentation was first studied by Cox (Citation1952). Authors such as Box and Hay (Citation1953), Hill (Citation1960), Daniel and Wilcoxon (Citation1966), and Cheng and Jacroux (Citation1988) have generated run orders that only consider the bias associated with the influence of factors that are unknown. The criterion of the cost associated with changes in factor levels was subsequently added, as in the paper by Draper and Stoneman (Citation1968), who aimed to minimize the cost in factorial experiments with eight runs in which the main effects were linear trend-free and there was a small number of level changes. Dickinson (Citation1974) expanded the findings of Draper and Stoneman (Citation1968) for designs with 16 and 32 experiments with a minimum cost and bias. De León, Grima, and Tort-Martorell (Citation2005) proposed run orders for designs with 8 and 16 runs that combined a good protection against unknown factors in all the effects of the design with minimum level changes. Correa, Grima, and Tort-Martorell (Citation2009) presented an expansion algorithm for a and
factorial design with a minimum number of changes and protection for all the effects of the design against possible biases caused by unknown factors. This expansion method consists of expanding the matrix of an initial design
to add a new factor in a specific order and thus obtain a design
with desired properties. Bhowmik, Varghese et al. (Citation2017) presented an algorithm to generate run orders in any factorial design that produced a minimum number of level changes and provided protection against bias for the main effects of the design.
This shows that the topic of generating run orders in factorial designs has been investigated from different perspectives: minimizing experimental costs, renating biases, and considering both criteria together. In addition, later studies have addressed previous questions and added elements that are part of the reality of many industrial processes, improving the efficiency of the application of the experimental design.
This paper presents a systematic literature review (SLR) that was conducted to identify lines of research and knowledge gaps regarding the generation of run orders in factorial designs. This SLR highlights the most common approaches that have been used, the components that have been considered, and the methods that have been employed to generate the orderings found in the literature. The contribution of this article about sequential factorial designs is that, as it categorizes and summarizes the literature on the subject, it makes it easier for professionals in the area to identify a proposal that presents the order of execution that is most useful according to the characteristics of its design, this is because each problem is often solved using a single technique.
The remainder of this paper is organized as follows: Section 2 presents the methodology of the SLR; the research question; the search, inclusion, and exclusion criteria; and a taxonomic classification of the selected documents. Section 3 analyzes the findings obtained from the SLR. Section 4 suggests future research directions based on the SLR. Finally, Section 5 draws conclusions and offers recommendations.
2 Methodology
This SLR was conducted adopting the methodology developed by Kitchenham (Citation2004) in order to identify existing contributions to the implementation of run orders in factorial designs since research into this topic started. This methodology allowed us to investigate, identify, analyze, and summarize research results published between 1952 and 2021; answer questions; and identify future research directions. presents the stages of the SLR carried out in this study.
2.1 Research question
The literature shows a recent interest in the study of run orders for factorial designs, leaving random orders behind. Thus, it is important to determine how this topic has been investigated, especially the algorithms that have been designed to determine run orders that optimize the application of the experimental design in terms of the efficiency and accuracy of the results. Therefore, this study poses the following research question: What models or methodologies have been proposed to generate run orders in factorial designs?
2.2 Search process
The search process included papers published between 1952 and 2021 in journals indexed in high-impact international databases. The papers were searched in databases such as Scopus, Science Direct, Wiley, Springer, Taylor & Francis, IEEE, Jstore, and Emerald. Databases with certain reach in Latin America, such as Scielo, Redalyc, and Latindex, were also searched.
The search was conducted using the TITLE-ABSTR-KEY criterion for any publication year, and the broadest possible search algorithm was the following: (“level change” or “experimentation order” or “run sequence” or “run order”) and (“factorial design” or “factorial experiment” or “experimental design”). A total of 560 results were thus retrieved from the databases.
2.3 Inclusion and exclusion criteria
Two inclusion criteria were defined to select documents that address run orders in factorial designs: (1) the main topic of the paper is run orders and their background, and (2) it presents a methodology to construct run orders. In turn, documents with the following characteristics were excluded: (1) it studies or is focused on random run orders, or (2) it solves randomization constraints using known experimental designs, such as Split-Plot.
2.4 Classification of the studies
After applying the inclusion and exclusion criteria, a total of 61 documents were selected to study the evolution of this topic. First, the documents were classified based on their objective using the taxonomy in , which has three categories: (1) to minimize the influence of unknown factors, (2) to minimize the number of level changes, and (3) to minimize bias and the number of level changes. Three subcategories were defined inside the third category: (3.1) bias priority, (3.2) level changes priority, and (3.3) balance between bias and level changes.
The SLR served to identify the components most considered to generate run orders in factorial designs; they are summarized in . In addition, the SLR revealed different methodologies that have been applied to generate run orders, e.g., exhaustive, and semi-exhaustive search, the expansion algorithm, the foldover method, the use of optimality criteria, and algebraic theory.
Table 1: Components commonly considered in the construction of run orders.
3 Results analysis
This section aims to answer the research question formulated above about the methodologies that are used to generate run orders in factorial designs. shows the taxonomy created here to classify the 61 selected documents. If the document fits the category or subcategory, it is marked with an “X” in the corresponding cell in . As a result, it was found that 25 documents (41%) discuss run orders that aim only to reduce the bias associated with the possible effect of factors that are alien to the experimentation. Eleven papers (18%) balance the bias and the number of level changes. Ten documents (16%) consider both bias and level changes but place more importance on level changes. The other nine documents (15%) are about minimizing level changes in experimentation, and six documents (10%) prioritize bias but also consider level changes.
Table 2: Taxonomical classification of the selected documents.
Table 3: Components considered in the selected documents.
details the main components considered in each paper to develop run orders. The most worked component is linear bias, since 87% of the documents consider it. It is followed by level changes (59%), fractional designs (38%), bias of an order higher than linear (34%), estimation of interaction effects (33%), and more than two-factor levels (25%). Other components have been addressed in 20% or less of the documents: asymmetry in factor levels (20%), blocking (15%), experimentation costs that change depending on the factor (13%), and response surface (8%).
Regarding the methods used to generate run sequences, shows that the most commonly applied have been foldover and algebraic theory, both with a proportion of 21%, followed by the expansion method with 15%. To a lesser degree, these studies have used exhaustive search (8%), semi exhaustive search (7%), and other procedures (28%) such as optimality criteria and optimization methods, e.g., linear programming and the Traveling Salesman Problem (TSP).
Table 4: Methodologies for constructing run orders in the selected documents.
The following subsections, which correspond to the taxonomy above, detail the main contributions of each paper regarding the generation of run orders in factorial designs:
3.1 To minimize the influence of unknown factors
The studies in this category aimed to minimize or eliminate the possible bias generated by factors that were unknown to the experimentation, disregarding how expensive having a lot of level changes could be. Cox (Citation1952) was the first to introduce the idea of not randomizing runs because a systematic order can generate benefits in accuracy or convenience if practical knowledge of the experiment is available. In his study, he presented an example where he provided a specific order that guaranteed a lack of influence of unknown factors. Box and Hay (Citation1953) presented, in a bioassay, the idea of creating time-consecutive blocks so that the possible trend in the response was eliminated.
Hill (Citation1960) proposed an experimental design that eliminated the linear or quadratic influence of unknown factors, removing the effect of time. He exemplified this with an experiment conducted for the chemical industry with four factors and 14 runs. Daniel and Wilcoxon (Citation1966) suggested run orders for designs, with
, which eliminated the linear and/or quadratic influence of unknown factors. Said influence was measured by the time count, which consisted of the inner product between the column of the effect and the response-vector that simulated the linear influence of unknown factors. In that case, the most interesting factors were assigned to unbiased columns.
Cheng and Jacroux (Citation1988) developed and
designs where the estimations of main effects and some two-factor interactions were independent of linear trends. Steinberg (Citation1988) presented the use of ARIMA models to simultaneously estimate main and temporary linear trend effects for two-level factorial designs. Jacroux and SahaRay (Citation1990a, Citationb) explained the problem of sequentially assigning treatments to experimental units in time or space, in which there may be a polynomial trend, and developed run sequences to optimally estimate the effects for complete and fractional two-level factorial designs using a design of
rows and columns.
John (Citation1990) described and
factorial designs free of linear and quadratic time trends in the main effects and two-factor interactions using the principle of the foldover method. Cheng and Steinberg (Citation1991) presented trend robust run orders in two-level designs with 16 and 32 runs, which were modeled using a first order autoregressive model; however, their proposal presented many level changes. Jacroux and SahaRay (Citation1991) develop run orders robust against polynomial spatial or time trends for two-level factorial designs.
Bailey, Cheng, and Kipnis (Citation1992) unified and extended the construction of factorial designs to any prime number of levels and estimations of main effects and two-factor interactions resistant to polynomial trends using algebraic group theory. Coster (Citation1993b) modified the foldover method in order to develop run orders orthogonal to polynomial trends when the number of levels for each factor was not a prime number. They also presented a case in which different factors have different numbers of levels and interactions between two or more factors should be estimated.
Jacroux (Citation1994a) introduced a method to construct linear trend-resistant factorial row-column designs, where factors may have more than two levels, using the generalized foldover technique. On the same year, Jacroux (Citation1994b) developed a new method using algebraic theory to obtain run orders for mixed designs that usually produced minimum square estimators for main effects with a higher degree of polynomial trend resistance. He reported the results for and
designs.
Atkinson and Donev (Citation1996) introduced an algorithm to find run orders resistant to linear and quadratic trends through D-optimal designs, including simple treatment, multifactorial, and response surface designs. Martin, Jones, and Eccleston (Citation1998a) reported some results of designs with two-level factors in the case in which the resulting observations were correlated, which requires run orders that obtain efficient estimations. In addition, they found that maximizing the number of level changes was not always enough to guarantee high efficiency. They also extended these results to the case of factors with more than two levels. Elliott, Eccleston, and Martin (Citation1999) presented an algorithm to create optimal or almost optimal designs for autoregressive and moving average dependency structures. Martin, Eccleston, and Chan (Citation2004) studied designs with two-level factors when observations were spatially correlated, which showed that a big number of level changes is important to obtain robust designs and efficient estimations. Wang and Wu (Citation2013) proposed a simple approach to generate run sequences for fractional factorial designs with blocked trends using the foldover method. Singh, Thapliya, and Budhraja (Citation2016) developed a method to construct trend-free fractional factorial designs with main effects and some two-factor interactions by using the parity check matrix of a linear code. Núñez and Goos (Citation2019) designed a multi-stage algorithm to find trend-robust run orders for any factorial design and response surface designs with two to six factors.
3.2 To minimize the number of level changes
The studies in this subsection aimed to minimize the number of level changes required during the experimentation, thus reducing the cost but disregarding the effect of unknown factors. Tiahrt and Weeks (Citation1970) studied run orders for two-level factorial designs when there were restrictions in randomization and blocking. They demonstrated that the minimum number of level changes in a two-level factorial design was achieved by changing the level of a single factor between consecutive experiments. Cheng, Martin, and Tang (Citation1998) developed run orders with the minimum and the maximum number of level changes for two-level factorial designs. The orders they found with a minimum number of changes generated maximum bias, and the orders with a maximum number of changes enhances the robustness to different types of trends, allowing to see that as the number of changes increases, the bias (linear or polynomial) decreases and vice versa. Chen and Wang (Citation2001) continued the work of Wang and Jan (Citation1995) and Cheng, Martin, and Tang (Citation1998) by generalizing the search for a minimum number of changes for fractional factorial designs with an prime number of levels without considering the bias and using the foldover method.
Bhowmik et al. (Citation2015) described an exhaustive method to obtain run sequences for a symmetrical two-level factorial design with a minimum number of level changes. In addition, they presented a macro in Statistical Analysis System (SAS) software to more easily generate run orders based on their proposal. Bhowmik, Varghese, Jaggi, and Varghese (Citation2017), using the expansion method, found run orders that minimize the number of level changes by using SAS software for four kinds of response surface designs.
Peng and Lin (Citation2019) proposed an algorithm to design optimal run orders for any factorial design by using the TSP method, whose optimization criterion was the distance between experimental runs measured as the number of changes. Bhowmik et al. (Citation2019) constructed central composite designs and Box–Behnken response surface designs with a minimum number of level changes using expansion method. Chanda et al. (Citation2021) performed an exhaustive search to obtain all the possible run orders with the minimum number of changes for two-level experiments with three factors.
3.3 To minimize bias and the number of level changes
This subsection summarizes the studies that have aimed to minimize both bias and level changes classified into subcategories that reflect their priority.
3.3.1 Bias priority
Bias-prioritizing papers are those that seek to minimize bias and consider doing this with few (but not the least) changes. One of the papers was written by Coster and Cheng (Citation1988). They presented run orders in two- and three-level fractional factorial designs that aim to minimize a cost function associated with the level changes of the factors and that simultaneously exhibit main effects resistant to polynomial trends. For this purpose, they used the generalized foldover method. Mossadeq and Koblinsky (Citation1992) constructed run orders for the case of asymmetric levels with minimum bias by employing abelian group theory, which was also used to find orders with minimum cost.
Coster (Citation1993a) presented 125 run plans for two- and three-level fractional factorial designs with V resolution, using the generalized foldover method with trend-free main effects and a minimum number of level changes. Wang and Jan (Citation1995) described some rules to generate orthogonal arrays in complete and fractional designs without a linear or quadratic trend for main effects and two-way interactions. In addition, they sought to find the lowest number of level changes when costs were different depending on the factor.
Kim (Citation1997) modified the generalized foldover method and called it the “systematic selection of foldover vectors,” which selects, from a set of generators, those that obtain run orders with main effects and two-factor interactions free of unknown linear influences with the lowest number of changes. Tack and Vandebroek (Citation2002) presented an algorithm to construct designs that are optimal to eliminate time trends, while considering the cost associated with level changes and time count.
3.3.2 Level changes priority
The papers that prioritize the number of level changes are those that minimize the number of changes with as little bias as possible. One of the papers was written by Dickinson (Citation1974). He described run orders for designs with 16 and 32 runs with a minimum number of level changes, out of which he selected those with minimum linear influence of unknown factors on main effects, thus expanding the work carried out by Draper and Stoneman (Citation1968). De León (Citation2004) and De León, Grima, and Tort-Martorell (Citation2005) generated run orders for designs with 8 and 16 runs that provided a good level of protection against the influence of unknown factors and also the minimum number of factor level changes for complete and fractional designs, considering all the effects in the design, using exhaustive search. They also reinterpreted the definition of time count.
Along the same lines, Correa (Citation2007) and Correa, Grima, and Tort-Martorell (Citation2009) generated, using expansion method, run orders for two-level factorial designs with any number of runs, which provided a good level of protection against the influence of unknown factors and the minimum number of factor level changes, prioritizing the latter. Afterward, Correa, Grima, and Tort-Martorell (Citation2012) presented the best run sequences for designs with 32 experiments as well as sequences for experiments with 64 and 128 runs with excellent properties.
Angelopoulos, Evangelaras, and Koukouvinos (Citation2009) constructed run orders for runs and
factors that minimized the number of level changes in each factor using expansion method. They orders can be used to estimate main and interaction effects in a more efficient manner when there are linear and quadratic time trends.
3.3.3 Balance between bias and level changes
The studies that consider bias and level changes with equal importance are those that seek to reduce both criteria, but without reaching the minimum in either of them. Draper and Stoneman (Citation1968) reported (complete and fractional) factorial designs with eight runs that aimed to balance the number of level changes and the linear influence of unknown factors on main and interaction effects. They defined the time count and established that the response variable is run order, using an exhaustive method. Joiner and Campbell (Citation1976) presented a procedure to find a run order for designs with a balance between the number of level changes and bias by jointly evaluating a quality measure of the design and the cost associated with the level change, whose resulting value was represented graphically on a plane. Here they use the semi-exhaustive method.
Wang (Citation1991) presented a procedure to find the number of level changes and the degree of trend resistance in the columns of symmetric factorial designs, which can be used to establish run orders when the costs of level changes for different factors are different. Wang (Citation1996) also studied two- and four-level asymmetric fractional factorial designs, which was later generalized by Wang and Chen (Citation1998) for designs with any number of levels. Cui and John (Citation1998) presented a new technique using the expansion method to construct linear trend-free run orders for designs with a minimum number of level changes for all even positive integers S. Furthermore, for each odd positive integer, a run order of
was constructed with the minimum number of level changes, and whose time count did not increase along with k.
Hilow (Citation2013) compared four algorithms to find run sequences in factorial designs, analyzing them from the perspective of minimizing the number of level changes and bias. The four algorithms were reported by Correa, Grima, and Tort-Martorell (Citation2009), Cui and John (Citation1998), Cheng and Jacroux (Citation1988), and Coster and Cheng (Citation1988). Subsequently, Hilow (Citation2014) evaluated run orders for non-regular fractional factorial designs in designs with 12 experimental runs with 4 to 11 two-level factors in terms of multiple criteria and discussed how to combine these designs. Afterward, Hilow (Citation2015a) developed three categories of complete
factorial designs of resolution III, where the main effects and/or two-factor interactions were linear/quadratic trend free, with a minimum number of level changes or even lower than that of existing proposals. For this purpose, he used the generalized foldover method. Hilow (Citation2015b) also developed run orders for
fractional designs with resolution IV with the same properties as previously mentioned, using the foldover method.
Oprime, Miranda, and Conceição (Citation2017) discussed the use of systematic methods to generate designs with few level changes and robust to linear trends. They analyzed three approaches for two-level factorial designs (orthogonal and non-orthogonal), comparing systematic and random sequences, and found that a systematic order minimizes costs and to increased robustness to linear trend effects.
4 Future research directions
According to this SLR, future studies into the generation of run orders in factorial designs should incorporate the unexplored areas and designs with additional characteristics that reflect the set of possible situations that can take place in the practical implementation of experimental design and improve the efficiency of its application. This SLR allowed us to identify the following future research directions:
Optimization of run orders: Algorithms or analytical methods can be designed to improve the properties of previously proposed orders in specific situations, considering an efficiency measure regarding the number of level changes and/or bias associated with unknown factors. For example, Hilow (Citation2013) compared different algorithms for
designs, obtaining that none is universally better, everything depends on the objectives of study and experimental characteristics, allowing it to be possible make improvements to existing orders.
Differential economic costs: Run orders can be designed considering that economic costs associated with level changes are not equal for any factorial design. So far, differential costs have only been studied when factors have two and three levels to estimate main effects. This situation can be seen in works such as Wang and Jan (Citation1995), Kim (Citation1997), Tack and Vandebroek (Citation2002), and Hilow (Citation2015b).
Balance between bias and level changes for any factorial design: Future research can find run orders that balance the number of level changes and good protection against bias, minimizing both criteria simultaneously. Because recent studies as Bhowmik, Varghese, Jaggi, and Varghese (Citation2017), Bhowmik et al. (Citation2020), Núñez and Goos (Citation2019), and Peng and Lin (Citation2019) give more priority to one of them criteria or only consider one for designs with any number of factors and levels.
Response surface designs: Run orders can be generated for response surface designs considering differential costs per factor seeking to minimize both bias and level changes. Because the most recent proposals on this topic, such as Bhowmik, Varghese, Jaggi, Varghese, and Kaur (Citation2017) and Bhowmik et al. (Citation2019) only consider level changes, and others such as Núñez and Goos (Citation2019) consider bias, and in both cases with equal costs.
Optimal designs: Run orders can be generated for any factorial design using the theory of optimal designs, because this idea has begun implemented in works such as Elliott, Eccleston, and Martin (Citation1999), Tack and Vandebroek (Citation2002), and Quinlan and Lin (Citation2015), but it has not been developed in designs with more than two levels and any number of factors.
Artificial intelligence techniques: These techniques have not been examined and could fill gaps in the existing literature and/or improve the efficiency of previous results. Although optimization methods have been implemented in Núñez and Goos (Citation2019) and Peng and Lin (Citation2019).
Examining the reality of the industry: A great number of industrial processes include specific characteristics that are not considered in the existing literature about sequential design of experiments, for example, run orders that achieve a balance between the number of level changes and bias (with a good protection against linear and polynomial trends for all the effects of the design), a mixed number of levels, differential costs per factor, and fractional designs.
Comparing existing algorithms: The advantages and disadvantages of algorithms or methodologies designed to optimize run sequences in factorial designs should be further studied. This would enable us to identify, among existing proposals aimed at solving the same kind of situation, the most suitable option for an experiment with specific characteristics. This comparative has been carried out with depth only in Hilow (Citation2013).
Analyzing results obtained after the design has been applied: Future studies should investigate if existing techniques employed to interpret the results of sequential designs are effective to obtain valid conclusions or if more complex techniques are required to interpret their findings adequately because the existing literature does not address this topic.
5 Conclusions
This systematic literature review (SLR) was carried out in order to establish how the topic of generating run orders in factorial designs has been investigated from the start and to identify the methodologies that have been proposed for that purpose. Implementing run orders in factorial designs has proven to be more efficient than randomizing runs because it further reduces the bias in the estimation of effects and the practical cost is lower.
Run sequences have been widely studied by different authors around the world. Some have researched this topic from a theoretical perspective, and their main objective has been to eliminate bias. Others have adopted a more practical and engineering approach, and their main objective has been to obtain experimental results to improve manufacturing and service-related processes. Each of the proposals considers specific characteristics in the design, so combining proposals or using them in cases not specified in the documents would prevent the fulfillment of the desired properties.
This SLR analyzed 61 documents (about generating run orders in factorial designs) and identified recent contributions from renowned researchers in this field, thus answering the research question posed at the beginning of this paper. This SLR also found gaps in literature and established valuable future research directions in this field.
Run orders with desired properties can be generated by implementing well-known methodologies together with metaheuristics. This is due to the number of possible runs for a given design and the fact that multiple components should be considered to study the problem from a more realistic point of view. Thus, the results can be easily understood by non-expert users, and the proposed developments can improve industrial processes. This paper aims to contribute to the body of knowledge about the construction of run orders in factorial designs.
Competing interests
The authors report there are no competing interests to declare.
Correction statement
This article has been corrected with minor changes. These changes do not impact the academic content of the article.
References
- Angelopoulos, P., H. Evangelaras, and C. Koukouvinos. 2009. Run orders for efficient two-level experimental plans with minimum factor level changes robust to time trends. Journal of Statistical Planning and Inference 139 (10):3718–24. doi: 10.1016/j.jspi.2009.05.002.
- Atkinson, A., and A. Donev. 1996. Experimental designs optimally balanced for trend. Technometrics 38 (4):333–41. doi: 10.1080/00401706.1996.10484545.
- Bailey, R. A., C. S. Cheng, and P. Kipnis. 1992. Construction of trend-resistant factorial designs. Statistica Sinica 2 (2):393–411. doi: 10.2307/24304867.
- Bhowmik, A., E. Varghese, S. Jaggi, and C. Varghese. 2020. On the generation of factorial designs with minimum level changes. Communications in Statistics - Simulation and Computation 51 (6):3400–09. doi: 10.1080/03610918.2020.1720244.
- Bhowmik, A., E. Varghese, S. Jaggi, and C. Varghese. 2015. Factorial experiments with minimum changes in run sequences. Journal of the Indian Society of Agricultural Statistics 69 (3):243–55.
- Bhowmik, A., E. Varghese, S. Jaggi, and C. Varghese. 2017. Minimally changed run sequences in factorial experiments. Communications in Statistics – Theory and Methods 46 (15):7444–59. doi: 10.1080/03610926.2016.1152490.
- Bhowmik, A., E. Varghese, S. Jaggi, C. Varghese, and C. Kaur. 2017. On the generation of cost-effective response surface designs. Computers and Electronics in Agriculture 133:37–45. doi: 10.1016/j.compag.2016.11.020.
- Bhowmik, A., E. Varghese, S. Jaggi, C. Varghese, and S. Lall. 2019. On the construction of response surface designs with minimum level changes. Utilitas Mathematica 110:293–303.
- Box, G. E. P. 1952. Multi-factor designs of first order. Biometrika 39 (1-2):49–57. doi: 10.2307/2332462.
- Box, G. E. P., and W. A. Hay. 1953. A statistical design for the removal of trends occurring in a comparative experiment with an application in biological assay. Biometrics 9 (3):304–19. doi: 10.2307/3001706.
- Box, G., W. Hunter, and J. S. Hunter. 1978. Statistics for Experimenters. New York: Wiley.
- Chanda, B., A. Bhowmik, S. Jaggi, E. Varghese, A. Datta, C. Varghese, N. Das-Saha, A. Bhatia, and B. Chakrabarti. 2021. Minimal cost multifactor experiments for agricultural research involving hard-to-change factors. The Indian Journal of Agricultural Sciences 91 (7):97–100. doi: 10.56093/ijas.v91i7.115125.
- Chen, M. H., and P. C. Wang. 2001. Multi-level factorial designs with minimum numbers of level changes. Communications in Statistics: Theory and Methods 30 (5):875–85. doi: 10.1081/STA-100002263.
- Cheng, C., and M. Jacroux. 1988. The construction of trend-free run orders of two-level factorial designs. Journal of the American Statistical Association 83 (404):1152–8. doi: 10.1080/01621459.1988.10478713.
- Cheng, C. S., R. J. Martin, and B. Tang. 1998. Two-level factorial designs with extreme numbers of level changes. The Annals of Statistics 26 (4):1522–39. doi: 10.1214/aos/1024691252.
- Cheng, C. S., and D. M. Steinberg. 1991. Trend robust two-level factorial designs. Biometrika 78 (2):325–36. doi: 10.1093/biomet/78.2.325.
- Correa, A. 2007. Órdenes de experimentación en diseños factoriales. PhD diss., Universitat Politècnica de Catalunya.
- Correa, A., P. Grima, and X. Tort-Martorell. 2009. Experimentation order with good properties for 2n factorial designs. Journal of Applied Statistics 36 (7):743–54. doi: 10.1080/02664760802499337.
- Correa, A., P. Grima, and X. Tort-Martorell. 2012. Experimentation order in factorial designs: New findings. Journal of Applied Statistics 39 (7):1577–91. doi: 10.1080/02664763.2012.661706.
- Coster, D. C. 1993a. Tables of minimum cost, linear trend-free run sequences for two and three-level fractional factorial design. Computational Statistics & Data Analysis 16 (3):325–36. doi: 10.1016/0167-9473(93)90133-E.
- Coster, D. C. 1993b. Trend-free run orders of mixed-level fractional factorial designs. The Annals of Statistics 21 (4):2072–86. doi: 10.1214/aos/1176349410.
- Coster, D. C., and C. S. Cheng. 1988. Minimum cost trend-free run orders of fractional factorial designs. The Annals of Statistics 16 (3):1188–205. doi: 10.2307/2241626.
- Cox, D. R. 1952. Some recent work on systematic experimental design. Journal of the Royal Statistical Society: Series B (Methodological) 14 (2):211–9. doi: 10.1111/j.2517-6161.1952.tb00114.x.
- Cui, X., and P. W. John. 1998. Time-trend free run orders with the minimum level changes. Communications in Statistics – Theory and Methods 27 (1):55–68. doi: 10.1080/03610929808832650.
- Daniel, C., and F. Wilcoxon. 1966. Factorial 2p−q plans robust against linear and quadratic trends. Technometrics 8 (2):259–78. doi: 10.1080/00401706.1966.10490346.
- De León, G. 2004. Análisis y propuestas sobre algunos aspectos de la aplicación del diseño de experimentos en la industria. PhD diss., Universitat Politècnica de Catalunya.
- De León, G., P. Grima, and X. Tort-Martorell. 2005. Experimentation order in factorial designs with 8 or 16 runs. Journal of Applied Statistics 32 (3):297–313. doi: 10.1080/02664760500054731.
- Dickinson, W. 1974. Some orders requiring a minimum number of factor level changes for 24 and 25 main effects plans. Technometrics 16 (1):31–7. doi: 10.2307/1267489.
- Draper, N. R., and D. M. Stoneman. 1968. Factor changes and linear trends in eight-run two-level factorial designs. Technometrics 10 (2):301–11. doi: 10.2307/1267045.
- Elliott, L. J., J. A. Eccleston, and R. J. Martin. 1999. An algorithm for the design of factorial experiments when the data are correlated. Statistics and Computing 9 (3):195–201. doi: 10.1023/A:1008965829964.
- Hill, H. M. 1960. Experimental designs to adjust for time trend. Technometrics 2 (1):67–82. doi: 10.1080/00401706.1960.10489881.
- Hilow, H. 2013. Comparison among algorithms for sequencing runs of the full 2n factorial experiment. International Journal of Experimental Design and Process Optimization 3 (4):397–406. doi: 10.1016/j.csda.2012.09.013.
- Hilow, H. 2014. Minimum cost linear trend-free 12-run fractional factorial designs. Journal of Applied Statistics 41 (4):802–16. doi: 10.1080/02664763.2013.856384.
- Hilow, H. 2015a. Minimum cost linear trend free 2n−(n−k) designs of resolution IV. Communications in Statistics – Theory and Methods 44 (15):3158–72. doi: 10.1080/03610926.2013.819925.
- Hilow, H. 2015b. Three categories of minimum cost systematic full 2n factorial designs. Journal of Statistical Theory and Practice 9 (3):463–78. doi: 10.1080/15598608.2014.932728.
- Hilow, H., and I. Al-Maayteh. 2021. Three categories of minimum cost trend fesistant 2n factorial experiments derivable from the normalized Sylvester 0 Hadamard matrices of size 2n×2n. Journal of Statistical Theory and Practice 15 (3):1–20. doi: 10.1007/s42519-021-00198-9.
- Jacroux, M. 1994a. On the construction of trend-resistant fractional factorial row-column designs. Sankhya: The Indian Journal of Statistics, Series B 56 (2):251–8. doi: 10.2307/25052842.
- Jacroux, M. 1994b. On the construction of trend resistant mixed level factorial run orders. The Annals of Statistics 22 (2):904–16. doi: 10.2307/2242297.
- Jacroux, M., and R. SahaRay. 1990a. On construction of trend–free row-column 2-level factorial experiments. Metrika 37 (1):163–80. doi: 10.1007/BF02613518.
- Jacroux, M., and R. SahaRay. 1990b. On the construction of trend-free run orders of treatments. Biometrika 77 (1):187–91. doi: 10.2307/2336061.
- Jacroux, M., and R. SahaRay. 1991. Run orders of trend resistant 2-level factorial designs. Sankhya: The Indian Journal of Statistics, Series B 53 (2):202–12. doi: 10.2307/25052692.
- John, P. W. 1990. Time trends and factorial experiments. Technometrics 32 (3):275–82. doi: 10.2307/1269104.
- Joiner, B. L., and C. Campbell. 1976. Designing experiments when run order is important. Technometrics 18 (3):249–59. doi: 10.2307/1268734.
- Ju, H. L., and J. M. Lucas. 2002. L k factorial experiments with hard-to-change and easy-to-change factors. Journal of Quality Technology 34 (4):411–21. doi: 10.1080/00224065.2002.11980173.
- Kim, K. 1997. Construction and analysis of linear trend-free factorial designs under a general cost structure. PhD diss., Virginia Polytechnic Institute and State University.
- Kitchenham, B. 2004. Procedures for performing systematic reviews. Keele University Technical Report TR/SE-0401 ISSN:1353-7776. Joint Technical Report. Australia: Department of Computer Science, Keele University.
- Martin, R. J., J. A. Eccleston, and B. S. P. Chan. 2004. Efficient factorial experiments when the data are spatially correlated. Journal of Statistical Planning and Inference 126 (1):377–95. doi: 10.1016/j.jspi.2003.08.001.
- Martin, R. J., G. Jones, and J. A. Eccleston. 1998a. Some results on two-level factorial designs with dependent observations. Journal of Statistical Planning and Inference 66 (2):363–84. doi: 10.1016/S0378-3758(97)00086-4.
- Martin, R. J., G. Jones, and J. A. Eccleston. 1998b. Some results on multi-level factorial designs with dependent observations. Journal of Statistical Planning and Inference 73 (1-2):91–111. doi: 10.1016/S0378-3758(98)00054-8.
- Mossadeq, E., and A. Koblinsky. 1992. Run orders and quantitative factors in asymmetrical designs. Applied Stochastic Models and Data Analysis 8 (4):259–81. doi: 10.1002/asm.3150080403.
- Núñez, J., and P. Goos. 2019. An integer linear programing approach to find trend-robust run orders of experimental designs. Journal of Quality Technology 51 (1):37–50. doi: 10.1080/00224065.2018.1545496.
- Oprime, P. C., V. M. Miranda, and S. Conceição. 2017. Systematic sequencing of factorial experiments as an alternative to the random order. Gestão & Produção 24 (1):108–22. doi: 10.1590/0104-530X1266-16.
- Peng, J., and D. K. L. Lin. 2019. Construction of optimal run order in design of experiments. Journal of Quality Technology 51 (2):159–70. doi: 10.1080/00224065.2018.1541381.
- Quinlan, K. R., and D. K. J. Lin. 2015. Run order considerations for Plackett and Burman designs. Journal of Statistical Planning and Inference 165:56–62. doi: 10.1016/j.jspi.2015.04.001.
- Singh, P., P. Thapliya, and V. Budhraja. 2016. A technique to construct linear trend free fractional factorial design using some linear codes. International Journal of Statistics and Mathematics 3 (1):73–81.
- Steinberg, D. M. 1988. Factorial experiments with time trends. Technometrics 30 (3):259–69. doi: 10.2307/1270080.
- Tack, L., and M. Vandebroek. 2002. Trend resistant and cost-efficient block designs with fixed or random block effects. Journal of Quality Technology 34 (4):422–36. doi: 10.1080/00224065.2002.11980174.
- Tiahrt, K. J., and L. Weeks. 1970. A method of constrained randomization for 2k factorial. Technometrics 12 (3):471–86. doi: 10.2307/1267197.
- Wang, P. C. 1991. Symbol changes and trend resistance in orthogonal plans of symmetrical factorial. Sankhya: The Indian Journal of Statistics, Series B 53 (3):297–303. doi: 10.2307/25052704.
- Wang, P. C. 1996. Level changes and trend resistance in LN(2p4q) orthogonal arrays. Statistica Sinica 6:471–9. doi: 10.2307/24306028.
- Wang, P. C., and M. H. Chen. 1998. Level changes and trend resistance on replacement in asymmetric orthogonal arrays. Journal of Statistical Planning and Inference 69 (2):349–58. doi: 10.1016/S0378-3758(97)00168-7.
- Wang, P. C., and H. W. Jan. 1995. Designing two-level factorial experiments using orthogonal arrays when the run order is important. The Statistician 44 (3):379–88. doi: 10.2307/2348709.
- Wang, P. C., and S. Wu. 2013. Trend-free designs in blocked factorial experiments. Communications in Statistics – Theory and Methods 42 (10):1870–8. doi: 10.1080/03610926.2011.597917.