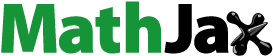
ABSTRACT
The fierce competition among brands on online marketplaces makes the optimization of offerings within this context a significant challenge. To address this challenge, we draw upon network theory and model the degree of competition through consumers’ consideration sets. We use a large empirical dataset from one of the biggest online marketplaces to explore the dynamic relationship between network position and the degree of competition, and we depict the redistribution of market share of related offerings after adjusting their array. In doing so, we provide a theoretical reference on when and how brands should optimize their product offerings on online marketplaces. We further demonstrate that intra-brand cannibalization relations have a significantly greater impact on the degree of competition compared to inter-brand ones, while intra-brand cannibalization relations represent the main reason for fluctuations in the degree of competition. Hence, contrary to existing theoretical insights and practical intuitions, our findings demonstrate that brands should minimize the number and heterogeneity of their offerings within a market segment to increase their sales on online marketplaces.
Introduction
The recent pandemic has drastically changed contemporary social and economic life, while the related implemented policies around the globe have put enormous pressure on the e-commerce resilience [Citation62]. The resulting uncertainties have, thus, increasingly led brands to migrate their business endeavors to online marketplaces; for instance, the largest retailer in the United States has currently more than 150,000 brands in its online marketplace, almost four times more than before the pandemic.Footnote1 Such an aggregation of brands has naturally led to fiercer competition on online marketplaces. To improve their degree of competition on online marketplaces, therefore, brands broadly adopt the competitive strategy of product proliferation [Citation52, Citation57], which can attract consumers from rivals and increase revenue as well as market share, leading to a further increase in the degree of competition amongst brands (inter-brand competition) [Citation52]. Product proliferation, however, can also result in a cannibalization effect, where products of a brand can be perceived as substitutes by consumers (intra-brand cannibalization) [Citation42]. A stronger cannibalization effect can lead to a long tail in the sales distribution of a brand [Citation15], increasing production and inventory costs, while also affecting the degree of inter-brand competition. Consequently, optimizing product offerings to enhance the degree of competition on online marketplaces is becoming increasingly vital for brands. Concurrently, rapid technological progress enables brands to shorten the cycle of product renewal. For example, Zara—a fast fashion brand—renews its product line twice a week.Footnote2 Therefore, if the decision support aids of online marketplaces continue to follow traditional approaches for analyzing the degree of competition of a brand [Citation54], they might misadjust production plans, which can lead to increased operational risks and a reduction in sales [Citation21]. To address the tensions of that phenomenon, we specifically explore:
Research Question: How can brands dynamically balance intra-brand cannibalization and inter-brand competition in product offerings to enhance their degree of competition on online marketplaces?
To answer the research question of our study and ensure the generalizability, and applicability of our findings, we draw upon network theory, and we use product consideration sets of consumers from multiple product categories. The literature to date has mostly used supply-side information to assess the degree of competition between brands, such as the market share [Citation39] or performance [Citation97]. For instance, Li et al. [Citation52] used data from the industry of personal computers and adopted an econometric approach to balance the effects of intra-brand cannibalization and inter-brand competition. Such approaches, however, are not consistent with the contemporary needs of brands on online marketplaces, as the time and economic cost of obtaining information on the supply side are relatively high, and such information is often outdated, not reflecting the real-time degree of competition of brands. Recent work has used the product consideration sets of consumers to identify competitive structures for brands and products from a market demand perspective. For example, Ringel and Skiera [Citation75] used product consideration sets of consumers to explore asymmetric competitive networks and identify distinct submarkets. An asymmetric competition network is defined according to the direction of competition between brands [Citation1, Citation27, Citation58]. For instance, when the competition between brands B1 and B2 is not equal to the competition between brands B2 and B1, then they have an asymmetric competitive relationship [Citation75]. We extend the line of research on product consideration sets of consumers through an asymmetric competitive network perspective, by focusing on the dynamic change of the market segment to which a brand or a product belongs, and specifically addressing how the network position of brands can dynamically affect their degree of competition.
The extant information system (IS) literature to date has used product consideration sets of consumers to 1) model their time-variant clicking behavior from a single- [Citation41] or multiple-website perspective [Citation60], 2) demonstrate the relation between the browsing behavior and purchase intention of consumers [Citation60, Citation78, Citation96], and 3) analyze the market structure and competition [Citation27, Citation32]. This line of research treats product consideration sets of consumers as static [Citation30, Citation52, Citation75]. The temporal dimension of consumers’ behavior, however, can reflect changes in their shopping process and provide a more consistent perspective on brand competition, thereby providing novel insights for optimizing product offerings, enhancing the degree of competition and unearthing factors that influence them over time. We use the product consideration sets of consumers in different periods to measure the temporal dimension of their behavior on online marketplaces. In doing so, we extend the relevant discussions on IS literature [Citation30, Citation52, Citation75] by using consideration sets of consumers over time to dynamically balance intra-brand cannibalization and inter-brand competition, as well as to show the dynamic change of the market segment to which the brand and product belongs.
To explore how the market position of brands can dynamically affect their degree of competition on online marketplaces, we draw upon network theory; specifically, we use the network measures of structural holes and centrality indexes. According to network theory, a structural hole is a nonredundant connection between two nodes [Citation17, Citation18] and describes a specific node that has direct connections with some nodes, but no connection or discontinuity with others. A brand in a structural hole refers to its position between other brands on an online marketplace, indicating that consumers rarely search for it at the same time along with others in a focal market segment; however, they do search for it at the same time along with others in other segments. Consequently, although the focal brand is less threatened by competition in a specific market segment, it can be threatened in others. Prior studies on structural holes have shown that nodes can gain advantages through brokerage opportunities created by the lack of ties among nodes [Citation77, Citation88]. Other studies, however, show that nodes can benefit from a confidential relationship with others, and the degree of such confidentiality is often measured by centrality indexes. The most commonly used centrality indexes are degree centrality and betweenness centrality [Citation34, Citation35, Citation92]. Degree centrality measures the control range of nodes; nodes with a higher degree centrality have a stronger influence. Since degree centrality represents the direct connection with a specific node, it reflects the impact of nodes on the local market [Citation69], which refers to the market of a brand and its direct competitors in which a consumer navigates from one to the other. Betweenness centrality represents the positioning of a node as an intermediary and measures the number of shortest paths passing through a focal node, without necessarily having direct ties [Citation35]. Betweenness centrality measures the impact of nodes on the global market, which refers to the network of direct and indirect competitors. High centrality indexes show that consumers often search for a brand along with other brands, indicating its popularity in the market; degree centrality reflects the local market and betweenness centrality reflects the global one.
We use the span of structural holes, betweenness centrality, and degree centrality as independent variables (IV) on a dataset of 6,549,484 records over a period of 10 weeks from one of the biggest online marketplaces in Asia. We explore the degree of brand competitive dynamics through the time-variant clicking behavior of consumers, using clickstream data [Citation43, Citation87], which can objectively reflect the underlying interests and preferences of consumers on online marketplaces [Citation41, Citation60]. To address the issue of interdependence in the network [Citation73], we used a spatial auto-regressive (SAR) model [Citation91], and we developed a unified framework to assess intra- and inter-brand competition. We further focus on how the position of a brand in a competitive network can dynamically affect its degree of competition by modeling the degree of inter-brand competition as a function of network attributes. Contrary to established theoretical insights as well as current practical intuitions and market practices [Citation85], we demonstrate that brands should minimize heterogeneity in product offerings on online marketplaces to increase sales. We find that intra-brand product relations have a greater impact on sales, and they are the main reason for competition fluctuations. Consequently, our findings bear novel insights into dynamic brand competition and the positioning of brands on online marketplaces.
The rest of our paper is organized as follows. In the second section, we first present the theoretical background of our study on asymmetric competition, implicit consumers’ preferences, consumers’ clicking behavior, networks, and the relationships among them. Second, in this section, we then present the theoretical background of our study on networks, as well as the relationships between networks and asymmetric competition. In the third section, we proceed to present the construction of the dynamic networks of our study, where we use consideration sets of consumers to quantify intra- and inter-brand competition. In the fourth section, we discuss the reduction of the intra-brand cannibalization effect. In the fifth section, we demonstrate how the network position of brands can dynamically affect their degree of competition in the context of online marketplaces. In the sixth section, we discuss the findings and contributions of our study, while in the seventh section, we conclude the paper with the implications of our study for both theory as well as practice and we delineate an agenda for future research on the topic.
Theoretical Background
Clicking Behavior and Implicit Preferences
Online marketplaces enable consumers to simultaneously compare the offerings of multiple brands, leading to more intense competition than in offline settings [Citation98]. In this context, the shopping process can be defined as the period between the initial searches for a product and the final purchase [Citation44]. Consequently, brands within the same product category that co-occur within a shopping process can be perceived as competitors [Citation24, Citation46, Citation65, Citation67, Citation75, Citation98]. Such a shopping process leaves behind trails in the form of clickstream data, which can be an effective resource for studying brand competition along with the interests and implicit preferences of consumers [Citation12, Citation16]. Clickstream data represent electronic records of consumers’ objective activities [Citation16] and reflect their online browsing behavior without the need for introspection. Therefore, consumers’ online browsing behavior can indirectly reflect their implicit preferences. The implicit preferences of consumers refer to those they do not need to introspect [Citation56]; that is, consumers are not aware of their implicit preferences, or cannot accurately express them. The implicit preference of consumers can reveal more accurately their interests [Citation33, Citation56], which can enhance the degree of competition for brands [Citation20, Citation21].
The literature has broadly explored the heterogeneity and degree of competition between pairs of brands [Citation89], with only a few studies focused on optimizing the product offerings of brands in competitive environments [Citation52], such as online marketplaces. This line of research shows that: 1) it is necessary to optimize product offerings in competitive environments [Citation31] such as online marketplaces, 2) there is a consistent inverse U-shaped relationship between the degree of competition and the length of the product line of a brand [Citation38], and 3) the degree of brand competition differs based on the category of products [Citation75]. One of the key challenges in analyzing brand competition on online marketplaces is the increasing number of competing products since traditional methods such as scanner panels are not practically feasible for product categories containing thousands of products [Citation67]. Prior studies have measured the implicit preferences of consumers by surveying the average number of their searches for each product before a purchase [Citation52]. Such data, however, can be limited due to the cognitive abilities of consumers, as it can be difficult to recall what they have searched for in the past during their online shopping sessions. Especially when the number of products searched for is large, the accuracy of surveys gradually decreases [Citation75]. In addition, the collection of survey data can be costly as well as time-consuming and cannot reflect the real-time preferences of consumers.
Data about the online clicking behavior of consumers can reflect their implicit preferences and can be used to construct their consideration sets before completing a purchase [Citation65]. Since consideration sets are the arbiters of competitive relationships [Citation72], they can enable the discovery of competitive market structures [Citation70]. Clickstream data can capture the instantaneous actual behavior of consumers, which is more reliable than survey and panel scan data [Citation68]. Consequently, the implicit preference of consumers that are captured by clickstream data is the most direct, effective, and objective reflection of potential asymmetric competitive relationships amongst brands. Prior studies have used online searching, clicking, browsing, and reviewing behaviors to reflect the implicit preferences of consumers, and to represent the potential competition relations of brands [Citation28, Citation46, Citation51, Citation52, Citation67]. Among them, Ringel and Skiera [Citation75] used data of consumer search from product- and price-comparison websites to analyze asymmetric intra- and inter-brand competition based on such consideration sets and to identify different submarkets. Less explored within this line of research, however, remain the dynamic identification of asymmetric intra- and inter-brand competition, as well as the in-depth analysis of their influencing factors for product positioning on online marketplaces. In further clarifying this lacuna, we present the similarities and differences of our study with the relevant prior ones in the literature on intra- and inter-brand competition networks, in the Online Supplemental Appendix 1. We, therefore, approach intra- and inter-brand competition networks in line with Ringel and Skiera [Citation75], while we further contribute to this line of research through the consideration of the dynamic asymmetric competition in various time-windows, and by further capturing the network-related factors that can influence the degree of asymmetric competition on online marketplaces. Such contributions are timely and methodologically vital for IS research, especially in the context of online marketplaces, as novel insights on these long-standing issues could lead to the reduction of production and inventory costs for brands, which is conducive to enhancing their degree of competition.
Asymmetric Competition Networks
We draw upon the foundations of network theory to explore the competing relations among brands, and to capture the factors influencing their degree of asymmetric competition. Network theory provides a robust way to measure the positional advantages of nodes within a network [Citation73], and has been widely adopted for studying organizations [Citation12, Citation25, Citation47], their strategy [Citation37], their innovation processes [Citation36, Citation40], as well as their operations management [Citation19]. In the context of our study, for the inter-brand network, brands represent the nodes of the network, while the ties indicate that the corresponding brands have been considered by consumers within a shopping process. The weight of the ties indicates the probability of brands being jointly considered by consumers. For the intra-brand networks, the products represent the nodes, while the ties indicate that the corresponding product has been considered by consumers within a shopping process. The weight of the ties indicates the probability of products being jointly considered by consumers.
For the inter-brand competition network, a brand in a structural hole refers to its bridging position between distinct groups of brands on an online marketplace, which indicates that consumers rarely consider a focal brand along with others in this market segment at the same time, but they consider this brand along with others in other market segments. This means that although the focal brand is less threatened by competition in the market segment, it is threatened by competition in other market segments. Thus, opposing to the bridging role of structural holes in organizations for social relationships [Citation17, Citation18], their role in the context of our study is expected to be negative. Consequently, brands residing in many structural holes are rarely considered with others, attracting fewer consumers, and as a result, achieving a lower volume of sales. Centrality is one of the most established network measures [Citation34, Citation35], as it reveals the structural advantages of the position of a node [Citation59]. For the inter-brand competition network, high centrality indicates that consumers consider the specific brand along with others with a high frequency, which means that the specific brand is well-known in the market segment. Thus, the combined use of structural holes and centrality measures can enable us to identify which brands are more competitive and to study the impact of their position in the inter-brand competition network on their degree of competition over time. We, thus, argue that brands residing close to each other in such networks have stronger competing relations, due to a higher number of consumers that concurrently compare the utility of their offerings during the shopping process.
To explore local and global market influence, we use betweenness and degree centrality [Citation69]. Betweenness centrality measures the number of shortest paths passing through a focal node, which do not necessarily have direct ties connected to it [Citation34, Citation35]. In the inter-brand network, such a focal node indicates that the brand is relatively close to the target brand, that is, consumers need to refer to attributes of the focal brand when searching for the target one. Although consumers do not make comparisons with target brands in a certain market segment frequently, they do refer to them in the global market. Betweenness, therefore, delineates the centrality of the entire market of related offerings. We argue, therefore, that a brand with high betweenness centrality is more closely linked to the target brand, suggesting a higher exposure of the brand and, therefore, higher sales volume.
Concurrently, degree centrality represents the number of nodes directly connected to a focal node [Citation69]. In contrast to betweenness centrality, therefore, the degree of a node describes its local centrality within a certain market segment. In the context of our study, therefore, we argue that the greater the value of a focal brand, the more traffic it generates, acquiring, thus, higher sales volume.
Dynamic Network Constructions
To construct the dynamic networks of our study, we first identify the intra- and inter-brand competition relations based on the clickstream data, and then we model the degree of dynamic competition for each brand, as visually depicted in . In doing so, our approach consists of four steps, as visually depicted in : 1) obtain data on consumers’ sequential clicking and purchasing behavior for a certain category of products within a fixed period; 2) aggregate the data for each consumer in a shopping process to form a consideration set; 3) construct the asymmetric competition matrix by combining all the consideration sets of consumers; the matrix represents the probability that a particular brand is considered by consumers; and 4) model the asymmetric intra-brand relationships at the product level as well as the asymmetric inter-brand competition relationships at the brand level.
Figure 1. Research process. Abbreviations: IV, independent variables; CV, control variables; DV, dependent variable.

Data Collection
We obtained data on the online clicking and purchasing behavior of consumers for a period of 10 weeks from one of the biggest integrated online retail marketplaces in Asia, which has more than 300 million consumers and its core categories are electrical appliances, computers, and mobile phones. The longitudinal dataset contained: 1) consumer sequential clicking behavior in the same product category; 2) the log value of each brand’s purchasing data; 3) consumer demographics (see ), and 4) the attributes of clicked or purchased product (i.e., product name, brand name, and product category). With privacy in mind, the dataset was pseudonymized with the use of a coding system.
Table 1. Descriptive statistics of demographic and products.
The dataset consists of 6,554,984 records of 54,269 consumers, 2,688 products, and 86 brands, where 6,982 purchases were made by 6,202 consumers, and covering 529 purchased products from 52 brands. The average number of products for each brand is 31.26 with a maximum of 198. The average number of purchases by a consumer is 1.13, with a maximum of 17. The average number of products purchased by a consumer is 1.08, with a maximum of 15; the average number of brands purchased by a consumer is 1.05, with a maximum of 8 (). To reflect the dynamic variability of asymmetric intra- and inter-brand competition, we first determined a suitable duration to define the time-windows. In doing so, the duration should not be set too short [Citation48, Citation49], otherwise, the selection behavior within a shopping process will be regarded as the next cycle, resulting inaccurate analysis and sparse data, which is not conducive to the analysis of asymmetric intra- and inter-brand competition. The duration should also not be too long either [Citation48, Citation49], otherwise useful information might be disregarded, resulting in the changing process of asymmetric intra- and inter-brand competition not being fully reflected. Since the change cycle is determined by the shopping process of consumers, the average duration between search and brand selection within the same shopping process is approximately 15 days, and the duration that consumers search for purchases is related to the product category [Citation13]. In line with this, we also find that 85 percent of consumers in our dataset had shopping cycles of 14 days (see the Online Supplemental Appendix 2). Considering such behavioral characteristics, we choose 2 weeks as the duration of time-windows, which is also in line with the relevant literature [Citation2, Citation13].
We use a sliding window filter [Citation2, Citation48, Citation66], which is a widely used approach in the analysis of dynamic social networks, [Citation1, Citation2, Citation48]. Sliding window filters are used to divide continuous data by time-windows of a fixed size, which can be either overlapping or non-overlapping. The use of overlapping sliding time-windows can improve the accuracy of analysis, as they are less prone to missing important events [Citation2, Citation48]. Considering the periodicity of consumer behavior, as well as the average purchase cycle of 6.17 days in our dataset, we overlap each consecutive period by one week to reflect the continuous process of changes in brand competition and consequently divide the 10 weeks into 9 windows. For each time-window, we construct consideration sets, while to ensure that brands are competitive, we use only data from consumers in products of the same category [Citation1].
Prior studies often use duration analysis to confirm the relationship between brands based on the purchasing and clicking behavior of consumers [Citation24, Citation98]. We also use these pieces of information from duration analysis to build a network of competitive relationships between brands. For instance, when a consumer clicks on brands B1, B2, B3 and B4 within a time-window, and purchases brand B4, this means that B4 has a competing relationship with B1, B2, and B3. Accordingly, we filter the clicking behavior of consumers with purchase behavior in each time-window. We employ a modular optimization-based heuristic approach to cluster and visualize communities, which outperforms all other known community detection methods in terms of computational time and community quality [Citation9].
In doing so, we constructed the undirected competition network of , which consists of 52 nodes representing the brands, and 916 ties representing competing dyads. We calculated that its density is 0.69, revealing that 69 percent of the brands have a competitive relationship. The average degree of the undirected competition network is 35.23, and the average path length is 1.35, indicating that the brands within a product category are very closely related. These values indicate that the degree of competition for the brands in our study is particularly fierce, compared to prior relevant studies that have used datasets demonstrating that consumers only click on an average of 2.8 brands before purchasing [Citation13]. The attributes of the competition network between brands, therefore, can indirectly explain the strong competitive relationship among the selected brands in our study.
Figure 3. Network graph of competition between any two brands. Note: The nodes represent 52 brands, and their size indicates sales volume. The ties indicate a competitive relationship between brands; the color of the ties represents the market segments. The color version of the figure is provided in the Online Supplemental Appendix 3, .

Constructing Consideration Sets
After identifying the competing brands, we establish consideration sets in different time-windows based on individual consumers (, second step), and then a joint consideration set is constructed for each brand (, third step). The joint consideration set is a combination of all consumers in units of brands, and the cell of the joint consideration set is the number of consumers who consider those brands at the same time-window. Specifically, in the matrix, the cell Njkt represents at time-window t, the number of consumers who consider the brands j and k together, and its mathematical expression is shown in the following Eq. (1):
where I represents the set of consumers who click on the brands j and k together in time-window t, and I represents one particular consumer. If the consideration set of consumer i includes brand j in the time-window t, then we have Lijt = 1; otherwise Lijt = 0. Similarly, if the brand k is included in the brand consideration set of the consumer i in time-window t, then we have Likt = 1; otherwise Lik t= 0.
Establishing Asymmetric Binary Matrix
The degree of competition between any two brands is asymmetric [Citation75], but this cannot be reflected in Eq. (1). To illustrate the construction of an asymmetric relations matrix, we take as an example the behavior of the three imaginary consumers presented in . Let us assume that during a shopping process, consumer i1 clicks consecutively on brands B1 and B2, consumer i2 clicks on B1, B2 and B3, and consumer i3 clicks on B1, B2 and B3. We present these relations in , and 4. According to the values in the 4th and 6th columns of , there is a difference between the consideration sizes. To reflect this difference, we use Eq. (2) to transform a symmetric matrix into an asymmetric one:
Table 2. Constructing Individual Consideration Sets.
where N*jkt is the degree of relations between brands j and k in time-window t and the other parameters are as in Eq. (1).
Table 3. Constructing joint symmetric consideration sets.
Visualizing Asymmetric Competition
We use Louvain community detection [Citation33] with resolution parameters to cluster the competition relations among brands and products, and we use the in-degree centrality to indicate the degree of competition in the first time-window. While it is possible to form nine such network graphs, one for each time-window of our study, for brevity we present the first time-window in . Each node in represents a brand, and its size indicates sales volume. Colors indicate market segment, while ties indicate that brands are in a consumer’s consideration set within a time-window. We use two-way ties to indicate asymmetric competition: the degree of competition for B1 to B2 is indicated by the edge weight of B2 to B1. Conversely, the degree of competition for B2 to B1 is indicated by the edge weight of B1 to B2, the weight of ties indicates the degree of competition. In different time windows, the number of any two brands considered by consumers at the same time is inconsistent. When any two brands are not considered by any consumers, they are not competitive and do not appear connected.
Figure 4. Network graph of asymmetric competition between brands in the first time-window. Note: Each node represents a brand, and their size indicates sales volume. The ties indicate a competitive relationship between brands; the color of the ties represents the market segments. The color version of the figure is provided in the Online Supplemental Appendix 3, .

Intra-Brand Cannibalization Analysis
By observing the asymmetric competition network in the nine time-windows, we find that the degree of competition fluctuates, which can pose risks to the operations and market positioning of brands. Therefore, it is necessary to conduct a further in-depth analysis of the underlying reasons for such fluctuations. To further explore such root causes, we take the products of brands as a unit of analysis and construct asymmetric competition networks amongst them, similar to the process for the asymmetric competition networks amongst brands, as we describe in the following section.
Dynamic Competition Process among Brands
To better portray the dynamics of competition among brands, we first identify the top 18 brands that account for 90 percent of the overall market share (see the Online Supplemental Appendix 4), and then calculate their degree of competition over time, as we demonstrate in . We use in-degree centrality in the inter-brand network to indicate the degree of competition. Although the degree of competition fluctuates within time-windows, the fluctuation ranges and can be divided into two phases; the fluctuation range of most brands is within five points of scale and a small number of brands have larger fluctuations (see details within the dashed box in ). Taking the brand with code ‘165’ as an example, in we can observe that in the third time-window its degree of competition is near 40, while in the fifth time-window its degree of competition is below 5. When the degree of competition fluctuates greatly, it can cause risks to the operations of a brand, which brings challenges to its production and market positioning. There is merit, thus, in further exploring the reasons behind such fluctuations over time.
Reasons for Dynamic Competition
In the context of online marketplaces, 10 percent of products account for 90 percent of the market share of a brand [Citation90, Citation98], similar to the Pareto principle [Citation14, Citation86]. We, thus, select the products with the top 90 percent sales volume for each brand and analyze the intra- and inter-brand competition in different time-windows.
The results are shown in , which consists of five directed network graphs that represent intra- and inter-brand competition in different time-windows. Each node represents a product, the numbers in the first graph represent the product code, and the products represented by the nodes in the subsequent graphs are the same as the first one. Nodes of the same color belong to the same brand, as shown in . Bilateral ties show the existence of intra- and inter-brand competition, while unilateral ties represent that one product poses a competitive threat to the others, but the reverse is not true. The weight of ties represents the degree of intra- and inter-brand competition. The number of ties between products within a brand and their weight is significantly higher than that between brands. This shows that intra-brand competition is much higher than inter-brand one and that consumers tend to consider products within the same brand rather than across different brands. By examining the competition network in different time windows, we can see that the ties and their thickness change over time, which demonstrates that the degree of intra- and inter-brand competition changes dynamically.
Figure 6. Intra- and Inter-brand competition in different time-windows. The color version of the figure is provided in the Online Supplemental Appendix 6, Figure 6.

To verify the aforementioned inference, we divided the 52 brands into three categories based on their sales volume (high, medium, low) and randomly selected one brand from each category. The intra-brand relations among products are divided into nine asymmetric networks. We calculate the indicators of each product and analyze the relationship between the indicators and the sales volume with a mixed regression. We find that 83.9 percent of sales fluctuations are contributed by intra-brand competition (Online Supplemental Appendix 7, ). The main reason for intra-brand competition is the high substitutability of products. Thus, optimizing product offerings is conducive to enhancing the degree of competition between brands. We can, thus, conclude that the main reason for fluctuations in the degree of brand competition is the interaction among products within the brand. The analysis of six brands with larger fluctuations of competitive relation shows that intra-brand competition is more intense than intra-brand one, which is in line with the extant literature on the topic [Citation52, Citation75]. We can, thus, infer that internal production-line adjustments are needed to optimize offerings within the brand, and we can calculate in advance how the market share of related products will change after such an adjustment.
Reduce Intra-Brand Cannibalization
Product proliferation can result in an increase in production cost, and consequently a decrease in profit margins. Brands, therefore, need to decide whether to adjust the original production line and estimate the loss/gain of market share when doing so. In the context of online marketplaces, the sales distribution of brands is considered fat-tailed [Citation11, Citation57]. Based on the asymmetric networks, we can model sales after adjusting the production line. Subsequently, we randomly select the brand with code ‘235’ as an example to illustrate the market-share redistribution when its production line is adjusted.
As we depict in , before production line adjustment, the market share of this brand in the first time-window was 29.09 percent, of which the market share of the top (i.e., best-seller) six products was 23.77 percent, and of the bottom (i.e., least sold) ten products was 5.32 percent. To further illustrate, let us assume the possibility of stopping producing the products with sales volume ranking of the bottom ten products for the brand with code ‘235’, and also assume that in the consumers’ consideration set each product has an equal probability of being considered. If consumer A considers four products at the same time-window, the probability of each product being considered is 25 percent, while if the number of products in the market decreases by one, the possibility of consumers considering other products increases. We use the consumers’ consideration set, by considering the idea that the probability of each product being considered is equal and we calculate the adjustment of market share after the brand reduces the production of the bottom ten products for the brand with code ‘235’. According to the result, we find that the adjusted market share does not decrease by 5.32 percent, but only by 3.08 percent. To the extreme, assuming that the production cost of each product is equal, producing six products only requires 37.5 percent of the overall production cost for 16 products, and hence removing the bottom ten products can save 62.5 percent of the original production costs. Thus, the inter- and intra-brand asymmetric competition network can also provide a strong reference for the production line adjustment.
Table 4. Constructing joint asymmetric consideration sets.
Table 5. Market-share distribution with product-line adjustment.
Inter-Brand Competition Analysis
The degree of brand competition is a manifestation of market power, and it can be measured through the sales volume [Citation79]. To capture competition dynamics between brands, we use the frequency of consumers’ conditional considerations to measure the degree of competition [Citation75]; the process is shown in . According to network theory [Citation5, Citation73, Citation76], different structural characteristics represent different consumers’ consideration sets, indicating that preference for a brand varies, having the potential to model sales. In line with this, therefore, we use the span of structural holes, betweenness centrality, and degree centrality as IV to model sales. To solve the auto-correlation problem, we use SAR models for parameter estimation [Citation95].
Variable Selection
We consider the frequency of consumers’ conditional considerations as directly proportional to their actual purchase [Citation64] and use brand sales to measure the degree of competition. Due to the significant difference in sales volume of brands, we take the log of sales logyjt as DV, where j indicates a brand and t the time-window. We use structural constraint indicators [Citation23, Citation93], and for each time-window the indicators are estimated by Eq. (3) and Eq. (4):
where Cjt represents the network constraint of brand j in the time t, where the larger the value, the greater the constraint and the fewer structural holes a brand would have. Since structural constraint is negatively related to the degree of structure holes, we use to represent structural holes index; K represents a brand directly adjacent to j; Cjkt indicates the degree of constraint of brand k directly adjacent to brand j at time t; q represents a common adjacent point for brand j; and k. pjkt represents the proportion of brand j in the neighboring nodes of brand k at time t.
indicates the indirect proportion of brand k on brand j. Considering that the network is asymmetric, pjkt is not equal to pkjt. Betweenness centrality is calculated according to Eq. (5) [Citation34]:
where gklt is the number of shortest paths between nodes k and l in time t, and gklt(j) represents the number of shortest paths through node j. Therefore, Eq. (5) shows how often node j is located on the shortest path between nodes k and l in time t. The equation for degree centrality is [Citation34, Citation35]:
where j is a focal node, k is a node other than j, N is the number of all nodes, x is the adjacency matrix, and the cell xjkt indicates the direct adjacency between nodes j and k in time t. When the value is 1, there is adjacency between nodes; otherwise, the value is 0. Degree and betweenness centrality measure the importance of a structural position of a node in a network; specifically, degree centrality measures the local influence of a node, while betweenness centrality measures the global impact of a node [Citation92]. In we summarize the descriptive statistics of the network indicators in our study.
Table 6. Descriptive statistics of variables.
Abbreviations: SD, standard deviation; DV, dependent variable; IV, independent variable; CV, control variable.
Control Variables
Since the intra-brand asymmetric competition network is constructed by consideration sets on the brand level, the demographics of consumers are considered to have an impact on sales [Citation55]. We, thus, control for the effects of gender, age, and membership level of consumers in our models. As females tend to have a higher purchase intention and a more positive attitude toward brands [Citation81], we control for gender on sales using the percentage of female in the dataset. Age is also a key factor of individual motivation [Citation29], as consumers of different ages have different purchasing motives, and options.
The consumers that completed a purchase in our dataset are between 26 and 45 years old. Therefore, we use the median value of 36 years and the percentage of consumers above 36 years of age to analyze the impact of age on the volume of sales. The membership level can explain the purchase and consumption experience of consumers, as the higher the membership level, the richer their shopping experience. Consumers with different experiences have different clicking behaviors and purchase possibilities. Thus, the membership level can impact brands sales. There are four levels of memberships in our dataset, according to the descriptive statistics, most of the purchasers are above level 4. Thus, we use the percentage of consumers on level 4 in our dataset to measure the effect of membership level. The correlations for all variables of interest are presented in Online Supplemental Appendix 8 ().
Estimation and Results
An extreme strategy in parameter estimation is to treat panel data as cross-sectional—since there is no individual effect—and perform pooled regression. We use a pooled regression model as baseline and a population-averaged (PA) estimator for the parameters. To avoid potential endogeneity issues, we lag the IV by one period [Citation7, Citation10, Citation74]. Considering the effects of IV and CV on the log of sales volume, our model is shown in EquationEq. (7(7)
(7) ), in which uj is the influence of the brand, and εjt is the error term:
The estimation results of the baseline model are shown in (models 1 and 2). To test multicollinearity among IV and CV, we performed a variance inflation factor (VIF) test [Citation80]. We present the results in the Online Supplemental Appendix 9, where the maximum value of VIF for all variable is 2.01, far less than its threshold of 10 [Citation80], indicating that multicollinearity among IV and CV is not an issue. However, the basic assumption of using ordinary least-squares (OLS) model for parameter estimation is that there is no auto-correlation among DV. Since the ties in the inter-brand network are derived from a clicking behavior in which consumers can be the same or overlapping, an auto-correlation between the individuals of a brand in different time-windows is possible. We use the global spatial auto-correlation Moran detection [Citation53] to test for auto-correlation among DV. According to the results in , the Moran index among the DV is -0.096, and the p value shows that the exponential relationship is significant, indicating a strict auto-correlation between DV. The negative value of the Moran index indicates a negative correlation in the sales of each brand, which is consistent with their competitive relationship.
Table 7. Result of global spatial auto-correlation test.
Table 8. Estimation results for OLS and SAR (DV = log sales).
SAR models come from spatial econometrics and have recently been widely adopted in neighboring management disciplines, mainly due to the increasing attention paid to the interaction between economic factors, such as peer effect, neighborhood effect, and spillover effect, as well as the rapid development of geographic IS, and the wide availability of spatial data. Whilst SAR was originally introduced for spatial data [Citation6], it has been increasingly gaining popularity in all variances of network analysis [Citation22, Citation26, Citation56], as well as for modeling interdependent consumer preferences [Citation94], since network data share many similarities with spatial data. For our study, the main reason for the existence of auto-correlation between the sales of each brand is that the ties in the inter-brand competition network are interdependent [Citation73]. Any two different brands in our dataset are related to each other through a series of shared consumers. For each brand in the same product category, since the total number of consumers is fixed, the overall market demand remains unchanged, and there is a negative correlation between the sales of each brand. Since there are three types of SAR models, we detail our selection in the Online Supplemental Appendix 10, considering DV as shown in Eq. (8):
where the parameters are consistent with the previous statements; Wjk is the 52 x 52-dimensional spatial weight matrix, in which cell values are the mean of all the brand asymmetric matrices; Ykt indicates the sales volume of other brands at the same time-window; Xjt-1 is the IV and CV of brand j lagging one period; and Δt is the time effect, referring to the nine time-windows. The estimations of Model 3 and 4 in are the corresponding results of the SAR model, and the influences of the three network measures on sales are significant. In addition, among the CV, only gender has a significant impact on sales. Meanwhile, according to the value of R square, we can conclude that the SAR model has a better fitting effect on the actual sales (0.687 > 0.539) compared to the baseline model.
Abbreviations: OLS, ordinary least-squares; CV, control variable, IV, independent variable; SAR, spatial auto-regressive; AIC, Akaike Information Criterion.
Robustness Check
Different product categories have different attributes, and the behavior of consumers varies per category [Citation50]. To test the robustness of our results, we use the clicking and purchasing behavior in different product categories. In , we compare the structures of the original and the test dataset. Compared to the original product category data, the product category selected in the test-set differs in three ways: 1) the number of purchased brands (in the test-set is about half of the original); 2) the number of consumers with click and purchase records (it is double, while the number of clicks is roughly equal); and 3) the purchase amount of the test-set (it is nearly double that of the original).
Table 9. Comparison of the original dataset and the test dataset.
The comparative analysis shows that the number of clicks on each product in the original dataset is almost twice that of the test-set, which indicates that brand’s attributes of the two types are considerably different, and there is a large difference in the identification process of consumers before the purchase. Concurrently, in the test-set, the average purchase amount of each consumer is 1.3 times that of the original dataset, indicating that products in the test-set are more aligned with the characteristics of daily consumables than the products in the original dataset. The comparison of the two data structures shows that the two products categories have greater heterogeneity. Heterogeneity refers to the degree of variance in the attributes of the focal network [Citation61] and captures the degree of differences in consumers’ browsing records and repurchases, as well as their consumption frequency.
Since the product characteristics of the two datasets are different, to reduce the dimensionality impact we standardized dataset indexes before comparing the product network. If the test-set results are consistent with the original, our findings can be considered robust. Thus, we re-analyze the test dataset according to the aforementioned process, and the results (Online Supplemental Appendix 11, ) show that the three network measures affect the brand sales in the same way as the original dataset. Specifically, the results of all IV are significant. Structural holes have a negative impact on brand sales, while degree centrality and betweenness centrality have positive impacts on brand sales, thus successfully demonstrating the robustness of our findings. Furthermore, prior research shows that consumers’ consideration sets are influenced by weekends and weekdays [Citation8]. Thus, to further verify the robustness of our findings, we divide consumers’ weekly consideration sets in the test-set into two-time windows: weekend and weekdays. The transformation process between consumers’ consideration sets and brand dynamic competition network in different time windows, and the analysis of the influencing factors of brand dynamic degree of competition are consistent with the analysis of the original data. The results (Online Supplemental Appendix 11, -11), show that structural holes have a negative impact on sales, in-degree centrality has a positive impact on sales, and betweenness centrality has no significant effect on sales. These results further verify the robustness of our findings.
Endogeneity Test
While the lagging of variables by one period can effectively alleviate the endogeneity issues of mutual causality, we still account for the two-way relation between sales of different brands within a time-window. We use the lagging log sales volume of competing brands within a time-window as an instrumental variable because the previous sales of competing brands affect their current sales, and the sales of competing brands at the same time-window affect each other. To further analyze the influence of the network measures on the volume of sales, we use a 2-stage least square (2SLS) regression to address the endogeneity issues in line with Perera and Tan [Citation71]. We follow the same approach for parameter analysis, showing the results of the endogeneity test in . Subsequently, we carried out a Sargan’s Test, showing that the instrumental variable is not used excessively (p = .94). The influence of network measures on sales is still consistent with the SAR model and, therefore, suggests that endogeneity is not an issue in our analysis.
Table 10. Endogeneity test (DV = log sales).
Abbreviations: 2SLS, 2-stage least square; SAR, spatial auto-regressive; CV, control variable; IV, independent variable; AIC, Akaike Information Criterion.
Discussion
Key Findings
Research on brand competition in the context of online marketplaces from the perspective of product offerings has timely reference value [Citation30, Citation52, Citation75]. Prior studies have identified the market segments and static asymmetric competition relations of brands by using consumers’ consideration sets. Brands on online marketplaces, however, compete for consumers’ attention in real-time, and consequently, being able to identify asymmetric competition in such a context is more challenging than in traditional ones. As the preferences and attention span of consumers constantly change, capturing their clickstream data and dynamically modeling the degree of competition can effectively reduce the potential risks for brands of losing market share. We propose a novel approach to identify brand product cannibalization and market competition relations through consumers’ consideration sets and use the volume of sales to verify this. Subsequently, we first analyze how brands should adjust their production lines to reduce intra-brand cannibalization. We further explore the factors that affect future sales of brands from the perspective of inter-brand competition. We use the span of structural holes, betweenness centrality, and degree centrality to analyze the volume of sales. Finally, regarding future sales, we handle the interdependence among brands, using SAR models for parameter estimation.
In doing so, our study brings forward three key findings. First, we find that intra-brand competition has a greater impact on the degree of dynamic competition than inter-brand. Considering the importance of intra- and inter-brand competition in optimizing product offerings, our findings underscore the need for brands to balance these two effects when offering products on online marketplaces. If the impact of intra-brand competition on the degree of dynamic competition is more pronounced, brands on online marketplaces are better off when reducing the number of their offerings, which addresses the research question of our study. This finding may be counterintuitive, as in practice online marketplaces are not limited by capacity or physical shelf space. In addition, our work can also predict in advance how the market share will change after product line adjustment.
Second, our findings show that products residing in structural holes serve as an outbound agent and hence create negative effects on sales volume, which broadly contradicts the insights from the extant management literature on the positive effects of the span of structural holes on organizational performance. When a brand is in a structural hole, this indicates that fewer consumers consider it at the same time along with other brands. For such brands, however, the degree of competition in a product category is relatively weak. Therefore, our findings demonstrate that in the context of online marketplaces, brands should minimize heterogeneity in their product offerings within their market segment to increase sales, which also contradicts intuitive current practices for pursuing individualization in product offerings to become more competitive [Citation28].
Third, our findings show the positive impact of degree centrality and betweenness centrality on the volume of brand sales on online marketplaces, which validates the two initial assumptions of our study. We find that the influence of degree centrality is higher than betweenness centrality, which means that intra-brand cannibalization is more intense than inter-brand competition. Thus, when brands consider their product design and marketing strategies for online marketplaces, they should give a higher reference weight to the characteristics of each brand in their market segments.
Theoretical Implications
Our work brings forward four key theoretical contributions. First, we consider an asymmetric competition relationship amongst brands, which is more consistent with contemporary reality, and provides better theoretical foundations for competition on online marketplaces. In addition, we balance intra-brand cannibalization and inter-brand competition to reduce the market risk of product offerings in this context. Second, we use consumers’ time-variant implicit preferences to unearth the dynamics of intra- and inter-brand asymmetric competition and provide a theoretical reference on when and how brands should optimize their offerings on online marketplaces to increase their sales volume. In addition, we integrate demand information of consumers’ implicit preferences with supply-side sales insights to increase the robustness of our findings. By doing so, we extend the literature on the use of consumers’ time-variant implicit preference information from the consumer level [Citation41, Citation60] to the brand level. Online marketplaces, due to their high product aggregation and fast updates on offerings, can result in constantly changing consumers’ preferences and attention spans. Capturing the dynamics of intra- and inter-brand asymmetric competition can provide a sorely needed theoretical reference on when and how brands should optimize their offerings on online marketplaces, thereby reducing the market risk. Third, we combine the span of structural holes with centrality measures to model the sales of brands on online marketplaces. In doing so, we evaluate the effects of structural holes and centrality measures and distinguish their impact on sales from the local and global markets. In addition, we expound the attributes of the brand competition network from the perspective of consumers’ consideration sets, expanding the applications of network theory to capture brand competition. Finally, we use consumers’ behavioral information reflecting their implicit preferences to consider the dynamics of asymmetric competition. In doing so, we explore the competition between brands and demonstrate the complex dynamics of product cannibalization. Our work, thus, shifts the discussions on asymmetric competition from a static to a dynamic paradigm. Consequently, the findings of our study provide a novel theoretical standpoint for brands on online marketplaces to predict sales volume based on their position in the global and local markets, which ultimately can provide information reference for product design as well as marketing strategies in this context.
Practical Implications
Our work also provides valuable insights for practice. Our findings demonstrate that in the context of online marketplaces the degree of inter-brand product cannibalization is higher than the degree of external competition among brands. Therefore, brands operating in this context need to adjust the length of the production line to optimize their offerings and increase their sales. Our findings, thus, suggest that to enhance the degree of competition on online marketplaces, brands must adjust their product line in ways fundamentally different from the contemporary industry practices. To this end, our work can provide brands with insights for predicting the redistribution of market shares, identify market risks, improve decision-making, and ultimately increase their sales. We show that to increase sales, brands should increase the number of ties with others in their asymmetric competition network and minimize heterogeneity in product offerings within their market segment. In practical terms, this finding means that brands should identify products with similar attributes to those with high sales in their target market segment when offering products on online marketplaces. Consumables and newly entered products usually take sales volume as the main goal, therefore, brands should consider the homogeneity of related products in the market segment, since most of online consumers have a lower level of perceived differences in such products [Citation75]. Our work, therefore, provides brands that operate on online marketplaces with novel insights that contradict contemporary practices. Our practical insights, thus, provide a bedrock for novel business models and enable brands to increase their sales.
Limitations and Future Research
Whilst we followed a thorough and structured research design, there are limitations that we need to acknowledge, which concurrently open future research avenues. The clicking behavior of consumers includes actions such as browsing, clicking, adding, or removing goods to the shopping cart, as well as purchasing. While such actions might stand for the preferences of consumers toward a brand, they share a uniform weight in our estimations. Future research, therefore, could assign different weights to the various actions of consumers, and reflect on the strength of sales. Moreover, in our study, we only consider the spatial auto-correlation problem when using network measures to reflect the future sales of brands. Due to the cumulative effect of brand sales, however, there is also a possible temporal correlation problem, and we encourage future research endeavors to reflect on the possibility of such an issue. Third, we only consider the volume of sales as a measure of brand competition, but we do not consider the impact of profit on the degree of competition. Our conclusions, thus, may be different from other profit-oriented studies, and future research endeavors could use brand sales along with profit for comparative analysis according to the strategic goals of brands. Additionally, when analyzing the dataset of our study, we considered the sequence of consumers’ behaviors in different time-windows, but we did not consider the sequence of their clicking behaviors within the same time-window, as this was beyond the scope of our paper. In addition, all our conclusions are based on the analysis of empirical data, and no additional experiments have been conducted. We encourage future research endeavors, therefore, to consider the sequence of consumers’ consideration sets within the same time-window when exploring the competition amongst brands, as well as to consider conducting field experiments. Finally, our work highlights the importance of access to information for extracting actionable insights, which makes paramount the quality of such information [Citation82, Citation84], especially for datasets of high volume, variety, and veracity [Citation3, Citation4, Citation45, Citation63]. Future research endeavors on the topic, thus, should further contribute to the extant IS research agenda [Citation83] by focusing on the challenges surrounding information quality in the use of large datasets for extracting actionable insights.
Conclusions
By examining market dynamics and the influence of network positions, we shed light on how brands can balance intra-brand cannibalization and inter-brand competition in their product offerings, ultimately enhancing their degree of competition on online marketplaces. The findings of our study offer a theoretical reference for brands seeking to optimize their offerings on online marketplaces. Specifically, if the impact of intra-brand competition on the degree of dynamic competition is more pronounced, brands would benefit from reducing the number of their offerings on online marketplaces. We further suggest that brands should strive to minimize heterogeneity in their product offerings within their market segment to increase sales. Moreover, brands should assign greater weight to the unique characteristics of each brand within their market segment. By considering these factors, brands can better position themselves to thrive in highly competitive online marketplaces.
Supplemental Material
Download MS Word (3.4 MB)Acknowledgments
The authors would like to kindly thank the editor and the two anonymous reviewers for their valuable comments and developmental approach throughout the review process.
Disclosure statement
No potential conflict of interest was reported by the authors.
Supplementary Information
Supplemental data for this article can be accessed online at https://doi.org/10.1080/07421222.2023.2267314.
Correction Statement
This article has been corrected with minor changes. These changes do not impact the academic content of the article.
Additional information
Funding
Notes on contributors
Meihua Zuo
Meihua Zuo ([email protected]) is a lecturer at Huizhou University, China. She received her Ph.D. from the Guangdong University of Technology, China. Dr. Zuo’s research interests include consumer behavior analysis, business intelligence, social network, and brand competition of data mining. Her work has been published in such journals as Information Systems Frontiers, Industrial Management & Data Systems, and Journal of Control & Decision, and presented at International Conference on Information Systems, Academy of Management, and the International Conference on Electronic Commerce.
Spyros Angelopoulos
Spyros Angelopoulos ([email protected]) is an Associate Professor at Durham University Business School, United Kingdom. Previously, he held academic appointments at Tilburg University, the University of Lugano, and the University of Nottingham. He holds a Ph.D. from Warwick Business School and has a background in engineering and management. Dr. Angelopoulos’s research focuses on user behavior on digital platforms, organizational adaptation during digital transformation, and on security and privacy issues of users on digital platforms. He has been Guest Editor of Special Issues of Journal of Operations Management, Journal of the Association for Information Systems, and International Journal of Information Management. He was selected as Faculty Expert by Google for his commitment to leading transformation in information systems research and education.
Carol Xiaojuan Ou
Carol Xiaojuan Ou ([email protected]) is a Professor of Digital Transformation and Information Management and Head at the Management Department in Tilburg University, the Netherlands. Dr. Ou’s research interests include digital transformation, applied business intelligence, computer-mediated communication, social commerce, smart recommendation agents and knowledge management. Her publications have appeared in such journals as Communications of the ACM, Decision Support Systems, Information & Management, Information Technology & People, Journal of AIS, and MIS Quarterly, among others. She serves as a senior editor of several IS journals and has chaired tracks in major Information-Systems conferences. She is also a Certified IS Auditor and an Academic Advocate of IS Audit and Control Association. She has led or participated in project grants of over €5 million. Dr. Ou is a distinguished member of the Association for Information Systems.
Hongwei Liu
Hongwei Liu ([email protected]) is a Professor of Information Management at the Department of Management Science and Engineering, Guangdong University of Technology, China. His research interests include business intelligence, systems design, and privacy issues of data mining. Dr. Liu’s publications have appeared in journals such as Information & Management, Information Systems, Advances in Modeling & Analysis, and Impulsive Dynamical Systems and Applications, among others. He was Dean at the School of Management and in charge of academic research. He also heads several projects of the Natural Science Foundation of China.
Zhouyang Liang
Zhouyang Liang ([email protected]) is an experimenter at the Guangdong University of Technology, China, where he also received his Ph.D.. His research interests include consumer behavior analysis, electronic commerce, Bayesian inference, and recommendation system. His work has been published in such journals as Journal of Control and Decision and presented at such conferences as International Conference on Information Systems, Annual Meeting of the Academy of Management, and International Conference on Electronic Commerce. Dr. Liang has participated in several projects of the Natural Science Foundation of China.
Notes
References
- Andrade-Rojas, M.G.; Kathuria, A.; and Konsynski, B.R. Competitive brokerage: How information management capability and collaboration networks act as substitutes. Journal of Management Information Systems, 38, 3 (2021), 667–703.
- Angelopoulos, S.; and Merali, Y. Sometimes a cigar is not just a cigar: Unfolding the transcendence of boundaries across the digital and physical. ICIS, Seoul, Korea: Association for Information Systems, 2017.
- Angelopoulos, S.; Brown, M.; McAuley, D.; Merali, Y.; Mortier, R.; and Price, D. Stewardship of personal data on social networking sites. International Journal of Information Management, 56 (2021), 102208.
- Angelopoulos, S.; Bendoly, E.; Fransoo, J.C.; Hoberg, K.; Ou, C.X., and Tenhiälä, A. Digital transformation in operations management: Fundamental change through agency reversal. Journal of Operations Management, Forthcoming, 69, 6 (2023), 876–889.
- Angelopoulos, S.; Canhilal, K.S., and Hawkins, M.A. From groups to communities: A resource mobilization theory perspective on the emergence of communities. Information Systems Frontiers (2023), 1–18.
- Banerjee, S.; Carlin, B.P.; and Gelfand, A.E. Hierarchical Modeling and Analysis for Spatial Data. Boca Raton, FL: CRC Press, 2014.
- Bellemare, M.F.; Masaki, T.; and Pepinsky, T.B. Lagged explanatory variables and the estimation of causal effect. The Journal of Politics, 79, 3 (2017), 949–963.
- Bhatnagar, A.; Sen, A.; and Sinha, A.P. Providing a window of opportunity for converting eStore visitors. Information Systems Research, 28, 1 (2017), 22–32.
- Blondel, V.D.; Guillaume, J.-L.; Lambiotte, R.; and Lefebvre, E. Fast unfolding of communities in large networks. Journal of Statistical Mechanics: Theory and Experiment, 2008, 10 (2008), P10008.
- Blundell, R.; and Bond, S. Initial conditions and moment restrictions in dynamic panel data models. Journal of Econometrics, 87, 1 (1998), 115–143.
- Bordley, R. Determining the appropriate depth and breadth of a firm’s product portfolio. Journal of Marketing Research, 40, 1 (2003), 39–53.
- Brands, R.A.; Menges, J.I.; and Kilduff, M. The leader-in-social-network schema: Perceptions of network structure affect gendered attributions of charisma. Organization Science, 26, 4 (2015), 1210–1225.
- Bronnenberg, B.J.; Kim, J.B.; and Mela, C.F. Zooming in on choice: How do consumers search for cameras online? Marketing Science, 35, 5 (2016), 693–712.
- Brynjolfsson, E.; Hu, Y.; and Smith, M.D. Research commentary—long tails vs. superstars: The effect of information technology on product variety and sales concentration patterns. Information Systems Research, 21, 4 (2010), 736–747.
- Brynjolfsson, E.; Hu, Y.; and Simester, D. Goodbye pareto principle, hello long tail: The effect of search costs on the concentration of product sales. Management Science, 57, 8 (2011), 1373–1386.
- Bucklin, R.E.; and Sismeiro, C. A model of web site browsing behavior estimated on clickstream data. Journal of Marketing Research, 40, 3 (2003), 249–267.
- Burt, R.S. The social structure of competition. Networks in the Knowledge Economy, 13, 2 (2003), 57–91.
- Burt, R.S. Structural holes and good ideas. American Journal of Sociology, 110, 2 (2004), 349–399.
- Chae, S.; Yan, T.; and Yang, Y. Supplier innovation value from a buyer–supplier structural equivalence view: Evidence from the PACE awards in the automotive industry. Journal of Operations Management, 66, 7–8 (2020), 820–838.
- Chen, M.-J. Competitor analysis and interfirm rivalry: Toward a theoretical integration. Academy of Management Review, 21, 1 (1996), 100–134.
- Chen, M.-J.; and Miller, D. Competitive dynamics: Themes, trends, and a prospective research platform. Academy of Management Annals, 6, 1 (2012), 135–210.
- Chen, X.; Chen, Y.; and Xiao, P. The impact of sampling and network topology on the estimation of social intercorrelations. Journal of Marketing Research, 50, 1 (2013), 95–110.
- Chi, L.; Ravichandran, T.; and Andrevski, G. Information technology, network structure, and competitive action. Information Systems Research, 21, 3 (2010), 543–570.
- Chintagunta, P.K.; and Haldar, S. Investigating purchase timing behavior in two related product categories. Journal of Marketing Research, 35, 1 (1998), 43–53.
- Clement, J.; and Puranam, P. Searching for structure: Formal organization design as a guide to network evolution. Management Science, 64, 8 (2018), 3879–3895.
- Cohen‐Cole, E.; Liu, X.; and Zenou, Y. Multivariate choices and identification of social interactions. Journal of Applied Econometrics, 33, 2 (2018), 165–178.
- DeSarbo, W.S.; and Grewal, R. An alternative efficient representation of demand‐based competitive asymmetry. Strategic Management Journal, 28, 7 (2007), 755–766.
- Dickson, P.R.; and Ginter, J.L. Market segmentation, product differentiation, and marketing strategy. Journal of Marketing, 51, 2 (1987), 1–10.
- Diehl, M.; and Hay, E.L. Self-concept differentiation and self-concept clarity across adulthood: Associations with age and psychological well-being. The International Journal of Aging and Human Development, 73, 2 (2011), 125–152.
- Dou, W.; Lim, K.H., Su, C.; Zhou, N., and Cui, N. Brand positioning strategy using search engine marketing. MIS Quarterly, 34, 2 (2010), 261–279.
- Draganska, M.; and Jain, D.C. Product‐line length as a competitive tool. Journal of Economics & Management Strategy, 14, 1 (2005), 1–28.
- Duan, W.; and Zhang, J. The comparative performance of online referral channels in e-commerce. Journal of Management Information Systems, 38, 3 (2021), 828–854.
- Fortunato, S.; and Barthelemy, M. Resolution limit in community detection. Proceedings of the National Academy of Sciences, 104, 1 (2007), 36–41.
- Freeman, L.C. Centrality in social networks conceptual clarification. Social Networks, 1, 3 (1978), 215–239.
- Freeman, L.C.; Roeder, D.; and Mulholland, R.R. Centrality in social networks: II. Experimental results. Social Networks, 2, 2 (1979), 119–141.
- Funk, R.J.; and Owen-Smith, J. A dynamic network measure of technological change. Management Science, 63, 3 (2017), 791–817.
- Gelper, S.; van der Lans, R.; and van Bruggen, G. Competition for attention in online social networks: Implications for seeding strategies. Management Science, 67, 2 (2021), 1026–1047.
- Giachetti, C.; and Dagnino, G.B. Detecting the relationship between competitive intensity and firm product line length: Evidence from the worldwide mobile phone industry. Strategic Management Journal, 35, 9 (2014), 1398–1409.
- Gnyawali, D.R.; Fan, W.; and Penner, J. Competitive actions and dynamics in the digital age: An empirical investigation of social networking firms. Information Systems Research, 21, 3 (2010), 594–613.
- Gómez‐Solórzano, M.; Tortoriello, M.; and Soda, G. Instrumental and affective ties within the laboratory: The impact of informal cliques on innovative productivity. Strategic Management Journal, 40, 10 (2019), 1593–1609.
- Huang, T.; and Van Mieghem, J.A. Clickstream data and inventory management: Model and empirical analysis. Production and Operations Management, 23, 3 (2014), 333–347.
- Hui, K.-L. Product variety under brand influence: An empirical investigation of personal computer demand. Management Science, 50, 5 (2004), 686–700.
- Jabr, W.; Ghoshal, A.; Cheng, Y.; and Pavlou, P. Maximizing online revisiting and purchasing: A clickstream-based approach to enhancing customer lifetime value. Journal of Management Information Systems, 40, 2 (2023), 470–502.
- Johnson, E.J.; Moe, W.W.; Fader, P.S.; Bellman, S.; and Lohse, G.L. On the depth and dynamics of online search behavior. Management Science, 50, 3 (2004), 299–308.
- Kar, A.K.; Angelopoulos, S.; and Rao, H.R. Big data-driven theory building: Philosophies, guiding principles, and common traps. International Journal of Information Management, 71, 102661 (2023), 1–7.
- Kim, J.B.; Albuquerque, P.; and Bronnenberg, B.J. Mapping online consumer search. Journal of Marketing Research, 48, 1 (2011), 13–27.
- Knoben, J.; Oerlemans, L.A.; Krijkamp, A.R.; and Provan, K.G. What do they know? The antecedents of information accuracy differentials in interorganizational networks. Organization Science, 29, 3 (2018), 471–488.
- Kossinets, G.; and Watts, D.J. Empirical analysis of an evolving social network. Science, 311, 5757 (2006), 88–90.
- Kossinets, G.; and Watts, D.J. Origins of homophily in an evolving social network. American Journal of Sociology, 115, 2 (2009), 405–450.
- Kushwaha, T.; and Shankar, V. Are multichannel customers really more valuable? The moderating role of product category characteristics. Journal of Marketing, 77, 4 (2013), 67–85.
- Lee, T.Y.; and Bradlow, E.T. Automated marketing research using online customer reviews. Journal of Marketing Research, 48, 5 (2011), 881–894.
- Li, B.; Li, X.; and Liu, H. Consumer preferences, cannibalization and competition: Evidence from the personal computer industry. MIS Quarterly, 42, 2 (2018), 661–678.
- Li, H.; Calder, C.A.; and Cressie, N. Beyond Moran’s I: testing for spatial dependence based on the spatial autoregressive model. Geographical Analysis, 39, 4 (2007), 357–375.
- Li, S.; Liu, Y.; and Feng, J. Who should own the data? The impact of data ownership shift from the service provider to consumers. Journal of Management Information Systems, 40, 2 (2021), 366–400.
- Lin, C.F. Segmenting customer brand preference: demographic or psychographic. Journal of Product & Brand Management, 11, 4 (2002), 249–268.
- Liu, X. Identification and efficient estimation of simultaneous equations network models. Journal of Business & Economic Statistics, 32, 4 (2014), 516–536.
- Liu, Y.; and Cui, T.H. The length of product line in distribution channels. Marketing Science, 29, 3 (2010), 474–482.
- Liu, Y.; Qian, Y.; Jiang, Y.; and Shang, J. Using favorite data to analyze asymmetric competition: Machine learning models. European Journal of Operational Research, 287, 2 (2020), 600–615.
- Markóczy, L.; Li Sun, S.; Peng, M.W.; Shi, W.; and Ren, B. Social network contingency, symbolic management, and boundary stretching. Strategic Management Journal, 34, 11 (2013), 1367–1387.
- Martínez‐de‐Albéniz, V.; Planas, A.; and Nasini, S. Using clickstream data to improve flash sales effectiveness. Production and Operations Management, 29, 11 (2020), 2508–2531.
- McEvily, B.; and Zaheer, A. Bridging ties: A source of firm heterogeneity in competitive capabilities. Strategic Management Journal, 20, 12 (1999), 1133–1156.
- Mena, C.; Karatzas, A.; and Hansen, C. International trade resilience and the Covid-19 pandemic. Journal of Business Research, 138 (2022), 77–91.
- Miranda, S.; Berente, N.; Seidel, S.; Safadi, H.; and Burton-Jones, A. Editor’s comments: Computationally intensive theory construction: A primer for authors and reviewers. MIS Quarterly, 46, 2 (2022), iii–xviii.
- Moe, W.W.; and Fader, P.S. Dynamic conversion behavior at e-commerce sites. Management Science, 50, 3 (2004), 326–335.
- Moe, W.W. An empirical two-stage choice model with varying decision rules applied to internet clickstream data. Journal of Marketing Research, 43, 4 (2006), 680–692.
- Moody, J.; McFarland, D.; and Bender-deMoll, S. Dynamic network visualization. American Journal of Sociology, 110, 4 (2005), 1206–1241.
- Netzer, O.; Feldman, R.; Goldenberg, J.; and Fresko, M. Mine your own business: Market-structure surveillance through text mining. Marketing Science, 31, 3 (2012), 521–543.
- Newman, J.W.; and Lockeman, B.D. Measuring prepurchase information seeking. Journal of Consumer Research, 2, 3 (1975), 216–222.
- Opsahl, T.; Agneessens, F.; and Skvoretz, J. Node centrality in weighted networks: Generalizing degree and shortest paths. Social networks, 32, 3 (2010), 245–251.
- Paulssen, M.; and Bagozzi, R.P. Goal hierarchies as antecedents of market structure. Psychology & Marketing, 23, 8 (2006), 689–709.
- Perera, S.; and Tan, D. In search of the “Right Price” for air travel: First steps towards estimating granular price-demand elasticity. Transportation Research Part A: Policy and Practice, 130 (2019), 557–569.
- Peter, J.P.; Olson, J.C., and Grunert, K.G. Consumer behavior and marketing strategy. DaLian: Dongbei Univeristy of Finance & Economics Press, 1998.
- Ravindran, K.; Susarla, A.; Mani, D.; and Gurbaxani, V. Social capital and contract duration in buyer-supplier networks for information technology outsourcing. Information Systems Research, 26, 2 (2015), 379–397.
- Reed, W.R. On the practice of lagging variables to avoid simultaneity. Oxford Bulletin of Economics and Statistics, 77, 6 (2015), 897–905.
- Ringel, D.M.; and Skiera, B. Visualizing asymmetric competition among more than 1,000 products using big search data. Marketing Science, 35, 3 (2016), 511–534.
- Rowley, T.; Behrens, D.; and Krackhardt, D. Redundant governance structures: An analysis of structural and relational embeddedness in the steel and semiconductor industries. Strategic Management Journal, 21, 3 (2000), 369–386.
- Sasidharan, S.; Santhanam, R.; Brass, D.J.; and Sambamurthy, V. The effects of social network structure on enterprise systems success: A longitudinal multilevel analysis. Information Systems Research, 23, 3-part-1 (2012), 658–678.
- Sismeiro, C.; and Bucklin, R.E. Modeling purchase behavior at an e-commerce web site: A task-completion approach. Journal of Marketing Research, 41, 3 (2004), 306–323.
- Sivakumar, K. Manifestations and measurement of asymmetric brand competition. Journal of Business Research, 57, 8 (2004), 813–820.
- Stine, R.A. Graphical interpretation of variance inflation factors. The American Statistician, 49, 1 (1995), 53–56.
- Stokburger-Sauer, N.E.; and Teichmann, K. Is luxury just a female thing? The role of gender in luxury brand consumption. Journal of Business Research, 66, 7 (2013), 889–896.
- Struijk, M.; Angelopoulos, S.; Ou, C.; and Davison, R.M. Influencing information quality: Evidence from a military organization. European Conference on Information Systems: Association for Information Systems, 2020.
- Struijk, M.; Ou, C.X.; Davison, R.M.; and Angelopoulos, S. Putting the IS back into IS research. Information Systems Journal, 32, 3 (2022), 1–4.
- Struijk, M.; Angelopoulos, S.; Ou, C.X.; and Davison, R.M. Navigating digital transformation through an information quality strategy: Evidence From a military organization. Information Systems Journal, 33, 4 (2023).
- Sun, C.; Zhang, X.; Zhou, Y.-W.; and Cao, B. Pricing, financing and channel structure for capital-constrained dual-channel supply chains with product heterogeneity. International Journal of Production Economics, 253 (2022), 108591.
- Tan, T.F.; Netessine, S.; and Hitt, L. Is Tom Cruise threatened? An empirical study of the impact of product variety on demand concentration. Information Systems Research, 28, 3 (2017), 643–660.
- Tan, X.; Jin, F.; and Dennis, A.R. How Appreciation and attention affect contributions to electronic networks of practice. Journal of Management Information Systems, 39, 4 (2022), 1037–1063.
- Tortoriello, M. The social underpinnings of absorptive capacity: The moderating effects of structural holes on innovation generation based on external knowledge. Strategic Management Journal, 36, 4 (2015), 586–597.
- Upson, J.W.; Ketchen Jr, D.J.; Connelly, B.L.; and Ranft, A.L. Competitor analysis and foothold moves. Academy of Management Journal, 55, 1 (2012), 93–110.
- Van Mierlo, T. The 1% rule in four digital health social networks: an observational study. Journal of Medical Internet Research, 16, 2 (2014), e2966.
- Ver Hoef, J.M.; Peterson, E.E.; Hooten, M.B.; Hanks, E.M.; and Fortin, M.J. Spatial autoregressive models for statistical inference from ecological data. Ecological Monographs, 88, 1 (2018), 36–59.
- Wasserman, S., and Faust, K. Social network analysis: Methods and applications. Cambridge: Cambridge University Press, 1994.
- Wu, L. Social network effects on productivity and job security: Evidence from the adoption of a social networking tool. Information Systems Research, 24, 1 (2013), 30–51.
- Yang, S.; and Allenby, G.M. Modeling interdependent consumer preferences. Journal of Marketing Research, 40, 3 (2003), 282–294.
- Yang, Y.; and Fik, T. Spatial effects in regional tourism growth. Annals of Tourism Research, 46 (2014), 144–162.
- Zhang, J.J.; Fang, X.; and Liu Sheng, O.R. Online consumer search depth: Theories and new findings. Journal of Management Information Systems, 23, 3 (2006), 71–95.
- Zheng, Z.; Fader, P.; and Padmanabhan, B. From business intelligence to competitive intelligence: Inferring competitive measures using augmented site-centric data. Information Systems Research, 23, 3-part-1 (2012), 698–720.
- Zuo, M.; Angelopoulos, S.; Liang, Z., and Ou, C.X. Blazing the Trail: Considering Browsing Path Dependence in Online Service Response Strategy. Information Systems Frontiers, 25, 4 (2022), 1605–1619.