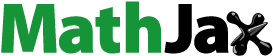
Abstract
This research aims to derive the average run length (ARL) evaluation of the double exponentially weighted moving average (double EWMA) control chart for observation data that follows exponential white noise in a time series model with an autoregressive model. Since most real-world data is automatically correlated, autoregressive models are available. Comparisons were made between the ARLs obtained using the explicit formula and the numerical integral equation (NIE) approach. The results showed that the explicit formula's use of the ARL outperformed the NIE approach in terms of computation time. After that, the efficacy of the exponentially weighted moving average (EWMA) and double EWMA charts is then compared using the suggested explicit ARL formula. The ARL of the double EWMA chart was found to perform better than the ARL of the EWMA chart in all situations. It also uses natural gas and diesel prices on stock exchanges around the world as cases studies. The results show that the double EWMA chart has better detection sensitivity than the EWMA chart, and the results are consistent with the experimental results. As a result, the sensitivity of the double EWMA chart in detecting changes makes it a good alternative for monitoring processes with real-world data.
1. Introduction
Time-series data analysis has been the subject of extensive research. Currently, it is utilised for predicting a variety of circumstances, including stock trends epidemic diseases, export sales and cost prediction (Cheng et al., Citation2020; Satrio et al., Citation2021). There are common uses for these models as an autoregressive (AR), a moving average (MA), and an autoregressive moving average (ARMA). Statistical process control (SPC) has been widely used in the industrial sector to monitor, control, and improve operations. Many researchers have studied SPC using real-world data and case studies. For instance, Tegegne et al. (Citation2022) suggested a new kind of multivariate control chart for the manufacturing process, which was utilised to better decide on quality control during the production process. They were conducted to validate the control chart by using a case study from the cement industry. Yeganeh et al. (Citation2023) employed SPC techniques, particularly control charts, to track the probability of connections between network surveillance system nodes using control charts based on machine learning.
The control chart is widely used in the fields of finance, business, engineering, healthcare, and others to track processes and detects sudden changes in the mean or variance of those processes, especially in the field of industries (Fugen, Citation2019; Zhang et al., Citation2022). Using control charts, it is possible to distinguish between common and unique sources of process variation and handle each case separately. The control limits on control charts are determined by common factors. Control charts provide precise instructions on how and when to modify a process. Nowadays, control charts are an essential tool in SPC, and their industrial significance lies in their ability to monitor and control process variability, improve process efficiency, reduce costs, ensure product quality, and support decision-making. There are many types of research that were presented that utilised the control chart in the field of industries. Shrestha (Citation2021) used a control chart to control the sausage manufacturing process. Tegegne et al. (Citation2022) used a control chart to decide on quality control during the production process in their case study of the Ethiopian cement industry, and so on.
The concept of the monitoring process was initially introduced in Shewhart's control chart (Citation1931). The quality of the Shewhart control chart is only effectively achieved by identifying large shifts in the process parameters. There are many viable alternatives to well-known and efficient technology for detecting and monitoring slight changes in processes. The cumulative sum (CUSUM) control chart was created by Page (Citation1954), and Montgomery (Citation2009) provided more information on the chart's specifics. The CUSUM control chart was employed in the current investigation because of its greater capacity to identify minor parameter adjustments and to benefit from the advantages of employing an upper-sided control chart. The exponentially weighted moving average (EWMA) control chart was suggested by Roberts (Citation1959), and it is generally recognised that it may be used to monitor and identify slight changes. After that, several researchers proposed a variety of different control charts utilising the EWMA control chart development to improve the ability to detect slight changes. The modified exponentially weighted moving average (modified EWMA) control chart was devised by Khan et al. (Citation2017) after being initially proposed by Patel and Divecha (Citation2011). The extended exponentially weighted moving average (extended EWMA) control chart was suggested by Naveed et al. (Citation2018). The extended EWMA statistics, which can detect minor changes faster than the EWMA control chart shown in Karoon et al. (Citation2022) and Karoon et al. (Citation2023), were created by adding the EWMA statistics with the exponential smoothing parameters. This approach was shown to be more successful at recognising tiny changes. Additionally, Shamma and Shamma (Citation1992) introduced the double exponentially weighted moving average (double EWMA) control chart before Mahmoud and Woodall (Citation2010) offered it. The double EWMA control chart performs better than the EWMA control chart when the monitor process is utilised with small shift sizes rapidly. It is now generally acknowledged that a reliable control chart is essential for quickly detecting subtle process changes.
The average run length (ARL) is frequently utilised to assess control chart performance. The ARL is separated into two states; the ARL is ARL0, which is described by the value as it happens from the in-control state and should be large, and ARL1, which is explained by the value as it occurs from the out-of-control stage and should be as tiny as feasible. A variety of methods can be employed to estimate the ARL of the control chart, including Monte Carlo and Markov Chain. Moreover, there is Numerical Integral Equations (NIE) method (see Karoon et al., Citation2021). Also, Areepong mentioned the NIE method for SPC in 2009. For quality control, it was used to identify the distribution change point. To evaluate the principal components of EWMA control charts for various distributions, NIE was proposed to derive a numerical algorithm and infer precise analytical formulas. In addition, ARLs were calculated using explicit formulas. There is a large body of literature that cites the explicit ARL formulas for control charts with time series models. Due to reality, the control chart's performance may suffer if the observations are serially associated. For this reason, the ARL has been suggested by several researchers when there is a serial correlation in the observations. Areepong and Sukparungsee (Citation2016) proposed an explicit formula for the ARL on the EWMA control chart with the ARIMA model. After that, Sunthornwat et al. (Citation2017) derived the analytical ARL on the EWMA control chart for a long-memory autoregressive fractionally integrated moving average (ARFIMA) model. On the EWMA control chart, Peerajit et al. (Citation2017) proposed the explicit formulas for the derivation of the ARL using the data are the ARFIMA model on the CUSUM chart. Later, The capacity of the ARL was put out by Supharakonsakun et al. (Citation2020), and it was obtained from an explicit formula for the MA(1) model of the modified EWMA control chart. Supharakonsakun (Citation2021) proposed an explicit formula of the ARL with observations of the MA(p) model. After that, Phanyaem (Citation2022) presented an explicit formula and NIE method of the ARL for the SARX(p,r)L model based on the CUSUM control chart. Petcharat (Citation2022) proposed the ARL of the CUSUM control chart when the data are SARX(p)L model. Moreover, Karoon et al. (Citation2022) proposed an explicit formula of ARL on the extended EWMA control chart when the observations are the AR(p) model. Recently, Peerajit and Areepong (Citation2023) proposed the ARL of an autoregressive fractionally integrated process with exponential white noise running on the modified EWMA control chart.
As was already indicated, these results show that the ARL may be used to compare efficiency with control charts and that explicit formulas require considerably less calculation time to assess the ARL than the other approaches. However, the explicit formulas for the double EWMA chart have not yet been released. As a result, the focus of this research was on developing an ARL for using an AR model to monitor change in a time series. This method is based on the double EWMA chart with underlying exponential white noise in different scenarios. The ARL that was obtained by an explicit formula was provided. And then, that is expanded for comparison with the typical EWMA control chart. Besides, the ARL for them is also extended to compare processes with the diesel and natural gas price datasets.
2. Process identification on the EWMA and double EWMA control charts
2.1. EWMA control chart
Roberts (Citation1959) created the first suggestion for the EWMA chart. It is a tool that is typically used to monitor and identify slight changes in the process. The equation below can be used to express the statistics of the EWMA control chart:
(1)
(1)
where the EWMA chart parameter
is a sequence of autoregressive AR(p) model and a sequence data at
with exponential white noise,
is an exponential smoothing parameter
,
is the initial value of the EWMA statistics that is equal to
and the variance of
is
. Therefore, the upper control limit (UCL) and lower control limit (LCL) of the EWMA chart can be established from the mean
, the standard deviation
, and a suitable control width limit
as follows below:
(2)
(2)
The stopping time of the EWMA control chart is expressed as:
(3)
(3)
where
is the upper control limit, a constant parameter.
2.2. Double EWMA control chart
The double EWMA control chart was proposed by Shamma and Shamma (Citation1992) and Mahmoud and Woodall (Citation2010) made it available. It was adjusted by two exponential smoothing and then extended from the EWMA chart. The equation below may be used to describe the statistics of the double EWMA chart.
(4)
(4)
where the double EWMA control chart parameter
is a sequence of autoregressive AR(p) model and a sequence data at
with exponential white noise,
and
is an exponential smoothing parameter
,
is the initial value of the double EWMA statistics that is equal to
and the variance of
is
. Therefore, the upper control limit (UCL) and lower control limit (LCL) of the EWMA control chart can be established from the mean
, the standard deviation
, and a suitable control width limit
as follows below:
(5)
(5)
The stopping time of the EWMA control chart is expressed as:
(6)
(6)
where
is the upper control limit, a constant parameter.
However, values of the double EWMA statistics were replaced by 1, and the double EWMA statistics is the EWMA statistics.
2.3. Cumulative Sum control chart (CUSUM)
Page (Citation1954) introduced the CUSUM control chart in quality control for identifying minor variations in process mean. The equation below can be used to express the statistics of the CUSUM chart.
(7)
(7)
where a is non-zero constant,
is the initial value of CUSUM with
,
is upper control limit (UCL), and the stopping time of the CUSUM chart is defined as
(8)
(8)
2.4. The ARL on double EWMA control chart for time series with AR model
The time series is a collection of chronologically organised data. The time series is observed and studied in order to identify its patterns of change and development and forecast its future trend. Time-series data can be divided into two categories: stationary data and non-stationary data. The time-series data collection with stationary data does not show a trend or a seasonal influence. The data collection solely contains random error as a cause of variation, whereas non-stationary time-series data is a time-series data set that exhibits a trend or a seasonal effect. There are other sources of variation in the data set besides just random error. The Box–Jenkins method is currently the most complete and accurate algorithm for analysing and predicting time series data. Methods for measuring timed data are referred to as times series. Autoregression (AR), Moving Average (MA), Autoregressive Moving Average (ARMA), Autoregressive Integrated Moving Average (ARIMA), and Seasonal Autoregressive Integrated Moving-Average (SARIMA) are examples of common types.
One of the most commonly used models is the autoregressive model, which was also used in this study. Regression models, known as autoregressive (AR) models, have a dependent or response variable that is a linear function of its previous values. The autoregressive model has an important parameter r, p, which is the order of an autoregressive term and is often used for actual data. The AR(p) model is defined by the following equation:
(9)
(9)
where
is the constant of the model,
,
are coefficients of autoregressive
. It is assumed that the error term (
) is an exponential white noise (
). The probability density function of
is given as
;
, and then initial values of the autoregressive AR(p) model;
are equal to 1. In earlier studies, ARL formulae for monitoring change processes using data that were characterised as the AR model were developed utilising the equation of the AR model in Equation (9). For instance, Phanthuna et al. (Citation2021), and Karoon et al. used the AR model in Equation (9) to fit with ARL for the EWMA type control chart. For the double EWMA control chart running an autoregressive model under exponential white noise in this study, let
represent the ARL conditional on the initial value. The initial value for monitoring the double EWMA statistic
is established at
. Let
denote the induced expectation and probability measure associated with
at change-point (
) under the density function
. The ARL for AR(p) model on the double EWMA control chart defined with
can be described as follows:
where ARL0 refers to the in-control ARL with
, which denotes no change in the statistical process. On the other hand, ARL1 refers to the out-of-control ARL with
, which designates the first-time point in the statistical process at which a change occurs place from
to
.
3. The methodology and the performance measurement
3.1. Explicit formulas of ARL on Double EWMA control chart for AR model
In this section, the explicit formula of the ARL is solved on the double EWMA chart with the AR model. Let’s start by substituting Equation (9) into Equation (4) as follows:
when the first time
such that
is determined, then the initial values
and
equal to 1. The double EWMA statistics with AR(p) can be described as:
In control process, the interval of
between the lower and upper bound control limits are expressed to be
and
can be written as follows below.
On the variable
, this interval can be rewritten as:
The Fredholm integral equation is used to express the integral equation of the ARL on the double EWMA control chart for the AR(p) model with an initial value
. The equation represented is as follows:
If
is obtained by substituting the integration variable,
is restructured as follows:
(10)
(10)
As the function of
has an exponential distribution,
can be expressed as follows:
(11)
(11)
The ARL solution is verified by Banach's fixed-point theorem. This is considered an ARL solution in terms of its existence and uniqueness (see Sofonea et al., Citation2005), from Equation (11), suppose that
Consequently, the ARL solution that is provided in Equation (11) can be reformulated by establishing additional variables as follows:
(12)
(12)
After that, the integral equation
, which can be written as:
(13)
(13)
Finally, Equation (12) is substituted into the solution of
as shown in Equation (13), which is obtained as:
(14)
(14)
Since the AR(p) model is used with the double EWMA chart, the explicit ARL formula is given in Equation (14). Moreover, the in-control process is replace
with
, whereas the out-of-control process is replace
with
, as well as
, and
denotes the shift size in the monitoring process.
3.2. Numerical integral equation of ARL on double EWMA control chart for AR model
The numerical integral equation method, or NIE method, of the ARL is used to compare with the explicit formula in terms of the computational time spent. Let denote the ARL of the double EWMA control chart on the AR(p) model with an exponential white noise, with the ARL solution being computed according to the midpoint quadrature rule. Specifically, after selecting a quadrature rule, the interval
is divided into
using a set of constant weights
.
In accordance with the quadrature rule, the approximation for an integral is assessed as follows:
(15)
(15)
Let
represent a numerical approximation to the integral equation. It is the result of solving the following linear equations:
(16)
(16)
The m linear equation system is shown as
. If the inverse
exists, the unique solution is shown as:
where
,
is the unit matrix order m,
is a column vector of
, and
is a matrix, the definition of (m,mth) elements of R matrix is expressed as
Finally,
is instead of
into
, the approximation of the NIE method of the ARL is represented as follows:
(17)
(17)
where
is a set of the division point within the interval
for
and
is a weight of the composite midpoint formula;
.
3.3. The existence and uniqueness of the explicit formula for the ARL based on the double EWMA control chart
In this section, the existence and uniqueness of the ARL solution are confirmed using Banach's fixed-point theorem. Banach’s Fixed-point Theorem, sometimes referred to the contraction theorem, is concern with certain mappings of a complete metric space into itself. As we shall see, a fixed point is a point that is mapped to itself, and this statement establishes requirements adequate for the existence and uniqueness of a fixed point.
Theorem 3.1.
Banach’s Fixed-point Theorem:
Let be a complete metric space and let
be a contraction mapping on Y. Then T has a unique fixed point
(such that
) with contraction constant
such that
, for all
. Then there exists a unique
such that
, i.e. a unique fixed-point in
(Sofonea et al., Citation2005).
Proof:
Let T defined in Equation (11) is a contraction mapping for .
To show that for all
with
.
Consider:
where
3.4. The performance measurement of control chart
The performance of control charts is commonly evaluated in terms of the average run length (ARL). It is used to detect changes in the mean of the monitoring process. As a result, the performances of the ARL, which are produced by explicit equations, and the NIE technique, which is used to monitor shifts with an autoregressive model, or AR model, on the double EWMA chart, were compared.
The absolute percentage relative change (%APRC) indicates the relative performance of two methods of the ARL (Phanthuna & Areepong, Citation2022), which is defined as follows:
(18)
(18)
After that, the performance of the ARL is then evaluated with different parameter values based on the double EWMA chart. And then it is extended for comparison with the EWMA chart. The ARL measurement is appropriate to evaluate the effectiveness of control charts in the process parameters when shift sizes are different. The performance of a control chart throughout a range of shifts (
) can be evaluated using overall performance measures, which are recommended by several researchers. Some of them have metrics that are used to assess their performance, such as the average extra quadratic loss (AEQL) and the performance comparison index (PCI) (see Alevizakos et al., Citation2021).
The AEQL is described mathematically as
(19)
(19)
where the specific of shift in the process is represented by
, and then
is the sum of number of divisions from
to
. In the study,
is determined from
to
. The most effective control chart is those with the lowest AEQL values.
The PCI measurement is the ratio of the AEQL of the control chart and the AEQL of the most effective control chart (shown as the AEQLlowest). It is described mathematically as
(20)
(20)
The most effective control chart's PCI value is 1, whereas the values of the other control charts in competition are more than 1.
4. The results
A common criterion for assessing the efficacy of a control chart is the ARL for identifying alterations in the process. That is an evaluation of its practicality and sensitivity. In this study, the efficiency of the explicit formula and the NIE method for determining the ARL for monitoring shifts were compared. These were evaluated utilising the double EWMA chart and the autoregressive (AR) model. The AR(1), AR(2), and AR(3) autoregressive models were expressed. The number of division points m = 500 is determined for the approximation of the ARL by the NIE method. The solutions have been split into two categories: simulated and real-world data. For an initial ARL, In the in-control process scenario, ARL0 is followed by exponential white noise with a mean of . In the out-of-control process scenario, ARL1 was computed with parameter values as exponential white noise with a mean of
, as
, and then
equals 0.001, 0.002, 0.003, 0.005, 0.01, 0.03, 0.05, 0.1, 0.5, 1. As previously stated, “exponential white noise” is defined as the residual of an exponential distribution with uncorrelated data. As a result,
indicates that the process is in-control, whereas
indicates that the process is out-of-control. ARL1 could have a low value on the efficacy control chart. Also, ARL0 was determined at 370, and the Mathematica, spec Intel(R) Xeon(R) CPU X5680 @ 3.33 GHz 3.33 GHz (2 processors) RAM 32.0 GB was used to evaluate through study.
The process can be succinctly stated as follows:
Step 1: Input parameters for example, the coefficients of autoregressive;
, the initial values of the autoregressive;
, and the control chart parameters;
Step 2: Impose the parameter of exponential white noise;
for the in-control process and assign the parameter of exponential white noise;
for the out-of-control process.
Step 3: Determine the initial value of known parameter;
and the initial value of the double EWMA statistic;
.
Step 4: Specify the lower control limit (
) equals 0 and fixed the initial value ARL0 for computing compute the upper control limit (
).
Step 5: Compare the ARL of the explicit formula and the NIE method.
Step 6: Compute the solution of ARL1 for shift sizes in the monitoring process where
, and then the upper control limit (
) is the result of Step 4.
4.1. Simulated data
The aforementioned algorithm was used to determine ARL0 and ARL1, as well as to evaluate the efficiency of the proposed ARL by the explicit formula and the ARL by the NIE method in situations where the in-control and out-of-control processes were present. The results computed based on specified parameters such as and
for ARL0 = 370 using the ARL on the double EWMA chart with the AR(p) model as AR(1), AR(2), and AR(3) models obtained from two techniques are reported in Table . The coefficient parameters for the AR(1), AR(2), and AR(3) models were set as
,
,
, respectively. The ARL values of the explicit formula technique denote as
, and it is computed by using Equation (14). And then, the NIE method denotes as
, it is calculated by Equation (17). The %APRC of all situations is extremely low, nearly equal to 0. However, the computational times for the ARL values calculated using the NIE technique are about 3.7–4.3 s, while the explicit formula appears practically instantly for all situations. Therefore, using these explicit formulas as the next step is a good idea.
Table 1. Comparing the ARL values of the explicit formula against the NIE method for AR(p) models on the double EWMA control chart with the initial ARL0 = 370 and based on different situations.
Next, the effectiveness of the explicit ARL based on the double EWMA chart running on the AR(p) model is compared with the EWMA and CUSUM charts and then investigated using various . Moreover, the double EWMA chart serves as the EWMA chart if instead of
equals 1. ARL0 value was fixed as 370 for in-control situation. ARL1 values were used to compare the efficiency of the EWMA, double EWMA, and CUSUM charts and were set to values mentioned above. The results in Tables show that the lower
shows a decrease in ARL1, and the double EWMA chart (denoted as DEWMA) showed the effectiveness exceeding the EWMA and CUSUM charts for all situations. Additionally, the AEQL and PCI values are used to confirm their effectiveness. The AEQL values of double EWMA chart have lower than AEQL values of EWMA and CUSUM charts and the PCI values of double EWMA chart with
are equals 1, same for AR(1), AR(2), and AR(3).
Table 2. Comparing the ARL values on the double EWMA and EWMA control charts for the AR(1) model with different and determined
and
.
Table 3. Comparing the ARL values on the double EWMA and EWMA control charts for the AR(2) model with different and determined
and
.
Table 4. Comparing the ARL values on the double EWMA and EWMA control charts for the AR(3) model with different and determined
and
.
4.2. Real-world data
The prices of diesel and natural gas are shown in Figure , with the time series brought up for study in this part using the AR(1) and AR(2) models, respectively. Two datasets, which comprise quarterly price data, were selected as the models by SPSS. The results are in Table shows that two datasets are suitable parameters for the AR model. The Kolmogorov–Smirnov test was then used to determine that white noise significantly fits the exponential mean, as shown in Table . For the diesel price, it was fitted to the AR(1), which expressed as For the natural gas price, it was fitted to the AR(2), which expressed as
Figure 1. The time series of datasets (a) Diesel price with AR(1) and (b) Natural gas price with AR(2).

Table 5. The AR(p) coefficients for two datasets from the first quarter 2000 to the second quarter 2022.
Table 6. Testing the datasets for the exponential white noise of the exponential distribution.
Tables – show that the results of the comparison of control charts between the double EWMA and EWMA charts and the results show the double EWMA chart with the lower had a lower ARL1 and was more effective than the EWMA chart in all situations. However, the performance of CUSUM charts is not compared in this section because CUSUM charts give ARL1 values that are much higher than EWMA and EWMA charts. Moreover, the results show the outcomes of two datasets in a way similar to simulated data, as illustrated in Figure . The AEQL and PCI values supported the control chart's effectiveness. The results verify that the double EWMA chart with
had the lowest AEQL and PCI equals 1 outperformed the double EWMA chart with higher
and the EWMA chart, all of which have higher AEQL and PCI > 1, as illustrated in Figure .
Figure 3. The AEQL and PCI values of two models: (a) and (b) for the AR(1), (c) and (d) for the AR(2).

Table 7. Comparing the ARL values on the double EWMA and EWMA control charts for the AR(1) model of the diesel price ($/gallon) with different and given
and
.
Table 8. Comparing the ARL values on the double EWMA and EWMA control charts for the AR(2) model of the natural gas price ($/mcf) with different and given
and
.
Additionally, Figures and also show how well the control chart performs to detect shift changes in the monitoring process. For the AR(1) model in Figure , the double EWMA control chart () recognises shifts as being out of control at the first observation, whereas the EWMA control chart (
) does so at the 15th observation. For the AR(2) model in Figure , the double EWMA control chart (
) recognises shifts as being out of control at the first observation, whereas the EWMA control chart (
) does so at the 34th observation. The results show that in the monitoring process, the double EWMA control chart may detect shift changes faster than the EWMA control chart. Besides, An excellent option for spotting change is the double EWMA control chart, which performs better than the EWMA control chart. And then, the exponential smoothing parameter
is recommended for the double EWMA control chart.
5. Discussions and conclusions
The explicit formula for the ARL on the double EWMA control chart was put out in this study. It is faster than the NIE method in computing the ARL for the AR(p) model with exponential white noise. The proposed ARL is compared with the ARL on the EWMA control chart based on in-control and out-of-control scenarios. It was found that the double EWMA control chart's ARL consistently outperformed the traditional control chart's ARL. The double EWMA chart with the lower had a lower ARL1 and was superior to the EWMA chart in terms of effectiveness for all cases. The exponential smoothing parameter
is recommended. The efficacy of the control chart was validated by the AEQL and PCI values. Moreover, the performance of the ARL was expanded to include use with real-world datasets. The prices of diesel and natural gas were used as real-world datasets. The outcomes of two datasets are displayed in the findings in a style equivalent to simulated data. However, the double EWMA control chart can be expanded for future study in order to integrate with more models and cover more distributions.
Supplemental Material
Download MS Word (24.5 KB)Acknowledgements
The authors would like to express our gratitude to National Research Council of Thailand (NRCT) and King Mongkut’s University of Technology North Bangkok for supporting the research fund with contact no. N42A650318.
Disclosure statement
No potential conflict of interest was reported by the author(s).
Additional information
Funding
References
- Alevizakos, V., Chatterjee, K., & Koukouvinos, C. (2021). The triple exponentially weighted moving average control chart. Quality Technology & Quantitative Management, 18(3), 326–354. https://doi.org/10.1080/16843703.2020.1809063
- Areepong, Y., & Sukparungsee, S. (2016). An explicit expression of average run length of exponentially weighted moving average control chart with ARIMA(p,d,q)(P,D,Q)L models. Advances and Applications in Statistics, 49(5), 369–385. https://doi.org/10.17654/AS049050369
- Cheng, J., Huiyun, D., Guang, S., Peng, G., & Jianjun, Z. (2020). Application of ARIMA model in financial time series in stocks. 1st ed., https://doi.org/10.1007/978-3-030-57884_21
- Fugen, Z. (2019). Application of Shewhart control charts in the inspection of pharmaceutical manufacturing process. International Journal of Pharmacy and Chemistry, 5(1), 1–11. https://doi.org/10.11648/j.ijpc.20190501.11
- Karoon, K., Areepong, Y., & Sukparungsee, S. (2021). Numerical integral equation methods of average run length on extended EWMA control chart for autoregressive process. In T. Athanasios, J. Kaivo-oja, M. Sarrafzadeh, H. R. Arabnia, H. de Garis, G. Megson, E. Anarim, H. Jin, C.-H. Lee, R. P. Agarwal, H. Ammari, D. Motreanu, & H. Yang (Eds.), Proceeding of International Conference on Applied and Engineering Mathematics (pp. 51–56). IAENG.
- Karoon, K., Areepong, Y., & Sukparungsee, S. (2022). Exact solution of average run length on Extended EWMA control chart for the firstorder autoregressive process. Thailand Statistician, 20(2), 395–411.
- Karoon, K., Areepong, Y., & Sukparungsee, S. (2023). Trend autoregressive model exact run length evaluation on a two-sided extended EWMA chart. Computer Systems Science and Engineering, 44(2), 1143–1160. https://doi.org/10.32604/csse.2023.025420
- Khan, N., Aslam, M., & Jun, C. H. (2017). Design of a control chart using a modified EWMA statistic. Quality and Reliability Engineering International, 33(5), 1095–1104. https://doi.org/10.1002/qre.2102
- Mahmoud, A. A., & Woodall, W. H. (2010). An evaluation of the double exponentially weighted moving average control chart. Communications in Statistics – Simulation and Computation, 39(5), 933–949. https://doi.org/10.1080/03610911003663907
- Montgomery, D. C. (2009). Introduction to statistical quality control chart. John Wiley and Sons, Inc.
- Naveed, M., Azam, M., Khan, N., & Aslam, M. (2018). Design of a control chart using extended EWMA statistic. Technologies, 6(6), 108–122. https://doi.org/10.3390/technologies6040108
- Page, E. S. (1954). Continuous inspection schemes. Biometrika, 41(1-2), 100–115. https://doi.org/10.1093/biomet/41.1-2.100
- Patel, A. K., & Divecha, J. (2011). Modified exponentially weighted moving average (EWMA) control chart for an analytical process data. Journal of Chemical Engineering and Materials Science, 2, 12–20.
- Peerajit, W., & Areepong, Y. (2023). Alternative to detecting changes in the mean of an autoregressive fractionally integrated process with exponential white noise running on the modified EWMA control chart. Processes, 11(2), 503. https://doi.org/10.3390/pr11020503
- Peerajit, W., Areepong, Y., & Sukparungsee, S. (2017). Analytical explicit formulas of average run length for long memory process with ARFIMA model on CUSUM control chart. Songklanakarin Journal of Science and Technology, 39(6), 779–786. https://doi.org/10.14456/sjst-psu.2017.95
- Petcharat, K. (2022). The effectiveness of CUSUM control chart for trend stationary seasonal autocorrelated data. Thailand Statistician, 20(2), 475–488.
- Phanthuna, P., & Areepong, Y. (2022). Detection sensitivity of a modified EWMA control chart with a time series model with fractionality and integration. Emerging Science Journal, 6(5), 1134–1152. https://doi.org/10.28991/ESJ-2022-06-05-015
- Phanthuna, P., Areepong, Y., & Sukparungsee, S. (2021). Run length distribution for a modified EWMA scheme fitted with a stationary AR(p) model. Communications in Statistics – Simulation and Computation, https://doi.org/10.1080/03610918.2021.1958847
- Phanyaem, S. (2022). Explicit formulas and numerical integral equation of ARL for SARX(P,r)L model based on CUSUM chart. Mathematics and Statistics, 10(1), 88–99.
- Roberts, W. S. (1959). Control chart tests based on geometric moving averages. Technometrics, 1(3), 239–250. https://doi.org/10.1080/00401706.1959.10489860
- Satrio, C. B. A., Darmawan, W., Nadia, B. U., & Hanafiah, N. (2021). Time series analysis and forecasting of coronavirus disease in Indonesia using ARIMA model and PROPHET. Procedia Computer Science, 179, 524–532. https://doi.org/10.1016/j.procs.2021.01.036
- Shamma, S. E., & Shamma, A. K. (1992). Development and evaluation of control charts using double exponentially weighted moving averages. International Journal of Quality & Reliability Management, 9(6), 18–25. https://doi.org/10.1108/02656719210018570
- Shewhart, W. A. (1931). Economic control of quality of manufactured product. D. Van Nostrand Company, Inc.
- Shrestha, N. (2021). Application of statistical process control chart in food manufacturing industry. International Journal of Engineering, Business and Management, 4(5), 82–87. https://doi.org/10.22161/ijebm.4.5.2
- Sofonea, M., Han, W., & Shillor, M. (2005). Analysis and approximation of contact problems with adhesion or damage. Chapman & Hall/CRC.
- Sunthornwat, R., Areepong, Y., & Sukparungsee, S. (2017). Average run length of the long-memory autoregressive fractionally integrated moving average process of the exponential weighted moving average control chart. Cogent Mathematics, 4(1), 1358536. https://doi.org/10.1080/23311835.2017.1358536
- Supharakonsakun, Y. (2021). Statistical design for monitoring process mean of a modified EWMA control chart based on autocorrelated data. Walailak Journal of Science and Technology (WJST), 18(12), 19813. https://doi.org/10.48048/wjst.2021.19813
- Supharakonsakun, Y., Areepong, Y., & Sukparungsee, S. (2020). The exact solution of the average run length on a modified EWMA control chart for the first-order moving-average process. ScienceAsia, 46(1), 109–118. https://doi.org/10.2306/scienceasia1513-1874.2020.015
- Tegegne, D. A., Azene, D. K., & Atanaw, E. B. (2022). Design multivariate statistical process control procedure in the case of Ethio cement. International Journal of Quality & Reliability Management, 39(7), 1617–1636. https://doi.org/10.1108/IJQRM-07-2021-0227
- Yeganeh, A., Chukhrova, N., Johnnssen, A., & Fotuhi, H. (2023). A network surveillance approach using machine learning based control charts. Expert Systems with Applications, 219, 119660. https://doi.org/10.1016/j.eswa.2023.119660
- Zhang, Z., Mei, X., & Zhang, Q. (2022). Application of Shewhart control chart in controlling adverse events in patients with severe acute organophosphorus pesticide poisoning undergoing blood purification. Emergency Medicine International, 5 pages. https://doi.org/10.1155/2022/9655423