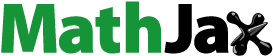
Abstract
Objective: The nasal cavity effectively captures the particles present in inhaled air, thereby preventing harmful and toxic pollutants from reaching the lungs. This filtering ability of the nasal cavity can be effectively utilized for targeted nasal drug delivery applications. This study aims to understand the particle deposition patterns in three age groups: neonate, infant, and adult.
Materials and methods: The CT scans are built using MIMICS 21.0, followed by CATIA V6 to generate a patient-specific airway model. Fluid flow is simulated using ANSYS FLUENT 2021 R2. Spherical monodisperse microparticles ranging from 2 to 60 µm and a density of 1100 kg/m3 are simulated at steady-state and sedentary inspiration conditions.
Results: The highest nasal valve depositions for the neonate are 25% for 20 µm, for infants, 10% for 50 µm, 15% for adults, and 15% for 15 µm. At mid nasal region, deposition of 15% for 20 µm is observed for infant and 8% for neonate and adult nasal cavities at a particle size of 10 and 20 µm, respectively. The highest particle deposition at the olfactory region is about 2.7% for the adult nasal cavity for 20 µm, and it is <1% for neonate and infant nasal cavities.
Discussion and conclusions: The study of preferred nasal depositions during natural sedentary breathing conditions is utilized to determine the size that allows medication particles to be targeted to specific nose regions.
Introduction
The human nasal cavity goes through a series of anatomical modifications from birth, through childhood up to adulthood due to which the airflow patterns in the nasal cavity undergo a considerable amount of changes. Studies have shown that treating children as small adults for nasal flows is not a desirable assumption, whereby extrapolation is done by simply scaling down the adult nasal cavity model (Di Cicco et al. Citation2021). From the nostrils to the lungs, the inhaled air goes through different cross-sectional areas. Though the human nasal airways are similar, it has been observed that the airflow is affected by the geometrical features of the airway cavity (Zhu et al. Citation2011; Zhao and Jiang Citation2014). Airflows in the nasal cavity are largely influenced by conditions like nasal septum, nasal inflammation, and polyps which also may have a larger influence on the flow of air in the nasal cavity (Wolfensberger Citation2002). A major physiological function of the nasal cavity includes olfaction, which provides a sense of smell that primarily depends on the airflow patterns inside the nasal cavity.
Some of the main problems associated with normal respiration are the inflammation caused by allergic rhinitis which is caused by allergens like pollen, dust, and flakes of the skin of some animals (Platts-Mills Citation1998; Dykewicz and Hamilos Citation2010; Richter Citation2011). Allergic rhinitis is believed to affect around one-third of people in Western countries (Dykewicz and Hamilos Citation2010). The diameters of these allergens range from 2 to 60 µm. The pollens from flowers range up to 60 µm, the domestic cat allergens are smaller than 5 µm, and the dust particles are about 50 µm in diameter (Luczynska et al. Citation1990; Salvaggio Citation1994; Jeong Citation2016). These allergens that enter the nasal cavity during inspiration get deposited in different regions and their deposition patterns depend on the particle size, breathing rate, diameter, and density of the inhaled particles.
With the use of high-end computing systems, computational fluid dynamics (CFD) has proved to be an exemplary tool for nasal airflow simulations and particle deposition studies (Kelly et al. Citation2000; Zamankhan et al. Citation2006; Zubair, Riazuddin, Abdullah, Ismail, et al. Citation2013; Zubair, Riazuddin, Abdullah, Rushdan, et al. Citation2013; Inthavong et al. Citation2019; Zuber et al. Citation2020; Salati et al. Citation2021; Valerian Corda, Emmanuel, et al. Citation2023; Valerian Corda, Satish Shenoy, et al. Citation2023). CFD simulates the airflow within the passage and evaluates the flow characteristics. It is of prime importance to understand that the nasal cavities of neonates and infants are different from those of adults. Neonates have an underdeveloped nasal cavity whereas in infants the nasal cavity is at developing stages and adults however have a fully developed nasal cavity (Xi et al. Citation2012). This implies that the particle deposition patterns are different for these three age groups. In addition to the deposition pattern of particles, the flow rate and profile also play a vital role. Alterations in air velocity and turbulence levels can occur with different flow rates, thereby impacting the forces acting on particles and modifying their trajectories. Moreover, the behavior of particles can be influenced by the flow profile, which denotes the variation in air velocity across the airway’s cross-section (Stuart Citation1984).
In our recent study, the airflow patterns in subjects of three different age groups neonate, infant, and adult were compared (Corda et al. Citation2022). This study showed that for neonates that have underdeveloped meatus, the major airflow is diverted to the middle and superior meatus. Studies by Xi et al. (Citation2012) also indicated underdeveloped inferior meatus in neonates. The nasal fossae increase in width with age from birth to adulthood (Contencin et al. Citation1999). For neonates and infants, the nasal cavity is soft and distensible compared to adults attributed to a larger mucosa and lymphoid tissue (Dickison Citation1987).
Particulate matter <10 µm in diameter, also known as PM10, is inhalable and is a major cause of respiratory diseases (Kyung and Jeong Citation2020). Bioaerosols are biological particles in the air. Plant-based ones are pollens, endospores, and leaf fragments. Animal-based ones are small hairs, feathers, and body fluid droplets (Douwes et al. Citation2003; Nasir et al. Citation2012). Other factors that release particulate matter into the atmosphere include agricultural and bush fires, heating and cooking at home, vehicle exhaust, and manufacturing processes (Chen et al. Citation2017). Studies have shown that household molds and mold spores that are present in an indoor environment cause upper airway infection in infants during the first year (Mercer et al. Citation2002; Belanger et al. Citation2003; Biagini et al. Citation2006). Household mold is reported to be a major cause of non-allergic rhinitis in 3-month-old infants (Stazi et al. Citation2002). This necessitates the requirement to study the nasal deposition patterns in neonates and infants which adds value to this study as the adult subjects are widely studied by many researchers. In this work, the nasal particle deposition patterns for three age groups namely the neonate, infant, and adult are compared. Additionally, the regional nasal microparticle depositions are examined.
Materials and methods
Nasal computational models
The construction of the nasal airway was carried out by obtaining the computed tomography (CT) scans from the repository of the Department of Radio Diagnosis and Imaging, Kasturba Medical College Manipal, India. The CT scans for the neonate (10 days) and infant (11 months) were obtained for the reasons of vomiting, lethargy, and seizures whereas the adult (27 years) had complaints of nasal anosmia for which CT scans were taken. CT scans were obtained when the subject was in the supine position and there were no complaints of breathing difficulties reported. The procured CT scans had a slice increment of 0.6, 0.45, and 0.45 mm for neonate infant and adult cavities. The pixel resolution was 512 × 512 for all the three nasal cavities. The slice count selected for developing the 3D model was 265 for neonates, 320 for infants, and 278 for the adult nasal cavity. The Institutional Ethical Committee clearance is obtained for this study and accordingly, the procured CT scans are anonymized. With the supervision of an ear, nose, and throat (ENT) and Radiology specialist, the boundary differentiating between the air and airway mucosa is identified for each of the slices. The sinuses are not considered in this study as researchers have shown that for healthy subjects the airflow and depositions in sinuses are negligible (Bahmanzadeh et al. Citation2015). The slice increment which is below 2 mm is conducive to accurately selecting the nasal airspace (Bailie et al. Citation2006). Segmentation of the CT scans is accomplished by using MIMICS 21.0 (Materialise, Ann Arbor, MI, USA) which is an image processing software. To accurately segment the complicated nasal airway a threshold value of −1024 to −444 HU (Hounsfield Unit) is used which follows the work of previous researchers (Cherobin et al. Citation2018). A 3D model of the segmented slices of the nasal cavity can be displayed in MIMICS 21.0 which is checked for anatomic integrity and is again processed using 3-MATIC 13.0 (Materialise, Ann Arbor, MI, USA). Further, CATIA V6 is used to generate a surface model of the nasal cavity. Each step of the segmentation process is witnessed by an expert ENT specialist so that the nasal cavity model is accurately captured.
indicates some selected scans of coronal and sagittal cross-sections and a 3D model that will be used for airflow simulations.
Flow field governing equations
The continuity and momentum equations as shown in EquationEquations (1)(1)
(1) and Equation(2)
(2)
(2) govern the airflow in the nasal cavity. These equations are mathematical forms of mass and momentum conservations, respectively. The energy equation is not accounted for in this study as temperature effects are not accounted for in this study.
(1)
(1)
(2)
(2)
Where ‘u’ represents air velocity in m/s, ‘ρ’ represents the fluid density in kg/m3 and ‘’ indicates the kinematic viscosity in Pa·s, and ‘p’ denotes pressure in Pa.
The airflow simulations in the nasal cavity are performed in steady-state for a range of breathing conditions with the variable mass flow at the nostrils. For adult nasal airflow simulations, a mass flow rate below 15 LPM is laminar in nature which corresponds to low Reynolds number flows typically below 2000. Beyond this value of 15 LPM, the fluid flow is turbulent in nature due to Reynolds numbers over 2000 (Zamankhan et al. Citation2006; Abouali et al. Citation2012; Farhadi Ghalati et al. Citation2012; Salati et al. Citation2021). The inhalation flow rates (Qin) are calculated as twice the breathing frequency (f) and the tidal volume (Vt) (Hofmann Citation1982; Deng et al. Citation2018).
(3)
(3)
The present study employs an inhalation flow rate of 1.84 LPM for the neonate, 3.6 LPM for the infant, and 14 LPM for the adult nasal cavity (Hofmann Citation1982). Particle movement and deposition in the nasal cavity are modeled using the Lagrangian trajectory methodology. This study employs a one-way interaction between the particles and the fluid phase which essentially means the moving particles do not affect the flowing fluid. Initially, the equations of flow are solved for the velocity in the computational domain following which the particle trajectory is analyzed. The equation for particle velocity using Lagrangian particle tracking methodology in the cartesian coordinate system is written as
(4)
(4)
In the above equation represents drag force per unit mass,
represents the gravitational acceleration per unit mass,
represents the Brownian forces, and
represents additional acceleration terms (e.g. Saffman lift force). As this study focuses only on the microparticles the Brownian motion and Saffman’s lift forces are not considered in this simulation (Ounis et al. Citation1991).
(5)
(5)
(6)
(6)
In the above equations ‘u’ and ‘’ represent the fluid and particle velocity, respectively, ‘μ’ is the dynamic viscosity, ‘
’ and ‘
’ are fluid and particle density, respectively,
is the particle diameter and
is the relative Reynolds number.
is the drag coefficient which the software utilizes from the works of Morsi and Alexander (Citation1972).
An ensemble of 35,000 particles ranging from 2 to 60 µm is introduced from the nostril inlets into the nasal cavity. This number of 35,000 microparticles is considered based on the particle independence test procedure performed where an increase of particles above 35,000 resulted in a variation of <1% for the depositions. Submicron or nanoparticles have negligible inertia due to which the Eulerian diffusion theory is used to compute their transport and deposition. The particle tracking is carried out using an uncoupled discrete phase method. Depending on the particle size and airflow patterns, microparticles released from the nostril inlet surface are deposited in different parts of the nasal cavity. The deposition efficiency (DE) is computed by taking a ratio of the particles deposited at a particular region in the nasal cavity to the particles injected from the nostril inlets.
Meshing, boundary condition, and simulation setup
Airflow simulation in the nasal cavity is performed using ANSYS FLUENT 2021 R2. This includes the meshing of the imported nasal cavity in a. STP file format. Meshing is achieved by using the polyhedral meshing technique in the FLUENT meshing component system. Compared to conventional meshing techniques, the polyhedral meshing method is more accurate, uses fewer cells by 3.5 times, and converges more quickly (Bass et al. Citation2019; Brüning et al. Citation2020). The skewness is limited to a maximum value of 0.7. The meshing is done in different levels of mesh densities for neonate (3.96 million, 5.22 million, and 6.103 million), infant (1.84 million, 4.84 million, and 6.47 million), and adult (2.14 million, 5.99 million, and 7.84 million) nasal cavities. The sample meshing figure is shown in for an adult nasal cavity.
The velocity contours across the nasal cavity are plotted for three different mesh densities to perform the mesh independence by plotting peak velocity at the nasal valve region. shows the mesh dependency test results for the three simulation models used in this study. The final mesh selected comprises 5.22 million cells for the neonate, 4.84 million for the infant, and 5.99 million cells for the adult nasal cavity since the velocity values stabilized after these values.
Regional divisions for evaluating particle deposition patterns in the nasal cavity are presented in .
This study uses boundary conditions in line with the work carried out by previous researchers (Wen et al. Citation2008; Zubair et al. Citation2015). The mass flow rate corresponding to sedentary inhalation is utilized as an inward mass flow rate at the nostrils. In this work, we consider the inlet flow rates at the nostril inlets. The flow rates are 1.84 LPM for neonates, 3.6 LPM for infants, and 14 LPM for the adult nasal cavity. At the nasopharynx which is the outlet, the outflow boundary condition is set which assumes a fully developed flow (Lim et al. Citation2021). The nasal wall is considered rigid and with no slip between the wall and the flowing fluid and the effect of mucous is not considered (Wang et al. Citation2012). The air, which is the fluid considered for this simulation is assumed incompressible in nature with a density and dynamic viscosity of 1.225 kg/m3 and 1.7894 × 10−5 kg/(ms), respectively (Cal et al. Citation2017; Yarragudi et al. Citation2020). For the particle phase, the inlet velocity is considered to be the same as the inhalation velocity. The inhaled particle is assumed to be monodispersed and considered an aerodynamic diameter meaning the density of 1100 kg/m3 (Yarragudi et al. Citation2020; Islam et al. Citation2021). The particles in spherical shape are injected with an ‘escape’ condition defined for the inlet nostrils and the outlet nasopharynx, allowing them to escape without any reflections when the boundary in question is encountered. In the case of the nasal airway walls, a ‘trap’ condition is implemented, which means that the particles adhere to the walls upon contact without bouncing back and thereby the trajectory calculations are terminated. These particles are injected at the same velocity as the inspired air.
The EquationEquations (1)(1)
(1) and Equation(2)
(2)
(2) that govern the fluid flow are discretized using the control volume method. Based on the generated computational mesh, the domain is discretized into control volumes. The governing equations are converted to integral forms so that the integration of each of the equations in the computational mesh can be done. This is followed by setting up and solving a set of algebraic equations of velocity and pressure which are the dependent variables. Solving the governing equations is done by using a pressure-based solver in ANSYS FLUENT 2021 R2, which is applicable for incompressible flows at low speeds. This method gives velocity by solving the momentum equation and the pressure field is obtained by solving for the required pressure. Further, the SIMPLE algorithm is used which relates velocity and pressure corrections to the enforcement of mass conservation and thereby arriving at the required pressure. Since this is a problem involving iterative numerical methods to obtain a solution, the residuals which are the direct quantification of errors in a set of equations, indicate the convergence. Residuals measure the local imbalance of the conserved variables, and this value of residuals though cannot be reduced down to exactly zero in numerical methods, a value as less as possible is desirable as it indicates a more accurate solution. Airflow field simulations were executed by considering non-dimensional residual errors smaller than 10−4.
Numerical model validation
Cross-sectional area comparison
The cross-sectional areas along the length of the nasal cavity from the nostrils to the nasopharynx are plotted in which are compared with the values in literature (Xi et al. Citation2012). The nasal valve region is the most constricted in the flow domain, resulting in the highest velocity which will be discussed in subsequent sections.
Figure 5. Cross-sectional area comparison with the literature (Xi et al. Citation2012).

The cross-sectional area along the nasal computational domain indicates the neonatal and adult nasal cavities match closely in terms of areas, but there is a difference observed in the cross-sectional areas of the infant nasal cavity. This may be primarily due to the inter anatomic variations and secondly due to their age as there is a 4-month difference between them.
Validating with deposition efficiency
The current study’s deposition efficiency is compared to experimental data and numerical CFD simulations to validate the numerical model (Kelly et al. Citation2004a; Inthavong et al. Citation2011; Schroeter et al. Citation2011; Hsu and Chuang Citation2012; Shang et al. Citation2015). The impaction parameter which is the product of the square of particle diameter (dp-µm2) and the inhalation flow rate (Q-cm3/s) is used to validate this study for the adult nasal cavity.
Microparticles ranging from 2 to 60 microns were evaluated in this study. shows the plot of deposition efficiency values against the impaction parameter for the adult nasal cavity and presents the same for the neonate and infant nasal cavities.
Figure 6. Validation of deposition efficiency (DE %) vs. impaction parameter (IP) for microparticles from 2 to 60 µm in an adult nasal cavity.

Figure 7. Validation of deposition efficiency (DE %) vs. impaction parameter (IP) for microparticles from 2 to 60 µm in (a) neonate and (b) infant nasal cavity.

From and , we can conclude that our predicted value for particle deposition is in close agreement with the values available in the literature. Based on the data presented in the graphs, it appears that there is a noticeable resemblance to the literature values. Nevertheless, it is worth noting that discrepancies may arise due to potential differences in the anatomical structure of the nasal cavities as seen in the neonatal and infant nasal cavity.
Results
Flow parameters
In this section, the airflow parameters in the nasal cavity which include the velocity profiles, pressure distribution, and wall shear stress will be addressed. represents the velocity patterns for the three age groups along the nasal cavity. Additionally, also depicts the lateral view of the velocity profiles which also shows that the nasal valve region experiences the highest velocity. There is an accelerated flow in the nasal valve region as the inspired air marches into the mid-nasal region. The angle by which the air changes its direction in the nasal valve region is about 60–70° and there is a reduction in the velocity of inspired air in the mid-nasal region. There is a gradual reduction in the velocity as the air enters the nasopharyngeal region and then into the lungs.
The maximum velocity plotted along the different regions of the nasal cavity is shown in which shows the plots of maximum velocity and the normalized maximum velocity.
Figure 9. Velocity plots along the nasal cavity (a) maximum velocity and (b) normalized maximum velocity.

From it can be seen that the velocity at the adult nasal cavity is about 1.7 times and 2.3 times higher when compared to the neonatal and infant nasal cavity. The normalized velocity plot is to indicate the maximum velocity occurring at the nasal valve region as stated previously.
represents the variation of wall shear stress (WSS) along the nasal cavity. When air in motion comes into contact with the nasal walls, it generates a force due to friction known as wall shear stress. This interaction plays a role in facilitating the exchange of heat and mass between the air and the mucosal lining (Bailie et al. Citation2009). Wall shear stress patterns indicate higher values in the mid-nasal region and decrease along the length of the nasal cavity. Higher values of WSS could result in nasal irritation and uneasiness in breathing.
Total particle deposition comparison between three age groups
The particle deposition for three subjects in the age groups the neonate, infant, and adult nasal cavities is shown in . The total deposition efficiency indicates the particles that get deposited in the nasal cavity and the remaining particles tend to escape through the nasopharynx and then into the lungs. Overall deposition trends show that for neonates and adults particles over 20 µm and for infants particles over 30 µm deposit with nearly 100% efficiency in the nasal cavity.
Regional particle deposition comparison
The particle deposition patterns at different regions of the nasal cavity computational domain are shown in . The vestibule is the first region in the nasal cavity that incorporates the nostrils and also plays a major role in the deposition of the majority of inhaled particles. The vestibular deposition patterns show that the adult nasal vestibule has the highest particle deposition followed by the infant and the neonatal vestibule. The deposition efficiency plot shows the peak depositions at higher particle diameters. The nasal valve region is one of the most constricted regions in the nasal cavity. The nasal valve region is of prime importance because, in this region, the inhaled air takes a nearly 90° turn into the mid-nasal region. also indicates the deposition patterns in the nasal valve region showing maximum deposition in the neonatal airways. Peak deposition of 25% is observed in neonatal airways for 20 µm, whereas the infant peak deposition is around 10% for 50 µm. For the adult nasal cavity, the peak deposition of 15% was observed for 15 µm after which the deposition reduced with increasing particle diameter for the nasal valve region.
Figure 12. Regional particle deposition (a) vestibule, (b) nasal valve, (c) mid nasal region, (d) olfactory, (e) anterior, and (f) nasopharynx region.

The mid-nasal region accommodates most of the middle and inferior turbinates. The infant nasal cavity shows the highest depositions in the mid-nasal region which is 15% for 20 µm, whereas the neonatal and adult nasal cavities show around 8% depositions at 10 and 20 µm particle sizes, respectively in the mid-nasal region.
Anterior regions of the middle and inferior turbinates are enclosed by the anterior turbinates. The deposition patterns at the anterior region show a peak deposition of 17 and 12% at 15 µm for the neonate and adult nasal cavities. However, the infant nasal cavity shows a peak deposition of 9% at 30 µm particle size. In general, the particle deposition patterns show an increasing trend up to a particular particle size after which the deposition percentages decrease.
The olfactory area is found in the nasal cavity’s dorsal posterior portion. Studies on drug delivery applications have focused on the olfactory region as a means of delivering medications to the central nervous system (Illum Citation2000). The nasopharynx region starts where the turbinates end at the posterior region and where the two sides of the nasal cavity passages join. The highest particle deposition at the olfactory region is about 2.7% for the adult nasal cavity for 20 µm which then decreases as the particle size increases. In the nasopharynx region, the neonatal nasal cavity experiences a maximum particle deposition of 6.5% for a 10 µm particle size. Sniffing directs air toward the olfactory region, possibly improving particle deposition. Nasal cycling significantly affects air flow rate and impaction.
Particle deposition comparison based on size
Based on the size of inhaled microparticles the particle deposition patterns at different locations in the nasal cavity are shown in (2, 10, 14, and 20 µm) and (30, 40, 50, and 60 µm). It can be seen from that the smallest particle of this study which is the 2 µm shows the highest deposition in the neonatal nasopharynx, adult, and infant vestibule. It can also be noted that the olfactory region deposits the least among all the areas. The 10 µm particle deposition trends show higher depositions in the nasal vestibular region when compared to 2 µm particles. The adult cavity deposits are highest followed by the neonate and infant. The infant mid-nasal region deposits the highest particles when compared to the others since the infants do not have inferior turbinates, most of the flow is directed through the mid-nasal region.
The deposition trends of 14 and 20 µm particles are almost the same across the nasal cavities with the highest deposition at the nasal vestibule, followed by the nasal valve and the anterior regions.
For higher particle sizes that range from 30 to 60 µm, it can be seen that the majority of particles deposited are in the nasal vestibule region followed by a small deposition in the nasal valve region. Another conclusion that can be drawn is that the vestibular region of the adult nasal cavity traps the highest particles followed by that of infants and neonates.
Discussions
This study is carried out to understand the particle deposition patterns in human nasal cavities of three age groups under sedentary breathing conditions. The particle diameters considered in this study ranged from 2 to 60 µm having a density of 1100 kg/m3. The smaller particles of 2 µm largely get deposited in the nasopharyngeal region and as the particle size increases the depositions shift toward the nasal valve and nasal vestibule region. The olfactory region shows negligible deposition for all three age groups when compared to other regions.
The regional depositions for 14 and 20 µm particles show lower depositions in the nasopharyngeal region and the deposition decreases as the age group increase. The nasal valve depositions decrease as the age for particles is 50 and 60 µm. The adult nasal depositions at the nasal valve and olfactory regions were compared with Schroeter et al. (Citation2015) indicate a difference of about 10% in numerical simulations and 5% in experimental calculations which may be attributed to the larger surface areas of the nasal valve region considered. However, the peak deposition and overall trend are similar. The olfactory region comparison shows a difference of around 5% for Schroeter et al. (Citation2015) and 4% for Yarragudi et al. (Citation2020) can also be attributed to the surface area considered. The difference between the experimental of Schroeter et al. (Citation2015) and the numerical value in this study is 3% for olfactory regional deposition.
Particle depositions in the nasal cavity are greatly influenced by the shape of the nasal geometry (Kelly et al. Citation2004a, Citation2004b). The results of the simulations can be a starting point for particle deposition studies using a nebulizer or particle deposition using a breathing assisting device. Micro-sized particles are predominantly influenced by the inertial impaction mechanism which is affected by the smoothness of the model as proved by some researchers based on the surface roughness of the casts used (Kelly et al. Citation2004a). The choice of CFD simulation models can impact both the total and localized deposition of particles, influenced by the surface quality of the employed models (Schroeter et al. Citation2011). The nasal valve region is the most constricted area in the nasal computational domain leading to a peak velocity (Van Strien et al. Citation2021). It was also found that the peak velocity at the nasal valve for the adult nasal cavity was higher by 40 and 55% when compared to the neonate and infant, respectively.
This study has some limitations. Firstly only inhalation with one breathing condition is considered for this study which is the light breathing condition that corresponds to the laminar flow of air in the nasal cavity. Future studies will be carried out for a range of inhalation flow rates spanning from laminar to turbulent flows in addition to cyclic breathing conditions. The future scope is to carry out particle deposition studies in transient conditions that are more realistic rather than in steady-state conditions, which have been carried out in this work. This approach will provide more accurate and applicable results. The study conducted assumes the particles to be monodisperse in nature and future studies in this regard shall include polydisperse particles to identify the particle depositions in the nasal cavity. The effect of the mucus layer and the thermal effects are not considered in this simulation and will be considered in the future scope of the study.
Conclusions
The use of computational methods has facilitated the understanding of the regional deposition patterns in three age-grouped nasal cavities for a range of particle sizes and at variable densities. The simulations were carried out in steady-state inhalation conditions to get an awareness of the particle deposition efficiencies in different regions of the nasal cavity which can also be extrapolated to study the drug delivery applications from the nostrils. The nasal valve and nasal vestibule play a major role in deposition and filtering out the inhaled particles, particularly >10 µm, which impacts the deposition in other posterior regions of the nasal cavity. Regional depositions in three subjects, one each of neonate, infant, and adult are considered in the study. Finally, the conclusions that can be drawn from this study are given below.
The nasal vestibule traps most of the inhaled particles.
The neonatal nasal valve region traps higher particles than the infant and adult.
The anterior regional depositions are highest for the neonatal nasal cavity following which the depositions decrease.
The olfactory region has the lowest regional deposition efficiency as only a small portion of inhaled air reaches this region. The adult nasal cavity experiences the highest deposition.
Ethical approval
The approval of the Institutional Ethics Committee (IEC 416/2020) for this study is obtained from Kasturba Medical College Manipal and all data is anonymized.
Author contributions
John Valerian Corda: conceptualization of the project, developing methodology and modeling of the work, analysis and processing data, manuscript writing, reviewing, and editing. B. Satish Shenoy: supervision of project, manuscript writing, reviewing, and editing. Kamarul Arifin Ahmad: developing methodology and modeling of the work. Leslie Lewis: identifying CT scans, checking for patent nasal cavities, analysis, and processing data. Prakashini K: identifying CT scans, and checking for nasal cavities. Anoop Rao: developing methodology and supervision of the project. Mohammad Zuber: conceptualization of the project, supervision of the project, developing methodology, and modeling of the work.
Acknowledgments
We thank the Department of Aeronautical and Automobile Engineering, Manipal Institute of Technology, Manipal Academy, Manipal for the computing resources provided to carry out this work.
Disclosure statement
No potential conflict of interest was reported by the author(s).
Data availability statement
All the data relevant to this study is given in this article.
Additional information
Funding
References
- Abouali O, Keshavarzian E, Farhadi Ghalati P, Faramarzi A, Ahmadi G, Bagheri MH. 2012. Micro and nanoparticle deposition in human nasal passage pre and post virtual maxillary sinus endoscopic surgery. Respir Physiol Neurobiol. 181(3):335–345. doi: 10.1016/j.resp.2012.03.002.
- Bahmanzadeh H, Abouali O, Faramarzi M, Ahmadi G. 2015. Numerical simulation of airflow and micro-particle deposition in human nasal airway pre- and post-virtual sphenoidotomy surgery. Comput Biol Med. 61:8–18. doi: 10.1016/j.compbiomed.2015.03.015.
- Bailie N, Hanna B, Watterson J, Gallagher G. 2006. An overview of numerical modelling of nasal airflow. Rhinology. 44(1):53–57.
- Bailie N, Hanna B, Watterson J, Gallagher G. 2009. A model of airflow in the nasal cavities: implications for nasal air conditioning and epistaxis. Am J Rhinol Allergy. 23(3):244–249. doi: 10.2500/ajra.2009.23.3308.
- Bass K, Boc S, Hindle M, Dodson K, Longest W. 2019. High-efficiency nose-to-lung aerosol delivery in an infant: development of a validated computational fluid dynamics method. J Aerosol Med Pulm Drug Deliv. 32(3):132–148. doi: 10.1089/jamp.2018.1490.
- Belanger K, Beckett W, Triche E, Bracken MB, Holford T, Ren P, McSharry J, Gold DR, Platts-Mills TAE, Leaderer BP. 2003. Symptoms of wheeze and persistent cough in the first year of life: associations with indoor allergens, air contaminants, and maternal history of asthma. Am J Epidemiol. 158(3):195–202. doi: 10.1093/aje/kwg148.
- Biagini JM, LeMasters GK, Ryan PH, Levin L, Reponen T, Bernstein DI, Villareal M, Khurana Hershey GK, Burkle J, Lockey J. 2006. Environmental risk factors of rhinitis in early infancy. Pediatr Allergy Immunol. 17(4):278–284. doi: 10.1111/j.1399-3038.2006.00386.x.
- Brüning J, Hildebrandt T, Heppt W, Schmidt N, Lamecker H, Szengel A, Amiridze N, Ramm H, Bindernagel M, Zachow S, et al. 2020. Characterization of the airflow within an average geometry of the healthy human nasal cavity. Sci Rep. 10(1):3755. doi: 10.1038/s41598-020-60755-3.
- Cal IR, Cercos-Pita JL, Duque D. 2017. The incompressibility assumption in computational simulations of nasal airflow. Comput Methods Biomech Biomed Eng. 20(8):853–868. doi: 10.1080/10255842.2017.1307343.
- Chen J, Li C, Ristovski Z, Milic A, Gu Y, Islam MS, Wang S, Hao J, Zhang H, He C, et al. 2017. A review of biomass burning: emissions and impacts on air quality, health and climate in China. Sci Total Environ. 579:1000–1034. doi: 10.1016/j.scitotenv.2016.11.025.
- Cherobin GB, Voegels RL, Gebrim EMMS, Garcia GJM. 2018. Sensitivity of nasal airflow variables computed via computational fluid dynamics to the computed tomography segmentation threshold. PLOS One. 13(11):e0207178. doi: 10.1371/journal.pone.0207178.
- Contencin P, Gumpert L, Sleiman J, Possel L, De Gaudemar I, Adamsbaum C. 1999. Nasal fossae dimensions in the neonate and young infant: a computed tomographic scan study. Arch Otolaryngol Head Neck Surg. 125(7):777–781. doi: 10.1001/archotol.125.7.777.
- Corda JV, Shenoy BS, Ahmad KA, Lewis L, K P, Khader SMA, Zuber M. 2022. Nasal airflow comparison in neonates, infant and adult nasal cavities using computational fluid dynamics. Comput Methods Programs Biomed. 214:106538. doi: 10.1016/j.cmpb.2021.106538.
- Deng Q, Ou C, Chen J, Xiang Y. 2018. Particle deposition in tracheobronchial airways of an infant, child and adult. Sci Total Environ. 612:339–346. doi: 10.1016/j.scitotenv.2017.08.240.
- Di Cicco M, Kantar A, Masini B, Nuzzi G, Ragazzo V, Peroni D. 2021. Structural and functional development in airways throughout childhood: children are not small adults. Pediatr Pulmonol. 56(1):240–251. doi: 10.1002/ppul.25169.
- Dickison AE. 1987. The normal and abnormal pediatric upper airway. Recognition and management of obstruction. Clin Chest Med. 8(4):583–596. doi: 10.1016/S0272-5231(21)00777-2.
- Douwes J, Thorne P, Pearce N, Heederik D. 2003. Bioaerosol health effects and exposure assessment: progress and prospects. Ann Occup Hyg. 47(3):187–200. doi: 10.1093/annhyg/meg032.
- Dykewicz MS, Hamilos DL. 2010. Rhinitis and sinusitis. J Allergy Clin Immunol. 125(2 Suppl 2):S103–S115. doi: 10.1016/j.jaci.2009.12.989.
- Farhadi Ghalati P, Keshavarzian E, Abouali O, Faramarzi A, Tu J, Shakibafard A. 2012. Numerical analysis of micro- and nano-particle deposition in a realistic human upper airway. Comput Biol Med. 42(1):39–49. doi: 10.1016/j.compbiomed.2011.10.005.
- Hofmann W. 1982. Mathematical model for the postnatal growth of the human lung. Respir Physiol. 49(1):115–129. doi: 10.1016/0034-5687(82)90106-2.
- Hsu D-J, Chuang M-H. 2012. In-vivo measurements of micrometer-sized particle deposition in the nasal cavities of Taiwanese adults. Aerosol Sci Technol. 46(6):631–638. doi: 10.1080/02786826.2011.652749.
- Illum L. 2000. Transport of drugs from the nasal cavity to the central nervous system. Eur J Pharm Sci. 11(1):1–18. doi: 10.1016/s0928-0987(00)00087-7.
- Inthavong K, Ma J, Shang Y, Dong J, Chetty ASR, Tu J, Frank-Ito D. 2019. Geometry and airflow dynamics analysis in the nasal cavity during inhalation. Clin Biomech. 66:97–106. doi: 10.1016/j.clinbiomech.2017.10.006.
- Inthavong K, Tu J, Heschl C. 2011. Micron particle deposition in the nasal cavity using the v2–f model. Comput Fluids. 51(1):184–188. doi: 10.1016/j.compfluid.2011.08.013.
- Islam MS, Larpruenrudee P, Hossain SI, Rahimi-Gorji M, Gu Y, Saha SC, Paul G. 2021. Polydisperse aerosol transport and deposition in upper airways of age-specific lung. Int J Environ Res Public Health. 18(12):6239. doi: 10.3390/ijerph18126239.
- Jeong KY. 2016. Physical and biochemical characteristics of allergens. Allergy Asthma Respir Dis. 4(3):157–166. doi: 10.4168/aard.2016.4.3.157.
- Kelly JT, Asgharian B, Kimbell JS, Wong BA. 2004a. Particle deposition in human nasal airway replicas manufactured by different methods. Part I: inertial regime particles. Aerosol Sci Technol. 38(11):1063–1071. doi: 10.1080/027868290883360.
- Kelly JT, Asgharian B, Kimbell JS, Wong BA. 2004b. Particle deposition in human nasal airway replicas manufactured by different methods. Part II: ultrafine particles. Aerosol Sci Technol. 38(11):1072–1079. doi: 10.1080/027868290883432.
- Kelly JT, Prasad AK, Wexler AS. 2000. Detailed flow patterns in the nasal cavity. J Appl Physiol. 89(1):323–337. doi: 10.1152/jappl.2000.89.1.323.
- Kyung SY, Jeong SH. 2020. Particulate-matter related respiratory diseases. Tuberc Respir Dis. 83(2):116–121. doi: 10.4046/trd.2019.0025.
- Lim ZF, Rajendran P, Musa MY, Lee CF. 2021. Nasal airflow of patient with septal deviation and allergy rhinitis. Vis Comput Ind Biomed Art. 4(1):14. doi: 10.1186/s42492-021-00080-2.
- Luczynska CM, Li Y, Chapman MD, Platts-Mills, T, AE. 1990. Airborne concentrations and particle size distribution of allergen derived from domestic cats (Felis domesticus): measurements using cascade impactor, liquid impinger, and a two-site monoclonal antibody assay for Fel d I. Am Rev Respir Dis. 141(2):361–367. doi: 10.1164/ajrccm/141.2.361.
- Mercer MJ, van der Linde GP, Joubert G. 2002. Rhinitis (allergic and nonallergic) in an atopic pediatric referral population in the grasslands of inland South Africa. Ann Allergy Asthma Immunol. 89(5):503–512. doi: 10.1016/S1081-1206(10)62089-3.
- Morsi SA, Alexander AJ. 1972. An investigation of particle trajectories in two-phase flow systems. J Fluid Mech. 55(02):193. doi: 10.1017/S0022112072001806.
- Nasir ZA, Colbeck I, Sultan S, Ahmed S. 2012. Bioaerosols in residential micro-environments in low income countries: a case study from Pakistan. Environ Pollut. 168:15–22. doi: 10.1016/j.envpol.2012.03.047.
- Ounis H, Ahmadi G, McLaughlin JB. 1991. Brownian diffusion of submicrometer particles in the viscous sublayer. J Colloid Interface Sci. 143(1):266–277. doi: 10.1016/0021-9797(91)90458-K.
- Platts-Mills TA. 1998. The role of allergens in allergic airway disease. J Allergy Clin Immunol. 101(2 Pt 2):S364–S366. doi: 10.1016/s0091-6749(98)70221-0.
- Richter D. 2011. [Allergic rhinitis in children]. Acta Med Croatica. 65(2):163–168.
- Salati H, Khamooshi M, Vahaji S, Christo FC, Fletcher DF, Inthavong K. 2021. N95 respirator mask breathing leads to excessive carbon dioxide inhalation and reduced heat transfer in a human nasal cavity. Phys Fluids. 33(8):081913. doi: 10.1063/5.0061574.
- Salvaggio JE. 1994. Inhaled particles and respiratory disease. J Allergy Clin Immunol. 94(2):304–309. doi: 10.1053/ai.1994.v94.a56009.
- Schroeter JD, Garcia G, JM, Kimbell JS. 2011. Effects of surface smoothness on inertial particle deposition in human nasal models. J Aerosol Sci. 42(1):52–63. doi: 10.1016/j.jaerosci.2010.11.002.
- Schroeter JD, Tewksbury EW, Wong BA, Kimbell JS. 2015. Experimental measurements and computational predictions of regional particle deposition in a sectional nasal model. J Aerosol Med Pulm Drug Deliv. 28(1):20–29. doi: 10.1089/jamp.2013.1084.
- Shang YD, Inthavong K, Tu JY. 2015. Detailed micro-particle deposition patterns in the human nasal cavity influenced by the breathing zone. Comput Fluids. 114:141–150. doi: 10.1016/j.compfluid.2015.02.020.
- Stazi M-A, Sampogna F, Montagano G, Grandolfo ME, Couilliot M-F, Annesi-Maesano I. 2002. Early life factors related to clinical manifestations of atopic disease but not to skin-prick test positivity in young children. Pediatr Allergy Immunol. 13(2):105–112. doi: 10.1034/j.1399-3038.2002.00070.x.
- Stuart BO. 1984. Deposition and clearance of inhaled particles. Environ Health Perspect. 55:369–390. doi: 10.1289/ehp.8455369.
- Valerian Corda J, Emmanuel J, Nambiar S, K P, Zuber M. 2023. Airflow patterns and particle deposition in a pediatric nasal upper airway following a rapid maxillary expansion: computational fluid dynamics study. Cogent Eng. 10(1):2158614. doi: 10.1080/23311916.2022.2158614.
- Valerian Corda J, Satish Shenoy B, Arifin Ahmad K, Lewis L, K P, Rao A, Zuber M. 2023. Micro- and nanoparticle transport and deposition in a realistic neonatal and infant nasal upper airway. Int J Modell Simul. doi: 10.1080/02286203.2022.2164155.
- Van Strien J, Shrestha K, Gabriel S, Lappas P, Fletcher DF, Singh N, Inthavong K. 2021. Pressure distribution and flow dynamics in a nasal airway using a scale resolving simulation. Phys Fluids. 33(1):011907. doi: 10.1063/5.0036095.
- Wang DY, Lee HP, Gordon BR. 2012. Impacts of fluid dynamics simulation in study of nasal airflow physiology and pathophysiology in realistic human three-dimensional nose models. Clin Exp Otorhinolaryngol. 5(4):181–187. doi: 10.3342/ceo.2012.5.4.181.
- Wen J, Inthavong K, Tu J, Wang S. 2008. Numerical simulations for detailed airflow dynamics in a human nasal cavity. Respir Physiol Neurobiol. 161(2):125–135. doi: 10.1016/j.resp.2008.01.012.
- Wolfensberger M. 2002. Anti-inflammatory and surgical therapy of olfactory disorders related to sino-nasal disease. Chem Senses. 27(7):617–622. doi: 10.1093/chemse/27.7.617.
- Xi J, Berlinski A, Zhou Y, Greenberg B, Ou X. 2012. Breathing resistance and ultrafine particle deposition in nasal–laryngeal airways of a newborn, an infant, a child, and an adult. Ann Biomed Eng. 40(12):2579–2595. doi: 10.1007/s10439-012-0603-7.
- Yarragudi SB, Kumar H, Jain R, Tawhai M, Rizwan S. 2020. Olfactory targeting of microparticles through inhalation and bi-directional airflow: effect of particle size and nasal anatomy. J Aerosol Med Pulm Drug Deliv. 33(5):258–270. doi: 10.1089/jamp.2019.1549.
- Zamankhan P, Ahmadi G, Wang Z, Hopke PK, Cheng Y-S, Su WC, Leonard D. 2006. Airflow and deposition of nano-particles in a human nasal cavity. Aerosol Sci Technol. 40(6):463–476. doi: 10.1080/02786820600660903.
- Zhao K, Jiang J. 2014. What is normal nasal airflow? A computational study of 22 healthy adults: normal human nasal airflow. Int Forum Allergy Rhinol. 4(6):435–446. doi: 10.1002/alr.21319.
- Zhu JH, Lee HP, Lim KM, Lee SJ, Wang DY. 2011. Evaluation and comparison of nasal airway flow patterns among three subjects from Caucasian, Chinese and Indian ethnic groups using computational fluid dynamics simulation. Respir Physiol Neurobiol. 175(1):62–69. doi: 10.1016/j.resp.2010.09.008.
- Zubair M, Ahmad KA, Abdullah M, Sufian SF. 2015. Characteristic airflow patterns during inspiration and expiration: experimental and numerical investigation. J Med Biol Eng. 35(3):387–394. doi: 10.1007/s40846-015-0037-4.
- Zubair M, Riazuddin VN, Abdullah MZ, Ismail R, Shuaib IL, Ahmad KA. 2013. Computational fluid dynamics study of the effect of posture on airflow characteristics inside the nasal cavity. Asian Biomedicine. 7(6):835–840.
- Zubair M, Riazuddin VN, Abdullah MZ, Rushdan I, Shuaib IL, Ahmad KA. 2013. Computational fluid dynamics study of pull and plug flow boundary condition on nasal airflow. Biomed Eng Appl Basis Commun. 25(4):1350044. doi: 10.4015/S1016237213500440.
- Zuber M, Valerian Corda J, Ahmadi M, Satish Shenoy B, Anjum Badruddin I, E. Anqi A, Arifin Ahmad K, M. Abdul Khader S, Lewis L, Anas Khan M, et al. 2020. Investigation of coronavirus deposition in realistic human nasal cavity and impact of social distancing to contain COVID-19: a computational fluid dynamic approach. Comput Model Eng Sci. 125(3):1185–1199. doi: 10.32604/cmes.2020.015015.