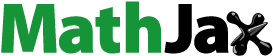
ABSTRACT
Adapting agriculture to climate change is essential for sustainable food production. However, the development of suitable adaptation mechanisms requires a clear understanding of the plant-climate interaction. The number of growing degree days (GDD) is a good proxy for understanding plant-climate interrelationship and farm productivity. Here, using a 2-year experiment of barley-sweet corn double cropping (DC) system and 20-year climate and yield data, we found that barley and sweet corn GDD and productivity were strongly related to changes in climate patterns. Furthermore, we found a positive effect of the barley-sweet corn DC system on farm productivity (18.5 and 5.6 tonnes. ha−1 for DC and single cropping respectively) and return on investment (1.8; 1.4 as benefit:cost ratios for DC and single cropping system respectively). Altogether the results of this study suggest that the winter barley-sweet corn double cropping system is a potential strategy to boost farm productivity as well as an adaptation mechanism to be considered for the changing climate in the study region.
Introduction
Understanding the plant-climate interaction is vital for sustainable crop management, food production, and adapting agriculture to the climate of the twenty-first century. The impacts of climate change are evident as the mean surface air temperature keep increasing. As such, agricultural resources are the most vulnerable to this change with severe consequences on crop production, food security, and farmer’s profitability. The Intergovernmental Panel on Climate Change (IPCC) estimated that the mean temperature on Earth’s surface will rise by 1.1–6.4°C by 2100, with significant effects on the length of the growing season. For example, it is estimated that under the harsh conditions of climate change, yields would drop by 10–20% by the end of the twenty-first century. Understanding the impact change in climate patterns has on agriculture is vital, especially in the Carpathian Basin (Farkas et al. Citation2014; Gelybó et al. Citation2018), as the Basin is considered as a hub for cereal production for Europe (Pinke and Lövei Citation2017). Furthermore, recent research in the Carpathian Basin have shown a significant decrease in yield associated with the rise in temperature and reduced precipitation. For example, in Hungary, climate accounted for 33–67% variability in cereal yields between 1981 and 2010 (Pinke and Lövei Citation2017). As such, the effect of climate change on future crop production in this dynamic Basin (Bartholy and Pongracz Citation2007; Bartholy et al. Citation2009) needs thorough analysis, especially the effect long and warm periods have on crop growth and yields. One of the possible ways to address the impact of climate change in the region could be double cropping (DC) – a cropping system where two crops are produced in one season or one cropping year (Kawasaki Citation2019). However, t is still debatable whether an increase in cropping frequency may help address the projected negative impacts of climate change on food production in different agroecological zones (Seifert and Lobell Citation2015; Teixeira et al. Citation2018; Heil et al. Citation2021; Burgess et al. Citation2022). For instance, multiple cropping systems that combine two different crop types and different farm management have been shown to eliminate the risk of crop failure under climate stress thereby improving crop yield and overall agricultural production (Seifert and Lobell Citation2015; Burgess et al. Citation2022). In addition, DC is profitable in areas where it is currently practiced, due to an increased return on investments to farmers (Swan et al. Citation1987; Rodríguez et al. Citation2015). Consequently, the land under DC system is significantly growing, suggesting a good alternative to restrict weather-driven losses in agriculture (Moore and Karlen Citation2013; Cohn et al. Citation2016; Pires et al. Citation2016).
The success of DC, depends on the region and the prevailing climate conditions, the special characteristics of the crop species, the market demand, and the interaction of all these factors (Kawasaki Citation2019). This is specifically important for the Carpathian Basin and the temperate regions as coping with weather patterns can be challenging (Farkas et al. Citation2014). This is because the second half of the cropping year is often not long enough to sustain the second crop mainly due to early drop in temperature or limited soil moisture to sustain crop development. The drop in temperature to below zero has a significant negative impact on the second crop’s growth and maturity (Rodríguez et al. Citation2015). It has been suggested that climate adaptation mechanisms like DC need to be integrated into agricultural policies, but their success still needs further assessment. Consequently, the main objective of this study was to understand the plant-climate interrelationship via double cropping and its effects on crop yield and farm profitability.
Specifically, the study was centred on three main questions: (1) How does double cropping interact with climate to influence barley-sweet corn yields? (2) Can double cropping be considered as a potential strategy to address the impact of climate change in the Carpathian region? (3) What are the climatic factors that influence barley-sweet corn double cropping system in the investigated sites? (4) Is there a positive return on investment as a result of a double cropping system vis-à-vis to single cropping system? We hypothesise that climate change has altered temperature patterns and has extended the daily thermal temperature (DTT) in our study regions, thereby creating suitable environmental conditions essential for a second crop in one cropping year. Consequently, a change in DTT will likely extend the number of growing degree days (GDD) in favour of double cropping system and higher return on investments for farmers.
Materials and methods
Study area
The study was conducted in five farms located in the East, and South-East of Hungary (), in the Great Hungarian Plain, which is a flat, fertile lowland and the country's main agricultural region. The climate in the region is classified as a moderately continental climate with cold summers. Long-term (20 years) data from nearby meteorological stations show that mean values for annual temperature (MAT) are (11.4 ± 0.75 ˚C.year−1) and mean annual precipitation (MAP) is (511.2 ± 131.6 mm.year−1) (). Note that climate stations selected for this study were on average 10 km distant from the investigated and there was no difference in temperature and precipitation between fields. The land use typology is dominated by arable farming with each of the study fields located close to natural or artificial streams that can be used to irrigate crops. The average field size for the study fields was 5 hectares characterised by very little relief intensity. Based on the World reference base for soil resources, the study areas are dominated by fertile Calcic Chernozems, with deep humus-rich topsoil and well-drained subsoil with loess parent material (WRB Citation2014).
Figure 1. An overview map of the study area with locations of the farms investigated in the cropping years 2019–2020 and 2020–2021.

Figure 2. Distribution patterns of mean annual precipitation and mean annual temperature for the period of 2002–2022. Both precipitation and temperature come from aggregated hourly data. Source: Hungarian Meteorological Service.

Prior to each study year, a soil sampling and soil laboratory analysis were performed to assess the soil characteristics of the topsoil (∼30 cm) to create crop-specific nutrient management plans based on soil nutrient status. Fertilisers were applied based on the nutrient plans, and seeding rates were varying according to field conditions, and crop varieties. Note that in the study region, sweet corn is always irrigated regardless of whether it is a main crop or a secondary crop.
Data collection
In 2019 to 2021, we collected data from the investigated farms including the actual farming practices, cropping system (rotation system), and crop yield. Furthermore, we collected financial data on production costs and return on investment for barley and sweet corn (Figure A5). For our analysis, we divided the data into two cropping systems namely ‘double cropping’ (barley followed by sweet corn in the same cropping year) and ‘single cropping’ system (barley alone). Note for single cropping, we used data collected before the implementation (experiment) of double cropping. Throughout the paper ‘year’ refers to the cropping year, not the calendar year. We then calculated the benefit–cost ratio as a metric to understand farm and cropping system productivity and profitability.
To assess the long-term effect of climate on the yield of barley and sweet corn, we used annual yield data published by the Hungarian Central Statistical Office for different crops and the climate data publicly available from the Hungarian Meteorological Service. Climate data included the minimum, mean, and maximum hourly temperature; the hourly precipitation; hourly humidity and hourly wind direction. For climate data, we used data from the closest meteorological station for each farm. Where two or three stations were available, an average of all stations was calculated to ensure the accuracy of weather patterns. To assess the success of sweet corn as a second crop in barley-sweet corn double cropping, we used the most common metric for crop development assessment ‘Growing Degree Days (GDD)’. To calculate GDD for sweet corn, we used the simplest formula as described in (McMaster and Smika Citation1988; Zhou and Wang Citation2018) and in the equations below (Equations 1 and 2). We also calculated the number of days suitable for growing sweet corn in each year following equation (Equation 3).
(1)
(1)
(2)
(2)
(3)
(3) where DTT is the daily thermal temperature, Tavg is the average temperature, Tb is the base temperature, and Tu is the upper threshold temperature. Note Tb for sweet corn we used 10°C and 4.5°C for barley. For Tu we used 35°C for all crops (Kukal and Irmak Citation2018; Zhou and Wang Citation2018). Nd is the number of days suitable for growing corn, D represents days, k is the day of sowing sweet corn (Generally 15 days after harvesting barley), and n is the day before the first record of minus temperature. In other words, it is the day before the temperature drops to below zero (< 0°C). Note that farmers harvest corn before the temperature drops to below zero degrees Celsius.
Statistical analysis
In the first part of the analysis, we assessed barley and sweet corn yield distribution across the investigated farms and over a two-year cropping period. To assess whether there was a difference in crop yield and benefit:cost ratios between years, and between two cropping systems (i.e. double cropping and single cropping systems) we used the non-parametric Kruskal–Wallis test (Kruskal and Wallis Citation1952). To estimate the uncertainty associated with the estimates of Kruskal–Wallis, we conducted the bootstrap resampling method (B. Efron Citation1979) with 3000 iterations and presented the 95% confidence interval. Both Kruskal–Wallis and the bootstrap approaches provide a robust way of hypothesis testing as they do not depend on normally distributed data (Pearce and Derrick Citation2019).
In the second step of the analysis, we conducted a correlation analysis between variables representing climate indices (GDD), sweet corn growing length, and time. This allowed us to assess whether there were clear trends over time. In the final stage of the analysis, we used selected climate variables in a Bayesian generalised linear model (Kruschke Citation2021; van de Schoot et al. Citation2021; van Doorn et al. Citation2021) to assess the effect of climate on sweet corn yield, and whether these variables significantly affect sweet corn growing length based on its GDD. Further, we used climate parameters to assess their influence on GDD patterns. Before the analysis, all explanatory and response variables were normalised using the Min–Max transformation method to avoid the effect of differences in units of measurement. For this final part of the analysis, we focused on sweet corn. This is because, sweet corn is hard to grow as a second crop due to a lack of sufficient soil moisture after barley harvest or lack of sufficient warmer days to support its growth up to maturity before temperature drops to below zero (). For the model setup and assessment, we used the Markov Chain Monte Carlo algorithm (MCMC) set up for 3000 iterations, using 6 separate chains. We then reported the distribution of parameters for each factor as the posterior effect size of that factor on the response variable (i.e. crop yield). For the model assessment, we used the trace plots, and the energy plot to evaluate the model goodness of fit and convergence over the course of the MCMC sampling process. All analyses for the Bayesian model were performed using the PyMC ecosystem (Salvatier et al. Citation2016).
Figure 3. A Bayesian generalised linear model architecture used for our explanatory analysis. The model included five climate variables: The growing degree days (GDD), maximum temperature (Tmax), average temperature (Tavg), minimum temperature (Tmin), and precipitation (Rain). The sigma represents the error term of the model. As all explanatory variables showed a normal distribution, we set the parameters of the prior regression model to ‘Normal’. In this model, the sweet corn yield and GDD were response variables.

Results
Effects of double cropping on sweet corn yield and farm profitability
Using experimental data from the investigated fields for the cropping years 2019–2020 and 2020–2021, we found that the average annual crop yield was 6.0 tonnes. ha−1.year−1 and 13.0 tonnes.ha−1.year−1 for barley and sweet corn respectively ((a)). The long-term observational data (20 years) show that on average the annual yield for barley was 4.3 tonnes.ha−1.year−1. For sweet corn, the annual average yield was 14.4 tonnes. ha−1.year−1. By combining barley and sweet corn yields during double cropping, the overall crop yield was 18.5 tonnes. ha−1; compared to single cropping 5.6 tonnes. ha−1 ((b)). When comparing yields between fields for the two cropping systems for 2019–2020 and 2020–2021, we found that the total yield of barley and sweet corn was not statistically different between the two cropping systems (i.e. Double and single cropping systems) ((a)). In contrast, when comparing aggregated data of total yield for both barley and sweet corn combined, we found a statistically significant difference between double and single cropping systems ((b)). When comparing financial data for the two cropping years periods, for all investigated farms, we observed significant differences in benefit:cost ratio between double and single cropping system. For all investigated fields, the benefit–cost ratios were higher (20–23%) in the cropping year 2020–2021 compared to 2019–2020 ((c)). When comparing the two cropping systems, that is, double cropping and single cropping, we found a significantly higher benefit–cost ratio (1.8 ± 0.7) associated with double cropping compared to the single cropping system (1.4 ± 0.4). The significance analysis test and the cut-off p-value were provided in the figure caption ((c)).
Figure 4. Crop production and financial data (Mean ± sd, N = 5) for the investigated fields for the cropping year periods of 2019–2020 and 2020–2021. (a) Comparison of barley and sweet corn yield between double and single cropping for the 5 farms investigated in this study. Kruskal–Wallis was conducted on double and single cropping systems separately. The letter ‘x’ indicates no significant differences based on bootstrap (10,000 iterations, p < 0.05), (b) Barley and sweet corn yield (combined data) during the cropping years 2019–2020 and 2020–2021 for double and single cropping system, (c) comparison of the benefit–cost ratio between double and single cropping system for the cropping years 2020 and 2021. For all panels, different letters indicate statistically significant differences in the distribution of values based on Kruskal–Wallis and Bootstrap (10,000 iterations, p < 0.05).

Explanatory power of climate parameters on sweet corn yield and sweet corn growing days degree (GDD) over time
We found clear trends of plant traits associated with climate over time. A closer analysis of correlation shows a significant positive correlation between plant traits such as the number of days suitable for corn growth and the year of data collection (r = 0.4, p-value < 0.05) ((a)). Furthermore, based on 20 years dataset we found a positive and significant correlation between the growing degree days (GDD) associated with sweet corn growth and time (r = 0.5, p-value < 0.05) ((b)). We found also a positive and significant relationship between barley GDD and time (r = 0.6, p-value < 0.05) ((c)). Consequently, the long-term yield data revealed a strong correlation between barley yield and year of harvest (r = 0.9, p-value < 0.05) ((d)).
Figure 5. Pearson correlation analysis between variables used in our analysis. (a) the correlation between corn growing days and time (years), (b) the correlation between sweet corn GDD and time, (c) the correlation between barley GDD and time, and (d) the correlation between barley yield and time.

Overall, we found a strong effect of climate variables on sweet corn production and sweet corn GDD ((a,b)). Based on the generalised linear model, we found that average annual precipitation and temperature emerged as the most important variables driving sweet corn yield over the last 20 years ((a), Figure A1). Specifically, the annual precipitation had the highest effect size on sweet corn, followed by the annual maximum temperature, and the mean annual temperature ((a)). The corn GDD showed a weak and negative effect on sweet corn. For sweet corn GDD, we found that maximum temperature, mean annual temperature and annual precipitation explain most of the variability in GDD of sweet corn, but the difference between precipitation and the mean annual temperature was not significant ((b), Figure A2). For all sampling chains, our data show that all models fitted well observed data and converged well throughout the Markov Chain Monte Carlo algorithm (MCMC) sampling process (Figure A3).
Figure 6. The distribution of standardised posterior effect size of major climatic parameters on (a) sweet corn yield, (b) GDD associated with sweet corn. (Rain) indicates mean annual precipitation, (Tmin) indicates annual minimum temperature, Tavg indicates mean annual temperature, (Tmax) indicates annual maximum temperature, and (GDD) growing degree days. The vertical, dashed line separates negative and positive effects. The white dot indicates the average effect size and the whisker represents a 95% confidence interval.

Discussion
Double cropping, crop yields, and farm profitability
Overall, the results of this study show that there is indeed an opportunity to integrate barley-sweet corn into a double cropping system. Experimental data shows that barley-sweet corn double cropping significantly increased the annual crop yields and farm profitability in the study sites (). When comparing the total yields of barley and corn (i.e. combined), we found that farm productivity associated with double cropping was three times higher compared to the single cropping system, resulting in higher farm productivity per unit area ((b)). This difference has significant implications on farm profitability as well. For example, using the benefit:cost ratio as a proxy of farm profitability, we found that farm profitability increased by two-fold in 2019–2020 and 2020–2021 as a result of double cropping compared to a single cropping system ((c)). However, when comparing data across the investigated fields, the results showed that investigated farms had slight differences in crop yield, but the differences were not statistically significant and consistent ((a)). These slight differences between fields are likely due to slight differences in soil characteristics and farm management. Soil properties and farm management practices can affect crop growth and productivity (van der Sloot et al. Citation2022). However, given that, the investigated fields were homogeneous in terms of soil properties and management, the small differences in yields observed across the investigated fields can be attributed to minor differences in soil properties, type of crop rotation, and farm management respectively (, Figure A4). These findings are consistent with existing literature. For example, the positive effect of double cropping on crop yields and farm productivity was also reported in other regions and its increasing adoption has been reported in other regions in the last decade (Liu et al. Citation2013; Ray and Foley Citation2013; Seifert and Lobell Citation2015; Pires et al. Citation2016). A few challenges remain though, especially in our study region. First, there is a common belief that farmers would need to harvest barley before the peak of its grain size, biomass, and yield. Second, the early drop in temperatures to below zero in our study sites (i.e. less warm days) may force farmers to harvest sweet corn before its maturity. Lastly, there are still assumptions that there is not enough moisture to support sweet corn growth after barley harvest. We acknowledge the role of best management practices (such as timing in planting and harvesting, and maintaining and increasing in-situ soil moisture content) as drivers of farm productivity and return on investment in the long term (Martinho Citation2019; Piñeiro et al. Citation2020; Liu and Brouwer Citation2022). However, the results of this study show that farmers can indeed integrate barley-sweet corn into one cropping year through a double cropping system if supported by irrigation (often practiced in our study sites) to maintain soil moisture. Furthermore, the success of the sweet corn as well as the implementation of barley-sweet corn double cropping depends on the heat accumulation of sweet corn in the second season of the cropping year. The results suggest that sweet corn GDD has significantly increased in the past 20 years ((a,b)) and this is likely to have a positive impact on barley-sweet corn double cropping system. Therefore, we argue that coupling double cropping with other best farming practices such as irrigation practice can boost yields in barley-sweet corn double cropping system in our study sites under the changing climate.
Table 1. Field management practices for the investigated fields.
The effect of climate on GDD, crop yield, and suitability of double cropping
When assessing the variability of GDD over time, we found a significant and positive correlation between GDD and year. Consequently, the number of days suitable for corn growth has been increasing over the past 20 years ((a–c)). An increase in GDD has potential implications for implementing a double cropping system. For example, GDD and the increasing number of days suitable for sweet corn production suggest an extended period of warmer days which are likely to increase the growing window (i.e. the length of the sweet corn season) for sweet corn in the second season of the cropping year. As such an increase in the length of sweet corn season will thereby provide farmers with favourable conditions for double cropping vis-à-vis the single cropping system. However, the relationship between GDD, double cropping, and crop yield remains unclear. This relationship depends largely on the plant’s heat accumulation throughout the season as has been reported in other studies (Swan et al. Citation1987; Mishra Citation2013). When assessing the effect of GDD on sweet corn, we found a good explanatory power of GDD on sweet corn yield, but this relationship was weaker when adding climate parameters to the model ((a)). This is because GDD depends also on climate parameters ((b)) which acted as cofounder factors in GDD, double cropping, and sweet corn yield interrelationship. For example, precipitation (rainfall) and maximum temperature showed the highest effects on sweet corn and GDD (). These results suggest that although GDD influences crop growth and productivity, precipitation and maximum temperature will play a big role in agriculture production under changing climatic conditions (i.e. water availability or occurrence of extreme heat). We acknowledge that the occurrence of extreme heat may lead to cereal heat stress and reduced farm productivity and profitability (Pinke and Lövei Citation2017). But the exact response of crops to rising temperature is still debatable among the scientific community. Other, studies have shown that the increase in temperature will likely be the most important driver of double cropping suitability in agroecosystems (Byjesh et al. Citation2010; Seifert and Lobell Citation2015). Based on the previous studies and the fact that our data showed a strong effect of temperature on GDD and sweet corn yield ((b)), provide evidence that the rising temperature will indeed create suitable conditions for double cropping, and this should be considered as a strategy to mitigate future impacts of climate change.
Conclusions
Changes in climate patterns are rapidly altering farming practices, especially in the lowlands where temperatures are higher compared to their counterparts’ highlands. The interaction of rising temperatures and plants and the impact this has on agriculture productivity still need further investigation. Using experimental and observational data, our findings suggest that double cropping system is a potential solution for mitigating the impact of climate change in the Carpathian Basin. The study revealed a strong effect of double cropping on barley-sweet corn productivity and farm profitability. Furthermore, our data suggest that variability in climate parameters (precipitation and maximum temperature) dictates the suitability of a double cropping system in the study region. Overall, we found that the variability of temperature over the last 20 years increased the sweet corn Growing degree days thereby creating suitable environmental conditions for a barley-sweet corn double cropping system. Consequently, this had a strong positive effect on overall cereal productivity and return on investment. Altogether, the results of this study suggest that double cropping system is a potential adaptation strategy to be considered to cope with the impact of climate change and this has implications for cereal production in this cereal-growing hub of Europe.
Acknowledgements
VL conceptualised the study and acquired the funding; BB, IV, KM, DS, SC. Curated the data; IC, IB, GPK, LV, and VL developed and conducted the experiment, BB analysed and visualised the data; VL, BB, and DD lead the writing – original draft; VL and BB finalised the manuscript. All authors have read and agreed to the latest version of the manuscript.
Disclosure statement
No potential conflict of interest was reported by the author(s).
Data availability statement
All data used to make conclusions in this study are available upon reasonable request to the corresponding author.
Additional information
Funding
Notes on contributors
Vince Láng
Vince Láng is the director and research scientist at Discovery Center Nonprofit Kft (DC). He has a PhD in environmental sciences obtained at the Hungarian University of Agriculture and Life Sciences. He served as a senior researcher at the Department of Soil Science before moving to DC. He was a visiting scientist at the Faculty of Agronomy, at Purdue University, and the International Institute for Tropical Agriculture in Tanzania to support the African Soil Information System (AFSIS) initiative.
Dimitra Dafnaki
Dimitra Dafnaki is an agronomist, and research associate, at the Agricultural University of Athens. She is also a Data Manager at Eden Library—a research company offering AI innovative solutions to farmers, such as crop health monitoring, yield estimation, and precision spraying.
István Balla
István Balla is currently an associate professor of Agronomy at the Hungarian University of Agriculture and Life Sciences. He is also serving as the Managing director of GAK—a Non-profit organization focusing on education, research, and farm operations.
Ivan Czako
Ivan Czako is a senior researcher at Karotin Ltd. He has a master's degree in plant protection and a bachelor's degree in horticulture engineering.
Sándor Csenki
Sándor Csenki is a Researcher at Discovery Center and PhD Student at the University of Miskolc. He has 5 years of experience in precision agriculture mainly focusing on digital soil mapping, and currently doing my PhD in the same area of research.
Gergő Péter Kovács
Gergő Péter Kovács is Associate professor professor at MATE, Institute of Agronomy and Deputy director of Institute of Agronomy. His research focus is on soil and land use management, regenerative agriculture, and adaptable crop production.
Kennedy Mutua
Kennedy Mutua is a researcher at the Institute of Agricultural Economics and a specialist in environmentally conscious agriculture. His fields of research stem from quantification of the impacts of climate change across the agricultural sector.
Dora Szlatenyi
Dora Szlatenyi is an agricultural engineer in environmental management at Agridron Ltd with 6 years of experience in different segments of agriculture. She is currently helping in the development of farmers' precision plans and managing the Discovery Center Living Lab
László Vulcz
László Vulcz is a Executive Director of OTP Hungaro-Projekt Ltd and PhD candidate at the Hungarian University of Agriculture and Life Sciences (MATE). As executive director, László Vulcz has been managing OTP Hungaro-Projekt Ltd, an EU tender writing and project management consultancy firm, since 2015. Between 2008 and 2015, as the director of the Directorate of Rural Development Grants of Agriculture and Rural Development Agency (MVH), he performed tasks related to the management of the Agency.
Benjamin Bukombe
Benjamin Bukombe is an early career researcher and is currently a lead researcher at Discovery Center Nkft. Over the past 7 years, I have gained experience developing and coordinating multidisciplinary research projects in Africa, the Middle East, and Europe. He has a PhD in Geosciences with a major focus on soil-plant-climate interaction.
References
- Bartholy J, Pongracz R. 2007. Regional analysis of extreme temperature and precipitation indices for the Carpathian Basin from 1946 to 2001. Glob Planet Change Extreme Clim Events. 57:83–95. doi:10.1016/j.gloplacha.2006.11.002.
- Bartholy J, Pongracz R, Torma C, Pieczka I, Kardos P, Hunyady A. 2009. Analysis of regional climate change modelling experiments for the Carpathian Basin. Int J Glob Warm. 1:238–252. doi:10.1504/IJGW.2009.027092.
- Burgess AJ, Correa Cano ME, Parkes B. 2022. The deployment of intercropping and agroforestry as adaptation to climate change. Crop Environ. 1:145–160. doi:10.1016/j.crope.2022.05.001.
- Byjesh K, Kumar SN, Aggarwal PK. 2010. Simulating impacts, potential adaptation and vulnerability of maize to climate change in India. Mitig Adapt Strateg Glob Change. 15:413–431. doi:10.1007/s11027-010-9224-3.
- Cohn AS, VanWey LK, Spera SA, Mustard JF. 2016. Cropping frequency and area response to climate variability can exceed yield response. Nat Clim Change. 6:601–604. doi:10.1038/nclimate2934.
- Efron B. 1979. Bootstrap methods: another look at the jackknife. Ann Stat. 7:1–26. doi:10.1214/aos/1176344552.
- Farkas C, Gelybó G, Bakacsi Z, Horel Á, Hagyó A, Dobor L, Kása I, Tóth E. 2014. Impact of expected climate change on soil water regime under different vegetation conditions. Biologia. 69:1510–1519. doi:10.2478/s11756-014-0463-8.
- Gelybó G, Tóth E, Farkas C, Horel Á, Kása I, Bakacsi Z. 2018. Potential impacts of climate change on soil properties. Agrokémia és Talajtan. 67:121–141. doi:10.1556/0088.2018.67.1.9.
- Heil K, Gerl S, Schmidhalter U. 2021. Sensitivity of winter barley yield to climate variability in a Pleistocene Loess area. Climate. 9:112. doi:10.3390/cli9070112.
- Kawasaki K. 2019. Double cropping as a strategy for climate change adaptation. Am J Agric Econ. 101:172–192. doi:10.1093/ajae/aay051.
- Kruschke JK. 2021. Bayesian analysis reporting guidelines. Nat Hum Behav. 5:1282–1291. doi:10.1038/s41562-021-01177-7.
- Kruskal WH, Wallis WA. 1952. Use of ranks in one-criterion variance analysis. J Am Stat Assoc. 47:583–621. doi:10.1080/01621459.1952.10483441.
- Kukal MS, Irmak S. 2018. U.S. agro-climate in 20th century: growing degree days, first and last frost, growing season length, and impacts on crop yields. Sci Rep. 8:6977. doi:10.1038/s41598-018-25212-2.
- Liu H, Brouwer R. 2022. Incentivizing the future adoption of best management practices on agricultural land to protect water resources: the role of past participation and experiences. Ecol Econ. 196:107389. doi:10.1016/j.ecolecon.2022.107389.
- Liu L, Xu X, Zhuang D, Chen X, Li S. 2013. Changes in the potential multiple cropping system in response to climate change in China from 1960–2010. PLoS One. 8:e80990. doi:10.1371/journal.pone.0080990.
- Martinho VJPD. 2019. Best management practices from agricultural economics: mitigating air, soil and water pollution. Sci Total Environ. 688:346–360. doi:10.1016/j.scitotenv.2019.06.199.
- McMaster GS, Smika DE. 1988. Estimation and evaluation of winter wheat phenology in the central great plains. Agric For Meteorol. 43:1–18. doi:10.1016/0168-1923(88)90002-0.
- Mishra V. 2013. 2.12 – food security implications of climate variability and climate change. In: Pielke RA, editor. Climate vulnerability. Oxford: Academic Press; p. 117–128. doi:10.1016/B978-0-12-384703-4.00223-9.
- Moore KJ, Karlen DL. 2013. Double cropping opportunities for biomass crops in the north central USA. Biofuels. 4:605–615. doi:10.4155/bfs.13.50.
- Pearce J, Derrick B. 2019. Preliminary testing: the devil of statistics? Reinvention Int J Undergrad Res. 12. doi:10.31273/reinvention.v12i2.339.
- Piñeiro V, Arias J, Dürr J, Elverdin P, Ibáñez AM, Kinengyere A, Opazo CM, Owoo N, Page JR, Prager SD, Torero M. 2020. A scoping review on incentives for adoption of sustainable agricultural practices and their outcomes. Nat Sustain. 3:809–820. doi:10.1038/s41893-020-00617-y.
- Pinke Z, Lövei GL. 2017. Increasing temperature cuts back crop yields in Hungary over the last 90 years. Glob Change Biol. 23:5426–5435. doi:10.1111/gcb.13808.
- Pires G, Abrahão G, Brumatti LM, Oliveira LJ, Costa MH, Liddicoat S, Kato E, Ladle R, Ladle R. 2016. Increased climate risk in Brazilian double cropping agriculture systems: implications for land use in Northern Brazil. Agric For Meteorol. 228:286–298. doi:10.1016/J.AGRFORMET.2016.07.005.
- Ray DK, Foley JA. 2013. Increasing global crop harvest frequency: recent trends and future directions. Environ Res Lett. 8:044041. doi:10.1088/1748-9326/8/4/044041.
- Rodríguez VM, Soengas P, Alonso-Villaverde V, Sotelo T, Cartea ME, Velasco P. 2015. Effect of temperature stress on the early vegetative development of Brassica oleracea L. BMC Plant Biol. 15:145. doi:10.1186/s12870-015-0535-0.
- Salvatier J, Wiecki TV, Fonnesbeck C. 2016. Probabilistic programming in Python using PyMC3. PeerJ Comput Sci. 2:e55. doi:10.7717/peerj-cs.55.
- Seifert C, Lobell D. 2015. Response of double cropping suitability to climate change in the United States. Environ Res Lett. 10:024002. doi:10.1088/1748-9326/10/2/024002.
- Swan JB, Schneider EC, Moncrief JF, Paulson WH, Peterson AE. 1987. Estimating corn growth, yield, and grain moisture from air growing degree days and residue cover. Agron J. 79:53–60. doi:10.2134/agronj1987.00021962007900010012x.
- Teixeira EI, de Ruiter J, Ausseil A-G, Daigneault A, Johnstone P, Holmes A, Tait A, Ewert F. 2018. Adapting crop rotations to climate change in regional impact modelling assessments. Sci Total Environ. 616–617:785–795. doi:10.1016/j.scitotenv.2017.10.247.
- van de Schoot R, Depaoli S, King R, Kramer B, Märtens K, Tadesse MG, Vannucci M, Gelman A, Veen D, Willemsen J, Yau C. 2021. Bayesian statistics and modelling. Nat Rev Methods Primer. 1:1–26. doi:10.1038/s43586-020-00001-2.
- van der Sloot M, Kleijn D, De Deyn GB, Limpens J. 2022. Carbon to nitrogen ratio and quantity of organic amendment interactively affect crop growth and soil mineral N retention. Crop Environ. 1:161–167. doi:10.1016/j.crope.2022.08.001.
- van Doorn J, van den Bergh D, Böhm U, Dablander F, Derks K, Draws T, Etz A, Evans NJ, Gronau QF, Haaf JM, et al. 2021. The JASP guidelines for conducting and reporting a Bayesian analysis. Psychon Bull Rev. 28:813–826. doi:10.3758/s13423-020-01798-5.
- WRB. 2014. World reference base for soil resources 2014.
- Zhou G, Wang Q. 2018. A new nonlinear method for calculating growing degree days. Sci Rep. 8:10149. doi:10.1038/s41598-018-28392-z.
Appendix
Figure A1. Model check: a trace plot showing the output of a Markov Chain Monte Carlo (MCMC) sampling process and sampled values of model parameters over the course of the 3000 sampling iterations, and the 6 chains. The left side of the trace plot shows the distribution of parameter values and each horizontal line corresponds to a different parameter (Rain, Tmin, Tavg, Tmax, and GDD) being sampled. The X-axis on the right side shows the iteration of the MCMC sampling process. It shows how the convergence of the parameter values changes over the course of the 3000 sampling iterations. Note the stability of the parameter values throughout the sampling process suggesting that they did not change significantly.

Figure A2. Model check: a trace plot showing the output of the MCMC sampling process and sampled values of model parameters throughout the 3000 sampling iterations, and the 6 chains. The left side of the trace plot shows the posterior distribution of parameter values while the right side shows the convergence of the posterior distribution of the parameter values throughout 3000 iterations and 6 chains. For details see (Figure A1).

Figure A3. The energy plot shows the marginal energy and the energy transition for the 3000 iterations of the MCMC sampling process. The distribution of the marginal energy values shows the current fit of the model to the data, while the energy transition reveals the progression of the energy values over the course of the sampling process. In this case, the good agreement between the two density plots and the similarity of the two distributions indicates that the model converged and fit well the data at each step of the sampling process.

Figure A4. Distribution of major soil properties for the investigated fields. (a) Distribution of soil organic matter content across the investigated fields, (b) Distribution of soil pH, (c) distribution of plant available phosphorus and (d) distribution of plant available potassium. The white dot in the middle indicates the mean value. Note that the Y-axis was rescaled to improve visualisation.
