ABSTRACT
While IT workforce research often examines job- and organizational-related reasons for turnover, studies rarely connect the immediate social context of IT work to IT professionals’ workplace behavior. To understand the influence of the immediate social context, we develop a social comparison model that connects the social influence of co-worker who have left an organization to turnover among IT professionals who remain. We test our model using a social network of 4,011 IT professionals employed in a large IT firm over four years. We complement our analysis of this data with a multiple-case study with five software development teams. Across the two studies, our results suggest that the departure of an IT professional increases the probability of turnover among remaining coworkers; further, we found that turnover is even more likely when the remaining IT professionals are similar in technical abilities and demographic attributes to the co-worker who left. Our results direct attention to the immediate social context as an influence on the turnover behavior of IT professionals and explain how similarity in domain-specific attributes shapes this turnover behavior. Practitioners should know that a single departure may cause a chain reaction in IT work teams and organizations and find suggestions for assigning new employees.
Introduction
The turnover of IT professionals poses many problems for organizations. First, it is difficult to find replacements for IT professionals because of the high demand in IT labor markets [Citation84]. Second, turnover creates high costs for IT organizations related to the direct costs of recruiting and indirect costs associated with the disruption of organizational processes [Citation82, Citation83] that range between 90% and 700% of the annual salary of an IT professional [Citation43]. Because of ongoing staffing difficulties and replacement costs, the antecedents of voluntary turnover are an important topic for IT workforce research [Citation37, Citation98].
Although IT workforce studies have examined how individual factors such as job satisfaction or role stress lead to turnover [Citation2], there has been scant empirical work probing how the immediate social context influences turnover among IT professionals. Some evidence has suggested that the formal structure of IT work shapes IT professionals’ job satisfaction and stress. For example, Ply, Moore, Williams, and Thatcher [Citation71] found evidence showing that software development structure impacts antecedents of turnover, such as satisfaction and work overload; however, they left the actual turnover of IT professionals unexamined. Similarly, while Tripp, Riemenschneider, and Thatcher [Citation86] found that work practices influence IT professionals’ perceived job characteristics and job satisfaction, they did not examine how these contextual factors relate to turnover. Generally speaking, the literature suggests that the structure of IT work shapes the beliefs and attitudes of IT professionals, which can lead to turnover or turnover intentions.
While IT workforce research has examined formal structure and job design, less attention has been paid to the informal social context, which is surprising given the broader discipline focuses on the interplay between people and technology in the broader sociotechnical systems in which IT professionals work. The informal social context is important, as disruptions in context can cause turnover. For example, departing employees (i.e., employees who have left or are in the process of leaving an organization) may provide personal insight into the interview process, clarify what attributes employers find desirable, or even attempt to recruit coworkers to leave with them. By doing so, a departing IT team member can disrupt remaining members’ connections to the organization by demonstrating that job opportunities exist; more importantly, they can do so by demonstrating to their peers that there is demand for people with similar abilities and characteristics [Citation68]. Thus, connecting social context to turnover among IT professionals is important because coworkers may share essential information [Citation13, Citation30] that informs their decision to turnover [Citation2, Citation5]. Hence, we investigate the question: “How does the departure of coworkers influence the turnover behavior of IT professionals remaining in the organization?”
We ground our investigation of IT professionals, their social context, and turnover in social comparison theory [Citation22], which suggests that individuals compare themselves to others, especially in novel and risky situations [Citation46, Citation80]. We contextualize this argument to the IT workforce and argue that IT professionals who view departing coworkers as possessing similar demographic attributes or contextually similar technical skills will be more likely also to leave the organization. We tested this using actual team and turnover data gathered from 4,011 IT professionals in one IT consulting organization’s social network from 2008-2012. Specifically, we estimated the dynamic probability of transmitting turnover information in the social network of IT professionals over time. We used survival analysis to determine whether the departure of 1,403 coworkers increased the probability of turnover among the remaining IT professionals in the organizational network. We update this understanding using qualitative data from 17 interviews gathered between 2019 and 2021 in five teams to confirm our findings on social comparison, technical ability, and demographic attributes. By triangulating across methods and time, our studies provide evidence that IT professionals’ social comparisons with departing co-worker magnify the impact of job options. We found that similarities in terms of abilities and personality attributes amplify the impact of a departing IT team member on turnover. Finally, we explain how our conceptualization of social comparison in turnover situations can scale to work in the IT function. Our findings highlight that managers should pay attention to why IT professionals leave and how that departure affects remaining coworkers, who may themselves become more vulnerable to turnover.
Theoretical Background
Since the inception of the information systems discipline, IS research has examined IT professionals [Citation37, Citation93, Citation98]. Factors linked to turnover include job-related factors such as role conflict, individual attributes such as age and gender, and organizational-related factors such as pay and promotability [Citation37, Citation70]. While these factors help explain why IT professionals leave a job, most of these models either leave the influence of coworkers unexamined or assume that the actions of others do not influence individual behavior. When models of turnover among IT professionals take context into account, they generally focus on the formal organization of work [Citation71, Citation86], internal or external labor markets [Citation3, Citation38], or broader economic conditions [Citation83].
While most studies focus on how IT professionals’ perceptions of their work or employer lead to turnover, evidence suggests a need to explore the impact of their immediate social context—i.e., coworkers—on turnover. First, a few studies have explored IT professionals’ turnover in groups or as a function of IT group culture. Naidoo [Citation63] observed that IT professionals jointly share experiences with organizational change and collectively engage in turnover as a response. Similarly, Burke and Moore [Citation10] described IT group culture as either tolerating or disapproving of turnover behavior among group members. Other studies have highlighted how the density of ties within working groups shapes behavior [Citation13, Citation29]. These studies suggest that collective turnover, or the turnover of multiple employees, results from shared communicative and negotiation processes within a network of IT professionals.
By focusing on the immediate social context, IT workforce research can help explain why closely-knit groups of IT professionals form withdrawal cognitions and leave organizations en masse. For example, the social characteristics of departing IT professionals, such as similarity [Citation96], social support [Citation51], and relational closeness [Citation89], may shape their remaining team members’ quitting intentions [Citation30]. Further, while some research has suggested that IT professionals’ social networks can prevent them from quitting [Citation17, Citation37, Citation58], we suspect that turnover within that same network can magnify withdrawal cognitions, as departing IT professionals likely share information related to their turnover with their former team members.
Comparison Theory
Social comparison theory (SCT) provides a plausible explanation for how the immediate social context shapes IT professionals’ behavior. SCT builds on the idea that individuals strive to validate their opinions and the value of their abilities [Citation22] through comparison with others [Citation11]. When complete information is not available, social comparison fills critical gaps in the information that individuals use to make decisions. For example, if individuals cannot find objective data to validate their opinions about specific potential outcomes, they often compare themselves to individuals with similar abilities in their environment, which makes them feel more confident in predicting the outcomes of their actions in a similar environment [Citation80].
SCT can help clarify why a coworker’s departure might inform an IT professional’s turnover decision. Individuals apply social comparison in novel, risky, and ambiguous situations [Citation22]. Since IT job transitions come with high levels of risk and uncertainty [Citation79], SCT can help explain how IT professionals who possess substantial “local knowledge” about IT, processes, and customers likely view the risks and benefits associated with changing jobs [Citation17]. For example, an IT professional may perceive a coworker’s departure as a signal that changing jobs is less risky than previously assumed, particularly if they have similar technical abilities. In essence, a coworker’s departure may cause IT professionals to feel less embedded in their organizations [Citation17, Citation83].
While SCT has not been used to explain the turnover of IT professionals or knowledge workers, evidence from studies of manual and clerical workers suggests that social information shapes turnover. In a study of fast-food employees, Krackhardt and Porter [Citation46] found that turnover communication negatively affects the attitudes of employees in similar job roles who remain in the organization. In a study of supermarket employees, Feeley and Barnett [Citation20] found that after a coworker’s departure, socially equivalent employees on the social network’s edges were more likely to quit. Finally, in a study of bank employees, Felps, Mitchell, Hekman, Lee, Holtom, and Harman [Citation21] found that turnover spread among coworkers across 45 branch locations. These studies suggest that information shared by departing coworkers impacts job attitudes and turnover intentions among remaining employees.
Contextualizing Social Comparison to Explain IT Professionals’ Turnover Behavior
While SCT directs attention to how individuals assess available options, we believe that social comparison by IT professionals may impact their abilities to realize other employment options. This subtle nuance, the difference between assessing availability and ability, is essential for understanding how the immediate social context shapes IT professionals’ turnover.
Regarding availability, IT professionals work in competitive markets, often with plentiful job alternatives. It is well-established that perceptions of job alternatives drive actual turnover among IT professionals [Citation83]. Even when general labor markets are weak, the specialized skills of IT professionals may afford them access to job opportunities, and they may be frequently contacted about job alternatives by peers in their network as well as headhunters [Citation39]. The competitive IT labor market has persisted over time, with IT job vacancy rates in the European Union ranging in the top three across all industries in the last decade. In 2021, the IT labor market vacancy rates were among the top five in Europe despite COVID-19ʹs direct impacts on industries such as accommodation and food services [Citation19].
We suspect that IT professionals making social comparisons with departing coworkers magnifies their beliefs about how well their abilities match job opportunities. Because of the collaborative nature of IT work and the resulting interpersonal relationships that develop, these social comparisons may be particularly impactful for IT professionals [Citation4, Citation29, Citation49, Citation54]. The concept of collaborative elaboration helps explain how IT professionals’ close collaboration in solving technical problems can translate into turnover [Citation55]. Research on IT project teams has reported strong bonds within teams of IT professionals, with mechanisms such as informal clan control structures shaping shared team vision, norms, and definitions of acceptable behavior [Citation13]. Such bonds allow IT professionals to closely observe and interact with their peers so that they can judge a previous coworker’s performance. IT professionals can use this knowledge of the peer performance of an IT professional as a reference point for their own behavior, particularly when evaluating job alternatives [Citation21]. We thus expect that IT professionals who deem themselves to be just as capable as the departing coworker would be more likely to switch jobs.
When making social comparisons to assess potential turnover opportunities, IT professionals may focus on coworkers with similar characteristics. Two important dimensions of similarity among IT professionals are contextually similar technical skills and personal characteristics. Contextually similar technical skills means that IT professionals possess similar skills required to collaborate to complete IT tasks such as software development or project management [Citation14, Citation47, Citation81]. IT professionals likely possess high levels of contextually similar technical skills if they have worked together closely to develop joint artifacts and have shared episodic knowledge about projects [Citation75, Citation76]. Such close collaboration is a particularly important characteristic of IT work because it helps mitigate the high levels of uncertainty associated with completing poorly structured tasks, increases goal alignment, facilitates straightforward feedback, and fosters positive interactions [Citation24, Citation48, Citation55].
However, similarity in IT professionals’ attributes is of particular interest, given that homogeneity in the IT workforce is thought to impact certain demographic groups negatively [Citation44]. Similarity in demographic attributes means that IT professionals possess comparable training and are similar in gender and age [Citation81, Citation91], for example, and evidence suggests that age and gender may be relevant attributes [Citation62]. Social networks of IT professionals often exclude female IT professionals [Citation1, Citation41], resulting in more frequent workplace discrimination among women [Citation85]. Also, depending on when they entered the IT workforce, IT professionals often share technology and work experiences, resulting in shared expectations regarding possible job opportunities [Citation67]. Other evidence confirms that gender correlates with discrimination experience [Citation25, Citation73, Citation74] and age correlates with job embeddedness and the motivation to update skills [Citation6, Citation96]. We argue that IT professionals who are similar to a departing coworker in terms of demographic attributes such as gender and age will be more likely to form favorable social comparisons about their ability to secure new employment and be more likely to leave the organization. We propose a parsimonious model of social comparison and turnover among IT professionals (see ) and further develop arguments that support our research model below.
Research Model
We hypothesize that a coworker’s turnover increases the likelihood of turnover among IT professionals. When a coworker quits, IT professionals are made more aware of potential employment alternatives, triggering a process through which they may compare and evaluate their present job vis-à-vis alternative jobs [Citation18, Citation59]. Particularly because of the collaborative nature of IT work, the turnover of an IT professional is likely to evoke a favorable assessment among coworkers regarding their ability to secure jobs that are well-suited to their individual needs [Citation21].
Hence, we posit that when an IT professional leaves an organization, it increases the likelihood of turnover among coworkers. More formally, we hypothesize:
H1: The turnover of an IT professional is positively related to coworkers’ turnover behavior.
Social comparison theory suggests that comparison with peers influences turnover motivation among IT professionals, particularly among peers perceived as being similar in ability [Citation47]. For example, when studying turnover in bank branches, Felps et al. [Citation21] found that the contagious effect exerted by a coworker leaving was stronger in smaller branches. The authors speculate that the effects were stronger due to closer collaboration, leading to a greater understanding of coworker ability [Citation21]. When IT professionals compare themselves to coworkers who are similar in ability, we presume that they will be more likely to turnover.
We anticipate that contextual similarity in technical skills amplifies the relationship between the immediate social context and turnover among IT professionals. IT professionals who possess similar technical skills likely have a better understanding of the work context and the reasons for coworker turnover and are more likely to compare themselves with a departing coworker [Citation14, Citation47, Citation81]. Close collaboration increases the contextual similarity in technical skills, particularly because IT professionals jointly produce intangible artifacts, resulting in shared topics, episodic technical knowledge [Citation75], and joint technical competencies [Citation76]. For example, IT outsourcing projects, which involve residual incomplete time-and-material contracts, are associated with high levels of uncertainty for IT professionals. Such contracts are often poorly specified and incomplete, requiring IT professionals to collaborate closely with the team and the customer to realize shared goals [Citation24]. IT professionals working on these projects thus become very familiar with each other, resulting in an immediate social context that strongly influences coworkers in turnover situations.
Summarizing this line of thought, we argue that similarity in technical skills magnifies the impact of a departing IT professional on turnover intentions among coworkers. We argue that IT professionals either already possess this similarity or develop it during IT projects on which they collaborate. More formally, we hypothesize:
H2: An IT professional’s turnover increases the probability of turnover among coworkers if they possess high contextual similarity in technical skills.
When making comparisons with departing coworkers, we anticipate that similarity in demographic attributes results in higher levels of turnover among IT professionals. SCT suggests that IT professionals are more likely to seek the advice of individuals with similar attributes [Citation81]. We focus on similarity in demographic attributes in terms of gender and age.
We predict that similarity in gender magnifies the effects of an IT professional’s turnover on coworker turnover intentions. Recent research supports the idea that female IT professionals are frequently redlined in IT work environments [Citation41]. In this case, redlining refers to limiting opportunities for women in IT by, for example, providing less support in the workplace, supporting fewer career choices, and offering fewer opportunities for career advancement [Citation1]. Also, in male-dominated IT work environments, men may exclude women from traditionally gendered conversations such as sports or gaming [Citation42]. Given these gendered fault lines in the IT workforce, we find it likely that IT professionals use gender to judge attribute similarity and make social comparisons.
We anticipate that similarity in age also magnifies the effects of a departing IT professional’s turnover on coworker turnover intentions. IT workforce research suggests that older IT professionals are less likely to leave their jobs [Citation96] because they either feel that their skills are increasingly obsolescent [Citation27, Citation34] or because they rely on their employing organization to provide the resources necessary to update skills and avoid becoming obsolete [Citation96]. IT professionals of a similar age likely have similar training, applied skills, and work experiences that they use to define themselves [Citation50]. When an IT professional leaves to take a new job, coworkers of similar ages may make favorable social comparisons about their ability to find a new job, which will thus likely increase turnover intentions. Hence, we posit that when an IT professional leaves to take a new job, the likelihood of turnover among coworkers of the same gender or age group will increase. More formally, we hypothesize:
H3a: The probability of turnover increases among coworkers of the same gender as a departing IT professional.
H3b: The probability of turnover increases among coworkers in the same age group as a departing IT professional.
Research Method
In the empirical assessment of the research model and its associated hypotheses, we followed a sequential mixed-methods research design using quantitative and qualitative data collection [Citation87]. The quantitative design comprised a network analysis of an archival data set provided by a large IT company, ALPHA.Footnote1 The subsequent qualitative analysis comprised a confirmatory multiple case study of five IT teams at ALPHA and other companies.
Study 1: Network Analysis
Our dataset captured the full network of IT professionals who worked at ALPHA from 2008 to 2012. We used network analysis to understand the effects of the immediate social context on turnover in the organizational network. Our analysis consisted of three steps. First, we used hours worked to calculate the probability of transmitting turnover information in the social network. Second, we calculated the continuation of this probability over time. Third, we conducted a survival analysis based on social comparison to predict the probability of IT professional’s turnover.
Dataset
Data were extracted from the internal project control and HR systems, which contained detailed information on the daily work history and assigned projects of IT professionals. ALPHA’s 4,011 IT professionals were, on average, 38.7 years old and comprised 927 (23%) women and 3,084 (77%) men. They worked on more than 15,000 projects, lasting 210 days, on average, with a typical team size of 4.4. They worked an average of 36.68 hours per week on 1.4 different projects per week in parallel.
We removed IT professionals who were likely to quit for reasons other than taking a new job. Based on advice from ALPHA’s HR department, we removed very young and very old IT professionals from the dataset. We removed IT professionals younger than 25 years of age (n=203) because managers at ALPHA indicated that this cohort is more likely to quit to pursue further studies or may be working as interns on limited-time contracts. We also removed professionals older than 60 (n=31) because members of this group may leave the organization due to retirement.
After removing these two age groups, the ALPHA dataset contained 1,403 turnover cases. On average, IT professionals who left had worked for ALPHA for 3.7 years. The annual turnover rate was between 10.2% and 12.3%, corresponding to a typical IT company’s average yearly turnover rate [Citation77].
The organizational network of ALPHA
Network analysis is commonly applied to social phenomena [Citation8, Citation9]. For our analysis, the organizational network is the set of dyadic relationships between IT professionals within ALPHA based on their work history [Citation88]. We used graphs as a mathematical representation of the organizational network, which made it easier to develop a vocabulary and perform the mathematical operations necessary to study the organizational network [Citation90]. As such, the employees are represented by nodes (in graph theory “vertices”) in the network, which are connected by edges (in graph theory “arcs”) [Citation90].
We constructed an event-type network by aggregating the individual times of collaboration among IT professionals [Citation9]. The underlying graph is undirected, valued, and single-node [Citation90]. It is undirected in that we know that there is a relationship, but the direction of the influence is unknown. The graph is valued, as the edges are weighted according to the time the IT professionals collaborated. It is single-node because we only have one type of node [Citation9]. The individual IT professionals in our organizational network represent the nodes of the graph; the edges of the graph represent the aggregated length of time they collaborated on the same project. illustrates these networks and shows how turnover events can spread over time, which we will elaborate on later.
Figure 2. Illustration of Organizational Network and Spread of Turnover in Sample Work Unit Consisting of Three Teams
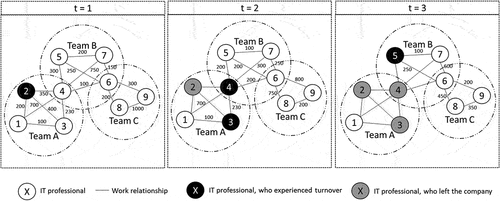
Since the collaboration of IT professionals has not been stable over time, we used weekly work data for our analysis (see for an illustration). For every week under consideration, we calculated the set of individuals with whom a particular professional worked on the same project in the past [Citation95]. On average, the organizational network of one of our professionals consisted of 46 direct contacts and 436 second neighbors (contact of a contact).
Assortativity, a standard measure of network quality, describes whether nodes with a similar degree tend to be connected to each other [Citation64] and results in values between -1 and 1. As the organizational network of ALPHA was not stable over time, we calculated the assortativity of the network for every week between the beginning of 2008 and the end of 2012. For our analysis, the assortativity score is 0.55, which is typical for social networks where people tend to be connected to people who are similar [Citation65]. We also calculated the average distance (hours worked together) between all nodes during 2008-2012 on a weekly basis. The average edge value of 3.31 shows that the network is well-connected [Citation65].
Calculating the effect of immediate social context on IT professionals’ turnover
To calculate the effect of the immediate social context on IT professionals’ turnover (H1), we analyzed individual ego networks [Citation8]. We thus analyzed each employee as the center of one network. For this analysis, we focused on the immediate ego network, where we considered the edges leading to the direct neighbors only. We weighted the application of degree centrality as the number of incident edges [Citation90]. We used this graph information as input for our survival analysis.
Our calculation of the effect of the immediate social context on the turnover of IT professionals considered whether there was a turnover case among direct contacts having a working relationship within the past year. We used an exemplary network () to illustrate the calculation of the effects of the social context. Node 2 represents an IT professional who experienced turnover in the time period t = 1. The edges were weighted with the hours the professionals worked together in the past year. In the exemplary organizational network of , Turnover Node 2 worked with Nodes 1, 3, and 4. In the following time period (t = 2), Nodes 3 and 4 were affected by Node 2ʹs turnover and engaged in turnover themselves. Finally, Node 5 in Team B was affected by Node 4ʹs turnover in the time period t = 3. The strength of turnover influence is calculated by dividing the number of hours worked during the last year with the coworker(s) who experienced turnover by the total number of hours worked with all coworkers during the last year.
Dynamic network analysis: Calculating turnover influence over time
Longitudinal data between 2008 and 2012 captures changes in the network over time. To capture this dynamic, we set the unit of analysis to one week to capture smaller influences [Citation95]. We calculated the strengths of turnover influence for every professional on a weekly basis between the beginning of 2008 and the end of 2012 ().
Since a coworker’s turnover in previous weeks may influence IT professionals, we employed a remembrance factor that transfers a certain amount of turnover “energy” to the next week. Not all of the influence is transferred because its influence diminishes over time. We calibrated three characteristics of our turnover network as follows: First, we limited the diminishment to 5% of the turnover influence. Thus, turnover influence is transferred until it drops below the 5% level. Second, we set a lag time of one year, i.e., the maximum possible turnover influence lasts for one year. Using one year as a lag time to analyze the influence of predictors on actual turnover is a common approach used in turnover research [Citation26]. Third, we specified a remembrance factor to account for turnover information from the previous weeks. Based on the first two characteristics, we set an optimal remembrance factor of 88%, which led to a duration of 12 months as lag time, given the defined diminishment level. This duration is in line with extant literature on turnover spread [Citation20, Citation21, Citation31, Citation45, Citation63].
In sum, the strength of turnover influence consists of the aggregated influence from the previous weeks plus the newly acquired influence within the present week. This led to a final dataset consisting of 575,279 weekly entries for 4,011 unique IT professionals, including those who experienced turnover and those who did not.
Survival analysis
We used the graph information from our social network analysis as input for our survival analysis. Survival analysis analyses the influence of external factors on the expected length of time it will take for a specific event to occur [Citation56, Citation60]. While survival analysis was developed to estimate patient survival time in a medical context, i.e., the length of time until death [Citation56, Citation60], it can be used to analyze the time it takes for the occurrence of any event that can be described in binary terms, i.e., either “the event happened” or “the event didn’t happen” [Citation61].
We used survival analysis to analyze our longitudinal dataset to estimate the probability of an IT professional leaving a job with a given set of covariates. We employed Cox proportional hazard models [Citation15] because they permit time-dependent covariates [Citation56]. In our case, some variables, such as the magnitude of turnover influence, age, and business confidence, vary over time. Cox proportional hazard models also allow for censored survival data [Citation60]. Our model includes right- and left-censored data because there were IT professionals in the dataset who had not experienced turnover by the end of our observation period, and there were IT professionals who started working at ALPHA before the beginning of the observation period. Finally, we used Cox proportional hazard models because they do not require assumptions about the baseline survival distribution [Citation60]. presents an overview of the definition and the calculation of our variables.
Table 1. Measures
Descriptive statistics
shows the mean, the standard deviation, and the correlation of the numerical variables of the dataset used for the survival analysis. We also used several categorical variables in our analysis. Age was categorized into seven five-year ranges: 26 to 30 years (666 professionals), 31 to 35 (659 professionals), 36 to 40 (589 professionals), 41 to 45 (605 professionals), 46 to 50 (433 professionals), 51 to 55 (259 professionals), 56 to 60 (128 professionals). The 15,289 conducted projects were categorized into nine different industry groups: internal (5,103 projects), automotive (3,650 projects), banking (2,317 projects), insurance (2,796 projects), information and communication (368 projects), manufacturing (307 projects), public administration (273 projects), utility (97 projects), other industries (358 projects). The contract type was grouped into fixed-price (3,941 projects), time-and-material (6,934 projects), and internal projects without a customer (4,394 projects).
Table 2. Means, Standard Deviations, and Correlations of Study Variables
Study 2: Confirmatory Case Study
We conducted Study 2 to confirm that the effects found in the older ALPHA dataset in Study 1 remain true. We conducted a multiple-case study of software development teams that experienced turnover in the last three years [Citation87, Citation94]. Between 2019 and 2021, we collected interview data for the five cases using a retrospective design to understand how the immediate social context of IT work influences IT professionals’ turnover [Citation72].
Data collection and analysis
We collected data from five software development teams that experienced turnover (see ). We refer to the teams based on the industry of the company in each case: Furniture, Insurance, Auto, SmartDevice, and Aviation. We theoretically sampled teams where turnover occurred, as we wanted to examine how turnover events unfolded. We went back to ALPHA to collect new data within the same organization (Insurance and SmartDevice cases) but also went to other organizations (Furniture, Auto, and Aviation cases). Teams differed in terms of size, similarity in demographic attributes, and contextual similarity in technical skills.
Table 3. Overview of Cases and Data Collected
Table 4. Survival Model for Turnover Influence from the Immediate Social Context
Table 5. Survival Models for Turnover Influence from the Immediate Social Context with or without Contextual Similarity in Technical Skills
Table 6. Survival Models for Turnover Influence from the Immediate Social Context with or without Similarity in Attributes (Gender)
Table 7. Survival Models for Turnover Influence from the Immediate Social Context with or without Similarity in Attributes (Age)
For the five cases, we conducted 17 semi-structured interviews with current and former team members who worked with a departing team member or were departing team members themselves. In the interviews, we asked about turnover events in the interviewee’s social context. Once we identified turnover events in the unit of analysis (the software development team), we focused on each event and analyzed how it affected the interviewee and the overall team [Citation94]. We identified all the following turnover events as either related to the initial turnover or not related.
The data were analyzed according to general themes representing our construct of interest. We used a pre-structured case analysis with our conceptual framework [Citation57]. We developed a case outline that focused on the immediate social context in turnover situations and demographic and task-specific attributes. The classification was performed by two expert coders who were not involved in Study 1. Before coding, we constructed a timeline of events for each case. Then, the experts coded each case using the case outline.
Results
To analyze our hypotheses, we estimated several survival models. Model 1 analyzes the effect of turnover influence on the immediate social context (). Models 2 and 3 consider the influence of the immediate social context with and without contextual similarity in technical skills (). Models 4 and 5 analyze the influence of the immediate social context with and without similarity in demographic attributes based on gender (). Models 6 and 7 analyze the influence of the immediate social context with and without similarity in demographic attributes based on age ().
The survival model for the turnover influence from direct relationships (Model 1 in ) shows a significant influence of 3.16, p < 0.01. Therefore, H1 (the turnover of an IT professional is positively related to coworkers’ turnover behavior) is supported. The two survival models that consider social influence with or without contextual similarity in technical skills show significant influences from relationships of close collaboration and relationships with distant collaboration (0.10, p < 0.01 in Model 2 and 0.079, p < 0.01 in Model 3). We conducted a Wilcoxon signed rank test to compare the two effects. Results reveal a significant difference in influences from relationships of close collaboration and relationships with distant collaboration (W = 178, p < 0.001). Therefore, H2 (an IT professional’s turnover increases the probability of turnover among coworkers if they possess high contextual similarity in technical skills) is also supported.
The two survival models that consider social influence with or without similarity in gender show significant influences from relationships with IT professionals of the same gender and relationships with IT professionals of different genders 0.09, p < 0.01 in Model 4 and 0.05, p < 0.01 in Model 5). We conducted a Wilcoxon signed rank test to compare the two effects. Results reveal a significant difference in influences from relationships of close collaboration and relationships with distant collaboration (W = 4570, p < 0.001). Therefore, H3a (the probability of turnover increases among coworkers of the same gender as a departing IT professional) is supported.
The two survival models that consider social influence with or without similarity in age attributes do not show a significant difference if the two IT professionals belong to the same age group (0.02, p < 0.1 in Model 6 vs. 0.09, p < 0.01 in Model 7).Footnote2 Therefore, H3b (the probability of turnover increases among coworkers in the same age group as a departing IT professional) is not supported.
Confirmatory Case Studies
Across all five cases, the departure of a team member caused other team members to leave the organization. Several team members described how a team member’s departure influenced them. One team member from the Furniture team described that the turnover of a team member inspired a process of reflection: “I would say [the turnover of a team member] made me think. Reflect on it. I mean, people are usually creatures of habit; once you are in a permanent employment relationship, you don’t just quit […]. The turnover of [close colleague] was something [that] hit me hard. Yes, ok, maybe now my time has also come to move.”
Many of the 17 interviewees perceived turnover events similarly. They described a dynamic process that occurred after the departure of a team member. In situations where more than one team member left, interviewees described a tipping point that caused a large part of the team to submit their resignations. One team member from the Insurance team described this collective process: “And then […] the whole team broke apart. Because two weeks after I quit, the next one left. […] And then still two weeks later, [Team Member C] and [Team Member D] resigned on the same day. […] Just a month later, [Team Member E] also resigned. That was the last one of us, then only the team leader and another one remained. But she hadn’t been on the team for long and had done something else. And then, within two months, everyone was gone.”
When asked about how turnover events influenced their thinking, interviewees described comparing themselves to the departing employees. Several mentioned a long-lasting personal relationship, e.g., joint university studies or shared leisure activities, as a reason for this comparison. They referred to a similar “value system” and a similar perception of strategic life decisions such as career choices. Others saw themselves as similar to the departing employees because they had similar work experience since they had worked on similar projects and tasks for a long time. A member of the Auto team explained: “My old buddy left. And he told me a lot about the new place: better structured, better organized, much better team. And, you know, he worked with me side by side for several years, he should know what I am looking for. So, I trust him. I met the [new] team, and I did like it. Liked the boss, the young team. They seemed to be very caring and very positive. And this is when I handed in my notice to join [my old buddy’s new team].”
The interviews revealed why personal relationships with departing employees were meaningful for individual turnover decisions. Given the high number of open IT positions, headhunters frequently contact IT professionals about open positions. However, when approached through their informal social network, the information is better tailored to individual needs—particularly when provided by a trusted source whom the IT professional believes has similar values and experiences: “It felt like the great gold rush for new positions. [The departing team member] actively pushed everybody to look for jobs, and it felt like we were very similar minds. […] Similar in training, similar job description, basically did the same things here. So we all had the same reasons. And I trusted him before, why not now? […] I trusted him in our daily work, built on his code, asked for his advice.”
Contextual similarity in technical skills
Our interviews revealed that social comparison was strongly affected by similarity in technical skills across the five teams’ different working contexts. Several developers stated that they found it easier to relate to a colleague’s motives for leaving if they had a good understanding of what the departing person’s duties were. This was particularly true when task characteristics rather than social perceptions drove the turnover. “We had a core SAP developer who left, and when he quit, […] I had to take over. And I had two days to talk to him and then basically had to do his job. […] When I did what he used to do, I could really put myself in his shoes, saw what bugged him, and understood why the new job at [competitor] was better. And, I have to admit, I started looking for similar positions as well.”
In general, when using agile methodologies, teams were well aware of their colleagues’ tasks, progress, and particular strengths and weaknesses. Team members working in similar roles were closer to their colleagues in terms of professional training, working style, and “strategic career design decisions.” This effect was intensified when team members with particular expertise, such as the product owner, tester, or developer, left the project. One developer admired a departing colleague’s skills: “[Departing team member] was of good standing here. Really. He was one of the leading cloud experts and got so much done. High quality, great ideas. He took leadership roles in our community of practice and was well-known for his professional and personal skills. It was a strong signal when he left. It is not like some random dude left, but a well-recognized expert.”
Similarity in demographic attributes
Across all cases, the interviewees confirmed that they were particularly close to team members with similar demographic attributes. Interviewees described feeling closer to team members who were similar in apparent attributes such as gender and age. The SmartDevice team consisted of three women and 11 men. One of the women described her relationship with one of the other women on the team: “We got along great and always hung out together. […] We had different topics to talk about than the guys. And when I thought about leaving, we also talked about it: new job and where and how. No specific companies or anything, but it was clear that something was more likely to happen now. And it actually happened that we both quit on the same day.”
Our interviews also confirmed that team members felt closer to members of the same age group versus members of different age groups. This similarity was driven by both technical knowledge and interpersonal similarities. Interviewees explained that individuals from the same age group learned the same technologies and development approaches during vocational training or undergraduate studies. One team member from the Auto team explained that working with certain colleagues for a long time in ALPHA drew them together because they worked for the same managers and faced the same fundamental organizational changes. Similarly, team members from the same age group tend to be in similar life stages, which can potentially affect career decisions. One team member explained: “It is those phases in life that you share. You started at 25 [years of age] and worked for 10 to 15 years. And suddenly you are 40 and ask yourself, ‘Will I stay here forever, until I retire [at] 60?’ Because if you turn 50 and decide to leave, it is really hard to find something new. In your early forties, you are at the best stage of your career. You have lots of experience and are not on the scrap heap yet.”
In general, individuals from teams that were similar in demographic attributes were very emotional about turnover events in their teams. One interviewee described the turnover of a close team member as being “really bad for the team. Some of them cried, so it was pretty blatant. The others were totally shocked.” Particularly regarding teams that have worked together for a long time, personal friendships develop, and team members frankly and frequently share information about their personal lives. For example, when one team member in the Insurance team handed in his resignation because he was moving to a different country to live with his fiancée, team members in similar life stages reported relating to his particular situation.
The interviews revealed the severe effect of the COVID-19 pandemic on turnover dynamics, in general, and on the immediate social context of IT work, in particular. The Insurance and Aviation team members we interviewed had to cope with a sudden move toward virtual collaboration. Six months into the pandemic, a team member from the Aviation team explained how this virtual collaboration created distance between individuals: “And this new normal is different in terms of close social relationships […] I have to admit, I don’t know my colleagues as well anymore. Don’t know what they do, what they think. Someone might be totally unhappy. And is actively looking for other jobs. You are no longer sitting in the same office space, and you have no joint lunch break. This is where you get to know people develop close relationships.”
After more than 18 months of dealing with the COVID-19 pandemic, interviewees described the effect of the “new normal” as twofold. On the one hand, the COVID-19 pandemic increased IT professionals’ job options, as many jobs included a work-from-home component, offering a more extensive range of possible jobs for IT professionals. Given the high demand for IT professionals, this effect did not increase turnover in the organizations we interviewed. On the other hand, the COVID-19 pandemic changed the previously close relationships with coworkers due to the increase in virtual work. One interviewee reflected on the past 18 months of working almost entirely virtually: “There are some people whom I never met. However, we have been working together for a very long time now. But [COMPANY] organizes many virtual events such as virtual barbecues, wine tastings, or online networking events to unite people.” The managers we interviewed also described the various actions the organizations took to improve team spirit and job embeddedness for virtual employees.
Discussion
Grounded in social comparison theory, our analysis found that the immediate social context influences turnover among IT professionals. We further found that similarity in technical skills and gender amplified the effect of a coworker’s turnover on the remaining IT professionals in an organization. Our results show that coworker turnover, along with its interplay with ability and attributes, meaningfully contributes to turnover among IT professionals.
Theoretical Implications
Our analysis of social comparison in the context of turnover among IT professionals has three important implications: (1) We direct attention to the immediate social context as an influence on the turnover behavior of IT professionals, which magnifies the impact of job options; (2) we explain how similarity in domain-specific attributes shapes the turnover behavior of IT professionals; and (3) we lay a foundation for conceptualizing how social comparison leads to turnover among groups of IT professionals.
The immediate social context as an antecedent of turnover among IT professionals
This study provides evidence that the immediate social context can drive turnover behavior among IT professionals and helps to explain collective turnover behavior among IT professionals [Citation10, Citation63]. Our results suggest that IT professionals are more likely to turnover if they witness coworkers leaving the organization to take different jobs. Our application of social comparison theory identifies a new characteristic of IT turnover culture within organizations [Citation10]. Study 2 illustrates how IT professionals making social comparisons with departing coworkers magnifies the impact of the departure on perceived job options. It also shows that social comparison exerts influence over time on turnover within the social networks of IT professionals. It further reveals the persistence of these effects over time. Future research should examine the consequences of the immediate social context on group-level effects using multilevel analysis to differentiate between collective turnover at one point and the dynamic development of collective turnover that spreads through a workgroup [Citation37, Citation98].
Our results imply that the embeddedness of IT professionals may not always yield positive outcomes for organizations. While extant theory highlights the positive effects of links and the fit associated with staying in an organization [Citation58, Citation96], the logic of social comparison suggests that social embeddedness can also have negative implications regarding turnover among IT professionals. For example, our confirmatory case analysis suggests that the turnover of a single highly embedded IT professional can have a shock-like bullwhip effect through indirect links among larger social networks of IT professionals [Citation66], resulting in a chain reaction of turnover or a more persistent turnover culture [Citation10]. Exploring this negative effect of social comparison could offer novel insights into the relationship between embeddedness and turnover by helping explain the heterogeneous effects of organizational links within the social networks of IT professionals.
There is a need for future research that further integrates the immediate social context with known causes of turnover among IT professionals. For example, it would be interesting to develop an integrative threshold model of turnover among IT professionals that explores how combinations of turnover antecedents lead to the point of no return, where an IT professional’s actual turnover behavior becomes inevitable [Citation36]. Developing a threshold model based on social comparison could have implications for constructing and testing process models that are specific to the IT workforce and grounded in the general turnover literature, such as the unfolding model of voluntary turnover [Citation52, Citation66], as well as for established models of IT professional turnover [Citation37, Citation93].
Similarity as a central characteristic of the immediate social context in the IT function
We identify similarity as an important factor in understanding the effects of the immediate social context of turnover behavior among IT professionals. Two dimensions of similarity magnified the impact of the immediate social context on IT professionals’ turnover— contextual similarity in technical skills and similarity in demographic attributes. In particular, we found that if IT professionals in the immediate social context possess similar skills, they are more likely to empathize with a colleague’s decision to leave the organization. Our Study 2 illustrates that IT professionals with similar technical skills are in a position to accurately evaluate each other’s task performance, including how they deal with technical challenges, potential pitfalls, and difficulties encountered with business users and management. This has important implications for teams that collaborate closely. For example, in agile teams with formalized forms of collaboration and proximity between team members, turnover is more likely to spread through the whole team [Citation67, Citation88].
These findings are important, particularly for the management of the IT function and the IT workforce. First, IT work tasks change from predefined to uncertain during the course of IT professionals’ careers. In the early career stages, IT professionals are typically involved in more independent coding work, moving to positions responsible for complex and collaborative architectural decisions as they gain experience [Citation7, Citation55]. Such tasks are associated with high levels of uncertainty and often initially lack clarity. Thus, professionals involved with these more complex projects may be better able to observe and judge their coworkers’ performance, which gives them a basis for judging their own skills when considering turnover. This could be a plausible explanation for why similarity in terms of age did not increase the effects of the immediate social context on turnover behavior (H3b) in our analysis of the large-scale dataset. Rather than judging similarity based on biological age, our findings suggest that IT professionals likely evaluate similarity based on “professional” age- that is, the skills acquired since joining the IT field. This nuance is important because while individuals become IT professionals at different stages of their careers [Citation38], and thus external observers might see differences in biological age, our analysis suggests that research and practice may need to calibrate around professional age and IT experience rather than biological age when seeking to model the influence of similarities on IT professionals’ turnover.
Second, contextual similarity in technical skills seems to amplify social comparison. Such similarity is a natural outgrowth of collaborative IT work [Citation29, Citation54], and understanding its implications is essential for predicting IT professionals’ turnover and other workplace behaviors. Considering that task complexity and increasing interactions between business and IT require more collaboration from IT professionals [Citation48, Citation67], improving the understanding of how social comparisons shape how IT professionals make comparisons, how they select among networks of relationships, and how they influence non-IT professionals is an exciting direction for future work. For example, it would be interesting to examine whether the turnover contagion goes beyond IT professionals engaged in similar tasks [Citation40] to include non-IT peers who work closely with the IT team as part of Agile or DevOps practices [Citation33, Citation92], potentially expanding the peril posed by departing team members for the retention of workers with domain knowledge relevant to IT work.
Our results show that gender similarity predicts turnover among IT professionals. Although gender is an individual attribute that attracts much attention, we lack a rich understanding of gender’s influence in the IT workforce [Citation85]. While studies have examined discrimination against female IT professionals in terms of promotion [Citation85], there is a need to understand whether gender influences social comparison. Our results show that IT professionals of the same gender as the departing coworker are more likely to be influenced by their turnover. We argue that this factor merits further scholarly attention within the IT function because evidence suggests that homophily, as defined by demographics, could lead to turnover spreading across an organization [Citation53]. Our results could help to explain differences in women’s and men’s turnover behaviors [Citation31, Citation32] since we show that female IT professionals are affected by the turnover of other female IT professionals. Our results call for future research on the consequences and antecedents of female IT professionals leaving an organization. Future research could examine whether the interplay of gender with additional demographic faultiness in the immediate social context, such as race, religion, or country of origin, influences IT professionals’ turnover.
Our findings suggest a need for future work that explains how organizational factors shape the speed and distance with which turnover trends spread within an organization. For example, could the physical structure of work, i.e., colocated workers versus virtual collaboration, amplify or mitigate the effects of the immediate social context [Citation76]? Similarly, given that the emerging gig economy has resulted in more software developers engaging in contract work [Citation28], is a minimum number of common projects or contact hours required before the immediate social context influences turnover effects? The swift development of trust has been found to influence teams in virtual contexts [Citation35]; therefore, understanding whether time-based thresholds exist could help to explain the interplay of the immediate social context with other antecedents of turnover [Citation37, Citation98].
Limitations
This research has certain limitations. First, we used a large, archival dataset from a single IT company, which may have weakened the generalizability of our results; however, this company offers a typical case for IT work regarding consulting, software development, and maintenance. We further sampled other cases outside of ALPHA in the confirmatory case analysis to increase the robustness of the results. Second, given the size of our organizational network, we had to limit the analysis of turnover influence over time. An accuracy score smaller than the chosen 0.01 for the network analysis could have included the last, minimal turnover effects in the network. In line with extant literature, our chosen accuracy score is small enough to predict turnover contagion as an “accuracy threshold [of] Tc = 0.01, […] is extremely small and accurate beyond necessity already” [Citation97]. In addition, we set a diminishment factor of 5%, given the calculation capacity of our school’s server. We compensated this in the dynamic network analysis with a remembrance factor of 88% to achieve a lag time of 12 months for turnover to spread. While these factors are calibrated similarly to other research [Citation16, Citation20, Citation21, Citation31, Citation45, Citation63], a smaller diminishment factor would have yielded more turnover information spreading in the network. However, we ran robustness checks at 10% and 1%, yielding robust results in our network analysis.
Third, we did not analyze the underlying reasons that cause individual turnover events. Future research could examine whether turnover events with different reasons affect the immediate social context differently. Fourth, we used a variance approach to analyze the spread of turnover among IT professionals. With our confirmatory case analysis, we offered some reasons for this relationship. Nevertheless, additional qualitative work could offer insights into the process through which a departing IT professional directly or indirectly affects their team members.
Practical Contributions
Our results have several implications for practice. First, our results suggest that even one departure increases the probability of further turnover events among IT professionals. We found that a single departure may cause a chain reaction in IT work teams and organizations. This suggests that managers should intervene when an IT professional leaves to prevent a chain reaction of departures. Second, when replacing IT professionals who have left, our results suggest that IT managers should consider when and where to assign new employees. Rather than focusing on homophily, our findings suggest that firms may reap unexpected retention benefits by constructing more heterogeneous teams in terms of attributes and, if possible, with a diverse skill mix because our findings suggest that this could decrease the spread of turnover. Third, our analysis suggests that turnover may result from direct observation or the spread of gossip within the organization [Citation23]. To mitigate the impact of subjective explanations for turnover, e.g., gossip, IT managers should directly and transparently address why coworkers leave. Overall, our findings suggest that managers should take the turnover of any IT professional seriously and consider measures to increase the retention of coworkers.
Conclusion
The turnover of IT professionals poses many difficulties for organizations. Based on social comparison theory, we posit that when IT professionals feel similar—in terms of technical ability, demographic attributes, or both—to coworkers who leave the organization to take another job, they will be more likely to also leave their jobs. Our empirical results indicate that the immediate social context affects the turnover behavior of IT professionals and is amplified by contextual similarity in technical abilities and gender. This suggests that further investigation of the immediate social context could enhance the understanding of why IT professionals leave organizations.
Acknowledgements
We thank the associate editor and reviewers for their developmental feedback and guidance throughout the review process. We thank Markus Schichtl, Leonard Przybilla, Mai Nguyen, and Katharina Wahl for their data collection and analysis support. We greatly appreciate the helpful comments on earlier versions of this paper from Damien Joseph, Sven Laumer, and the brown bag seminars at the University of Mannheim and ESSEC Business School, Paris.
Disclosure statement
No potential conflict of interest was reported by the author(s).
Additional information
Funding
Notes on contributors
Manuel Wiesche
Manuel Wiesche is a Full Professor and Chair of Digital Transformation at TU Dortmund University, Germany. He holds a doctoral degree and a habilitation degree from TUM School of Management, Technical University of Munich, Germany. Dr. Wiesche’s research interests include IT workforce, IT project management, digital platform ecosystems, and IT service innovation. His research has been published in MIS Quarterly, Journal of Information Technology, Journal of the AIS, and many other journals.
Christoph Pflügler
Christoph Pflügler holds a Ph.D. from the Technical University (TUM) of Munich. He graduated in Finance and Information Management from TUM and Universität Augsburg, Germany. His main research interests include IT turnover, retention strategies, IT team composition, and governance of IT outsourcing projects.
Jason Bennett Thatcher
Jason B. Thatcher is the Tandean Rustandy Esteemed Endowed Chair in the Leeds School of Business at the University of Colorado Boulder. He is also a professor of Information Systems and Digital Transformation at the University of Manchester, UK. Dr. Thatcher received degrees from the University of Utah and Florida State University. His work appears in Information Systems Research and MIS Quarterly. He serves as Senior Editor at Information Systems Research. He has been honored by the Technical University of Munich, an Honorary Professor by the University of Nottingham-Ningbo, and as a member of the Circle of Compadres by the KPMG Ph.D. Project.
Notes
1. A pseudonym to ensure the company’s anonymity.
2. In order to test all possible empirical configurations of the age, we ran additional analyses to understand whether age brackets or dilution of effect size might exist that affect our analysis. We did not find any significant effects. These additional analyses are available upon request.
3. For the social comparison measure, we ran a face validity check by surveying a smaller set of IT professions about their social comparison behavior to close and distant colleagues. This analysis is available up-on request.
References
- Ahuja, M.K. Women in the information technology profession: A literature review, synthesis and research agenda. European Journal of Information Systems, 11, 1 (2002), 20–34.
- Ahuja, M.K., Chudoba, K.M., Kacmar, C.J., McKnight, D.H., and George, J.F. Ict road warriors: Balancing work-family conflict, job autonomy, and work overload to mitigate turnover intentions. MIS Quarterly, 31, 1 (2007), 1–17.
- Ang, S., and Slaughter, S. Turnover of information technology professionals: The effects of internal labor market strategies. ACM SIGMIS Database, 35, 3 (2004), 11–27.
- Armstrong, D., and Hardgrave, B. Understanding mindshift learning: The transition to object-oriented development. MIS Quarterly, 31, 3 (2007), 453–474.
- Armstrong, D.J., Brooks, N.G., and Riemenschneider, C.K. Exhaustion from information system career experience: Implications for turn-away intention. MIS Quarterly, 39, 3 (2015), 713–728.
- Aryee, S. Combating obsolescence: Predictors of technical updating among engineers. Journal of Engineering and Technology Management, 8, 2 (1991), 103–119.
- Balijepally, V., Mahapatra, R.K., Nerur, S.P., and Price, K. Are two heads better than one for software development? The productivity paradox of pair programming. MIS Quarterly, 33, 1 (2009), 91–118.
- Borgatti, S.P., Mehra, A., Brass, D.J., and Labianca, G. Network analysis in the social sciences. Science, 323, 5916 (2009), 892–895.
- Borgatti, S.P., and Halgin, D.S. On network theory. Organization science, 22, 5 (2011), 1168–1181.
- Burke, L.A., and Moore, J.E. Turnover culture: Toward understanding the problem of retaining technology professionals. In, Beard, J.W., (ed.), Managing impressions with information technology Praeger Publishers/Greenwood Publishing Group, 2004, pp. 55–71.
- Buunk, A.P., and Gibbons, F.X. Social comparison: The end of a theory and the emergence of a field. Organizational Behavior and Human Decision Processes, 102, 1 (2007), 3–21.
- CES ifo Group. Ifo business climate index. 2017, http://www.cesifo-group.de/ifoHome/facts/Survey-Results/Business-Climate.html.
- Chua, C.E.H., Lim, W.-K., Soh, C., and Sia, S.K. Enacting clan control in complex it projects: A social capital perspective. MIS Quarterly, 36, 2 (2012), 577–600.
- Collins, R.L. Among the better ones. In, Suls, J., and Wheeler, L., (eds.), Handbook of social comparison, New York: Kluwer Academic/Plenum, 2000, pp. 159–171.
- Cox, D.R. Regression models and life-tables. Journal of the Royal Statistical Society. Series B (Methodological), 34, 2 (1972), 187–220.
- Dasgupta, K., Singh, R., Viswanathan, B., Chakraborty, D., Mukherjea, S., Nanavati, A.A., and Joshi, A. Social ties and their relevance to churn in mobile telecom networks. Proceedings of the 11th international conference on Extending database technology: Advances in database technology, 2008, pp. 668–677.
- Dinger, M. Understanding the role of embeddedness in shaping it professional behavior. Clemson, SC: Clemson University, 2011.
- Dinger, M., Thatcher, J.B., Treadway, D., Stepina, L., and Breland, J. Does professionalism matter in the it workforce? An empirical examination of it professionals. Journal of the Association for Information Systems, 16, 4 (2015), 281–313.
- European Commission. Eurostat: Job vacancy statistics by nace rev. 2 activity - quarterly data (from 2001 onwards). Luxembourg: EUROSTAT, 2021.
- Feeley, T.H., and Barnett, G.A. Predicting employee turnover from communication networks. Human Communication Research, 23, 3 (1997), 370–387.
- Felps, W., Mitchell, T.R., Hekman, D.R., Lee, T.W., Holtom, B.C., and Harman, W.S. Turnover contagion: How coworkers’ job embeddedness and job search behaviors influence quitting. Academy of Management Journal, 52, 3 (2009), 545–561.
- Festinger, L. A theory of social comparison processes. Human Relations, 7, 2 (1954), 117–140.
- Foster, E.K. Research on gossip: Taxonomy, methods, and future directions. Review of general psychology, 8, 2 (2004), 78–99.
- Gopal, A., Sivaramakrishnan, K., Krishnan, M.S., and Mukhopadhyay, T. Contracts in offshore software development: An empirical analysis. Management Science, 49, 12 (2003), 1671–1683.
- Gorbacheva, E., Beekhuyzen, J., vom Brocke, J., and Becker, J. Directions for research on gender imbalance in the it profession. European Journal of Information Systems, 28, 1 (2019), 43–67.
- Griffeth, R.W., Hom, P.W., and Gaertner, S. A meta-analysis of antecedents and correlates of employee turnover: Update, moderator tests, and research implications for the next millennium. Journal of Management, 26, 3 (2000), 463–488.
- Gussek, L., Schned, L., and Wiesche, M. Obsolescence in it work: Causes, consequences and counter-measures. Lecture Notes in Information Systems and Organization (2021), 572–586.
- Gussek, L., and Wiesche, M. It professionals in the gig economy: The success of it freelancers on digital labor platforms. Business & Information Systems Engineering (2023), online first.
- Guzman, I.R., Stam, K.R., and Stanton, J.M. The occupational culture of is/it personnel within organizations. ACM SIGMIS Database: the DATABASE for Advances in Information Systems, 39, 1 (2008), 33–50.
- Hester, A.J., Moore, J.E., and Yager, S.E. The role of voice in retention of it workers: Paving the higher road. 2014 47th Hawaii International Conference on System Sciences: IEEE, 2014, pp. 3980–3992.
- Holtom, B.C., Mitchell, T.R., Lee, T.W., and Eberly, M.B. Turnover and retention research: A glance at the past, a closer review of the present, and a venture into the future. The Academy of Management Annals, 2, 1 (2008), 231–274.
- Hom, P.W., Lee, T.W., Shaw, J.D., and Hausknecht, J.P. One hundred years of employee turnover theory and research. Journal of Applied Psychology, 102, 3 (2017), 530.
- Huck-Fries, V., Nothaft, F., Wiesche, M., and Krcmar, H. Job satisfaction in agile information systems development: A stakeholder perspective. Information and Software Technology (2023), forthcoming.
- Jacks, T., Palvia, P., Iyer, L., Sarala, R., and Daynes, S. An ideology of it occupational culture: The aspire values. ACM SIGMIS DATABASE: The Database for Advances in Information Systems, 49, 1 (2018), 93–117.
- Jarvenpaa, S.L., and Leidner, D.E. Communication and trust in global virtual teams. Organization Science, 10, 6 (1999), 791–815.
- Josefek, J.R.A., and Kauffman, R.J. Nearing the threshold: An economics approach to pressure on information systems professionals to separate from their employer. Journal of Management Information Systems, 20, 1 (2003), 87–122.
- Joseph, D., Ng, K.-Y., Koh, C., and Ang, S. Turnover of information technology professionals: A narrative review, meta-analytic structural equation modeling, and model development. MIS Quarterly, 31, 3 (2007), 547–577.
- Joseph, D., Boh, W.F., Ang, S., and Slaughter, S.A. The career paths less (or more) traveled: A sequence analysis of it career histories, mobility patterns, and career success. MIS Quarterly, 36, 2 (2012), 427–452.
- Joseph, D., Soon, A., and Slaughter, S.A. Turnover or turnaway? Competing risks analysis of male and female it professionals’ job mobility and relative pay gap. Information Systems Research, 26, 1 (2015), 145–164.
- Joshi, K.D., and Kuhn, K.M. What it takes to succeed in information technology consulting: Exploring the gender typing of critical attributes. Information Technology & People, 20, 4 (2007), 400–424.
- Kenny, E.J., and Donnelly, R. Navigating the gender structure in information technology: How does this affect the experiences and behaviours of women? Human Relations, online first (2019), 0018726719828449.
- Kirton, G., and Robertson, M. Sustaining and advancing it careers: Women’s experiences in a uk-based it company. The Journal of Strategic Information Systems, 27, 2 (2018), 157–169.
- Kochanski, J., and Ledford, G. “How to keep me” - retaining technical professionals. Research Technology Management, 44, 3 (2001), 31–38.
- Korrigane, L. A demographic snapshot of the it workforce in europe. Communications of the ACM, 62, 4 (2019), 32–32.
- Krackhardt, D., and Porter, L.W. When friends leave: A structural analysis of the relationship between turnover and stayers’ attitudes. Administrative science quarterly (1985), 242–261.
- Krackhardt, D., and Porter, L.W. The snowball effect: Turnover embedded in communication networks. Journal of Applied Psychology, 71, 1 (1986), 50–55.
- Kruglanski, A.W., and Mayseless, O. Classic and current social comparison research: Expanding the perspective. Psychological bulletin, 108, 2 (1990), 195.
- Kudaravalli, S., Faraj, S., and Johnson, S.L. A configural approach to coordinating expertise in software development teams. MIS Quarterly, 41, 1 (2017), 43–64.
- Langer, N., and Jain, T. Peer influence and it career choice. Information Systems Research (2023), forthcoming.
- Lau, D.C., and Murnighan, J.K. Demographic diversity and faultlines: The compositional dynamics of organizational groups. Academy of Management Review, 23, 2 (1998), 325–340.
- Lee, P.C.B. The social context of turnover among information technology professionals. Proceedings of the 2002 ACM SIGCPR conference on Computer personnel research, Kristiansand, Norway: ACM, 2002, pp. 145–153.
- Lee, T.W., and Mitchell, T.R. An alternative approach: The unfolding model of voluntary employee turnover. Academy of Management Review, 19, 1 (1994), 51–89.
- Lo, J., and Riemenschneider, C. Heterogeneity of it employees: An analysis of a model of perceived organizational support by job type. ACM SIGMIS Database: the DATABASE for Advances in Information Systems, 42, 3 (2011), 71–95.
- Lounsbury, J.W., Moffitt, L., Gibson, L.W., Drost, A.W., and Stevens, M. An investigation of personality traits in relation to job and career satisfaction of information technology professionals. Journal of Information Technology, 22, 2 (2007), 174–183.
- Majchrzak, B.A., Beath, C.M., Lim, R.A., and Chin, W.W. Managing client dialogues during information systems design to facilitate client learning. MIS Quarterly, 29, 4 (2005), 653–672.
- Martinussen, T., and Scheike, T.H. Dynamic regression models for survival data. New York: Springer, 2006.
- Miles, M.B., and Huberman, A.M. Qualitative data analysis: An expanded sourcebook. London: Sage, 1994.
- Mitchell, T.R., Holtom, B.C., Lee, T.W., Sablynski, C.J., and Erez, M. Why people stay: Using job embeddedness to predict voluntary turnover. Academy of Management Journal, 44, 6 (2001), 1102–1121.
- Mobley, W.H. Intermediate linkages in the relationship between job satisfaction and employee turnover. Journal of Applied Psychology, 62, 2 (1977), 237–240.
- Moore, D.F. Applied survival analysis using r. Switzerland: Springer International Publishing, 2016.
- Morita, J.G., Lee, T.W., and Mowday, R.T. Introducing survival analysis to organizational researchers: A selected application to turnover research. Journal of Applied Psychology, 74, 2 (1989), 280–292.
- Muro, M., Liu, S., and Whiton, J. Gender bias plays out in the digital workforce, but not in every industry. Washington, DC: Brookings Institution, 2018.
- Naidoo, R. A communicative-tension model of change-induced collective voluntary turnover in it. Journal of Strategic Information Systems, 25, 4 (2016), 277–298.
- Newman, M.E. Assortative mixing in networks. Physical Review Letters, 89, 20 (2002), 1–5.
- Newman, M.E. Mixing patterns in networks. Physical Review E, 67, 2 (2003), 026126.
- Niederman, F., Sumner, M., and Maertz Jr, C.P. Testing and extending the unfolding model of voluntary turnover to it professionals. Human Resource Management, 46, 3 (2007), 331–347.
- Niederman, F., Ferratt, T.W., and Trauth, E.M. On the co-evolution of information technology and information systems personnel. ACM SIGMIS Database: the DATABASE for Advances in Information Systems, 47, 1 (2016), 29–50.
- Pee, L.G., Kankanhalli, A., Tan, G.W., and Tham, G. Mitigating the impact of member turnover in information systems development projects. IEEE Transactions on Engineering Management, 61, 4 (2014), 702–716.
- Petter, S., DeLone, W., and McLean, E.R. Information systems success: The quest for the independent variables. Journal of management information systems, 29, 4 (2013), 7–62.
- Pflügler, C., Wiesche, M., Becker, N., and Krcmar, H. Strategies for retaining key it professionals. MIS Quarterly Executive, 17, 4 (2018).
- Ply, J.K., Moore, J.E., Williams, C.K., and Thatcher, J.B. Is employee attitude and perceptions at varying levels of software process maturity. MIS Quarterly, 36, 2 (2012), 601–624.
- Poole, M.S., Van de Ven, A.H., Dooley, K., and Holmes, M.E. Organizational change and innovation processes: Theory and methods for research. Oxford University Press, 2000.
- Quesenberry, J.L., and Trauth, E.M. The (dis) placement of women in the it workforce: An investigation of individual career values and organisational interventions. Information Systems Journal, 22, 6 (2012), 457–473.
- Reid, M.F., Allen, M.W., Armstrong, D.J., and Riemenschneider, C.K. Perspectives on challenges facing women in is: The cognitive gender gap. European Journal of Information Systems, 19, 5 (2010), 526–539.
- Robillard, P.N. The role of knowledge in software development. Communications of the ACM, 42, 1 (1999), 87–92.
- Sarker, S., and Sahay, S. Understanding virtual team development: An interpretive study. Journal of the Association for Information Systems, 4, 1 (2003).
- Scott, A., Klein, F.K., and Onovakpuri, U. Tech leavers study - a first-of-its-kind analysis why people voluntarily left jobs in tech. The Kapor Center for Social Impact 2017.
- Statistisches Bundesamt. Unemployment. 2017, https://www.destatis.de/EN/FactsFigures/NationalEconomyEnvironment/LabourMarket/Unemployment/Unemployment.html.
- Steel, R.P. Turnover theory at the empirical interface: Problems of fit and function. Academy of Management Review, 27, 3 (2002), 346–360.
- Suls, J., and Wheeler, L. A selective history of classic and neo-social comparison theory. Handbook of social comparison: Springer, 2000, pp. 3–19.
- Suls, J., Martin, R., and Wheeler, L. Social comparison: Why, with whom, and with what effect? Current directions in psychological science, 11, 5 (2002), 159–163.
- Sumner, M., and Niederman, F. The impact of gender differences on job satisfaction, job turnover, and career experiences of information systems professionals. Journal of Computer Information Systems, 44, 2 (2003), 29–39.
- Thatcher, J.B., Stepina, L.P., and Boyle, R.J. Turnover of information technology workers: Examining empirically the influence of attitudes, job characteristics, and external markets. Journal of Management Information Systems, 19, 3 (2002), 231–261.
- Thibodeau, P. It jobs will grow 22% through 2020, says u.S. 2012, http://www.computerworld.com/article/2502348/it-management/it-jobs-will-grow-22–through-2020–says-u-s-.html.
- Trauth, E.M., Quesenberry, J.L., and Huang, H. Retaining women in the us it workforce: Theorizing the influence of organizational factors. European Journal of Information Systems, 18, 5 (2009), 476–497.
- Tripp, J.F., Riemenschneider, C., and Thatcher, J.B. Job satisfaction in agile development teams: Agile development as work redesign. Journal of the Association for Information Systems, 17, 4 (2016), 267.
- Venkatesh, V., Brown, S.A., and Bala, H. Bridging the qualitative–quantitative divide: Guidelines for conducting mixed methods research in information systems. MIS Quarterly (2013).
- Vidgen, R., and Wang, X. Coevolving systems and the organization of agile software development. Information Systems Research, 20, 3 (2009), 355–376.
- Wang, X.W., Yang, X., Teo, H.-H., and Tong, Y. It employees’ organizational identification: Examining its antecedents and impact on turnover. Thirty First International Conference on Information Systems, St. Louis, 2010, pp. paper 252.
- Wasserman, S., and Faust, K. Social network analysis: Methods and applications. Cambridge university press, 1994.
- Wheeler, L., Martin, R., and Suls, J. The proxy model of social comparison for self-assessment of ability. Personality and Social Psychology Review, 1, 1 (1997), 54–61.
- Wiedemann, A., Wiesche, M., Gewald, H., and Krcmar, H. Understanding how devops aligns development and operations: A tripartite model of intra-it alignment. European Journal of Information Systems, 29, 5 (2020), 458–473.
- Wiesche, M., Joseph, D., Thatcher, J., Gu, B., and Krcmar, H. It workforce. MIS Quarterly Research Curation (2019).
- Yin, R. Case study research: Design and methods. Thousand Oaks, CA: Sage, 2009.
- Young-Hyman, T. Cooperating without co-laboring: How formal organizational power moderates cross-functional interaction in project teams. Administrative Science Quarterly, 62, 1 (2017), 179–214.
- Zhang, X., Ryan, S.D., Prybutok, V.R., and Kappelman, L. Perceived obsolescence, organizational embeddedness, and turnover of it workers: An empirical study. ACM SIGMIS Database: the DATABASE for Advances in Information Systems, 43, 4 (2012), 12–32.
- Ziegler, C.-N., and Golbeck, J. Models for trust inference in social networks. In, Król, D., Fay, D., and Gabryś, B., (eds.), Propagation phenomena in real world networks. Intelligent systems reference library, Cham: Springer, 2015, pp. 53–89.
- Zylka, M.P., and Fischbach, K. Turning the spotlight on the consequences of individual it turnover: A literature review and research agenda. ACM SIGMIS Database: the DATABASE for Advances in Information Systems, 48, 2 (2017), 52–78.