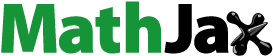
Abstract
Background: Scalp-related symptoms such as dandruff and itching are common with diverse underlying etiologies. We previously proposed a novel classification and scoring system for scalp conditions, called the scalp photographic index (SPI); it grades five scalp features using trichoscopic images with good reliability. However, it requires trained evaluators.Aim: To develop artificial intelligence (AI) algorithms for assessment of scalp conditions and to assess the feasibility of AI-based recommendations on personalized scalp cosmetics.Methods: Using EfficientNet, convolutional neural network (CNN) models (SPI-AI) ofeach scalp feature were established. 101,027 magnified scalp images graded according to the SPI scoring were used for training, validation, and testing the model Adults with scalp discomfort were prescribed shampoos and scalp serums personalized according to their SPI-AI-defined scalp types. Using the SPI, the scalp conditions were evaluated at baseline and at weeks 4, 8, and 12 of treatment.Results: The accuracies of the SPI-AI for dryness, oiliness, erythema, folliculitis, and dandruff were 91.3%, 90.5%, 89.6%, 87.3%, and 95.2%, respectively. Overall, 100 individuals completed the 4-week study; 43 of these participated in an extension study until week 12. The total SPI score decreased from 32.70 ± 7.40 at baseline to 15.97 ± 4.68 at week 4 (p < 0.001). The efficacy was maintained throughout 12 weeks.Conclusions: SPI-AI accurately assessed the scalp condition. AI-based prescription of tailored scalp cosmetics could significantly improve scalp health.
Introduction
Scalp-related symptoms, such as dandruff and pruritus, are considerably common. A dandruff survey conducted in China and the United States revealed dandruff prevalence rates of 30–40%, 66–82%, and as high as 81–95% among the Chinese, Caucasian, and African-American populations (Equation1(1)
(1) ). Furthermore, the prevalence of scalp pruritus is reported to be 13–45% (Citation2). These symptoms are caused by various underlying scalp conditions, which must be assessed accurately to enable personalized treatment. However, no systematic classification systems have been established for the scalp to describe diverse scalp characteristics and objectively quantify the overall conditions, unlike for glabrous skin (for which classification systems such as the Baumann skin type are widely used) (Citation3). One of the reasons is the inherent difficulty in directly observing the scalp. Several studies employed dermoscopy to observe the scalp structures in detail and designed various scalp assessment systems for their outcome measures. However, these approaches failed to provide a comprehensive evaluation and lacked validation (Citation4–8).
Therefore, we previously devised the scalp photographic index (SPI) to address this issue (Citation9). The SPI is an instrument that classifies the scalp types and scores the severity of the following five features of scalp conditions: (Equation1(1)
(1) ) dryness: fine lines or tiny scales on the scalp surface, (Citation2) oiliness: presence of greasy sebum or shine on the scalp surface, (Citation3) erythema: erythema or telangiectasia between the hair pores, 4) folliculitis: erythema or pustules of the hair pores, and (Citation5) dandruff: coarse and large white scales on the scalp surface. Based on magnified (× 60) scalp photographs and a standard photographic scale, the severity of these five features is graded as follows: 0 (none), 1 (mild), 2 (moderate), and 3 (severe). The sum of each score reflects the overall severity of the scalp problems and a feature of grade ≥2 enables the classification of the scalp types. We previously demonstrated that the SPI is correlated with the individual’s symptoms and the investigator’s visual evaluation. Furthermore, the SPI has excellent internal consistency and inter- and intra-rater reliability.
Using this system, physicians can provide treatments suitable for the specific scalp type and monitor the treatment response using the total SPI score. However, the wider application of the SPI is limited by the fact that grading requires trained evaluators. Automating the evaluation will enable a rapid and reliable assessment of scalp conditions; this will benefit not only healthcare providers, but also the beauty industry and general population. Furthermore, the automated system would also facilitate customization of scalp cosmetics.
In this study, we aimed to establish artificial intelligence (AI) algorithms to assess scalp conditions and evaluate whether they can be improved by an AI-based personalized prescription of scalp cosmetics.
Materials and methods
Development of AI algorithms for scalp photographic index scoring (SPI-AI)
Dataset
A total of 163,870 60-fold magnified images were acquired from 32,774 Koreans using a trichoscope (ASM-202TM camera, Aram Huvis Co., Ltd, Seongnam, Korea), and 126,948 images, excluding those where the scalp was obscured by hair, were included in the dataset. The demographics are summarized in . All images were uploaded to a web-based annotation program developed by HealthCloud Co., Ltd, (Seoul, Korea) for data cleaning, labeling, and review.
Table 1. Demographics of the 32,774 Koreans whose scalp images were used to develop the SPI-AI.
Step 1: Data cleaning
After removing duplicates and poor-quality images, a total of 101,027 images were selected.
Step 2: Data labeling (SPI) and review
For each image, SPI grading was initially performed by trained laypersons, and then by two experts (J. W. S. and H. R. C.) who subsequently reviewed the grades and one expert (J. I. N.) who confirmed the final grades.
Step 3: Training, validation, and test sets
Images for each scalp feature were divided into the training, validation, and test sets in a 7:2:1 ratio.
Algorithm training
Using EfficientNet-B7 which achieved the highest accuracy on the ImageNet classification task in 2019 and surpassed other convolutional neural networks (CNNs) (Citation10), we developed five CNN models SPI-AI that can classify each of the five scalp features into grades 0–3. We fine-tuned EfficientNet using PyTorch 1.7 for 50 epochs with a batch size of 128 (width coefficient 2.0; depth coefficient 3.1; default resolution 600; dropout rate 0.5). All the layers were frozen except the final fully connected layer.
Accuracy assessment
The accuracies of the five CNN models were assessed using the following equation:
(1)
(1)
For example, if the model predicted a grade 3 image as being a grade 3 image, the prediction was deemed correct. Conversely, if the model predicted a grade 3 image as being a grade 1 or 2 image, the prediction was deemed incorrect.
Study design and population
This prospective, single-center, pre–post comparative study was approved by the institutional review board (IRB No. B-2107-697-301) and was performed in accordance with the Declaration of Helsinki. All participants provided written informed consent prior to participation. The inclusion criteria were as follows: (Equation1(1)
(1) ) healthy adults aged >19 years and (Citation2) presence of scalp-related discomfort (such as itching, a sensation of heat, dandruff, excessive sebum, dryness, pain, or pimple). The exclusion criteria were as follows: (Equation1
(1)
(1) ) presence of active infectious scalp diseases and (Citation2) history of treatment with topical or systemic steroids, oral immunosuppressants, topical or systemic antibiotics, or antifungal agents that could affect the scalp conditions, within 4 weeks prior to the baseline. Furthermore, participants who had difficulty understanding or following the protocol were also excluded at the discretion of the investigator. In total, 100 participants met the eligibility criteria and were enrolled in this study. All participants had not been included in the previous image acquisition for training of SPI-AI.
For each participant, 60-fold magnified images were acquired from five standard areas (vertex, anterior, posterior, right, and left) of the scalp using a trichoscope; following an SPI-AI analysis, the scalp type was determined. A scalp feature of grade ≥2 was regarded as positive since it indicated clinically significant severity that needed treatment. Taken prescriptions for positive scalp features into account, the scalp types were classified into nine categories (normal, dry, oily, erythema, dry erythema, oily erythema, dry dandruff, oily dandruff, and folliculitis) as shown in .
Figure 1. Classification of scalp types. scalp photographic index-artificial intelligence (SPI-AI) diagnosed a feature of grade ≥2 as positive and classified the scalp type according to the positivity. aIf both dryness and oiliness were positive in one scalp area, only oiliness of grade 3 was considered positive. For example, a scalp with grade 2 or 3 dryness, grade 2 oiliness, grade 2 or 3 erythema could be defined as a dry erythema type.
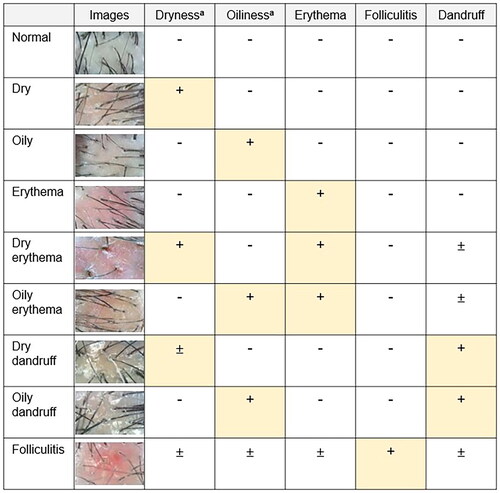
In cases when the scalp types of the five standard areas did not match each other, it was determined by setting priorities in the following order: folliculitis, oily erythema, dry erythema, oily dandruff, dry dandruff, erythema, oiliness, dryness, and normal.
For example, a scalp with grade 2 or 3 oiliness and dandruff could be defined as an oily dandruff type, whereas a scalp with grade 2 or 3 oiliness and folliculitis could be classified as a folliculitis type. If one area is classified as folliculitis and another area as oily erythema, a prescription for folliculitis is given. This is because the most numerous active ingredients are included in prescriptions for folliculitis.
Treatment
The personalized scalp cosmetics (shampoos and scalp serums) were manufactured by Aram Huvis Co. Ltd (Seongnam, Korea). The combination and concentrations of the ingredients in the shampoos and scalp serums were determined according to the patients’ scalp types classified by the SPI-AI analysis. Active ingredients included hyaluronic acid, dexpanthenol, inositol, piroctone olamine, allantoin, beta-glucan, and ceramide (Citation11–20).
Study visits occurred at the baseline and week 4; during these, compliance and history of adverse events were examined under investigator supervision. On day 1, all participants were dispensed the customized shampoo and scalp serum and instructed on their usage as below.
Soak your hair with lukewarm water before applying shampoo.
Start lathering up from the roots, and then massage down toward the tips.
After scrubbing the scalp gently, rinse the shampoo out thoroughly for a minimum of three minutes.
After shampooing, dry the scalp with a towel and apply the scalp serum gently to the entire scalp using fingers.
Thereafter, the agents were applied once daily for 4 weeks at home. The study was extended until week 12 for volunteers.
Participants who used other topical or systemic treatments concomitantly for scalp conditions during the trial period, those who applied the study medications for less than 8 d out of 4 weeks, and those who experienced adverse events and could not continue the trial were regarded as drop-outs at the discretion of the investigator. This clinical study was conducted from 27 July 2021 to 7 January 2022.
Outcomes
A single dermatologist (J.I.N.) performed the SPI evaluation at every visit using the trichoscopic images of the participants’ scalp. The images were obtained using the same device with identical settings at each visit, and the scalp positioning ruler was used to replicate the exact spot of each image (Supplementary Figure 1). The participants were instructed against washing their scalps or using the scalp serum within 12 h before the evaluation. The primary endpoint was the change from the baseline (CFB) in the total SPI score at week 4; the key secondary endpoints were the CFB in the total SPI score at weeks 8 and 12.
A seven-item questionnaire was used to assess the subjective improvement in scalp conditions (namely, dryness, oiliness, dandruff, itching, scalp pimple, pain, and heating) relative to the baseline. The participant’s satisfaction was evaluated as follows: 0 (unsatisfied), 1 (neutral), 2 (satisfied), and 3 (very satisfied). The secondary endpoints comprised the proportion of participants achieving improvement in more than one item on the questionnaire and the proportion of participants satisfied with the products at week 4. Furthermore, the participants were asked to report on any adverse events that occurred at any time during the treatment. The investigators also checked for adverse events during each follow-up visit.
Statistical analysis
Each measurement at the follow-up visit was compared with the baseline value using paired t-tests. The results are expressed as mean ± standard deviation, and a p value of <0.05 was considered statistically significant. IBM SPSS version 20 (IBM Corp., Armonk, NY) was used to analyze all data.
Results
Accuracy of SPI-AI
displays the SPI results of the 101,027 scalp images considered for algorithm training, validation, and testing. Dryness, oiliness, erythema, folliculitis, and dandruff were observed in 17,434, 80,416, 67,414, 4,592, and 40,482 of these images, respectively. A total of 811 images were graded 0 for all five features, implying good scalp conditions.
Table 2. Classification of 101,027 scalp images labeled with the severity grade based on the SPI scoring system.
Five CNN models, i.e., those for dryness, oiliness, erythema, folliculitis, and dandruff, were created. The training and test sets for each model and their accuracies are summarized in . Every model exhibited an accuracy of >85%; the dandruff model exhibited the highest accuracy (95.2%), followed by the dryness model (91.3%). Conversely, the folliculitis model exhibited the lowest accuracy (87.3%), possibly due to its smaller training set. When grades 0 and 1 were regarded as negative and grades 2 and 3 as positive, the sensitivity, specificity, and F1 score of each model were as follows: dryness (sensitivity 0.983, specificity 0.984, F1 score 0.984), oiliness 9, sensitivity 0.949, specificity 0.947, F1 score 0.944), erythema (sensitivity 0.839, specificity 0.999, F1 score 0.912), folliculitis (sensitivity 0.794, specificity 1.000, F1 score 0.885), dandruff (sensitivity 0.980, specificity 0.968, F1 score 0.964) (Supplementary Figure 2).
Table 3. Accuracy of the SPI-AI.
Participant characteristics
All the 100 participants enrolled in the study completed the treatment. The mean age was 34.90 ± 7.85 years (range, 19–61 years), and women accounted for 69% of the study population. The mean total SPI score at the baseline was 32.70 ± 7.40 (range, 15–50). The scalp types were defined by SPI-AI, and were as follows: folliculitis, 73%; oily dandruff, 12%; oily erythema, 7%; dry erythema, 4%; dry dandruff, 3%; and erythema, 1% (Supplementary Table 1). Forty-three participants (mean age, 36.40 ± 8.34 years) further participated in the extension study and completed the schedule until week 12.
Primary and secondary endpoints
The CFB in the total SPI score was from 32.70 ± 7.40 to 15.97 ± 4.68 at week 4 (p < 0.001), and all scalp subtypes showed consistent results (). Participants enrolled in the extension study also demonstrated a significant decrease in the total SPI score. The CFB in the total SPI score was from 31.42 ± 8.33 at baseline to 15.33 ± 4.65 and 16.05 ± 4.80 at weeks 8 and 12, respectively (p < 0.001 for both; ). shows the photographs of two representative cases that presented a marked improvement at week 4.
Figure 2. Sixty-fold magnified scalp photographs of two representative cases with significant improvement in the scalp photographic index (SPI) from before to after treatment. (a) S10 (29-year-old man, folliculitis type): the total SPI score decreased from 38 at the baseline to 14 at week 4. (b) S31 (26-year-old man, oily erythema type): the total SPI score decreased from 33 at the baseline to 16 at week 4.

Table 4. Primary outcomes after 4 weeks of treatment.
Table 5. Changes from the baseline in the total SPI score at weeks 4, 8, and 12 in patients who participated in the extension study.
At week 4, 93% of the participants achieved an improvement in more than one items on the questionnaire. Furthermore, 63% and 1% of the participants rated the customized products as satisfactory and unsatisfactory, respectively; 31% of the participants had a neutral opinion about these products.
Adverse events
Six of the 100 participants (6.0%) enrolled experienced aggravation of symptoms, such as itching, scalp pimples, pain, and a sensation of heat. All complications were mild, and discontinuation of the study and rescue treatment was not required. Four of the aforementioned six participants recorded improvements in symptoms other than the aggravated item on the questionnaire; five of these participants further evaluated the customized products as satisfactory. No other local adverse events occurred in the treatment area.
Discussion
Although scalp-related symptoms are considerably common, studies on their etiopathogenesis and treatment are limited (Citation2, Citation21). Dandruff, one of the most common complaints of patients visiting dermatological clinics, is often considered an inflammatory condition related to Malassezia spp. (sebum-dependent yeasts); accordingly, antifungal agents are typically administered to patients (Citation22). However, in a Korean study, approximately 46% of the patients with dandruff had dry scalps, with sebum levels lower than those of normal controls; this suggested differences in the etiopathogenesis between dry and oily dandruff (Citation23). Many patients choose cosmetic products that are inappropriate for their scalp condition.
One of the reasons why scalp conditions have not been widely studied is that they are difficult to observe (Citation8). People cannot directly observe their own scalps, and photographing the scalp is difficult due to hair coverage. Magnified scalp photographs facilitate an easier analysis of scalp conditions covered with hair; this not only allows the observation of both the hair follicles and inter-follicular scalp, but also renders it possible to determine whether the scalp problem has affected the follicle or the inter-follicular epithelium. We used a trichoscope, a dedicated camera for hair and scalp imaging, to capture the scalp images in our study. Unlike dermoscopes, trichoscopes are fixed-focusing, allowing non-experts to easily capture well-focused photos. They are cheaper than dermoscopes; therefore, they are potentially more affordable to both healthcare providers and the general population. The use of a dedicated camera for the scalp also enables the capture of standardized photographs, thereby facilitating the automation of image evaluation.
In this study, over 100,000 scalp images were collected and graded according to each feature’s severity. Through this unprecedented effort, it was possible to train an AI algorithm (SPI-AI) to automatically evaluates scalp conditions; this algorithm exhibited an excellent performance, despite using a simple training technique. The SPI-AI could classify scalp conditions by detecting the presence or absence of five common scalp symptoms (dryness, oiliness, erythema, folliculitis, and dandruff) from magnified scalp images; this facilitated the identification of an individual’s scalp type, which can be used for recommending a suitable product and administering tailored treatment for improved scalp health.
Although some researchers have proposed a deep learning-based inspection of the scalp (Citation8), studies on the feasibility of AI usage in clinical care settings have been sparse to date. In this study, we demonstrated a significant improvement in the condition of all scalp subtypes after prescribing personalized care according to the algorithm’s classification of the scalp types. Subjective evaluations revealed high participant satisfaction. The participant satisfaction and high treatment adherence were in part attributable to standardized scalp photography, which provided objectivity and consistency to the evaluations (Citation24, Citation25).
Our study has some limitations. These include non-comparability and a lack of biophysical measurements. Further randomized studies with pre- and post-treatment biophysical measurements and a long-term follow-up will help establish the validity of our findings. Given that this study focused on Koreans, studies with larger populations with different skin types are required to obtain generalizable results and advance the performance of the AI-based model.
In conclusion, we developed a deep-learning algorithm trained using a substantial number of magnified scalp images and confirmed its ability to both accurately grade the severity of five scalp-condition features and diagnose the scalp type. Our data suggest that SPI-AI potentially provides an automated, prompt, and accurate diagnosis of an individual’s scalp condition; thus, this tool may be useful in suggesting personalized and more-tailored treatments for improved scalp health. We foresee a widespread use of this tool by the hairdressing industry and the general public owing to its automated nature.
Supplemental Material
Download Zip (701.7 KB)Acknowledgments
IRB approval status: reviewed and approved by the Institutional Review Board of Seoul National University Bundang Hospital (IRB No. B-2107-697-301).
Disclosure statement
In accordance with Taylor & Francis policy and my ethical obligation as a researcher, I am reporting that Aram Huvis Co., Ltd (Seongnam, Korea) provided a trichoscope (ASM-202TM) and manufactured the personalized shampoo and scalp serum that were all used in this study. I have disclosed these interests fully to Taylor & Francis, and have in place an approved plan for managing any potential conflicts arising from this arrangement.
Data availability statement
For any purpose, with investigator support, anyone can request the data through email-id [email protected]
Additional information
Funding
References
- Hay RJ. Malassezia, dandruff and seborrhoeic dermatitis: an overview. Br J Dermatol. 2011;165(2):1–8. doi: 10.1111/j.1365-2133.2011.10570.x.
- Rattanakaemakorn P, Suchonwanit P. Scalp pruritus: review of the pathogenesis, diagnosis, and management. Biomed Res Int. 2019;2019:1268430–1268411. doi: 10.1155/2019/1268430.
- Baumann L. The skin type solution: a revolutionary guide to your best skin ever. New York (NY): Bantam Books; 2006.
- Satchell AC, Saurajen A, Bell C, et al. Treatment of dandruff with 5% tea tree oil shampoo. J Am Acad Dermatol. 2002;47(6):852–855. Dec doi: 10.1067/mjd.2002.122734.
- Trznadel-Grodzka E, Błaszkowski M, Rotsztejn H. Investigations of seborrheic dermatitis. Part II. Influence of itraconazole on the clinical condition and the level of selected cytokines in seborrheic dermatitis. Postepy Hig Med Dosw (Online). 2012;66:848–854. doi: 10.5604/17322693.1019648.
- Mubki T, Rudnicka L, Olszewska M, et al. Evaluation and diagnosis of the hair loss patient: part II. Trichoscopic and laboratory evaluations. J Am Acad Dermatol. 2014;71(3):431 e1–431 e11.
- Park DS, Koo JE, Oh SY, et al. Physiological profiles of hair and scalp conditions in korea population: survey, hairological expert-diagnosis and new diagnostic device. Adv Bioeng Biomed Sci Res. 2021;4(2):63–71.
- Chang WJ, Chen LB, Chen MC, et al. ScalpEye: a deep learning-based scalp hair inspection and diagnosis system for scalp health. IEEE Access. 2020;8:134826–134837. doi: 10.1109/ACCESS.2020.3010847.
- Kim BR, Won SH, Kim JW, et al. Development of a new classification and scoring system for scalp conditions: scalp photographic index (SPI). J Dermatolog Treat. 2023;34(1):2181655.
- Tan M, Le Q. Efficientnet: rethinking model scaling for convolutional neural networks. Proc Mach Learn Res. 2019;97:6105–6114.
- Burnett CL, Boyer IJ, Bergfeld WF, et al. Safety assessment of ceramides as used in cosmetics. Int J Toxicol. 2020;39(3):5S–25S. doi: 10.1177/1091581820958692.
- Chiu CH, Huang SH, Wang HM. A review: hair health, concerns of shampoo ingredients and scalp nourishing treatments. Curr Pharm Biotechnol. 2015;16(12):1045–1052. doi: 10.2174/1389201016666150817094447.
- Dall’ Oglio F, Tedeschi A, Fusto CM, et al. A novel cosmetic antifungal/anti-inflammatory topical gel for the treatment of mild to moderate seborrheic dermatitis of the face: an open-label trial utilizing clinical evaluation and erythema-directed digital photography. G Ital Dermatol Venereol. 2017;152(5):436–440.
- Davis MG, Thomas JH, van de Velde S, et al. A novel cosmetic approach to treat thinning hair. Br J Dermatol. 2011;165(3):24–30. doi: 10.1111/j.1365-2133.2011.10633.x.
- Du B, Bian Z, Xu B. Skin health promotion effects of natural beta-glucan derived from cereals and microorganisms: a review. Phytother Res. 2014;28(2):159–166. doi: 10.1002/ptr.4963.
- Ebner F, Heller A, Rippke F, et al. Topical use of dexpanthenol in skin disorders. Am J Clin Dermatol. 2002;3(6):427–433. doi: 10.2165/00128071-200203060-00005.
- Guerra-Tapia A, Gonzalez-Guerra E. Sensitive scalp: diagnosis and practical management. Actas Dermosifiliogr. 2023;114(2):141–146. doi: 10.1016/j.ad.2022.09.009.
- Lodén M, Wessman C. The antidandruff efficacy of a shampoo containing piroctone olamine and salicylic acid in comparison to that of a zinc pyrithione shampoo. Int J Cosmet Sci. 2000;22(4):285–289. doi: 10.1046/j.1467-2494.2000.00024.x.
- Rapaport M. A randomized, controlled clinical trial of four anti-dandruff shampoos. J Int Med Res. 1981;9(2):152–156. doi: 10.1177/030006058100900213.
- Trüeb RM. Shampoos: ingredients, efficacy and adverse effects. J Deutsche Derma Gesell. 2007;5(5):356–365. doi: 10.1111/j.1610-0387.2007.06304.x.
- Meray Y, Gençalp D, Güran M. Putting it all together to understand the role of malassezia spp. in dandruff etiology. Mycopathologia. 2018;183(6):893–903. doi: 10.1007/s11046-018-0283-4.
- Borda LJ, Wikramanayake TC. Seborrheic dermatitis and dandruff: a comprehensive review. J Clin Investig Dermatol. 2015;3(2). doi: 10.13188/2373-1044.1000019.
- Yoon J, Shim J, Lim J, et al. Biophysical characteristics of dandruff-affected scalp categorized on the basis of sebum levels. J Cosmetic Dermatol. 2021;20(3):1002–1008. Mardoi: 10.1111/jocd.13626.
- Pathoulas JT, Flanagan KE, Walker CJ, et al. Evaluation of standardized scalp photography on patient perception of hair loss severity, anxiety, and treatment. J Am Acad Dermatol. 2021;85(6):1640–1641. Dec doi: 10.1016/j.jaad.2020.12.059.
- Lee S, Lee H, Lee CH, et al. Photographic assessment improves adherence to recommended follow-up in patients with androgenetic alopecia and alopecia areata: a retrospective cohort study. Indian J Dermatol Venereol Leprol. 2019;85(4):431–433. doi: 10.4103/ijdvl.IJDVL_696_18.