ABSTRACT
Additive manufacturing (AM), also known as 3D printing, is widely believed to enhance supply chain resilience (SCR). However, there is a lack of empirical frameworks to provide directions for practitioners and scholars in this regard. Motivated by the dynamic capabilities view, this exploratory survey research aims to overcome this gap. To this end, empirical data are collected from a heterogeneous sample of experts involved in different industries at the forefront of AM. These data are used to explore pathways through which AM adoption leads to enhancing SCR via different resilience practices. More specifically, the collected data are analyzed to explain how AM adoption affects different resilience practices and how these practices in turn affect SCR. Based on these findings, a preliminary practice-based framework is developed that can support practitioners in deploying AM-enabled resilience practices aimed at generating the supply chain (SC) capabilities necessary for dealing with SC vulnerabilities and therefore enhancing SCR. Moreover, relevant propositions are put forward that reflect these findings and open up avenues for future research.
1. Introduction
In today’s unpredictable and turbulent markets, supply chain (SC) vulnerabilities are an issue of significance for many firms around the world (Christopher and Peck Citation2004). SC vulnerabilities are fundamental factors that make a firm susceptible to disruptions (e.g. pandemics, natural disasters, geopolitical unrest), thus undermining its supply chain resilience (SCR) (Pettit, Fiksel, and Croxton Citation2010, Pettit et al. Citation2013). To deal with SC vulnerabilities, firms strive to develop SC capabilities, which are attributes that enable them to anticipate and overcome disruptions (Pettit, Croxton, and Fiksel Citation2013; Pettit, Fiksel, and Croxton Citation2010). To build SC capabilities and enhance their SCR, many firms are inclined toward adopting Industry 4.0 technologies (Hägele, Grosse, and Ivanov Citation2023). This trend became quite evident during the COVID-19 pandemic when many industrial firms focused on evaluating the opportunities that arise from the adoption of these digital technologies to enhance SCR (Belhadi et al. Citation2021; Ivanov, Blackhurst, and Das Citation2021).
Recent empirical studies such as Zouari et al. (Citation2020) and Balakrishnan and Ramanathan (Citation2021) mention the importance of adopting digital technologies such as additive manufacturing (AM) to enhance SCR. However, the literature lacks adequate empirical results to specify the opportunities that arise from adopting AM technology for enhancing SCR (Ali and Gölgeci Citation2019; Iftikhar et al. Citation2022). This gap is highlighted by Naghshineh and Carvalho (Citation2022a), who performed a systematic search and review of the literature to propose the potential implications of AM technology adoption for SCR. They posit that adopting AM technology affects the state of the SC in ways that influence important SC capabilities and vulnerabilities that underlie SCR. There are dispersed pieces of evidence in extant literature that support their proposition. For example, AM technology enables the distributed manufacturing of products in locations closer to customers (e.g. localized manufacturing/on-site manufacturing), considerably reducing their delivery lead time (Durão et al. Citation2017; Naghshineh, Fragoso, and Carvalho Citation2023). Reducing delivery lead time is a resilience practice that improves SC adaptability (Carvalho et al. Citation2022), helping to cope with SC vulnerabilities such as unpredictability in customer demand and therefore enhancing SCR (Pettit, Croxton, and Fiksel Citation2013; Pettit, Fiksel, and Croxton Citation2010).
Given the opportunity to deploy such AM-enabled resilience practices, different firms are willing to adopt this technology to build SC capabilities against SC vulnerabilities and enhance their SCR (Mellor, Hao, and Zhang Citation2014; Pettit, Croxton, and Fiksel Citation2019). This assertion is in line with Balakrishnan and Ramanathan (Citation2021), who posit that using digital technologies such as AM ‘will encourage the firm’s supply chain resilience practices’, and Zouari et al. (Citation2020) who posit that adopting digital technologies such as AM improves the SC capabilities necessary to enhance SCR. Thus, in line with the dynamic capabilities view, understanding how AM technology adoption can affect resilience practices is important for developing the SC capabilities necessary to deal with SC vulnerabilities (Naghshineh and Carvalho Citation2022b; Pettit, Croxton, and Fiksel Citation2019) and therefore enhancing SCR (Chowdhury and Quaddus Citation2017; Ponomarov and Holcomb Citation2009). However, to the best of the authors’ knowledge, to date, no empirical study has focused on investigating the implications of AM technology adoption for SC capabilities and vulnerabilities that underlie SCR while considering the role of resilience practices. Hence, this study aims to overcome this knowledge gap and put forward a practice-based framework based on empirical evidence by addressing the following research questions (RQs):
RQ1.
How does AM technology adoption affect different resilience practices?
RQ2.
How do AM-enabled resilience practices affect different SC capabilities and vulnerabilities that underlie SCR?
Moreover, by drawing on the answers to the mentioned RQs, propositions are put forward to address the following RQ:
RQ3.
What opportunities would AM technology adoption present for enhancing SCR via different resilience practices?
The remainder of this paper is organized as follows. In section two, the theoretical background is presented and the theoretical perspective leading to the conception of the research model is discussed. In section three, the research methodology is described. In section four, the results are discussed and compared with the literature to derive the theoretical implications of the study, which are summarized in the form of propositions. Subsequently, in section five, a practice-based framework is put forward that outlines the managerial implications of the study. Finally, in section six, conclusions are drawn and directions for future research are suggested.
2. Theoretical background
2.1. Additive manufacturing and supply chain resilience
The adoption of digital technologies brings about fundamental changes in the SC (Dolgui and Ivanov Citation2020), ‘and SCR in particular’ (Ivanov, Blackhurst, and Das Citation2021). AM is a digital technology that uses raw materials and computer-aided design (CAD) software to produce objects layer by layer on a build platform (ISO/ASTM 52900 Citation2021). The ability to produce physical objects directly from raw materials using digital design files renders AM a ‘direct digital manufacturing’ technology (Ford and Despeisse Citation2016).
Recently, AM has received considerable attention from the industry and the research community, mainly due to its ability to support supply chain management (SCM) practices (Zouari, Ruel, and Viale Citation2020), particularly resilience practices aimed at dealing with SC vulnerabilities and enhancing SCR (Balakrishnan and Ramanathan Citation2021; Naghshineh and Carvalho Citation2022b). For instance, with the outbreak of the COVID-19 pandemic, AM has been used globally in response to ongoing shortages in the medical SC, relieving strained healthcare systems around the world (Kunovjanek and Wankmüller Citation2020). An important use case of AM during this period was displayed by Formlabs (a 3D printing technology developer and manufacturer headquartered in the US), which launched a support network by pooling multiple 3D printers in different locations to address the critical shortage of medical parts and devices. One of the main adoption features of AM technology that has made it successful in dealing with this problem is the distributed manufacturing of medical parts and devices close to their point of use (Verboeket et al. Citation2021). This AM adoption feature would allow manufacturers to considerably reduce the delivery lead times and costs of medical parts and devices, thus improving the resilience of the medical SC (Durão et al. Citation2017; Verboeket et al. Citation2021).
As a resilience practice, reducing delivery lead time augments adaptability (Carvalho et al. Citation2022), which is the capability of the SC to swiftly readjust and respond to arising SC vulnerabilities (Pettit, Croxton, and Fiksel Citation2013; Pettit, Fiksel, and Croxton Citation2010). This is an instance where the adoption of AM technology enables firms to exercise a resilience practice (i.e. reduce delivery lead time) to develop an SC capability (i.e. adaptability), which is necessary for reducing the vulnerability of the SC to disruptions, therefore enhancing SCR (Carvalho et al. Citation2022; Naghshineh and Carvalho Citation2022b).
In this work, SCR is considered a higher-order construct composed of a set of SC capabilities that are necessary to deal with SC vulnerabilities (Pettit, Croxton, and Fiksel Citation2013, Citation2019; Pettit, Fiksel, and Croxton Citation2010; Ponomarov and Holcomb Citation2009). This consideration is based on the seminal works of Pettit et al. (Citation2010, Citation2013), who define SCR as an exhaustive set of interlinked SC capabilities and vulnerabilities. According to Pettit et al. (Citation2010), ‘supply chain resilience increases as capabilities increase and vulnerabilities decrease’. Moreover, they state that ‘linkages exist between each vulnerability and a specific set of capabilities’, which can be controlled to improve SCR.
2.2. Research gap
The authors critically reviewed empirical studies that imply adopting AM technology contributes to building SC capabilities by enabling certain resilience practices (). However, no empirical study was found that explicitly delineated the effects of AM technology adoption on different resilience practices, explaining how various SC capabilities and vulnerabilities that underlie SCR would be affected as a result. Hence, based on the following two premises: a) adopting digital technologies such as AM ‘will encourage the firm’s supply chain resilience practices’ (Balakrishnan and Ramanathan Citation2021); and b) ‘resilience practices are a means of achieving SC capabilities’ (Carvalho et al. Citation2022) for dealing with SC vulnerabilities and enhancing SCR (Pettit, Fiksel, and Croxton Citation2010, Citation2013,Citation2019; Ponomarov and Holcomb Citation2009), this study aims to explore how AM technology adoption affects different resilience practices (RQ1), and how these practices would, in turn, affect different SC capabilities and vulnerabilities that underlie SCR (RQ2). Subsequently, an empirical framework together with propositions will be put forward, reflecting the opportunities that AM technology adoption would present for enhancing SCR via different resilience practices (RQ3). Given these considerations, the research model is conceptualized in . It should be noted that the arrows in this model do not represent cause-and-effect relationships, but rather the effect that each research variable has on the other.
Table 1. Resilience practices and SC capabilities in the context of AM adoption and SCM.
To control the scope of this study, nine resilience practices and their metrics were selected from Carvalho et al. (Citation2022) (), which proved to be more relevant in the context of AM technology adoption and SCM (see ). The metrics were used as observable items to collect data from the industry and empirically examine how AM adoption affects different resilience practices aimed at building different SC capabilities for dealing with SC vulnerabilities, thus enhancing SCR. The definitions for the identified SC capabilities and vulnerabilities along with their subfactors, which define each SC capability/vulnerability in more detail, are available in Appendix A.
Table 2. Resilience practices and their metrics adapted from Carvalho et al. (Citation2022).
2.3. Theoretical lens
From a theoretical perspective, the dynamic capabilities view is employed to investigate the subject matter. The use of established theoretical perspectives helps to identify the underlying relationships between the research variables and contributes to the generalizability of the findings (Walker et al. Citation2015). The most established theoretical lenses to view the concept of SCR are the resource-based view and dynamic capabilities view (Kochan and Nowicki Citation2018; Tukamuhabwa et al. Citation2015).
While the resource-based view regards technology adoption as a resource for improving the firm’s performance (Wernerfelt Citation1984), it fails to consider the dynamic nature of the SC in which the firm operates (Cavusgil, Seggie, and Talay Citation2007; Towill Citation1991), which is particularly important in the context of SCR (Ponomarov and Holcomb Citation2009). This shortcoming is addressed by the dynamic capabilities view, whereby investing in resources (e.g. technology adoption) and deploying the necessary SCM practices (e.g. resilience practices) are considered in conjunction with the dynamic nature of the SC to achieve distinctive capabilities that help the firm gain a sustainable competitive advantage (Ponomarov and Holcomb Citation2009; Teece, Pisano, and Shuen Citation1997). In other words, from the dynamic capabilities perspective, investing in technology adoption (e.g. AM adoption) is an SCM strategy for developing SC capabilities and gaining a competitive advantage over other players in the market, especially in dynamic SC environments that are constantly under pressure due to impending SC vulnerabilities (Kalaitzi et al. Citation2019; Teece Citation2014).
Relevantly, by enabling resilience practices, AM technology adoption helps to develop the necessary SC capabilities to deal with SC vulnerabilities. This assertion is corroborated by Balakrishnan and Ramanathan (Citation2021) who posit that adopting digital technologies such as AM ‘will encourage the firm’s supply chain resilience practices’, and Carvalho et al. (Citation2022) who state that ‘resilience practices generate the capabilities that the SC needs to reduce the severity of disturbances as well as the recovery time while keeping the costs at a reasonable level’.
Unlike many studies using the resource-based view and dynamic capabilities view that fall short of identifying processes, resources, and pathways to develop SC capabilities (Chowdhury and Quaddus Citation2017), this study aims to put forward a preliminary Additive Manufacturing-Supply Chain Resilience practices (AM-SCRP) framework that allows the identification of suitable pathways to building SC capabilities for mitigating SC vulnerabilities via deploying AM-enabled resilience practices, thus enhancing SCR.
3. Research methodology
3.1. Research design and instrumentation
In this study, exploratory survey research was employed as it is particularly useful at ‘the early stages of research into a phenomenon, when the objective is to gain preliminary insight on a topic’ (Forza Citation2002), especially in the area of operations and SCM. As Forza (Citation2002) states, ‘Descriptive and exploratory survey research are important and widely used in OM [Operations Management]’. Contrary to case studies and interviews, this research method allows targeting larger samples to gain preliminary insights and is more likely to lead to the participation of experts who are not easy to get hold of (Babbie Citation1990).
This study implements a mixed-methods exploratory survey research design similar to that of Durach et al. (Citation2017), who investigated the impacts of AM technology adoption on the SC. They used a combination of Likert scale questions and text fields to collect both quantitative and qualitative data in the form of expert perceptions and explanations. Similarly, in this study, experts with experience in the fields of AM technology and SCM were targeted for collecting quantitative and qualitative empirical data. Likert scale questions and open-ended text fields were used to capture the experts’ perceptions and explanations of how AM technology adoption affects the resilience practices under study (see ). Furthermore, these data were used to explicate how AM technology adoption affects different SC capabilities and vulnerabilities that underlie SCR via these resilience practices, thus affecting SCR.
The survey questionnaire (see Appendix B) included general questions regarding the country, industry sector, SC tier, etc., as well as questions regarding the AM processes and applications that the companies developed. To devise the Likert scale questions, the empirically well-grounded metrics representing the resilience practices in Carvalho et al. (Citation2022) were used. These questions were based on a five-point scale (where 1 signified ‘Very low effect’ and 5 signified ‘Very high effect’) aimed at assessing the experts’ perceptions of the extent to which the use of AM technology affects different resilience practices in their SC operations. Moreover, open-ended text fields were provided right after each Likert scale question, allowing the experts to explain the reasons for their Likert scale responses. The questionnaire was first reviewed by an expert and an academic in the field and then pilot tested in multiple companies that were using AM technology. After making improvements based on their feedback, the questionnaire was administered online using Google Forms, which was accompanied by a cover letter describing the purpose of the survey and ensuring the anonymity of the experts and their companies.
3.2. Sampling and data collection
To investigate the subject matter from the industry’s viewpoint, an exploratory survey was conducted among experts who were involved in industries with more experience in using AM technology. According to the report by Wohlers (Citation2021): ‘3D printing and additive manufacturing global state of the industry’, AM is widely adopted by the automotive, aerospace, energy, and medical industries, among others. Moreover, according to Tortorella et al. (Citation2022), conducting ‘cross-sectional survey-based studies’ with ‘multiple respondents from several SC sectors and tiers’ tends to yield a more holistic view of the contribution of Industry 4.0 technologies such as AM to SCR. This is corroborated by Patil et al. (Citation2023), who explicitly state that they selected a heterogeneous sample of experts from several industries ‘to allow for the emergence of alternative explanations’ and ‘capture a range of viewpoints’ concerning the contingent adoption of AM technology in different SCs.
Therefore, to find a heterogeneous sample of experts who worked for companies in the aforementioned industries, the expert sampling method was employed using the LinkedIn professional network platform. It is worth noting that the LinkedIn platform is already used for expert sampling by relevant works in this field, e.g. Kurpjuweit et al. (Citation2021). Using this sampling method, professionals with expertise in the AM and SCM fields were found worldwide in the aforementioned industries, who otherwise would have been very difficult to find via probability sampling techniques. Different search keywords (e.g. ‘Production manager’, ‘Operations manager’, ‘Managing director’, ‘Supply chain manager’, etc., together with ‘Additive manufacturing’ and ‘3D printing’) were used to search for registered professionals on the LinkedIn platform. The search returned 417 expert profiles, of which 400 were selected after reviewing the accuracy of the search results. This selection process was performed by carefully considering the evidence in each profile (e.g. work experience, skillset, role in the company, educational background, licenses and certifications) to ensure that the expert had knowledge and experience in using AM technology. The experts in the sample had an average working experience of 9 years in the AM field (mainly in managerial positions), indicating an appropriate level of experience for taking part in the survey.
Subsequently, 400 invitations accompanied by a brief description of the survey and a weblink to the online questionnaire were sent out over the course of December 2021. Completed questionnaires were sent back from mid-December 2021 until the end of January 2022. After sending reminders to late responders during this period, 69 completed questionnaires were returned, corresponding to a response rate of 17.3%. However, 9 questionnaires were discarded because they contained multiple unanswered questions and unfilled text fields, resulting in 60 usable questionnaires. This sample size is similar to the sample sizes in exploratory surveys performed by Hübner et al. (Citation2016) and Kembro and Norrman (Citation2022), which are 60 and 50 respectively.
To ensure that the derived sample possessed the characteristics of the intended target population, its descriptive analysis was compared with the aforementioned report by Wohlers (Citation2021), which indicated a high level of similarity. For instance, the reported percentages in regarding the industry sector of the companies were found to conform with the diffusion of AM technology adoption among different industries reported by Wohlers (Citation2021). Furthermore, the derived percentages in regarding the adopted AM processes and their applications in the sample were also benchmarked against the report by Wohlers (Citation2021), which resulted in a high level of similarity as well. Hence, this comparison helped validate the representativeness of the derived sample, which can be prone to researcher bias when using the expert sampling technique (Sharma Citation2017).
Table 3. Descriptive analysis of the sample.
Table 4. Descriptive analysis of the AM processes and their applications in the sample.
Moreover, as shown in , the sample includes a ‘diverse range of participants and companies, which could lead to more robust findings and a more in-depth understanding of the subject’ (Belhadi et al. Citation2022). This is also endorsed by Tortorella et al. (Citation2022) who recommend conducting ‘cross-sectional survey-based studies’ at the interface of Industry 4.0 technologies such as AM and SCR ‘with multiple respondents from several SC sectors and tiers’. Along the same lines, as the sample is composed of companies operating in rather developed economies, the findings are likely to be robust, as Industry 4.0 technologies such as AM are more mature in developed economies (Wagire et al. Citation2021). This is also in line with Belhadi et al. (Citation2022), who suggest that the ‘exploration of AM technology adoption in developed nations’ is an important avenue for research.
3.3. Data analysis method
To understand how AM adoption affects the resilience practices under study, initially, the average scores of the experts’ quantitative answers to the Likert scale questions were computed. Similar to Durach et al. (Citation2017), Jiang et al. (Citation2017), and Ukobitz and Faullant (Citation2021) among others, this study employs this descriptive statistical analysis method to project experts’ perceptions regarding the subject matter. Likewise, to estimate to what degree the experts agreed on the subject matter, the level of dispersion in the answers was considered by calculating the standard deviation (SD). It should be noted that before proceeding with the analysis of the Likert scale responses, Cronbach’s α was used to assess the reliability of the scales (Cronbach Citation1951). The Cronbach’s α was 0.89, surpassing the widely accepted value of 0.70, and therefore confirming the reliability of the scales (O’Leary-Kelly and Vokurka Citation1998).
Next, to explain how AM adoption affects the resilience practices under study, consequently affecting the SC capabilities and vulnerabilities that underlie SCR, a two-step qualitative data analysis process was performed. To begin this process, specific research dimensions (i.e. coding criteria) were required to help analyze and summarize the experts’ qualitative explanations based on the same underlying criteria (Miles and Huberman Citation1994). To this end, the AM-SCR framework by Naghshineh and Carvalho (Citation2022a) was used as a) it provides a comprehensive list of AM adoption features and barriers that affect the metrics representing the resilience practices in this study, and b) it provides a comprehensive list of SC capabilities and vulnerabilities that are related to these metrics.
In the first step of this process, the AM-SCR framework was used to code the explanations. For example, the explanation ‘Producing on site and on demand means no transportation costs and shorter delivery lead times’ (industry expert) was coded as ‘Distributed manufacturing’, which, according to the AM-SCR framework, is an AM adoption feature. This explanation was provided by an expert to complement his Likert scale response on how AM affects the metric ‘Ease (cost) of reducing delivery lead time’, which represents the resilience practice ‘Reduce delivery lead time’ (see ). In the second step, the AM-SCR framework was used to identify the SC capabilities and vulnerabilities that would be affected by the resilience practices under study. For example, according to the AM-SCR framework, ‘Distributed manufacturing’ enhances the ‘Adaptability’ of the SC by reducing delivery lead time, thus mitigating the vulnerability of the SC to ‘Turbulence’ that is caused by ‘Unpredictability in customer demand’.
This two-step process is exemplified in , which illustrates how the aforementioned research dimensions enabled the analysis of the experts’ explanations to explicate how AM technology adoption affects the resilience practices under study, consequently affecting the SC capabilities and vulnerabilities that underlie SCR. Additionally, supporting evidence from the literature (i.e. secondary data) was sought to triangulate the findings. For instance, the excerpt shown in lends support to the validity of the mentioned example in .
Figure 3. Text segment from Durão et al. (Citation2017).

This two-step process allowed the authors to code, sort, and display the qualitative data in a structured manner and draw conclusions accordingly (more illustrative examples are available in Appendix C). This process was performed multiple times to ensure the relevance and transparency of the findings (Miles and Huberman Citation1994). Subsequently, multiple pathways were explored that originate from AM adoption and lead to interlinked SC capabilities and vulnerabilities via the resilience practices under study, which will be discussed in the following section.
4. Results and discussion
The results of the quantitative data analysis (Likert-scale responses) are presented in . In the subsections that follow, the effects of AM adoption on each resilience practice and the ensuing implications for SC capabilities and vulnerabilities that underlie SCR are discussed based on the employed qualitative data analysis. In addition, propositions are put forward at the end of each subsection that summarize the theoretical implications of the study, proposing the potential opportunities that AM adoption would present for enhancing SCR via different resilience practices.
Table 5. Quantitative data analysis results.
4.1. Change the production schedule
The experts’ answers revealed that AM adoption has the highest effect on the ease (in terms of cost and time) of changing production schedules. Moreover, the dispersion in the quantitative answers was the lowest, indicating a consensus among the experts regarding the enhancing effect of AM on this resilience practice. This effect was attributed to different AM features, among which fast production setup and tool-less manufacturing were the most pronounced features. Some experts explained that production setup through AM is rather fast and easy, providing the flexibility to quickly change the production plans to meet erratic customer demands without incurring high costs. ‘Highly agile, fast to program and start a job’ (industry expert). Also, it was noted that the tool-less nature of AM further enhances this flexibility and allows manufacturers to quickly switch their printers to different products to manage sudden changes in customer demands. ‘We commonly switch the machines to different products to manage changes in demand’ (industry expert) is an illustrative explanation in this regard.
Another mentioned AM feature was the need for fewer materials and inventory compared to conventional manufacturing methods (CM), making it easier to change the production schedules, thus enhancing the flexibility to fulfill erratic customer orders on short notice. For instance, according to an expert, ‘BOM [bill of materials] is first level (raw materials only)’, meaning that there are fewer materials and inventory in AM settings. This is because only raw materials and 3D models in the form of digital files (e.g. digital inventory) are required as input to print a complex part, whereas in CM, the BOM is normally composed of different components and subassemblies (i.e. multi-level BOM).
Given these AM features, a demand-pull SCM strategy can be deployed where production is postponed until customer demand is materialized (i.e. Flexibility (Production postponement)). In this way, it will be easier to deal with erratic customer demands (i.e. Turbulence (Unpredictability in customer demand)) as well as potential customer disruptions (i.e. Supplier/Customer Disruptions (Customer disruptions)) by being able to change the production schedules quickly.
However, certain barriers were also mentioned that inhibit the enhancing effect of AM adoption on changing production schedules. Slow production speed and low throughput rate (i.e. the rate at which parts can be printed from start to finish) were noted to hinder the timely changing of production plans by eroding the processing times, thus making it difficult to respond to sudden changes in customer demands. Excessive post-processing was mentioned as another barrier that takes away from AM’s agility to quickly fulfill customer orders. For instance, an expert noted, ‘overall is less agile if the part requires complex machining and other post processes’. Another mentioned barrier is the present instability in AM processes that can hinder the timely completion of the production plans. One of the experts explained that production planning is rather easy with AM, especially when compared to CM; however, unsteady AM processes may cause problems. Lastly, information and communication technology (ICT) inadequacies were identified as another barrier. For instance, an expert noted that ‘there is no good MES [Manufacturing Execution Systems] solution quite yet in the market.’ MES provides ‘a common user interface and data management system’ (Saenz de Ugarte, Artiba and Pellerin Citation2009), helping to establish proper data management, thus facilitating AM operations.
Proposition 1.
AM adoption has a high positive effect on changing the production schedules, which enhances the flexibility in order fulfillment. This mitigates the unpredictability in customer demands as well as customer disruptions.
4.2. Reduce delivery lead time
Reducing delivery lead time is the second resilience practice that is highly affected by AM adoption. The dispersion in the quantitative answers was relatively low, implying general agreement among the experts. Primarily, emphasis was placed on the distributed manufacturing and on-demand manufacturing of parts. As two of the experts explained respectively, ‘Producing on site and on demand means no transportation costs and shorter delivery lead times’ and ‘AM is much cheaper and faster than traditional methods, especially as it cuts transportation costs and times.’ Since the need for the distribution and delivery of parts is reduced via these AM features, adaptability (i.e. Adaptability (Lead time reduction)) will increase against shortages in distribution capacity (i.e. Resource limits (Distribution capacity)) as well as sudden changes in customer demands that may necessitate faster delivery lead times.
Furthermore, design freedom and part consolidation were two other interrelated AM features pointed out by the experts. One expert explained that ‘Delivery lead time is reduced not just due to reduced manufacturing lead time, but also due to reduced part development lead time powered by the high degree of additive manufacturing design freedom’, whereas another expert explained that ‘With AM lead times for products can be reduced due to fewer parts and elimination of many assembly processes.’ Therefore, not only will SC adaptability increase against possible distribution capacity shortages and erratic customer demands, but there will also be fewer cases of inventory theft and damage (i.e. Deliberate Threats (Piracy and theft)). Moreover, rapid prototyping of some parts with AM was noted to reduce delivery lead time. For instance, one expert explained that AM is a ‘Good option to reduce the lead time for prototype components (i.e. 3D printed samples can simulate airbags, sub-components or vehicle parts and be used for testing purposes).’ In this way, the manufacturing firm can go through multiple iterations during the product development phase, allowing it to quickly develop and deliver complex products that conform with customer demands, hence increasing the adaptability of the SC to erratic customer demands.
Despite all the highlighted advantages that AM adoption presents for reducing delivery lead time, excessive post-processing requirements can act as a barrier. For instance, an expert noted that ‘Lead time is longer if machining is required.’ Under this circumstance, adaptability to changes in customer demands becomes rather difficult since post-processing steps can be costly and time-consuming.
Proposition 2.
AM adoption has a high positive effect on reducing delivery lead time, which enhances adaptability. This mitigates limited distribution capacity, unpredictability in customer demands, and piracy and theft.
4.3. Use maximum capacity
The answers indicated that AM adoption has a high effect on the ease (in terms of cost and time) with which the adopting firm can adjust its production capacity. The derived SD score indicated that there was consensus among the experts regarding the enhancing effect of AM on the use of maximum capacity. This effect was mainly attributed to the possibility of manufacturing parts/products on demand and in small batches. Multiple experts mentioned that it is easy to change the production capacity when needed, e.g. ‘It is not a problem to adjust production to closely match demand’ or ‘We can scale up production capacity very quickly when needed’. It was noted that as an automated manufacturing method, AM can bring about efficiency, e.g. ‘Automated system. The same number of operators can operate more machines’ (industry expert), supporting the notion of economies of technology whereby it is possible to operate multiple machines by a single operator (Tuck, Hague, and Burns Citation2007), thus generating outputs with minimal resource requirements (i.e. Efficiency (Asset utilization)). Hence, these AM adoption features would allow the manufacturer to adjust its production capacity efficiently (i.e. without incurring high costs and time) to address the unpredictability in customer demand.
Nevertheless, limited build volumes and low throughput rate were identified as two interrelated barriers that inhibit the enhancing effect of AM adoption on utilizing maximum production capacity. One expert explained that ‘The change of the production capacity is only easy up to the maximum building chamber volume. If more parts are needed, the job preparation and the post material recycling steps make it difficult’, drawing attention to AM’s low throughput rate as a barrier, which is mainly caused by the limited build volumes of the 3D printers. Additionally, the lack of multi-material printing was considered to make the job preparation somewhat challenging. ‘If machines are dedicated to a particular material but are flexible in processing other materials, time and labor must be spent to clean out the old material, change machine parameters, run tests, etc. before capacity can be modified using the new desired material’ (industry expert). This AM barrier is also mentioned by Eyers et al. (Citation2018), where all the cases in their study considered material loading a labor-intensive task. This condition is exacerbated by frequent material changeovers since extensive cleaning of the printers becomes necessary before a different type of material can be loaded into them. Lastly, it was noted that the high cost of AM processes, especially in Metal AM, makes it difficult to scale up the production capacity cost-efficiently to fulfill customer orders that are placed at short notice. According to an expert, ‘Metal AM technology is still very expensive, and it’s difficult to justify increasing capacity for short run projects and demand variability’. Another reason for this issue was mentioned to be the high cost of industrial-grade AM machines. As one expert explained, ‘Industrial machines are expensive. So it is not easy to scale up and not cheap either. Making a decision to install new machines can take time.’
Proposition 3.
AM adoption has a high positive effect on the use of maximum capacity, which enhances efficiency. This mitigates the unpredictability in customer demands.
4.4. Reduce response time
The quantitative answers indicated that AM adoption has a high effect on the ease with which production lead time can be reduced. Moreover, the derived SD indicated consensus among the experts’ opinions. Rapid prototyping and rapid manufacturing of parts were highlighted as prominent AM features that help reduce production lead time. One expert noted that ‘Rapid design iteration with additive gets to functional components faster.’ These AM features not only enable the adopting firm to quickly develop new products to respond to unpredictable customer demands but also help it to deal with competitive innovations that stem from fierce competition in the market (i.e. External pressures (Competitive innovation)). This is especially true when viewed from the dynamic capabilities perspective, where the firm needs to constantly adapt to rapidly changing markets by developing new products to gain a competitive advantage (Leonard‐Barton Citation1992; Teece, Pisano, and Shuen Citation1997). Furthermore, tool-less manufacturing via AM leads to a fast turnaround time, which is the time needed to design, print, and post-process a part. ‘No tooling means fast production turnaround’ (industry expert). Apart from these AM features, attention was also drawn to fast production setup and part consolidation, both of which contribute to reducing the response time. ‘Faster and cheaper to build and install new AM production line’ and ‘Simplified and faster production process due to reduction of parts in subassemblies’ were among the explanations given by different experts that refer to these AM features. By reducing response time, these AM features boost the adaptability of the SC to develop new customized products that comply with erratic customer demands.
Nevertheless, some barriers exist that take away from AM’s enhancing effect on reducing response time. Excessive post-processing was pointed out by some experts. One expert explained that ‘the production time is rather comparable to conventional manufacturing, since AM also includes significant post-processing.’ Slow production speed was implicitly mentioned by another expert, who stated that ‘New machines are especially much more performant and quicker.’ Moreover, unstable AM processes hinder reducing the response time. According to an expert, ‘AM may or may not save production time. It depends on the process, the materials, postprocessing steps, etc.’ In other words, some AM processes are not completely reliable, which may lead to unforeseen failures that disrupt the firm’s production operations (i.e. Turbulence (Unforeseen technology failures)), and as a consequence prolong the production lead time. Finally, the high cost of industrial-grade AM machines was mentioned multiple times. For instance, one expert noted that ‘The lower production time is offset by the higher machine costs.’ In summary, these barriers can undermine AM’s ability to reduce production lead time to quickly adapt and respond to unpredictable customer demand.
Proposition 4.
AM adoption has a high positive effect on reducing response time, which enhances adaptability. This mitigates the unpredictability in customer demands and competitive innovations. However, unforeseen technology failures may deteriorate due to the present instability in AM processes.
4.5. Supply chain common response
Overall, the experts perceived the effect of AM technology adoption on the degree of cooperation with other firms in the SC to be moderate. Close collaboration was mentioned in the experts’ explanations multiple times. There were several explanations similar to ‘We cooperate very frequently and at a high level with both customers and suppliers.’ (industry expert), supporting the notion that the adoption of AM technology requires close collaboration with suppliers and customers throughout the SC. Apart from generating new ideas based on the customers’ requirements, which helps the adopting firm to maintain its market position (i.e. Market position (Customer relationships)) against external pressures stemming from competitive innovations, close collaboration with partners in an AM SC can also help the firm to deal with other SC vulnerabilities such as unpredictability in customer demands as well as potential customer or supplier disruptions (i.e. Supplier/Customer Disruptions (Supplier disruptions)), particularly through AM-enabled postponement of orders (i.e. Collaboration (Postponement of orders)). This corroborates the findings of Naghshineh and Carvalho (Citation2022b), who identify close collaboration with SC partners as a practice aimed at mitigating different SC vulnerabilities, e.g. supplier capacity limitations. Moreover, close collaboration with SC partners aimed at developing AM technology can help avoid quality problems that may cause SC vulnerabilities such as customer disruptions. ‘We are collaborating on technology development to ensure we are getting the right quality’ (industry expert). Similarly, an expert explained that ‘Cooperation with business partners is very important. Especially before and after every transaction (raw material acquisition and post processing).’
Along the same lines, consumer-centric production and co-design/co-creation were explained as two other AM features, both of which are likely to have similar implications in terms of SC capabilities and vulnerabilities. Through a consumer-centric business model based on co-design/co-creation, the firm has better prospects of maintaining a stronger and longer-term relationship with its customers, especially as these AM features can help the firm to better deal with erratic customer demands and provide products that properly fit customer needs, thus avoiding potential customer disruptions. For the most part, the findings indicate that AM technology adoption leads to a more integrated SC by promoting cooperation between SC members (i.e. the adopting firm, suppliers, and customers).
With respect to the existing barriers, complications regarding intellectual property (IP) rights were mentioned several times by the experts. For instance, ‘Could be higher but there are constraints about IP’ (industry expert). This is the case since many firms are concerned with infringements that may occur after sharing sensitive information (e.g. 3D models and design data) with other SC members. It was noted that the lack of methods and regulatory frameworks (e.g. copyright frameworks, patents, trademarks, and licensing) for dealing with potential infringements exacerbates this situation. Moreover, a lack of trust in AM was mentioned as a barrier that hinders SC common response. As one expert noted, ‘There are still some doubts regarding AM in many industries that require more cooperation’. Lastly, the lack of consumer awareness and acceptance was pointed out as another barrier by the experts. These barriers negatively affect the level of cooperation among SC members, thus reducing the capability of the SC to meet customer demands.
Proposition 5.
AM adoption has a moderate positive effect on practicing SC common response, which improves market position and collaboration. This mitigates competitive innovations, unpredictability in customer demands, and supplier/customer disruptions.
4.6. Align supply chain information systems
The experts’ answers indicated that AM has a moderate effect on the ease (in terms of cost and time) with which SC partners can exchange reliable and timely information. The dispersion in the Likert scale responses was rather high, implying different opinions among the experts. Many experts emphasized the possibility of sharing information using digital files in an AM setting (i.e. digital file distribution) and making inventory management easier using digital inventory instead of finished goods inventory (FGI). These AM features improve the visibility of the SC by facilitating the exchange of information between SC partners (i.e. Visibility (Information exchange)). Therefore, through improved SC visibility, it would be easier to interact with other SC members, e.g. outsourcing production (i.e. Connectivity (Degree of outsourcing)). Moreover, this improvement in SC visibility would also help mitigate supplier disruptions and the unpredictability in customer demand.
AM technology adoption also facilitates inventory management due to its compatibility with different information systems. According to an expert, ‘Since AM is a digital process, it is amenable and compatible with other digital technologies for sharing information: MES [Manufacturing Execution System], MRP [Material Requirement Planning], ERP [Enterprise Resource Planning], etc.’ This flexibility in inventory management (i.e. Flexibility (Inventory management)) is likely to facilitate demand forecasting (i.e. Anticipation (Demand forecasting)), especially when dealing with unpredictability in customer demands that can lead to other SC vulnerabilities such as production capacity shortages (i.e. Resource limits (Production capacity)).
Some experts considered the digital nature of AM to be a contributing factor to information exchange. Therefore, by taking advantage of AM’s digital nature, it would be easier to participate in exchanging information with other SC members (i.e. Collaboration (Collaborative information sharing)) and perform collaborative forecasting (i.e. Collaboration (Collaborative forecasting)) to deal with unpredictability in customer demands and customer disruptions. Lastly, it was noted that AM-enabled distributed manufacturing may as well serve as an incentive for SC members to align their information systems. As one expert noted, ‘One design already produced in the US can be replicated in Europe in real time, if the same equipment is available, or in a few days with different equipment.’
However, despite all the mentioned advantages, some experts expressed concerns about potential IP rights complications. Two experts mentioned that ‘Too much is at stake in terms of IP’ and that there are ‘IP restrictions.’ This impediment negatively affects the capability of the firm to share information with other SC members and perform tasks such as collaborative forecasting, turning continuous information flow for everyday operations into an SC vulnerability (i.e. Connectivity (Reliance upon information flow)). Also, this situation leaves the firm’s technology and products vulnerable to industrial espionage (i.e. Deliberate threats (Industrial espionage)). Another relevant barrier in many AM SCs is inadequate ICT. ‘We still have to work on streamlining the information flow between the sites’ is an explanation given by an expert that draws attention to this barrier. Furthermore, ICT inadequacies can lead to cybersecurity issues, increasing the risk of data breaches. Thus, in the absence of adequate ICT, connectivity issues may arise that undermine the capability of the firm to exchange reliable and timely information with SC partners, therefore causing SC vulnerability to reliance on information flow.
Proposition 6.
AM adoption has a moderate positive effect on aligning SC information systems, which enhances visibility, anticipation, flexibility in order fulfillment, and collaboration. This mitigates the degree of outsourcing, supplier and customer disruptions, unpredictability in customer demands, and production capacity shortages. However, reliance on information flow and industrial espionage may deteriorate due to potential IP rights complications and ICT inadequacies.
4.7. Use alternative suppliers
The quantitative answers indicated that the effect of AM technology adoption on the use of alternative sources of supply is moderate. There was no considerable dispersion in the answers, implying general agreement between the experts. Although no particular AM features emerged from the explanations, multiple AM barriers were detected that inhibit the number of available alternatives for sources of supply.
The most pronounced barrier that emerged from the explanations was the limited variety and supply of AM materials and industrial-grade machines. ‘There is a high dependency on a small number of suppliers in the industry, which means we are single sourcing materials and machines. This is something we are working to change, as it means high risk of supply’ and ‘A stable delivery network (every time more than one supplier for each material) is necessary for additive manufacturing’ are two illustrative explanations provided by different experts in this regard. According to an expert, this situation is further exacerbated when AM machines are supplied as closed-source systems (vs. open-source systems), which can only work with specific materials. ‘Too many closed systems do not allow for a variety of feedstock suppliers’ (industry expert). Moreover, as some experts noted, the AM market is still young and many patents protect the suppliers by forcing customers to only work with them (i.e. vendor/customer lock-in). ‘In my opinion, same as other technologies/fields. Even less I might say as the market is young and still many patents protect suppliers and force you to work with them’ (industry expert). Altogether, the limited variety and supply of AM materials and industrial-grade machines reduce the sourcing flexibility of the manufacturing firm as there are only few alternative suppliers available (i.e. Flexibility (Alternate suppliers)). This situation can give rise to interrelated SC vulnerabilities such as a shortage of production capacity at the manufacturing firm due to a shortage of raw materials (i.e. Resource limits (Raw material availability)) or machines caused by the limited number of suppliers. In other words, this situation creates a high degree of dependence on the capacity of a limited number of suppliers (i.e. Resource limits (Supplier capacity)) to provide the necessary input materials and machines for production to take place at the manufacturing firm (i.e. Connectivity (Reliance upon specialty sources).
The second most pronounced barrier was the lack of regulation and standardization of AM materials. ‘Raw material supply is maturing but qualification presents a barrier to switching suppliers quickly’ (industry expert). The present lack of regulation and standardization necessitates extensive quality assurance (QA) and quality control (QC) procedures to be carried out by manufacturers in-house, which can become a burdensome task. Moreover, the lack of regulation and standardization makes the traceability of AM materials difficult. Thus, the traceability of AM materials should be ensured by manufacturers, which according to an expert compels them to ‘audit and validate the suppliers in more detail’. Multiple experts also mentioned the high cost of AM materials as a barrier. These barriers can also reduce the sourcing flexibility of raw materials, causing the same SC vulnerabilities that were discussed earlier in this subsection. For instance, the lack of regulation and standardization of AM materials coupled with their limited traceability can make multiple-sourcing difficult and lead to dependency on a limited number of suppliers whose materials meet the quality standards of the manufacturing firm, while the high cost of AM materials can limit the production capacity of the manufacturing firm.
Furthermore, some experts remarked that the high cost of industrial-grade AM machines is another barrier that makes multiple-sourcing even more difficult. It was also noted that the lack of AM process standards turns the qualification of new machine suppliers into a rigorous task. These barriers can also give rise to SC vulnerabilities to production capacity as well as reliance on a limited number of qualified machine suppliers.
Proposition 7.
AM adoption has a moderate negative effect on the use of alternative suppliers, which diminishes the flexibility in sourcing, causing vulnerabilities to raw materials availability, supplier capacity, production capacity, and reliance on specialty sources.
4.8. Use alternative production sites
The effect of AM adoption on the use of alternative production sites was perceived to be low, with moderate dispersion in the experts’ quantitative answers when compared to other resilience practices. Distributed manufacturing was the most pronounced AM feature that enables the use of alternative production sites. It was noted that ‘At the cost of a printer and few other ancillary equipment, production can be set up almost anywhere’ (industry expert), supporting the notion of local manufacturing whereby it is possible to gain access to geographically dispersed production facilities. An important implication of this AM-enabled resilience practice is the capability to distribute production facilities at different locations (i.e. Dispersion (Distributed capacity)), hence avoiding the concentration of production capacity in one location (i.e. Sensitivity (Concentration of capacity)). This also helps mitigate the risk of deliberate misconduct such as terrorism and sabotage (i.e. Deliberate threats (Terrorism and sabotage)), as well as piracy and theft. According to an expert, ‘If one production site is down, we are able to divert production to another site.’ In other words, the firm will have the flexibility (i.e. Flexibility (Reallocation of production)) and the adaptability (i.e. Adaptability (Rerouting of requirements)) to adjust its operations promptly and gain access to adequate production and distribution capacities to fulfill and deliver the customer orders in the face of disruptions. For instance, mobile manufacturing by means of AM technology, which is intrinsically another form of AM-enabled distributed manufacturing, can improve SCR in the mentioned ways. According to Stillström and Jackson (Citation2007), mobility in manufacturing is ‘the ability to change between geographically different places with little penalty in time, effort, cost, or performance’. This concept was evident in many of the explanations given by the experts.
Along the same lines, it was noted that ‘AM can enable local or regional production reducing the effects of delays in shipping and other disruptions’ (industry expert). This would enable the firm to protect itself against potential supplier/customer disruptions that may take place at different nodes across the SC. ‘Having a backup plant for additional capacity is fairly easy to setup’ (industry expert), implying the possibility of pooling the necessary production capacity from alternative production sites. Capacity pooling helps buffer the negative effects of disruptions (Khajavi, Partanen, and Holmström Citation2014), e.g. the ripple effect or bullwhip effect (Jain, Wadhwa, and Deshmukh Citation2009), thus increasing the manufacturing flexibility of the firm against unexpended surges in demand. As Ivanov et al. (Citation2019) state, ‘The core of additive manufacturing applications to SCM is the usage of 3D printers at different stages in the SC to increase manufacturing flexibility’.
However, explanations such as ‘More specialized production infrastructure is required for additive compared to subtractive’ or ‘Harder to invest due to higher infrastructure cost and capital cost’ by different experts revealed the lack of specialized infrastructure as a barrier that can impede the use of alternative production sites as a resilience practice, limiting production and distribution capacities in this case. An illustrative example of this issue is the underdeveloped ICT infrastructure of many firms for transferring AM digital files (Kumar et al. Citation2022; Verboeket and Krikke Citation2019). Also, the high cost of machine infrastructure for industrial-grade AM inhibits flexibility in production capacity. If the firm does not have a specialized infrastructure in place, one option is to outsource the production operations to AM service providers/bureaus. As one expert explained, ‘AM is becoming more mainstream and there is a good chance a company can have alternative production options’, which implies the possibility of outsourcing production to AM service providers/bureaus in different locations. This practice can open up opportunities for gaining access to markets that are geographically dispersed (i.e. Dispersion (Dispersion of markets)), thus expanding the scale and extent of the firm’s supply network (i.e. Connectivity (Scale and extent of supply network)) and establishing a globally distributed SC (i.e. Connectivity (Import/export channels)). In this instance, despite increased dependence on external entities (Patil et al. Citation2023), outsourcing production may still help the firm to mitigate potential supplier/customer disruptions.
Proposition 8.
AM adoption has a low positive effect on the use of alternative production sites, which enhances dispersion, flexibility, and adaptability. This mitigates concentration of capacity, terrorism and sabotage, piracy and theft, the scale and extent of supply network, import/export channels, supplier/customer disruptions, and production and distribution capacity shortages. However, when production is outsourced to AM service providers/bureaus, the degree of interdependence on external entities increases.
4.9. Use alternative transport modes
AM technology adoption was perceived to have the lowest effect on the use of different modes of transport, with the highest dispersion in the answers. The most noticeable AM features in the explanations were digital file distribution and digital inventory, both of which were considered to be enablers of distributed manufacturing. As one expert explained, ‘AM can eliminate the transportation of goods and materials since production could theoretically be located as close as possible to the point of use.’ He continued, ‘AM parts are represented by digital files so inventory can be digital too and production can take place on demand.’ This explanation emphasizes some key benefits of distributed AM, i.e. reduction of transportation by manufacturing on demand close to the point of use. Also, the ability to store parts as digital inventory (vs. FGI) and distribute them digitally can further reduce the need for physical storage and transportation of parts throughout the SC. As noted by an expert, ‘With AM, we will move from transcontinental transport problem (container, packaging, inventory redundancies) to last mile transport.’ Moreover, another expert explained that it is ‘Easy to use multiple transportation methods’. This is particularly true because AM materials normally come in fewer types and basic forms; therefore, it is easier to transport them with alternative modes of transport. As Ford and Despeisse (Citation2016) also state, ‘Logistics are simplified as fewer, more basic material inputs are needed’.
Furthermore, parts can be printed in transit using transport vehicles that are equipped with 3D printers (i.e. mobile manufacturing), reducing the lead time and improving flexibility in terms of production capacity. Notably, this manufacturing method is particularly feasible for small batch production because there is no need for product-specific tools or complex machine setups, making it possible to equip transport vehicles with 3D printers. Lastly, an expert mentioned that it is possible to use typical courier services to ship 3D-printed parts, as they are normally printed in low volumes. All these AM features add to flexibility in order fulfillment by allowing the parts to be delivered via different transport modes and routing options (i.e. Flexibility (Alternate distribution channels)), thus mitigating potential shortages in distribution and production capacities. Also, such AM-enabled freedom in transportation and distribution helps the adopting firm to deal with potential geopolitical disruptions, which can lead to supplier disruptions and negatively affect the import/export of products in overextended SCs (i.e. Turbulence (Exposure to geopolitical disruptions)).
Despite all the mentioned AM features, the average score of the quantitative answers still indicated a low effect of AM adoption on the use of alternative transport modes. With reference to potential barriers, it was noted that the ‘Transport of hazardous powders is slower’ (industry expert). In the case of hazardous materials, distribution capacity may become limited since transportation requires special care and conditions, limiting the flexibility to swiftly alternate between different transport modes and routing options. However, as this issue also applies to other production methods, it was not considered an adoption barrier in the context of AM technology adoption and SCR.
Proposition 9.
AM technology adoption has a low positive effect on the use of alternative transport modes, which enhances flexibility in order fulfillment. This mitigates production and distribution capacity shortages, supplier disruptions, and exposure to geopolitical disruptions.
5. Managerial implications
This study aimed to develop a preliminary practice-based framework that can help managers and policymakers make more informed decisions about how to improve SCR via different resilience practices in a context where AM technology is used. Drawing on the gathered empirical data, it was possible to explain how AM technology adoption affects different resilience practices and how these resilience practices, in turn, affect various SC capabilities and vulnerabilities that underlie SCR. In doing so, various pathways were explored that originate from the identified AM adoption features/barriers and lead to 48 sets of interlinked SC capabilities and vulnerabilities via the resilience practices under study (see Section 4 for details). These pathways are marked by black/red arrows in the AM-SCRP framework (), where the red arrows represent the pathways that lead to the deterioration of SC capabilities and consequently their related SC vulnerabilities. Moreover, the AM barriers highlighted in red are the cause of this deterioration, as explained throughout Section 4.
Figure 4. Additive manufacturing-supply chain resilience practices (AM-SCRP) framework (see the online version for colors).
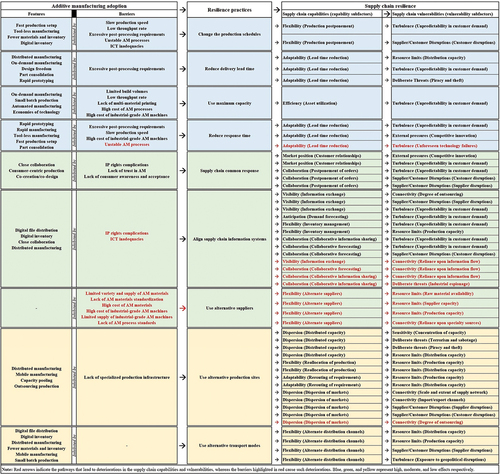
As indicated in the AM-SCRP framework, AM adoption affects SC ‘Flexibility’ via the resilience practices ‘Change the production schedule’, ‘Align supply chain information systems’, ‘Use alternative suppliers’, ‘Use alternative production sites’, and ‘Use alternative transport modes’. Firstly, the findings suggest that AM adoption has a high positive effect on changing production schedules, which in turn enhances flexibility in order fulfillment by facilitating production postponement. For instance, storing parts as digital inventory (rather than FGI) in an AM setting makes it easier to change the production schedules by moving the order penetration point (OPP) upstream the SC toward the make-to-order (MTO) policy (Verboeket and Krikke Citation2019), thus facilitating production postponement when compared to the make-to-stock (MTS) policy. This flexibility in production postponement, in turn, allows the firm to better deal with the unpredictability in customer demands and potential customer disruptions. However, barriers such as slow production speed, low throughput rate, or excessive post-processing requirements take away from this flexibility. Secondly, AM technology adoption has a moderate positive effect on aligning SC information systems, which tends to enhance flexibility in order fulfillment by facilitating inventory management (e.g. digital inventory vs. FGI). This flexibility in inventory management allows the firm to mitigate the unpredictability in customer demands and potential shortages in production capacity. Thirdly, AM adoption has a moderate negative effect on the use of alternative suppliers as there are multiple existing barriers to the supply of AM materials and industrial-grade AM machines (see ), which reduce the flexibility of the SC in sourcing, causing interrelated SC vulnerabilities to raw material availability, supplier capacity, production capacity, and reliance on specialty sources. These findings support the proposition put forward by Naghshineh and Carvalho (Citation2022b) that ‘SC vulnerabilities caused by AM adoption barriers negatively affect SCR’. Fourthly, AM adoption has a low positive effect on the use of alternative production sites. Nonetheless, this effect increases the flexibility of the SC in order fulfillment by making it possible to reallocate production to different sites through distributed manufacturing, mobile manufacturing, capacity pooling, or even outsourcing production to AM service providers/bureaus. This increase in flexibility allows the firm to mitigate possible shortages in distribution and production capacities. However, the lack of specialized production infrastructure inhibits the positive effect of AM adoption on this resilience practice. Lastly, AM technology adoption has a low positive effect on the use of alternative transport modes. Nevertheless, this effect improves the flexibility of the SC in order fulfillment by making it easier to alternate between different distribution channels (i.e. transportation modes and routing options), which is attributed to different AM adoption features such as fewer materials and inventory. This feature, for instance, facilitates the use of different transport options, as raw materials can be more easily adapted to alternative modes of transport. The resultant flexibility, in turn, mitigates SC vulnerabilities to shortages in production and distribution capacities, as well as potential supplier and geopolitical disruptions. Overall, the findings are in accordance with Delic and Eyers (Citation2020) who state that AM adoption improves SC flexibility. In contrast, however, this study shows how adopting AM technology can deteriorate the flexibility of the SC in sourcing by limiting the use of alternative suppliers, therefore causing SC vulnerabilities that negatively affect SCR.
AM adoption affects ‘Adaptability’ via the resilience practices ‘Reduce delivery lead time’, ‘Reduce response time’, and ‘Use alternative production sites’. Firstly, the findings suggest that AM adoption has a high positive effect on reducing delivery lead time, which augments SC adaptability. For instance, AM enables the consolidation of many parts into one complex part utilizing a high degree of design freedom. Not only does this allow the adopting firm to better respond to unpredictable customer demands for complex parts/products, but it also reduces the need for warehousing and distribution of many subcomponents along the SC, thus reducing SC vulnerabilities to distribution capacity shortages, as well as instances of inventory theft and damage. Another important AM feature that contributes to reducing delivery lead time is the on-demand manufacturing of parts close to their point of use. However, excessive post-processing inhibits the effectiveness of AM adoption in this case. Secondly, AM adoption has a high positive effect on reducing response time, enhancing the ability of the firm to quickly adapt its operations to respond to sudden challenges or opportunities. AM features such as rapid prototyping and rapid manufacturing of parts empowered by tool-less manufacturing and fast production setup help reduce the production lead time, thus reducing the response time. This will help increase the adaptability of the SC to respond to vulnerabilities caused by the unpredictability in customer demands and competitive innovations in the market. As Niaki and Nonino (Citation2017) state, ‘AM is most advantageous in market environments characterized by demand for customization and flexible parts’, referring to contemporary market environments where frequent competitive innovations drive many firms to quickly adapt their product portfolios to erratic customer demands to gain a competitive advantage, which is in line with the dynamic capabilities view of the firm. However, slow production speed and excessive post-processing requirements are among the barriers that inhibit the enhancing effect of AM adoption on this resilience practice. Moreover, the present instability in AM processes can lead to unforeseen failures in production operations, thus prolonging the response time. Thirdly, AM adoption has a low positive effect on the use of alternative production sites, which helps improve adaptability. In the case of disruptions, AM-enabled distributed manufacturing allows the firm to promptly reroute its production to different facilities/sites (i.e. capacity pooling) or even to other manufacturing firms (i.e. outsourcing production), thereby mitigating SC vulnerabilities caused by shortages in production and distribution capacities. Nonetheless, provisioning a specialized infrastructure – at each and every production site – to ensure consistent AM operations can be difficult, which inhibits the enhancing effect of AM on the use of alternative production sites.
AM adoption affects ‘Dispersion’ via the resilience practice ‘Use alternative production sites’. According to the findings, AM adoption has a low positive effect on the use of alternative production sites, which tends to positively influence distributed capacity and dispersion of markets. AM features such as distributed manufacturing enable the firm to set up production facilities at various locations and avoid SC vulnerabilities that stem from high level of SC density, deliberate threats such as terrorism and sabotage, or even piracy and theft. These AM features also allow the firm to expand the scale and extent of its supply network by gaining access to international markets. Nevertheless, the lack of specialized production infrastructure is a barrier that inhibits the enhancing effect of AM adoption on this resilience practice. Issues such as affording expensive AM machine infrastructure and/or establishing a secure IT infrastructure create this barrier. Furthermore, outsourcing production to AM service providers/bureaus can increase SC vulnerability due to increased dependence on external entities.
AM technology adoption affects ‘Collaboration’ via the resilience practices ‘Supply chain common response’ and ‘Align supply chain information systems’. Firstly, the findings suggest that AM adoption has a moderate positive effect on practicing SC common response. This effect positively influences SC collaboration, particularly by enabling postponement of orders. AM-enabled consumer-centric methods of production tend to mitigate SC vulnerabilities caused by the unpredictability in customer demands and customer disruptions. However, potential IP rights complications and the lack of consumer awareness and trust in the technology inhibit the enhancing effect of AM adoption on this resilience practice. Secondly, AM adoption has a moderate positive effect on aligning SC information systems, which tends to positively affect collaborative information sharing and forecasting with SC partners. For instance, the opportunity to manufacture parts in a decentralized SC setting (i.e. distributed manufacturing) via AM motivates SC partners to align their information systems to enable this production method. Such an initiative aimed at improving collaborative information sharing would, in turn, reduce SC vulnerabilities to unpredictability in customer demand and customer disruptions. For the most part, these findings complement those of Oettmeier and Hofmann (Citation2016) and Luomaranta and Martinsuo (Citation2020), who state that AM adoption promotes collaboration throughout the SC. However, it should also be noted that potential IP rights complications and ICT inadequacies are barriers that not only negatively affect collaborative information sharing and forecasting capabilities but can also cause SC vulnerabilities to industrial espionage and dependence on continuous information flow. This is because digitalizing the SC with AM technology requires coordination and real-time communication between different information systems throughout the SC; therefore, the SC can become ‘vulnerable to cyberattacks. These attacks can include industrial espionage, IP leakage, or even production sabotage’ (Tang and Veelenturf Citation2019).
As shown in the AM-SCRP framework (), AM adoption affects ‘Visibility’ and ‘Anticipation’ via the resilience practice ‘Align supply chain information systems’. More specifically, AM features such as digital inventory or digital file distribution, which empower the distributed manufacturing of parts across the SC, incentivize SC members to align their information systems, hence improving SC visibility and anticipation capabilities. This improvement in SC visibility not only facilitates the outsourcing of operations but also mitigates the vulnerability of the SC to supplier disruptions that originate from upstream SC. Additionally, by staying aligned and connected, forecasting methods can be used more effectively to anticipate the unpredictability in customer demand that originates from downstream the SC, hence improving SCR (Naghshineh and Lotfi Citation2019). Nonetheless, ICT inadequacies and IP rights complications not only inhibit the enhancing effect of AM adoption on this resilience practice but can also cause SC vulnerability to dependence on continuous information flow for everyday operations, calling for a secure ICT infrastructure.
AM technology adoption affects ‘Efficiency’ via the resilience practice ‘Use maximum capacity’. The findings suggest that AM adoption has a high positive effect on using maximum capacity due to different features such as automated manufacturing and small batch production. These features support the notion of economies of technology and can help solve the dilemma concerning economies of scale. For instance, economies of technology would be possible by allocating a single operator to control multiple AM machines, reducing labor and manpower costs. Moreover, as parts/products can be produced in small batches or even as one-offs, the manufacturing firm is not constrained by economies of scale. Therefore, such features make AM an ideal technology for easily adjusting production capacity, which allows the adopting firm to efficiently use the resources at its disposal, thus generating outputs with minimal resource requirements. This efficiency in production helps the manufacturing firm to cope with the unpredictability in customer demand, especially when considering time and cost factors. These findings lend support to Belhadi et al. (Citation2022) who argue that AM adoption increases SC efficiency. However, currently, barriers such as limited build volumes and lack of multi-material printing undermine AM’s ability to easily adjust production capacity, thus negatively affecting efficiency due to incurred time and cost penalties.
Lastly, AM adoption affects ‘Market position’ via the resilience practice ‘Supply chain common response’. The findings suggest that AM adoption has a moderate positive effect on practicing SC chain common response, mainly due to co-design/co-creation and consumer-centric production, which allow the customer to be involved in the design and production phases of a product. By allowing customers to co-design and co-create the products, not only can the firm respond better to the unpredictability in their demands and generate customer satisfaction, but it can also cope with external competitive innovations more effectively, thus consolidating its position in the market. However, concerns for IP rights as well as lack of consumer awareness and trust in AM are barriers that inhibit the enhancing effect of AM adoption on this resilience practice.
6. Conclusion and future work
In line with the dynamic capabilities view of the firm toward technology adoption, the objective of this study was to investigate the implications of AM technology adoption for SCR while considering the role of resilience practices. More specifically, this study aimed to explore how AM technology adoption affects different resilience practices, and subsequently, how AM-enabled resilience practices help the adopting firm to develop SC capabilities to deal with SC vulnerabilities, thus enhancing SCR. To factor in the viewpoint of the industry on the subject matter, an exploratory survey was conducted whereby both quantitative and qualitative empirical data were collected from a heterogeneous sample of experts working for companies involved in different industries that had adopted various AM processes for a wide range of applications. The quantitative data were used to estimate to what extent AM adoption affects different resilience practices, while the qualitative data were analyzed to explain the effects of AM adoption on the resilience practices and how they would in turn affect the SC capabilities and vulnerabilities that underlie SCR. Subsequently, the findings were triangulated through comparison with the existing evidence in the AM and SCM literature. Thus, a preliminary practice-based framework based on empirical data from industry was put forward (), which clarifies the underlying relationships between AM technology adoption, resilience practices, and SC capabilities and vulnerabilities that underlie SCR. Moreover, these findings were used to put forward propositions that indicate the opportunities that AM technology adoption would present for enhancing SCR.
The contributions of this study are manyfold. At the interface of digital technologies and SCR, ‘many past studies are still conceptual and lack empirical corroboration’ (Iftikhar et al. Citation2022). Therefore, by conducting an exploratory survey in different industries, this study addressed an unexplored theme in the body of literature based on empirical evidence. Moreover, as the survey targeted companies operating in developed economies, the findings are more likely to be generalizable since digital technologies such as AM are more mature in developed economies (Wagire et al. Citation2021). Additionally, by drawing on empirical insights sought from experts involved in different industries and countries, practical knowledge was derived in the context of AM technology adoption and SCR management that can be applied across international markets (Balakrishnan and Ramanathan Citation2021). This led to putting forward testable propositions informed by empirical insights concerning the opportunities that AM technology adoption would present for enhancing SCR, which can be examined by future research in different contexts. Furthermore, using this practical knowledge, the conceptual AM-SCR framework put forward by Naghshineh and Carvalho (Citation2022a) was refined and transformed into a preliminary practice-based framework (i.e. AM-SCRP) that can be tested by practitioners and policymakers.
One of the main contributions of the AM-SCRP framework is the specification of various resilience practices that are linked to certain SC capabilities and vulnerabilities. Hence, in the face of impending SC vulnerabilities, the AM-SCRP framework can help practitioners and policymakers decide which AM-enabled resilience practices to deploy for developing necessary SC capabilities, thereby generating SCR. Moreover, this framework helps practitioners and policymakers to evaluate the adoption features of AM technology that enable resilience practices, while also highlighting the potential barriers that not only inhibit the enhancing effects of AM technology adoption in this regard but may also give rise to certain SC vulnerabilities. While there are a handful of broad-based frameworks in the literature that present a general overview of the impacts of different digital technologies on SCR (e.g. Hägele, Grosse, and Ivanov Citation2023; Ivanov, Dolgui, and Sokolov Citation2019; Spieske and Birkel Citation2021), to the best of the authors’ knowledge, to date, no empirical framework – other than the AM-SCRP framework – outlines the implications of AM technology adoption for SCR while considering the role of resilience practices. Hence, this study managed to address a noticeable gap in the body of literature regarding ‘Additive Manufacturing Enabled Supply Chain Management’ (Sonar, Khanzode, and Akarte Citation2022), particularly for managing SCR (Ivanov, Blackhurst, and Das Citation2021; Tortorella et al. Citation2022).
Regarding the research limitations, first, the resilience practices under study had to be limited in number to control the scope of the research. However, future research may extend upon the findings of this study by exploring more resilience practices. Moreover, as this research is the first to empirically explore the implications of AM technology adoption for SCR while considering the mediating role of different resilience practices, rather than claiming generalizability of the results, generic propositions are put forward that are open to further validation and refinement. Therefore, future research should conduct single or multiple case studies to test these propositions and validate the AM-SCRP framework in certain industries with specific AM processes and SC settings. Conducting such studies would result in context-specific variations of the AM-SCRP framework, thus advancing this preliminary framework. Last but not least, as AM technology progresses and its adoption barriers gradually disappear, longitudinal research can help estimate the change in the effectiveness of AM-enabled resilience practices over time.
Declaration of generative AI and AI-assisted technologies
No Generative AI or AI-assisted technologies have been used in the writing and preparation of this manuscript.
Acknowledgments
The first author acknowledges Fundação para a Ciência e a Tecnologia (FCT–MCTES) for its financial support via the Doctoral grant PRT/BD/152828/2021 (MIT Portugal Program). The second author acknowledges Fundação para a Ciência e a Tecnologia (FCT–MCTES) for its financial support via the projects UIDB/00667/2020 and UIDP/00667/2020 (UNIDEMI).
Disclosure statement
No potential conflict of interest was reported by the author(s).
Data availability statement
The participants of this study did not give written consent for their data to be shared publicly, so due to the sensitive nature of the research supporting data is not available.
Correction Statement
This article has been republished with minor changes. These changes do not impact the academic content of the article.
Additional information
Funding
References
- Ali, I., and I. Gölgeci. 2019. “Where Is Supply Chain Resilience Research Heading? A Systematic and Co-Occurrence Analysis.” International Journal of Physical Distribution & Logistics Management 49 (8): 793–815. https://doi.org/10.1108/IJPDLM-02-2019-0038.
- Babbie, E. 1990. Survey Research Methods. Stamford, CT: Cengage Learning.
- Balakrishnan, A. S., and U. Ramanathan. 2021. “The Role of Digital Technologies in Supply Chain Resilience for Emerging Markets’ Automotive Sector.” Supply Chain Management: An International Journal 26 (6): 654–671. https://doi.org/10.1108/SCM-07-2020-0342.
- Belhadi, A., S. Kamble, C. J. C. Jabbour, A. Gunasekaran, N. O. Ndubisi, and M. Venkatesh. 2021. “Manufacturing and Service Supply Chain Resilience to the COVID-19 Outbreak: Lessons Learned from the Automobile and Airline Industries.” Technological Forecasting & Social Change 163:120447. https://doi.org/10.1016/j.techfore.2020.120447.
- Belhadi, A., S. S. Kamble, M. Venkatesh, C. J. C. Jabbour, and I. Benkhati. 2022. “Building Supply Chain Resilience and Efficiency Through Additive Manufacturing: An Ambidextrous Perspective on the Dynamic Capability View.” International Journal of Production Economics 249:108516. https://doi.org/10.1016/j.ijpe.2022.108516.
- Bogers, M., R. Hadar, and A. Bilberg. 2016. “Additive Manufacturing for Consumer-Centric Business Models: Implications for Supply Chains in Consumer Goods Manufacturing.” Technological Forecasting & Social Change 102:225–239. https://doi.org/10.1016/j.techfore.2015.07.024.
- Carvalho, H., B. Naghshineh, K. Govindan, and V. Cruz-Machado. 2022. “The Resilience of On-Time Delivery to Capacity and Material Shortages: An Empirical Investigation in the Automotive Supply Chain.” Computers & Industrial Engineering 171:108375. https://doi.org/10.1016/j.cie.2022.108375.
- Cavusgil, E., S. H. Seggie, and M. B. Talay. 2007. “Dynamic Capabilities View: Foundations and Research Agenda.” Journal of Marketing Theory & Practice 15 (2): 159–166. https://doi.org/10.2753/MTP1069-6679150205.
- Chekurov, S., S. Metsä-Kortelainen, M. Salmi, I. Roda, and A. Jussila. 2018. “The Perceived Value of Additively Manufactured Digital Spare Parts in Industry: An Empirical Investigation.” International Journal of Production Economics 205:87–97. https://doi.org/10.1016/j.ijpe.2018.09.008.
- Chiu, M. C., and Y. H. Lin. 2016. “Simulation Based Method Considering Design for Additive Manufacturing and Supply Chain: An Empirical Study of Lamp Industry.” Industrial Management & Data Systems 116 (2): 322–348. https://doi.org/10.1108/IMDS-07-2015-0266.
- Chowdhury, M. M. H., and M. Quaddus. 2017. “Supply Chain Resilience: Conceptualization and Scale Development Using Dynamic Capability Theory.” International Journal of Production Economics 188:185–204. https://doi.org/10.1016/j.ijpe.2017.03.020.
- Christopher, M., and H. Peck. 2004. “Building the Resilient Supply Chain.” The International Journal of Logistics Management 15 (2): 1–13. https://doi.org/10.1108/09574090410700275.
- Cronbach, L. J. 1951. “Coefficient Alpha and the Internal Structure of Tests.” Psychometrika 16 (3): 297–334. https://doi.org/10.1007/BF02310555.
- Delic, M., and D. R. Eyers. 2020. “The Effect of Additive Manufacturing Adoption on Supply Chain Flexibility and Performance: An Empirical Analysis from the Automotive Industry.” International Journal of Production Economics 228:107689. https://doi.org/10.1016/j.ijpe.2020.107689.
- Delic, M., D. R. Eyers, and J. Mikulic. 2019. “Additive Manufacturing: Empirical Evidence for Supply Chain Integration and Performance from the Automotive Industry.” Supply Chain Management: An International Journal 24 (5): 604–621. https://doi.org/10.1108/SCM-12-2017-0406.
- Dolgui, A., and D. Ivanov. 2020. “Exploring Supply Chain Structural Dynamics: New Disruptive Technologies and Disruption risks.” International Journal of Production Economics 229:107886. https://doi.org/10.1016/j.ijpe.2020.107886.
- Durach, C. F., S. Kurpjuweit, and S. M. Wagner. 2017. “The Impact of Additive Manufacturing on Supply Chains.” International Journal of Physical Distribution & Logistics Management 47 (10): 954–971. https://doi.org/10.1108/IJPDLM-11-2016-0332.
- Durão, L. F. C., A. Christ, E. Zancul, R. Anderl, and K. Schützer. 2017. “Additive Manufacturing Scenarios for Distributed Production of Spare Parts.” International Journal of Advanced Manufacturing Technology 93 (1): 869–880. https://doi.org/10.1007/s00170-017-0555-z.
- Eyers, D. R., and A. T. Potter. 2015. “E-Commerce Channels for Additive Manufacturing: An Exploratory Study.” Journal of Manufacturing Technology Management 26 (3): 390–411. https://doi.org/10.1108/JMTM-08-2013-0102.
- Eyers, D. R., A. T. Potter, J. Gosling, and M. M. Naim. 2018. “The Flexibility of Industrial Additive Manufacturing Systems.” International Journal of Operations & Production Management 38 (12): 2313–2343. https://doi.org/10.1108/IJOPM-04-2016-0200.
- Ford, S., and M. Despeisse. 2016. “Additive Manufacturing and Sustainability: An Exploratory Study of the Advantages and Challenges.” Journal of Cleaner Production 137: 1573–1587. https://doi.org/10.1016/j.jclepro.2016.04.150.
- Forza, C. 2002. “Survey Research in Operations Management: A Process‐Based Perspective.” International Journal of Operations & Production Management 22 (2): 152–194. https://doi.org/10.1108/01443570210414310.
- Ghadge, A., G. Karantoni, A. Chaudhuri, and A. Srinivasan. 2018. “Impact of Additive Manufacturing on Aircraft Supply Chain Performance.” Journal of Manufacturing Technology Management 29 (5): 846–865. https://doi.org/10.1108/JMTM-07-2017-0143.
- Hägele, S., E. H. Grosse, and D. Ivanov. 2023. “Supply Chain Resilience: A Tertiary Study.” International Journal of Integrated Supply Management 16 (1): 52–81. https://doi.org/10.1504/IJISM.2023.127660.
- Hübner, A., J. Wollenburg, A. Holzapfel, and C. Mena and Michael Bourlakis. 2016. “Retail Logistics in the Transition from Multi-Channel to Omni-Channel.” International Journal of Physical Distribution & Logistics Management 46 (6/7): 562–583. https://doi.org/10.1108/IJPDLM-08-2015-0179.
- Iftikhar, A., I. Ali, A. Arslan, and S. Tarba. 2022. “Digital Innovation, Data Analytics, and Supply Chain Resiliency: A Bibliometric-Based Systematic Literature Review.” Annals of Operations Research 1–24. https://doi.org/10.1007/s10479-022-04765-6.
- ISO/ASTM 52900. 2021. “Additive manufacturing — general principles —fundamentals and vocabulary.” West Conshohocken: ASTM International.
- Ivanov, D., J. Blackhurst, and A. Das. 2021. “Supply Chain Resilience and Its Interplay with Digital Technologies: Making Innovations Work in Emergency Situations.” International Journal of Physical Distribution & Logistics Management 51 (2): 97–103. https://doi.org/10.1108/IJPDLM-03-2021-409.
- Ivanov, D., A. Dolgui, and B. Sokolov. 2019. “The Impact of Digital Technology and Industry 4.0 on the Ripple Effect and Supply Chain Risk Analytics.” International Journal of Production Research 57 (3): 829–846. https://doi.org/10.1080/00207543.2018.1488086.
- Jain, V., S. Wadhwa, and S. G. Deshmukh. 2009. “Enhancing Flexibility in Supply Chains: Modelling Random Demands and Non-Stationary Supply Information.” International Journal of Computer Integrated Manufacturing 22 (8): 812–822. https://doi.org/10.1080/09511920902866070.
- Jiang, R., R. Kleer, and F. T. Piller. 2017. “Predicting the Future of Additive Manufacturing: A Delphi Study on Economic and Societal Implications of 3D Printing for 2030.” Technological Forecasting & Social Change 117:84–97. https://doi.org/10.1016/j.techfore.2017.01.006.
- Kalaitzi, D., A. Matopoulos, M. Bourlakis, and W. Tate. 2019. “Supply Chains Under Resource Pressure: Strategies for Improving Resource Efficiency and Competitive Advantage.” International Journal of Operations & Production Management 39 (12): 1323–1354. https://doi.org/10.1108/IJOPM-02-2019-0137.
- Kembro, J., and A. Norrman. 2022. “The Transformation from Manual to Smart Warehousing: An Exploratory Study with Swedish Retailers.” The International Journal of Logistics Management 33 (5): 107–135. https://doi.org/10.1108/IJLM-11-2021-0525.
- Khajavi, S. H., J. Partanen, and J. Holmström. 2014. “Additive Manufacturing in the Spare Parts Supply Chain.” Computers in Industry 65 (1): 50–63. https://doi.org/10.1016/j.compind.2013.07.008.
- Khajavi, S. H., J. Partanen, J. Holmström, and J. Tuomi. 2015. “Risk Reduction in New Product Launch: A Hybrid Approach Combining Direct Digital and Tool-Based Manufacturing.” Computers in Industry 74:29–42. https://doi.org/10.1016/j.compind.2015.08.008.
- Knofius, N., M. C. van der Heijden, and W. H. Zijm. 2016. “Selecting Parts for Additive Manufacturing in Service Logistics.” Journal of Manufacturing Technology Management 27 (7): 915–931. https://doi.org/10.1108/JMTM-02-2016-0025.
- Kochan, C. G., and D. R. Nowicki. 2018. “Supply Chain Resilience: A Systematic Literature Review and Typological Framework.” International Journal of Physical Distribution & Logistics Management 48 (8): 842–865. https://doi.org/10.1108/IJPDLM-02-2017-0099.
- Kumar, S., R. D. Raut, E. Aktas, B. E. Narkhede, and V. V. Gedam. 2022. “Barriers to Adoption of Industry 4.0 and Sustainability: A Case Study with SMEs.” International Journal of Computer Integrated Manufacturing 1 (5): 657–677. https://doi.org/10.1080/0951192X.2022.2128217.
- Kunovjanek, M., and G. Reiner. 2020. “How Will the Diffusion of Additive Manufacturing Impact the Raw Material Supply Chain Process?” International Journal of Production Research 58 (5): 1540–1554. https://doi.org/10.1080/00207543.2019.1661537.
- Kunovjanek, M., and C. Wankmüller. 2020. “An Analysis of the Global Additive Manufacturing Response to the COVID-19 Pandemic.” Journal of Manufacturing Technology Management 32 (9): 75–100. https://doi.org/10.1108/JMTM-07-2020-0263.
- Kurpjuweit, S., C. G. Schmidt, M. Klöckner, and S. M. Wagner. 2021. “Blockchain in Additive Manufacturing and Its Impact on Supply Chains.” Journal of Business Logistics 42 (1): 46–70. https://doi.org/10.1111/jbl.12231.
- Leonard‐Barton, D. 1992. “Core Capabilities and Core Rigidities: A Paradox in Managing New Product Development.” Strategic Management Journal 13 (S1): 111–125. https://doi.org/10.1002/smj.4250131009.
- Luomaranta, T., and M. Martinsuo. 2020. “Supply Chain Innovations for Additive Manufacturing.” International Journal of Physical Distribution & Logistics Management 50 (1): 54–79. https://doi.org/10.1108/IJPDLM-10-2018-0337.
- Mellor, S., L. Hao, and D. Zhang. 2014. “Additive Manufacturing: A Framework for Implementation.” International Journal of Production Economics 149:194–201. https://doi.org/10.1016/j.ijpe.2013.07.008.
- Miles, M. B., and A. M. Huberman. 1994. Qualitative Data Analysis: An Expanded Sourcebook. New Delhi: sage.
- Naghshineh, B., and H. Carvalho. 2022a. “The Implications of Additive Manufacturing Technology Adoption for Supply Chain Resilience: A Systematic Search and Review.” International Journal of Production Economics 247:108387. https://doi.org/10.1016/j.ijpe.2021.108387.
- Naghshineh, B., and H. Carvalho. 2022b. “Exploring the Interrelations Between Additive Manufacturing Adoption Barriers and Supply Chain Vulnerabilities: The Case of an Original Equipment Manufacturer.” Journal of Manufacturing Technology Management 33 (8): 1473–1489. https://doi.org/10.1108/JMTM-04-2022-0148.
- Naghshineh, B., M. Fragoso, and H. Carvalho. 2023. “Rethinking Additive Manufacturing for Spare Parts Supply Chain Management.” Research-Technology Management 66 (4): 7970. https://doi.org/10.1080/08956308.2023.2207970.
- Naghshineh, B., and M. Lotfi. 2019. “Enhancing Supply Chain Resilience: An Empirical Investigation.” Continuity & Resilience Review 1 (1): 47–62. https://doi.org/10.1108/CRR-09-2018-0002.
- Niaki, M. K., and F. Nonino. 2017. “Impact of Additive Manufacturing on Business Competitiveness: A Multiple Case Study.” Journal of Manufacturing Technology Management 28 (1): 56–74. https://doi.org/10.1108/JMTM-01-2016-0001.
- Oettmeier, K., and E. Hofmann. 2016. “Impact of Additive Manufacturing Technology Adoption on Supply Chain Management Processes and Components.” Journal of Manufacturing Technology Management 27 (7): 944–968. https://doi.org/10.1108/JMTM-12-2015-0113.
- Oettmeier, K., and E. Hofmann. 2017. “Additive Manufacturing Technology Adoption: An Empirical Analysis of General and Supply Chain-Related Determinants.” Journal of Business Economics 87 (1): 97–124. https://doi.org/10.1007/s11573-016-0806-8.
- O’Leary-Kelly, S. W., and R. J. Vokurka. 1998. “The Empirical Assessment of Construct Validity.” Journal of Operations Management 16 (4): 387–405. https://doi.org/10.1016/S0272-6963(98)00020-5.
- Patil, H., S. Niranjan, G. Narayanamurthy, and A. Narayanan. 2023. “Investigating Contingent Adoption of Additive Manufacturing in Supply Chains.” International Journal of Operations & Production Management 43 (3): 489–519. https://doi.org/10.1108/IJOPM-05-2022-0286.
- Pettit, T. J., K. L. Croxton, and J. Fiksel. 2013. “Ensuring Supply Chain Resilience: Development and Implementation of an Assessment Tool.” Journal of Business Logistics 34 (1): 46–76. https://doi.org/10.1111/jbl.12009.
- Pettit, T. J., K. L. Croxton, and J. Fiksel. 2019. “The Evolution of Resilience in Supply Chain Management: A Retrospective on Ensuring Supply Chain Resilience.” Journal of Business Logistics 40 (1): 56–65. https://doi.org/10.1111/jbl.12202.
- Pettit, T. J., J. Fiksel, and K. L. Croxton. 2010. “Ensuring Supply Chain Resilience: Development of a Conceptual Framework.” Journal of Business Logistics 31 (1): 1–21. https://doi.org/10.1002/j.2158-1592.2010.tb00125.x.
- Ponomarov, S. Y., and M. C. Holcomb. 2009. “Understanding the Concept of Supply Chain Resilience.” The International Journal of Logistics Management 20 (1): 124–143. https://doi.org/10.1108/09574090910954873.
- Rylands, B., T. Böhme, R. Gorkin, J. Fan, and T. Birtchnell. 2016. “The Adoption Process and Impact of Additive Manufacturing on Manufacturing Systems.” Journal of Manufacturing Technology Management 27 (7): 969–989. https://doi.org/10.1108/JMTM-12-2015-0117.
- Saenz de Ugarte, B., A. Artiba, and R. Pellerin. 2009. “Manufacturing Execution System–a Literature Review.” Production Planning & Control 20 (6): 525–539. https://doi.org/10.1080/09537280902938613.
- Sharma, G. 2017. “Pros and Cons of Different Sampling Techniques.” International Journal of Applied Research 3 (7): 749–752.
- Sonar, H., V. Khanzode, and M. Akarte. 2022. “Additive Manufacturing Enabled Supply Chain Management: A Review and Research Directions.” Vision: The Journal of Business Perspective 26 (2): 147–162. https://doi.org/10.1177/09722629221087308.
- Spieske, A., and H. Birkel. 2021. “Improving Supply Chain Resilience Through Industry 4.0: A Systematic Literature Review Under the Impressions of the COVID-19 Pandemic.” Computers & Industrial Engineering 158:107452. https://doi.org/10.1016/j.cie.2021.107452.
- Steenhuis, H. J., and L. Pretorius. 2017. “The Additive Manufacturing Innovation: A Range of Implications.” Journal of Manufacturing Technology Management 28 (1): 122–143. https://doi.org/10.1108/JMTM-06-2016-0081.
- Stillström, C., and M. Jackson. 2007. “The Concept of Mobile Manufacturing.” Journal of Manufacturing Systems 26 (3–4): 188–193. https://doi.org/10.1016/j.jmsy.2008.03.002.
- Tang, C. S., and L. P. Veelenturf. 2019. “The Strategic Role of Logistics in the Industry 4.0 Era.” Transportation Research Part E: Logistics & Transportation Review 129:1–11. https://doi.org/10.1016/j.tre.2019.06.004.
- Teece, D. J. 2014. “The Foundations of Enterprise Performance: Dynamic and Ordinary Capabilities in an (Economic) Theory of Firms.” Academy of Management Perspectives 28 (4): 328–352. https://doi.org/10.5465/amp.2013.0116.
- Teece, D. J., G. Pisano, and A. Shuen. 1997. “Dynamic Capabilities and Strategic Management.” Strategic Management Journal 18 (7): 509–533. https://doi.org/10.1002/(SICI)1097-0266(199708)18:7<509:AID-SMJ882>3.0.CO;2-Z.
- Tortorella, G., F. S. Fogliatto, S. Gao, and T. K. Chan. 2022. “Contributions of Industry 4.0 to Supply Chain Resilience.” The International Journal of Logistics Management 33 (2): 547–566. https://doi.org/10.1108/IJLM-12-2020-0494.
- Towill, D. R. 1991. “Supply Chain Dynamics.” International Journal of Computer Integrated Manufacturing 4 (4): 197–208. https://doi.org/10.1080/09511929108944496.
- Tuck, C., R. Hague, and N. Burns. 2007. “Rapid Manufacturing: Impact on Supply Chain Methodologies and Practice.” International Journal of Services and Operations Management 3 (1): 1–22. https://doi.org/10.1504/IJSOM.2007.011459.
- Tukamuhabwa, B. R., M. Stevenson, J. Busby, and M. Zorzini. 2015. “Supply Chain Resilience: Definition, Review and Theoretical Foundations for Further Study.” International Journal of Production Research 53 (18): 5592–5623. https://doi.org/10.1080/00207543.2015.1037934.
- Ukobitz, D., and R. Faullant. 2021. “Leveraging 3D Printing Technologies: The Case of Mexico’s Footwear Industry.” Research-Technology Management 64 (2): 20–30. https://doi.org/10.1080/08956308.2021.1864919.
- Verboeket, V., S. H. Khajavi, H. Krikke, M. Salmi, and J. Holmström. 2021. “Additive Manufacturing for Localized Medical Parts Production: A Case Study.” Institute of Electrical and Electronics Engineers Access 9:25818–25834. https://doi.org/10.1109/ACCESS.2021.3056058.
- Verboeket, V., and H. Krikke. 2019. “The Disruptive Impact of Additive Manufacturing on Supply Chains: A Literature Study, Conceptual Framework and Research Agenda.” Computers in Industry 111:91–107. https://doi.org/10.1016/j.compind.2019.07.003.
- Wagire, A. A., R. Joshi, A. P. S. Rathore, and R. Jain. 2021. “Development of Maturity Model for Assessing the Implementation of Industry 4.0: Learning from Theory and Practice.” Production Planning & Control 32 (8): 603–622. https://doi.org/10.1080/09537287.2020.1744763.
- Wagner, S. M., and R. O. Walton. 2016. “Additive Manufacturing’s Impact and Future in the Aviation Industry.” Production Planning & Control 27 (13): 1124–1130. https://doi.org/10.1080/09537287.2016.1199824.
- Walker, H., D. Chicksand, Z. Radnor, and G. Watson. 2015. “Theoretical Perspectives in Operations Management: An Analysis of the Literature.” International Journal of Operations & Production Management 35 (8): 1182–1206. https://doi.org/10.1108/IJOPM-02-2014-0089.
- Wernerfelt, B. 1984. “A Resource‐Based View of the Firm.” Strategic Management Journal 5 (2): 171–180. https://doi.org/10.1002/smj.4250050207.
- Wohlers, T. 2021. “3D Printing and Additive Manufacturing Global State of the Industry.” Annual Worldwide Progress Report, Wohlers Associates. https://wohlersassociates.com/product/wohlers-report-2021/.
- Zanoni, S., M. Ashourpour, A. Bacchetti, M. Zanardini, and M. Perona. 2019. “Supply Chain Implications of Additive Manufacturing: A Holistic Synopsis Through a Collection of Case Studies.” International Journal of Advanced Manufacturing Technology 102 (9): 3325–3340. https://doi.org/10.1007/s00170-019-03430-w.
- Zouari, D., S. Ruel, and L. Viale. 2020. “Does Digitalising the Supply Chain Contribute to Its Resilience?” International Journal of Physical Distribution & Logistics Management 51 (2): 149–180. https://doi.org/10.1108/IJPDLM-01-2020-0038.
Appendix A
– Definitions for the SC capabilities and SC vulnerabilities and their subfactors adapted from Pettit et al. (Citation2013)
Appendix B
– Questionnaire (supplemental online material)
https://osf.io/ysjnm/?view_only=2a36f15093bd4610bbfb780f92b2ceef
Appendix C
– Illustrative examples for qualitative data analysis (supplemental online material)
https://osf.io/ysjnm/?view_only=2a36f15093bd4610bbfb780f92b2ceef