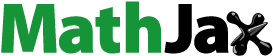
Abstract
Mega Railway Projects (MRPs) are expensive and account for an increasing percentage of many a nation’s annual infrastructure expenditure. These MRPs frequently exceed their budget and schedule. The challenge of achieving reliability or availability targets stands out as a contributing factor to these overruns. A robust and targeted Reliability, Availability, and Maintainability (RAM) process, which covers systems and subsystems that comprise the railway, that is imbedded in the project from the outset and that is managed throughout the life cycle of the project, is crucial for success. However, a RAM process for MRPs is not readily available. While BS EN 50126-1Footnote1 sets out the required RAM related tasks there is no guidance on how these tasks are to be undertaken or managed. This omission is likely to increase the challenge faced by RAM or Systems engineers as they put forth their case for ring-fenced funds and labour at the outset of an MRP. It is therefore important that RAM on an MRP is reviewed so that next steps in developing robust RAM process plan guidelines can be determined. The authors of this paper discuss why RAM is undertaken and the conceptualisation of RAM along with its fundamental features. Its application on railways focusing on RAM techniques and BS EN 50126-1 is outlined. A Systematic Literature Review (SLR) is undertaken to show the state-of-the-art by using a meta and content analysis within the context of railway systems, RAM techniques, RAM standards and Reliability levels. Furthermore, a set of Derived RAM requirements (DRR) based on BS EN 50126-1 are derived to determine the critical areas of RAM and are thus recommended for further development by researchers or RAM practitioners.
1. Introduction
1.1. Background
Mega projectsFootnote2 frequently exceed projected cost and programme allowances, do not fulfil their requirements and generate insufficient operational income (Davies et al., Citation2014). They are demanding to manage, often fail to achieve their objectives (Denicol et al., Citation2020) and are deemed complex (Denicol et al., Citation2020).
A complex project carries uncertainty, is everchanging and has unpredictable political, social and economic elements. Other types of projects, which are self-contained, comprehensible and predictable (Chapman, Citation2016) are considered complicated.
More and more Megaprojects are now being built with greater financial value (Flyvbjerg, Citation2017). Research on MRPs has been undertaken on the themes of:
systems integration (Gholz et al., Citation2018; Hobday et al., Citation2005; Muruganandan et al., Citation2022; Prencipe, Citation2003; Whyte & Davies, Citation2021; Whyte et al., Citation2022),
innovation (Davies et al., Citation2009; Davies et al., Citation2019; Davies et al., Citation2014; Worsnop et al., Citation2016),
organisation and management (Davies et al., Citation2017; Denicol & Davies, Citation2022; Denicol et al., Citation2020; Denicol et al., Citation2021; Flyvbjerg, Citation2017; Wright et al., Citation2017).
However, RAM as a single discipline is not explicitly covered, hence it is a fertile ground for developing new knowledge and knowhow. This paper deals with the RAM contribution and undertaking on an MRP.
MRPs have special characteristics (Chapman, Citation2016). These are a function of:
the quantity and variety of systems under consideration, e.g., signalling, rolling stock,
the many legal entities involved and that require management,
wide ranging social and environmental implications,
the large number of diverse stakeholders, many with conflicting requirements, and
the volume of interfaces and interactions between them (Locatelli et al., Citation2014).
In fact, The Infrastructure and Projects Authority (IPA) views projects within the Department for Transport’s (DfT) remit as having the highest whole life cost and the greatest risk of failing to deliver projects on time and to scope (Institute For Government, Citation2020). Railway projects top the list.
Railways are Systems of Systems (SoSFootnote3) (Hoehne, Citation2016) that perform a transport function. A system is regarded as a whole comprised of interacting parts or an integrated set of elements and subsystems, e.g., hardware, software, processes, people, facilities, services (INCOSE, Citation2015), which deliver an objective.
A railway system includes many systems and elements. , adapted from British Standards (Citation1999), offers guidance for visually decomposing a Guided Transport System (GTS). The main elements, attributes and contents of a railway system have been outlined. Content from the Crossrail (CRL) MRP, now known as the Elizabeth Line (Wright et al., Citation2017), was added by the authors.
Complex projects apply Systems Engineering (SE) techniques, (Mabelo & Sunjka, Citation2017) especially in railways (INCOSE, Citation2014). These SE techniques arrange and consolidate system and project elements by engaging in an iterative process and applying an interdisciplinary approach (INCOSE, Citation2015).
The ‘Vee’ model, introduced by Forsberg and Mooz (Citation1991), is a helpful way to visualise the sequential process of SE, with its focus on the verification and validation of the stakeholders’ requirements. It systematically presents the SE activities required during the lifecycle stages (INCOSE, Citation2015). A typical example is shown in (CENELEC, Citation2017).
The different colours in are used to designate responsibility. Stages 1–10 are managed by the project team who then hand over the system to the railway’s infrastructure manager and operator for stages 11 and 12. The SE process requires various types of activities. RAM engineering is one of the specialty engineering activities required under SE (Wasson, Citation2006; INCOSE, Citation2015).
In MRPs, where SE is deployed, the Railway RAMS Management standard BS 50126-1 is applicableFootnote4 (Muruganandan et al., Citation2022). The standard describes a systematic RAMS management process applied by railway authorities in the UK, e.g., Transport For London (TfL) and Network Rail (NR) to any new system or change to a railway configuration (CENELEC, Citation2017). Considered in greater detail in 1.5.
Unfortunately, there is little current knowledge and knowhow on the practicalities of applying the standard’s framework. Given that MRPs are littered with uncertainty and challenges, and having identified this limitation, we present this paper on the state-of-the-art for RAM in railway mega projects.
The authors critically survey the knowledge in literature, analyse and synthesise current thinking, identify gaps in the knowledge required to implement RAM management in MRPs. An SLR process was adopted. The following research questions were considered (Kraus et al., Citation2020):
1.2. Research questions
Research Question 1 (RQ1) – What is RAM management as applied on railway projects?
The concept of RAM management and its application in railways is discussed in Sections 1.3–1.5.
Research Question 2 (RQ2) – What is state-of-the-art of RAM application and systems coverage on railway projects?
The authors answer this question starting with the search strings in journals and databases and extraction with a meta and content analysis.
Research Question 3 (RQ3) – What is needed to deliver MRPs successfully, in terms of RAM management?
We answer this question using Derived RAM Requirements (DRR) measuring importance and consolidate using valid papers and gaps found during our assessments.
1.3. Content and methodology
The main process and content of the paper is illustrated in . A literature review process was used, which successfully verified and narrowed the scope of the research question(s) (Kitchenham, Citation2007).
Section 1 outlines the research and scope of the paper. The background, the need for the paper and the review questions are presented. Further, RAM management and its application to railways is introduced, and key RAM terms are defined (Baker, Citation2000). Section 2 describes the SLR process (Baker, Citation2000), including the search terms and filters applied. In Section 3, data from the relevant papers is extracted through meta-analysis and combining subcategories quantitatively. Arguments are formed surrounding this data to provide content analysis. In parallel and using the SLR in Section 4, critical areas of RAM are identified using Derived RAM Requirements (DRR), a survey and a final assessment. Overall discussion, conclusions and summary are presented in Sections 5 and 6. The scope covers railway systems, RAM techniques & management. Exclusions are; safety, maintenance, Life Cycle Cost (LCC) and operations.
1.4. Conceptualisation of RAM management
The conceptualisation of a topic is recommended before starting a Literature Review (LR) search (Vom Brocke et al., Citation2009). A concept map can be used to identify key search terms for a LR, to clarify thinking around theoretical concepts and the relationships between them (Rowley & Slack, Citation2004). is just such a concept map for RAM derived and consolidated by the authors from publications in this field (Birolini, Citation2013; Dhillon, Citation2006; Eduardo Calixto, Citation2016; Enrico Zio, Citation2018; Kececioglu, Citation2002; Smith, Citation2017).
1.4.1. Definitions of RAM terminology
1.4.1.1 Reliability
‘[The] ability to perform as required, without failure, for a given time interval, under given conditions’. BS EN 50126-1 (CENELEC, Citation2017).
1.4.1.2. Maintainability
‘[The] ability to be retained in, or restored to, a state to perform as required, under given conditions of use and maintenance’ BS EN 50126-1 (CENELEC, Citation2017).
1.4.1.3. Availability
‘[The] ability of an item to be in a state to perform a required function under given conditions at a given instant of time or over a given time interval, assuming that the required external resources are provided’ BS EN 50126-1 (CENELEC, Citation2017).
1.4.2. Goals and objectives of RAM
The goals and objectives of RAM are shown in , Overall, the aim of RAM is to increase operating time, design life, and availability. Conversely it is to decrease the need for corrective actions caused by failures and the resulting downtime and cost for rehabilitation. When the system has failed and action is needed, it is usually corrected by the replacement or repair of a part that is defective (Ebeling, Citation1997). Finding the root causes of defects is important (Denniss, Citation2017) to eliminate the recurrence of failures. To achieve the goals, RAM objectives, or tasks, are enacted, e.g., to study, analyse, predict, monitor, measure and demonstrate the performance of the system, known as Reliability Engineering or RAM engineering (Smith, Citation2017).
illustrates this further. A power system is taken through a typical system and RAM life cycle (Smith, Citation2017), with the RAM concept added by the authors. The RAM goals will be achieved if RAM is applied successfully.
1.5. Application of RAM in railways
1.5.1. Introduction
RAM is widely applied across many industries, including defence, space, nuclear oil and gas (Eduardo Calixto, Citation2013; Ebeling, Citation1997; Enrico Zio, Citation2018; Kececioglu, Citation2002). Different industries use different RAM standards and guidelines. Defence, in the USA, has DOD standards (Department of Defense, Citation2005) and an array of Military Standard (MIL) handbooks. NASA has various technical standards (NASA, Citation2006). The oil and gas industries use ISO standards (CEN ISO/TR 12489:2016, Citation2016). This is evidence that the industry includes the RAM management process in projects and support this by guidelines.
Literature also confirms that RAM is applied to railway systems, e.g., rolling stock (Kwansup Lee et al., Citation2017; Lee et al., Citation2007; Puntis & Walley, Citation2007), signalling (Hwang & Jo, Citation2008), traction power (Hayashiya et al., Citation2017), track infrastructure; track and switches (Ghodrati et al., Citation2016). Railways have their own RAM standard, BS EN 50126-1 (Rajabalinejad et al., Citation2020). The standard provides a systematic RAMS management process for the railway sector (CENELEC, Citation2017). In Europe the standard is used as EN 50126-1. The remainder of this section describes the application of RAM in railways.
1.5.2. System engineering life cycle, RAM tasks and documents
The authors added BS EN 50126-1 RAM related tasksFootnote5 onto the SE lifecycle in . For example, Stage 1, RAM requirements and policy, Stage 5 Apportionment to systems and subsystems. Verification is needed at each stage. The standard provides RAM related tasks, however, it omits guidelines or details on how tasks are undertaken (E Calixto, Citation2014a, Citation2014b).
A suite of documents are created e.g., RAM plans and RAM analysis. For RAM policy, the duty holder’s RAM targets are investigated. Later activities, such as testing reliability and reliability growth (CENELEC, Citation2017) are provided in RAM validation and integration or demonstration documents. A RAM case is built up incrementally by assembling evidence from all subsystems. In MRPs, due to their staged handovers, the overall RAM case can be broken down into smaller cases by section/area/function. The case needs to be set out early to bank as much evidence as practicable, allowing for early reliability growth and accumulation of time/operation/stress testing on subsystems. Demonstrating RAMs at Stage 10 on the railway system prior to handover is challenging. This can be due to, for example:
difficulty achieving the final configuration of all subsystems, so the railway system is in final configuration;
lack of operational time/operations;
high MTBF targets;
railway authority risk appetite e.g., if low risk more operational hours/operations are required.
To help mitigate for this, handover should be staged with subsystems that require less integration handed over as soon as practical. Planning the amount and type of RAM testing/demonstration, including operational time/operations needed for subsystems and railway system to meet reliability targets, should be defined at an early stage e.g., Stage 4. This work should be fully managed, costed and integrated into the project schedule, thereby increasing the chance that it survives the inevitable financial and scheduling pressures on an MRP. On MRPs, subsystems become overlapping Vees within Vees in the overall system lifecycle. This makes the RAM tasks even more challenging. On final completion of all subsystems a ‘real test environment’ is created for the railway for final integration. At this time ‘fine tuning’ of software multiple technologies of the subsystems is required, but this adds time on the programme. Definitions within the paper are provided in Appendix F.
1.5.3. RAM techniques
RAM techniques, or methods, are applied to undertake RAM tasks. They are described by many authors, (Birolini, Citation2013; Kececioglu, Citation2002; O’Connor & Kleyner, Citation2012; Smith, Citation2017) and applied at different stages of the life cycle.
Railway projects following BS EN 50126-1 are directed to its Appendix D for RAMS technique guidance and application. We further explain how a Railway RAM practitioner would be guided on RAMS techniques following the standard in . This lists the selection of RAMS techniques, under 5 headings, depicting which area of the process needs to be developed. Guidance is provided under each heading. However, headings 1, 3 and 5 we discount on the basis that heading 1 refers to EN 61160, which is only a design review guideline suggesting no techniques. Headings 3 and 5 are for Engineering Safety and Life Cycle Cost (LCC). Safety is not part of this review due to the large size of the topic and its LCC, which leaves RAM analysis (2) and RAM testing (4) for further explanation in this section.
For RAM analysis we can summarise the following.
1.5.3.1. Commonly used RAM analysis procedures
EN 60300-3-1 for guidance on analysis procedures. Dependability standard EN 60300 is a major industry standard. Part 3-1 offers guidance on techniques. It defines analysis techniques applicable (6 off) or supporting (6 off). Applicable means a technique is recommended for the complete RAM task, while supporting is applicable but not a stand-alone method for the task. The further supporting analysis is also detailed, e.g., maintainability studies, variation simulation modelling (VSM) software reliability engineering (SRE), for which there are many software models, e.g., Goel-Okumoto Mode (Haque & Ahmad, Citation2021). We provide descriptions of these techniques in Appendix D.
1.5.3.2. Various RAM analysis
We can detail the following.
other analysis techniques: fault tree, FMEA, RBD, and Markov and the respective standards, which are the same applicable techniques mentioned above.
supporting statistical RAM data: reference MIL-HDBK – 217F, reliability prediction of electronic components, contains two methods: parts stress and parts count. Again, they are the same as the failure rate prediction methods presented in the dependability standard (EN 60300-3-1). EN 61709 is a standard for reliability that references the condition of electronic components. It is targeted at organisations that have their own failure databases, or wish to develop these and describes how to change baseline failure rates to their environmental or operating conditions. Other standards do exist, such as the Siemens SN 29500, which is based on the 61709.
Maintainability: Refers to IEC 60706, based on BS EN 60706 series (BSI: British Standards, Citation1982). This covers requirements in specifications and contracts, programme, test and diagnostic procedures, maintainability design procedures, maintainability verification, and presentation of data related to maintainability.
For RAM testing we summarise the following approaches.
1.5.3.3. Equipment reliability testing: IEC 61123
Guidance on test plans, e.g., truncated sequential test plan and fixed trial/failure terminated test plan for failure ratio/success ratio of the system. The IEC 60605 is detailed below;
Part 2 – general procedure for the design of additional test, and Part 3-1 – test conditions for indoor portable equipment (withdrawn). Neither require further detail.
Part 4 – statistical procedures, e.g., point estimates, confidence intervals, prediction intervals and tolerance, which follow the exponential distribution.
Part 6 – procedures to verify assumptions of a constant failure rate/intensity and discover patterns in failure rate/intensity. These include statistical tests or graphical methods, e.g., reliability plots, total time test (TTT), hazard plots or M (t) plots.
1.5.3.4. Reliability growth:
EN 60104 is the standard for growth programmes. This references EN 61164, where the Duane model and power law methods are presented (BSI:British Standards, Citation2004).
1.5.3.5. Steady state availability:
IEC 61070 provides techniques for availability performance testing of maintained items in steady-state availability/unavailability. This is used for compliance testing for up-state and down-state, under six conditions.
1.5.3.6. RAM data: IEC 60300-2-3:
Guidance on the collection of data from the field, e.g., usage, environment, events, inventory, data sources. It is also called up by 60300-3-1 on statistical reliability methods for supporting analysis. IEC 60319 is withdrawn.
Selecting a suitable technique is a highly project specific process and should be carried out by a team of experts in the field. RAM techniques have various benefits and limitations. For example, the RBD technique results in fewer errors in the construction of the model as it follows the functional block diagram, deals with most systems and is easily adapted for variations. However, it does not provide fault analysis and is primarily directed towards success analysis. The Markov technique, by contrast, is adaptable for complex redundant configurations, complex maintenance policies and degraded modes of operation. However, as the number of components increases there is an exponential growth in the number of states, resulting in labour intensive analysis. EN 60300-3-1 recognises that techniques have different attributes and provides some guidance. This is shown in Appendix E, RAM technique characteristics.
1.5.4. Performance in railways
The lead measure for punctuality was the Public Performance Measure (PPM) (Toossi et al., Citation2017) on the main line railways in Great Britain (GB). It combines punctuality and reliability in a single measure (Bititci & Veiseth, Citation2006). PPM is the percentage of trains arriving at the terminus within a threshold, e.g., 5 mins, calling at all of the planned station stops (Johnson et al., Citation2017). However, new measures of punctuality have been introduced recently, which are (ORR: Office of Rail and Road, Citation2021; Network Rail, Citation2017):
On Time – percentage of recorded station stops called at on time or early. To be on time it has to be less than one minute late measured against the timetable (at each of the stations stops).
Time to ‘x’ – percentage of recorded station stops within ‘x’ minutes of the planned time. E.g. a successful time to ‘3’ would be 2 min and 59 s measured against the timetable (at each of the station stops).
Time to 15 – the percentage of recorded station stops within 15 min of the planned time. A success would be within 15 min measured against the timetable (at each of the stations stops).
Cancellations – measures the number of trains cancelled as a percentage of trains planned.
Reduction in performance impacts both passengers and operators; infrastructure managers can be financially penalised. Therefore, performance is critical in meeting passenger needs and for business survival. To evaluate performance, railway projects in the UK simulate the operation of the railway that affects the PPM. TRAIL (Transport Railway Availability Integrated Logistics) software is commonly used (Best, Citation2004). This allows an investigation of the railway e.g., to match existing performance, to identify areas of poor performance, to model and quantify proposed improvements. Additionally, simulation allows the prediction of future performance related to timetable changes, infrastructure upgrades, rolling stock introduction and operational incentives and maintenance strategies. TRAIL analyses infrastructure and trains on a minute by minute basis 24 h/d 7 d/week, accounting for timetabled journeys, design layouts, equipment performance, operation strategies, and maintenance strategies (Best et al., Citation2012). Inputs and outputs are shown in .
TRAIL uses discrete event simulation, i.e., the modelling of events in time, with an occupancy model (signal to signal), recovery and re-routing of trains. Inputs include:
the frequency of trains, e.g., timetable,
operations, e.g., trespass, vandalism, crew lateness,
train reliability, e.g., each type of train, and systems infrastructure reliability, e.g., signalling, track, communications, and
maintenance, e.g., ad-hoc, possessions.
Sometimes not all the subsystems can be input in detail, e.g., Signalling and therefore the RAM characteristics of blocks/parts of the subsystem are provided. The key output is PPM and delay minutes for different railway configurations. is an example (from CRL).
1.5.5. RAM targets
The TRAIL model requires system RAM targets to be included for accuracy. RAM targets can be provided in several formats. For reliability, it can be Mean Time Between Failure (MTBF), which is the average time that a system or component should run without a failure. Alternatively, a failure rate can be used (Chauhan & Pancholi, Citation2013), both are shown below.
Availability targets can be stated as a percentage, e.g., 99.98% availability. Availability brings in maintainability, e.g., the Mean Time to Restoration (MTTR), which is the time from when the failure occurs to when the system is again ready for service. For inherent availability (Ai), which does not account for preventative maintenance and repair begins immediately after failure, the following is used:
RAM targets for components can be sourced from databases such as the IEEE gold book (IEEE, Citation2007) or by investigating other railways database/failure management systems such as Train Running Under System TOPS (TRUST), where TOPS is Network Rail’s Total Operations Processing System.
Once the simulation has been processed and the performance level, e.g., PPM. is achieved with the proposed RAM targets, these are released into suppliers’ contracts for designing, manufacturing, installing and testing the subsystems. These systems account for roughly 40% of predicted PPM. RAM Engineering studies analyse, predict, measure, monitor and demonstrate (Ebeling, Citation1997) that the new systems meet the RAM targets. To allow RAM to be effective it must also be fully integrated in the development of the systems architecture and its configuration (Wasson, Citation2006). The remaining 60% of predicted PPM is operational and is managed by the performance team including; timetable, crew management, staff, stations, control centre, etc. Robust procedures are developed that aim to support efficient recovery from a fail state, thereby minimising downtime.
1.5.6. Reliability levels and apportionment
The concept of reliability levels is a method to frame reliability hierarchy (see ). The concept was introduced by Network Rail (Best, Citation2004). Railway (Route) level reliability is top and this includes operational elements, rolling stock and fixed infrastructure (systems and subsystems or assets) which affect PPMFootnote6. For example, failure of a subsystem e.g., track circuit, can affect the system e.g., signalling, which affects railway level and thus PPM. Weather, train crew and passengers are operational events which can also impact PPM and indeed are a large contributor (RSSB, Citation2009). The authors added metrics, process, apportionment in to illustrate the concept.
Apportionment, or allocation, is a known RAM technique to define a satisfactory numerical reliability value at the subsystem or system level such that the required overall reliability is reached at the railway level, e.g., PPM. This is common in large systems (O’Connor & Kleyner, Citation2012) where different design teams or contractors are involved.
2. Literature search
2.1. Overview
The process and detail of the literature review searches and results are shown in . This section details the selection of terms for the literature review, filtering and final selections of papers.
2.2. Pilot Searches
Pilot searches were undertaken to test search terms identified from the content in the introduction and from the research questions. Searches were made of frequently used journals selected from the fields of RAM and mega projects. The terms used and Boolean expressions are shown in . Pilot search terms are numbers No1 to No 4. No 1 offered many returns while No 2, No 3, and No 4 returned fewer.
Table 1. Search terms.
2.3. Database searches
Searches were undertaken with four databases in abstract, titles, and keywords in database search 1 (DS1, pilot) and database search 2 (DS2). DS1 kept terms No 1 to No 4 and in DS2 terms were refined in No 5 to No 8. DS1 offered significant returns for searches No 1, No 2. No 3, but fewer for No 4. However, this helped us develop and refine terms for searches No 5 to No 8 in DS2. In No 5 we initially used the term ‘mega project’, and later added ‘major project’ as these terms may be used interchangeably in the literature. The difference between them is shown in . To further examine this we added ‘Railway’, as the area of application of our study, also shown in . However, this returned an even fewer number of results. On this basis, mega project and major project terms had to be discounted. Indeed, this supports the hypothesis of a lack of knowledge in literature, and the need for this paper. However, we ensured relevance to megaprojects when we read the papers in the filtering of papers process detailed in Section 2.4. Searches No. 6 and No. 7 also returned low numbers and were not furthered. However, term No. 8, which is an adaption of No. 4 indicated an appropriate level of returns. It includes the sector i.e., railways and also the term reliability. The term Railway offered 3.7 times more returns than Metro, see . Additionally, we favoured the term Reliability over Availability or Maintainability due to the most returns using terms individually. Collectively they were too many, confirmed by search terms No. 4. Thus, search terms No 8 were continued to the review/filtering stage.
Table 2. Search term reviews.
2.4. Review and filtering
We removed any duplications which reduced papers from 5074 to 3711 (filter 1). We read the title and abstracts of these and reduced the number from 3711 to 251 (filter 2). Then read the full paper to get to 56 (filter 3). The filtering (filter 1 & 2) process needed to include a Railway system and a RAM technique category as shown in . When reading the papers (filter 3) we included items in filter 2 but also identified their relevance and applicability to mega projects and if so they were included. When filtering we excluded structural elements, e.g., concrete, walls, bridges and operations (see below). Exclusions are listed in more detail in . The exclusion criteria were chosen as they do not tend to be included in RAM management or in the BS EN 50126Footnote7 process. We do recognise operational items are a large contributor to PPM loss e.g., train scheduling, passengers, environment, train crew, planning, possessions, adhesion, weather, police, security, trespass, human (RSSB, Citation2009). Operations is a major subject and too vast to be included.
3. Analysis and synthesis
3.1. Meta and content analysis
To identify the state-of-the-art for RQ2, meta data was extracted from papers across a range of ‘categories’ e.g., Railway Systems and ‘subcategories’ e.g., Signalling, as shown in . This emerged as the most logical and systematic representation during the conceptualisation and filtering process and the results are also shown tabulated in Appendix B. Each paper (of the 56) ‘subcategory’ is presented in this section under a ‘category’ heading. The subcategories are numerically added (contribution) to illustrate and determine the state-of-the-art.
3.1.1. Railway systems
Systems that make up the railway e.g., signalling are used as subcategories under railway systems. Where there is no actual system, papers are categorised as either model/framework or RAM management. Contributions are shown in and the following offers an overview of the content.
Leading the way is the signalling system and papers cover content such as interlocking software (Weiqi & Shenghua, Citation2016) and high speed train control (Jiang et al., Citation2019). Chinese railway papers often review their own national signalling system CTCS (Cai et al., Citation2016; Jiang et al., Citation2018; Su & Che, Citation2013a, Citation2013b). Also recognised are the European counterparts e.g., ERTMS/ETCS (Flammini et al., Citation2006).
Model/Framework is the next highest contribution and papers illustrate various novel ideas and concepts, such as an innovative model simulator for railway service quality (Dazzi et al., Citation2007) and calculating average train delay from a probabilistic approach (Cosulich et al., Citation1996). Simulation is used to assess availability with train frequency and maintenance times (Stenström et al., Citation2016), and modelling using a standard reliability approach and views failure effects on operations (MacChi et al., Citation2012). Others provide what is actually practised by railway authorities for modelling and simulation at railway levels (Best, Citation2004; Halliday, Citation2004). Conceptual method framework (Dabla et al., Citation2017) and modelling framework are also presented (Fourie & Zhuwaki, Citation2017).
For rolling stock, traction drives (Fazio et al., Citation2001; Hayashiya et al., Citation2017; Liu et al., Citation2013), and locomotive electrical equipment (Giorgio et al., Citation2006) have been studied. Subsystems on rolling stock are covered for axle bearing (Yonghua et al., Citation2016), air and brake (Ji-liang et al., Citation2011). For fleet/whole rolling stock (Milutinović & Lučanin, Citation2005) and (Rezvanizaniani et al., Citation2009), diesel locomotives fleet (Kazantseva et al., Citation2019) and finally, for the whole electric traction system on a train (Navas et al., Citation2017).
Traction Power Supply is covered (Feng et al., Citation2019; Hayashiya et al., Citation2017; Yang & Beijing, Citation2009; Yilin et al., Citation2019). AC traction power feeding arrangements (Chen et al., Citation2014), and for UK (750 V) DC traction Systems – (Yilin et al., Citation2019), catenary system (Ku & Cha, Citation2011), and high speed lines in Shanghai (Zhen et al., Citation2016) using relay protection.
Track and point machines field data is used to define the maintenance strategy (Ghodrati et al., Citation2016), and to look at failure trends on 5 stations with point machines field data in Indian railways (Panja & Ray, Citation2007). Various point machines are reviewed (Panchenko et al., Citation2019), and (Bemment et al., Citation2018) find human error is a huge factor in track switch failures (Donat et al., Citation2008).
Communications systems for global navigation satellite system (Lu et al., Citation2013), and wireless high speed control suggesting interleaving to improve reliability (Junfeng & Xishi, Citation2001). Proposed SCADA systems are analysed for implementation in Mass Transit Railway Corporation suggesting a fivefold improvement (MTRC) (Hampton et al., Citation1998).
RAM Management in rolling stock is covered highlighting the issues surrounding the BS EN 50126 regarding the lack of guidance on methods (E Calixto, Citation2014b). How RAM management can be integrated into the model of mass rapid transport in China is considered by Ju et al (Citation2011), and the integration of RAMS assurance from a suppliers viewpoint by Vintr and Vintr (Citation2008).
3.1.2. RAM techniques
RAM techniques are shown in which illustrates the collection of techniques applied. Where no technique is recognised it is shown as ‘no technique’. Additionally, we show which railway systems apply the techniques e.g., fault tree/event tree, traction power the most.
We further summarise each of the techniques and where they are applied.
Statistical – Life Data A process to determine the failure pattern is provided for points and point machine (Panja & Ray, Citation2007) failures, concluding that they follow a non-homogeneous process (NHPP) pattern. Weibull distribution (Bemment et al., Citation2018) is the selected distribution analysis for switch component lifetimes. The IEC process is implemented and tested on rolling stock and lift failure data (Navas et al., Citation2017). Weibull, and the lognormal distribution were found to best model rolling stock wheel sets (Rezvanizaniani et al., Citation2009). Pareto analysis is featured and used to represent failures on locomotives (Kazantseva et al., Citation2019).
No technique is where none have been utilised in papers, however, they can still promote methods and techniques to follow on railway projects (Best, Citation2004; Halliday, Citation2004). Additionally framework type papers for processes are provided by MacChi et al., (Citation2012), Fourie & Zhuwaki (Citation2017) in a RAMS environment. The RAMS management process is also illustrated (Vintr & Vintr, Citation2008; Ju et al., Citation2011) for a projet. For other approaches, in power, we see reliability indices used (Yang & Beijing, Citation2009), using a basic formulae, e.g., availability = MTBF/MTBF + MTTR (Hayashiya et al., Citation2017), and with rolling stock (Milutinović & Lučanin, Citation2005). However, no particular techniques are used.
Statistical – Bayesian, Petri Net and Markov can be grouped together. Signalling systems use Bayesian the most. Bayesian is also used for switches and crossings (Baglietto et al., Citation2018) and IGBT power modules (Dabla et al., Citation2017).
Bayesian is used in signalling:
To determine failure rate of components in a railway environment where failure data is lacking (Mokhtarian et al., Citation2013).
To assess the reliability of CTCS signalling systems (Cai et al., Citation2016; Jiang et al., Citation2018; Su & Che, Citation2013a, Citation2013b), and the reliability of track circuits (Xiaomin, Yiliu, & Lei, Citation2016).
For reliability of a signalling system (Baglietto et al., Citation2018), and a study on the reliability for the European Train Control System (ETCS) standard (Flammini et al., Citation2006).
Petri Nets are utilised for reliability assessment on:
Global satellite navigation systems (GNSS) (Lu et al., Citation2013; Nguyen et al., Citation2015), signalling control centres (Yu et al., Citation2013), as part of a reliability assessment in signalling for an overall simulation process (Firpo & Savio, Citation1997).
Markov techniques are utilised for:
Architecture of Automatic Train Protection Systems (ATPS) (Yan & Wang, Citation2000). Data transmission on high speed train (Yan & Wang, Citation2000). Argumentation concept with RAM on rolling stock (Gandibleux et al., Citation2012). Reliability analysis of node in signalling systems (Chandra & Kumar, Citation1997).
In summary, Petri Nets (PN) are considered a solution to model fault trees (Nguyen et al., Citation2015) but Bayesian is preferred to PN for its greater efficiency (Flammini et al., Citation2006). To model complex behaviour different models are needed, such as Markov and PN, but for the large systems they are not compatible due to the state space explosion problem, here, Bayesian is preferred. Bayesian is also suitable to model uncertain knowledge and overcome the explosion issue (Baglietto et al., Citation2018; Bernardi et al., Citation2013; Jiang et al., Citation2019; Mokhtarian et al., Citation2013; Su & Che, Citation2013b, Citation2013a). We can suggest that this is the main reason signalling systems use Bayesian, as they tend to be complex and large.
FMECA and RBD
FMECA is rarely used as a standalone technique but can be useful with other techniques. FMECA brings out failure modes on systems for further assessment. This is evidenced by FMECA being used to:
obtain failure events to input to a Monte Carlo simulation (Feng et al., Citation2019), for the traction power supply of a high speed railway.
provide failures and failure frequency to establish criticality (Panchenko et al., Citation2019), for railway turnouts, e.g., points.
develop each fault mode further to generate a main cause (Ji-liang et al., Citation2011), for rolling stock braking system.
analyse each potential failure mode and their effects to each system component (Yonghua et al., Citation2016), for axle bearing system on rolling stock.
classify failures, and analyse failure severity (Cai et al., Citation2016), for on board signalling equipment.
highlight key issues on a system (Saponara et al., Citation2015), for uninterruptible power systems.
mitigate poor design, material configuration and drive improvement in the RAMS management process (Calixto, Citation2014b)
RBD is used in conjunction with reliability indices for a train traction system (Liu et al., Citation2013), and for simulation (Fazio et al., Citation2001; Cosulich et al., Citation1996). RBD is also used as part of a RAMS management process (Calixto, Citation2014b). It can be used to analyse more complex systems than FTA (E Calixto, Citation2014b) and to present functional relationships and logical relationships between various parts of systems (Liu et al., Citation2013). RBD can model parallel configuration (Dazzi et al., Citation2007) and establish the reliability and availability of multichannel architectures (Bemment et al., Citation2018). RBD is stated to be limited in expressive power but efficient and easy to use (Flammini et al., Citation2006), and RBD (and FT) is used to map analyses onto more advanced methods, such as BN (Jiang et al., Citation2019).
Fault Tree (FT)/Event Tree (ET). We see ET is only used in an assessment of the overhead catenary system (Ku & Cha, Citation2011). However, FT is mainly used in papers about research dealing with reliability assessments. It can calculate the reliability of the system and the importance of components (Su & Che, Citation2013b). FT is used for:
signalling reliability assessment (Flammini et al., Citation2006; Jiang et al., Citation2018; Su & Che, Citation2013b, Citation2013a).
on power & OHLE assessments (Feng et al., Citation2019; Ku & Cha, Citation2011; Yilin et al., Citation2019; Zhen et al., Citation2016).
a tool to map to a Bayesian Network, e.g., FT to BN (Su & Che, Citation2013a).
Variation Simulation Model (VSM). Provided for a traction power system on a high speed network, where FMECA and FTA were used initially and the Monte Carlo simulation method to provide reliability indices (Feng et al., Citation2019). Monte Carlo is also used for a rolling stock traction drive failure simulator (Dazzi et al., Citation2007), before calculating train delays. A SCADA system was analysed by simulating the failed states to understand the availability using the Monte Carlo method (Hampton et al., Citation1998).
Reliability Testing. A reliability growth programme is deployed for a fleet of trains for a demonstration period to meet its RAM targets utilising a goodness of fit and a mixed Poisson approach (Giorgio et al., Citation2006). Duane and least medium squares (LMS) were used to determine the behaviour and failure rate and reliability growth of an aerial power conductor (García-Escudero et al., Citation2005). The Crow-AMSAA model (Ghodrati et al., Citation2016) is used to understand the time to failure of switches and crossing (S&C) subassemblies, e.g., blades, based on historic failures to work out the availability of an S&C.
Software reliability growth models (SRGM) are applied to signalling interlocking software by comparing classic types of NHPP SRGM, e.g., Goel-Okumoto, using criteria for the goodness of fit. Wang proposes a new version to enable developers to improve reliability before it is deployed in operation (Weiqi & Shenghua, Citation2016). Traffic management systems are assessed for reliability evaluation both with qualitative and quantitative approaches. For quantitative purposes NHPP models are proposed, e.g., Jelinski Moranda (D’Addio et al., Citation1997).
3.1.3. RAM standards and reliability level
We reviewed papers to understand if RAM standards, e.g., BS EN 50126, are applied and where they are aimed, e.g., at the railway (route), system or subsystem levels. Where there is a mixture of systems we have indicated this. The contributions of the subcategories are shown in .
The majority of Papers do not mention or refer to RAM(S) standards. The most frequently applied is BS EN 50126-1. From this evidence we can argue that most papers do not apply the standard, and those which have used the standard we summarise below.
Recognised by authors in China for new projects (Yang & Beijing, Citation2009), however, does not provide quantitative standards, only qualitative (Feng et al., Citation2019).
Tasks presented (life cycle stages 4 and 5) focusing on the design and production of systems and equipment and a RAMS programme (Vintr & Vintr, Citation2008).
Used to define availability and the process (Milutinović & Lučanin, Citation2005), before the purchase of assets.
Mapped onto a project life cycle and must be provided in RAM management (Ju et al., Citation2011).
Describes a process but lacks tools for reliability engineer to implement in each phase (Calixto, Citation2014b).
Offers guidelines for specifications but lacks details for the operation and performance part of the life cycle (Stenström et al., Citation2016).
Reliability predictions need to be carried out in the development of signalling systems to demonstrate that the requirements are met (Renpenning, Citation2004).
Required under BS EN 50126-1, signalling needs to be reviewed in terms of its availability (Iwata et al., Citation2009).
From a different viewpoint we include a horizontal view of the different Reliability levels in . Additionally, we show associated contributions of subcategories. TP2, TP19 etc are the technical papers in the study. This illustrates the concept shown in , in more detail.
From we recognise model/framework papers are dominant as traditional RAM techniques generally do not simulate the railway e.g., with timetable and operations at the railway level. Whereas dropping to the system level, in , a significant increase in the number of papers occurs. Signalling appears most in terms of railway systems. Finally, it can also be noted that the sub category ‘No RAM standard’ is dominant within all of the Reliability levels. From this we can argue that the RAM standards are not frequently applied.
4. DRR and assessments
The Derived RAM requirements (DRR) concept is a suite of requirements (DRR 1-18) developed by the authors to analyse the meta and content analysis. It is based on BS EN 50126-1 lifecycle, objectives, activities and deliverables. The full set of DRRs are shown in Appendix B. After developing the DRRs we illustrate how they are used as part of the next stage of the study in . It also shows where each part is detailed e.g., DRR concept, Appendix A, initial assessment Appendix B.
The initial assessment firstly categorised each paper number, e.g., TP1, the author, system/subsystem/or type, e.g., Signalling. We assessed each paper for the lifecycle phase according to BS EN 50126-1 where it could be implemented into a railway project and its RAM technique. Each paper is given a DRR requirement e.g., DRR1, DRR2 or N/A where no DRR is recognised. We also validated by ‘Y’ or ‘N’ and why, e.g., proven in the field or recognised by railway authorities. shows the DRR coverage which is low with 5 of 18 (28%) DRRs covered. We also realise DRRs have a disproportionate contribution coverage per DRR, e.g., DRR10 (RAM analysis) shows a high contribution where others are low, e.g., DRR16 (RAM validation).
As results showed a large gap in coverage we needed an approach to focus on DRRs rated by importance. To do this we identified that a survey could be used as the mechanism to reveal the critical DRRs and named it the DRR Importance Survey.
The DRR Importance Survey is conducted with RAM experts working for national railways and on MRPs in the UK, e.g., High Speed 2, Crossrail, and internationally. The survey is individually evaluated with a scoring regime from 1 to 5 with a weighted average from the assessment of each DRR; importance to project 40%, technical difficulty 30%, influence on system reliability 20% and effort required 10%.
The measurement is called the grading factor (GF). The higher the GF, the more important the DRR. Each weighted average, e.g., the GFs from the individual surveys were averaged to obtain a final GF level. We stated that a target of = >4, (GF Target) must be reached if the DRR is to be considered. Results show DRR7,10,11,15,16 &18 meet the target in . Full details are shown in Appendix C.
The final assessment uses the validated papers, GF and gaps, with results in . The validated papers determined the gap which is where the contribution of DRR is = <3 (gap threshold). Anything above this is declared no gap. For example, if we look at RAM analysis (DRR10) the contribution is 11 and GF of 4.2. However as there is no gap in the literature, (11 > 3), we do not consider this as topic for consideration. However, if there is a gap and the GF target is reached, they are considered. Based on these principles we can declare DRR7, DRR11, DRR15 and DRR18 (shown as grey, yellow and green in ) are considered further ref. .
5. Results and discussion
We found the conceptualisation and definition process extremely useful for generating knowledge, search terms and identifying topics. We also learned that there is an abundance of mega project papers that cover valuable current topics such as systems integration, innovation, organisation, governance and management. However, we found that they do not cover RAM in railways satisfactorily.
From our viewpoint, we frame the state-of-the-art into three main themes which are based on the railway system, RAM technique and reliability level. Under each of the themes we focus the subthemes on: contribution, content (detailed in section 3), and the other two remaining categories. An example for railway systems is signalling, therefore we select these papers only and discuss the subthemes surrounding signalling. The results are shown in and we also add in the number of associated papers, their associated DRR numbers and if validated. The following paragraphs show more of the details.
Table 3. State-of-the-art summary.
5.1. Railway systems
Signalling is the most widely used system in RAM application. Platform Screen Doors (PSD) and station type systems are absent. Signalling favours the Bayesian technique. Reliability level indicates this is at system level.
5.2. RAM technique
The most significant input is through statistical life data. Contributions from the Rolling stock subcategory show that it is difficult to obtain failure data in the railway industry. Lack of failure data is a common problem, since its collection is labour intensive and hard to manage (Stone, Citation2005). Failure data in railways can be considered an area for further research. Reliability level contributions indicate a similar amount of coverage as system and subsystem level papers. ‘No technique’ papers contribution is also high and in modelling/framework which indicates not a settled category. Indeed, industry shows TRAIL is used on projects e.g., CRL, HS2, and the West Coast Main Line. However, other railway projects such as the Trans Pennine upgrade (TRU) are willing to find pursue other types of solutions in this areaFootnote8.
5.3. Reliability level
The Reliability level is at the systems level, and signalling and Bayesian are the most used system and technique, respectively, as already discussed.
Whilst we have not discussed RAM standards in much detail, we confirm that BS EN 50126-1 is not commonly applied. This is surprising as this is the standard to use in railways whilst undertaking RAM.
The DRR concept was shown to be very effective. It enabled papers to be analysed systematically within RAM area narrowing our focus. From initial assessment we uncovered considerable gaps. The expert survey on the DRR enabled us to focus in even more on the important areas in the application of RAM in railways, and was a particularly successful part of the study. We consolidated the results using the GF, validated DRR, and gap threshold to produce the topics. These are the critical areas of RAM in railways which is the main focus of the study, and can be used in future research development studies. These along with state-of-the-art are shown in .
6. Conclusions and future research
We conclude that gaps in RAM knowledge for railways, when applying the railway RAM standard, have been uncovered. We have further exposed the most critical gaps. Therefore, we recommend research is conducted in the following areas of RAM application on MRPs, to close these gaps:
Apportionment and allocation
Data collection system
Integrated demonstration
RAM case
Furthermore, we also recommend from our findings that further research be provided on the following:
State-of-the-art of performance modelling of the railway at railway level, and its interface with RAM
Investigation into failure data in Railways
Investigation of BS EN 50126-1
The larger than typical introduction defining and conceptualising RAM allows the reader to grasp its wider concept and context more thoroughly. The work to implement the SLR process of this study was time consuming; on completion the authors determined that a standard ‘off the shelf process’ may have been more efficient. Researchers can use the output of the study identifying gaps as topics to develop their own research; indeed, this was one of the aims of the paper. RAM practitioners can use this paper as an index to papers covering the various examples of the railway systems and their RAM techniques, and to guide their approach to RAM analysis.
Supplemental Material
Download MS Word (35 KB)Supplemental Material
Download MS Word (32.5 KB)Supplemental Material
Download MS Word (33.7 KB)Supplemental Material
Download MS Word (39.2 KB)Supplemental Material
Download MS Word (79.4 KB)Supplemental Material
Download MS Word (36.3 KB)Disclosure statement
No potential conflict of interest was reported by the author.
Correction Statement
This article has been corrected with minor changes. These changes do not impact the academic content of the article.
Additional information
Notes on contributors
Jonathan King
Jonathan King graduated from University of Wales Swansea with a BEng Hons degree in Electrical and Electronic Engineering. He also holds an MSc in Railway Systems Engineering from UOB, where he is currently a PhD candidate. He has vast experience working in industry mainly within the Mega Railway Projects sector.
Gemma Nicholson
Gemma Nicholson received the MMath and MSc degrees in mathematics from the University of Bath, UK, and the PhD degree with a focus on research into the design of microwave bandpass filters using optimization algorithms from the University of Birmingham, UK.
She is currently a Research Fellow with the Birmingham Centre for Railway Research and Education (BCRRE), University of Birmingham, UK. Her research interests lie in using modelling, simulation, analytical and strategic approaches to applied railway planning and operations questions.
Felix Schmid
Felix Schmid graduated from the Swiss Federal Institute of Technology with the Diploma in electrical and electronic engineering. He worked as a railway traction engineer for GEC in Manchester, for UMIST, for the University of Salford and Brunel University, where he obtained his doctoral degree. He joined the University of Sheffield in 1994 and became Professor of Railway Systems Engineering at The University of Birmingham, where he taught a range of railway engineering subjects and conducted research into railway capacity and operations. He became emeritus in June 2023 but continues to teach and research.
Notes
1 The BS EN 50126 part 1 – the railway RAM process standard – all references to the standard will be part 1, excepting the SLR process.
2 We recognise the terms major and mega projects maybe used interchangeably. We use mega projects in this paper.
3 A System of Systems (SoS) is formed of a set of independent systems that, together, deliver greater functionality than the individual systems. This is through communication and collaboration that include multiple organizations and disciplines engaged in planning, designing, implementing, constructing, delivering, operating and managing (Shimohara, Citation2019).
4 MRPs in GB include - High Speed 1, High Speed 2, 4LM, Northern Line extension, Thames Link, Crossrail (Author).
5 The RAM related tasks are part of the Railway RAM Process which is taken from BS EN 50126-1.
6 We note that not all operations and systems or subsystems impact PPM. However, if deemed critical they are included in RAM analysis e.g., fire protection systems, but not included in the hierarchy to the top railway level (route level).
7 RAM studies in MRPs are mainly undertaken in railway systems such as rolling stock, signalling, power, communications (King & Gugala, Citation2018).
8 TRU – meeting in London (2022) with TRU performance team, who presented their concepts and approaches for modelling railway systems to determine the railway level performance for their intended upgrade.
References
- Davies, A., Gann, D., & Douglas, T. (2009). Innovation in megaprojects: Systems integration at London Heathrow terminal 5. California Management Review, 51(2), 101–125. https://doi.org/10.2307/41166482
- Baglietto, E., Consilvio, A., & Febbraro, A. D. (2018). A Bayesian Network approach for the reliability analysis of complex railway systems. 2018 International Conference on Intelligent Rail Transportation (ICIRT) (pp. 1–6). https://doi.org/10.1109/ICIRT.2018.8641655
- Baker, M. J. (2000). Writing a literature review. The Marketing Review, 1(2), 219–247. https://doi.org/10.1362/1469347002529189
- Bemment, S. D., Goodall, R. M., Dixon, R., & Ward, C. P. (2018). Improving the reliability and availability of railway track switching by analysing historical failure data and introducing functionally redundant subsystems. Proceedings of the Institution of Mechanical Engineers, 232(5), 1407–1424. https://doi.org/10.1177/0954409717727879
- Bernardi, S., Flammini, F., Marrone, S., Mazzocca, N., Merseguer, J., Nardone, R., & Vittorini, V. (2013). Enabling the usage of UML in the verification of railway systems: The DAM-rail approach. Reliability Engineering & System Safety, 120, 112–126. https://doi.org/10.1016/j.ress.2013.06.032
- Best, N. (2004). Metrics and models RAM metrics and models: context translating outputs into requirements modelling through the lifecycle lessons learned. The IEE Seminar on Railway System Modelling – Not Just for Fun (pp. 31–47). IEEE.
- Best, N., Hyland, B., & Waters, S. (2012). UK railway system reliability - modelling the future - a case study. 22nd Annual International Symposium of the International Council on Systems Engineering, INCOSE 2012 and the 8th Biennial European Systems Engineering Conference 2012, EuSEC (pp. 945–962). https://doi.org/10.1002/j.2334-5837.2012.tb01376.x
- Birolini, A. (2013). Reliability engineering – theory and practice (7th ed). Springer Heidelberg.
- British Standards. (1999). PD R009-003: 1999 Guide to the Specification of a guided transport system.
- British Standards. (2004). BS EN 61164:2004 Reliability Growth—Statistical test and estimation methods (Vol. 3).
- BSI: British Standards. (1982). IEC 60706 - Part 1 Maintainability of equipment. Part 1 Guide to specifying and contracting for maintainability (Vol. 3).
- Cai, B., Zhang, F., Shangguan, W., Wang, J., & Chen, L. (2016). Performance degradation based reliability prediction method for CTCS on-board equipment. 2016 IEEE International Conference on Intelligent Rail Transportation (pp. 80–88). IEEE. https://doi.org/10.1109/ICIRT.2016.7588714
- Calixto, E. (2014a). Integrated RAMS analysis methodology: The railway case study. European Safety and Reliability Conference, ESREL, 2013 (pp. 1281–1290). https://doi.org/10.1016/j.accinf.2010.07.019
- Calixto, E. (2014b). RAMS analysis: How reliability engineer and risk analysis tools can be applied to improve asset management on train life cycle. In A.C.W.M. Vrouwenvelder, P.H.A.J.M. van Gelder, R.D.J.M. Steenbergen, S. Miraglia (Eds.), Safety, reliability and risk analysis: Beyond the horizon (pp. 1773–1780). CRC Press.
- Calixto, E. (2013). Gas and oil reliability engineering. Elsevier.
- Calixto, E. (2016). Gas and oil reliability engineering: Modeling and analysis. Elsevier.
- CEN ISO/TR 12489:2016. (2016). Petroleum, petrochemical and natural gas industries—Reliability modelling and calculation of safety systems (pp. 257). BSI Standards Publication. https://doi.org/10.3403/3026A0176u
- Cenelec, E. C. (2017). BS EN 50126-5 BSI Standards Publication Railway Applications: The Specification and Demonstration of Reliability, Availability, Maintainability and Safety (RAMS) National foreword (Part 1).
- Chandra, V., & Kumar, K. V. (1997). Reliability and safety analysis of fault tolerant and fail safe node for use in a railway signalling system (pp. 57). Elsevier Science Limited.
- Chapman, R. J. (2016). A framework for examining the dimensions and characteristics of complexity inherent within rail megaprojects. International Journal of Project Management, 34(6), 937–956. https://doi.org/10.1016/j.ijproman.2016.05.001
- Chauhan, N. D., & Pancholi, P. N. H. (2013). Guidelines to understanding to estimate MTBF. International Journal of Scientific Research & Development, 1(3), 493–495.
- Chen, S. k., Ho, T. k., Mao, B. h., & Bai, Y. (2014). A bi-objective maintenance scheduling for power feeding substations in electrified railways. Transportation Research Part C, 44(2014), 350–362. https://doi.org/10.1016/j.trc.2014.04.016
- Cosulich, G., Firpo, P., & Savio, S. (1996). Power electronics reliability impact on service dependability for railway systems: A real case study (pp. 996–1001). IEEE.
- D’Addio, G. F., Fracchia, M., & Imovilli, A. (1997). Reliability assessment in transport integrated operation systems. IFAC Proceedings Volumes, 30(8), 949–954.(17)43943-7 https://doi.org/10.1016/s1474-6670
- Dabla, E. A., Andrianoelison, F., Martin, C., Fournier, C., Peres, F., & Piton, M. (2017). Methodological framework for implementation of a prediction reliability model of IGBT power modules used in railway applications. 2017 4th International Conference on Control, Decision and Information Technologies, CoDIT 2017 (pp. 639–644). https://doi.org/10.1109/CoDIT.2017.8102666
- Davies, A., Dodgson, M., Gann, D. M., & MacAulay, S. C. (2017). Five rules for managing large, complex projects. MIT Sloan Management Review, 59(1), 72–78.
- Davies, A., MacAulay, S. C., & Brady, T. (2019). Delivery model innovation: insights from infrastructure projects. Project Management Journal, 50(2), 119–127. https://doi.org/10.1177/8756972819831145
- Davies, A., Macaulay, S., Debarro, T., & Thurston, M. (2014). Making innovation happen in a megaproject: London’s Crossrail suburban railway system. Project Management Journal, 45(6), 25–37. https://doi.org/10.1002/pmj.21461
- Dazzi, G., Savio, S., Firpo, P., & Carlo, M. (2007). Estimate of components reliability and maintenance strategies impact on trains delay. Proceedings 21st European Conference on Modelling and Simulation. https://doi.org/10.7148/2007-0447[]
- Denicol, J., & Davies, A. (2022). The megaproject-based firm: Building programme management capability to deliver megaprojects. International Journal of Project Management, 40(5), 505–516. https://doi.org/10.1016/j.ijproman.2022.06.002
- Denicol, J., Davies, A., & Krystallis, I. (2020). What are the causes and cures of poor megaproject performance? A systematic literature review and research agenda. Project Management Journal, 51(3), 328–345. https://doi.org/10.1177/8756972819896113
- Denicol, J., Davies, A., & Pryke, S. (2021). The organisational architecture of megaprojects. International Journal of Project Management, 39(4), 339–350. https://doi.org/10.1016/j.ijproman.2021.02.002
- Denniss, S. (2017). A case study: the benefits and challenges of root cause analysis presented through a real world example from the rail industry. Safety and Reliability, 37(4), 197–216. https://doi.org/10.1080/09617353.2018.1471189
- Department of Defense. (2005). DOD guide for achieving reliability, availability and maintainabilty. Department of Defense.
- Dhillon, B. S. (2006). Maintainability, maintenance, and reliability for engineers. CRC Press.
- Donat, R., Bouillaut, L., Aknin, P., & Leray, P. (2008). Reliability analysis using graphical duration models. ARES 2008 – 3rd International Conference on Availability, Security, and Reliability (pp. 795–800). https://doi.org/10.1109/ARES.2008.25
- Ebeling, C. E. (1997). Introduction to reliability & maintainability engineering. McGraw-Hill.
- Enrico Zio, Q. M. (2018). Handbook of RAMS in railway systems – theory and practice. Taylor & Francis.
- Fazio, V., Firpo, P., & Savio, S. (2001). Effect of MTTF and MTTR statistical uncertainty on mission dependability for power electronics equipped systems. IEEE International Symposium on Industrial Electronics (pp. 1339–1344). IEEE.
- Feng, D., Lin, S., Yang, Q., Lin, X., He, Z., & Li, W. (2019). Reliability evaluation for traction power supply system of high-speed railway considering relay protection. Proceedings of the IEEE International Conference on Software Engineering and Service Sciences, ICSESS (pp. 441–444). IEEE. https://doi.org/10.1109/ICSESS.2018.8663764
- Firpo, P., & Savio, S. (1997). A new approach for the evaluation of train delay as a function of system components reliability in railway applications. IFAC Proceedings Volumes, 30(8), 835–842.(17)43925-5 https://doi.org/10.1016/s1474-6670
- Flammini, F., Marrone, S., Mazzocca, N., & Vittorini, V. (2006). Modeling system reliability aspects of ERTMS/ETCS by fault trees and Bayesian networks. Proceedings of the European Safety and Reliability Conference 2006, ESREL 2006 – Safety and Reliability for Managing Risk, 3, March 2016 (pp. 2675–2683).
- Flyvbjerg, B. (2017). Introduction: The iron law of megaproject management. University of Oxford.
- Forsberg, K., & Mooz, H. (1991). The Relationship of System Engineering to the Project Cycle. INCOSE International Symposium, 1(1), 57–65.
- Fourie, C. J., & Zhuwaki, N. T. (2017). A modelling framework for railway infrastructure reliability analysis. South African Journal of Industrial Engineering, 28(4), 150–160. https://doi.org/10.7166/28-4-1763
- Gandibleux, J., Cauffriez, L., & Branger, G. (2012). Improving the reliability/availability of a complex system by an active monitoring based onto “augmentation concept”: Application onto a railway system. Advances in Safety, Reliability and Risk Management - Proceedings of the European Safety and Reliability Conference, ESREL 2011, (April) (pp. 2706–2713).
- García-Escudero, L. A., Fernández, M. A., Duque, O., & Zorita, A. (2005). Assessing trends in Duane plots using robust fits. Reliability Engineering & System Safety, 90(1), 106–113. https://doi.org/10.1016/j.ress.2005.03.013
- Garmabaki, A. H. S., Ahmadi, A., Mahmood, Y. A., & Barabadi, A. (2016). Reliability modelling of multiple repairable units. Quality and Reliability Engineering International, 32(7), 2329–2343. https://doi.org/10.1002/qre.1938
- Ghodrati, B., Famurewa, S., & Hoseinie, S. H. (2016). Railway switches and crossings reliability analysis. IEEE International Conference on Industrial Engineering and Engineering Management, 2016-December (pp. 1412–1416). https://doi.org/10.1109/IEEM.2016.7798110
- Gholz, E., James, A. D., & Speller, T. H. (2018). The second face of systems integration: An empirical analysis of supply chains to complex product systems. Research Policy, 47(8), 1478–1494. https://doi.org/10.1016/j.respol.2018.05.001
- Giorgio, M., Guida, M., & Pulcini, G. (2006). Reliability-growth analysis of locomotive electrical equipment. Journal of Quality Technology, 38(1), 14–30. https://doi.org/10.1080/00224065.2006.11918581
- Halliday, B. (2004). Performance by design. IEEE Conference Publication. https://doi.org/10.1145/3322276.3322372
- Hampton, I. M., Bruce, A. G., & Chang, C. S. (1998). Reliability analysis of MTRC’S proposed power SCADA system. Quality and Reliability Engineering International, 14(3), 123–127. https://doi.org/10.1002/(sici)1099-1638(199805/06)14:3<123::aid-qre173>3.0.co;2-1
- Haque, M. A., & Ahmad, N. (2021). An effective software reliability growth model. Safety and Reliability, 40(4), 209–220. https://doi.org/10.1080/09617353.2021.1921547
- Hayashiya, H., Masuda, M., Noda, Y., Suzuki, K., & Suzuki, T. (2017). Reliability analysis of DC traction power supply system for electric railway. 2017 19th European Conference on Power Electronics and Applications, EPE 2017 ECCE Europe, 2017-January (pp. 1–6). https://doi.org/10.23919/EPE17ECCEEurope.2017.8098953
- Hobday, M., Davies, A., & Prencipe, A. (2005). Systems integration: A core capability of the modern corporation. Industrial and Corporate Change, 14(6), 1109–1143. https://doi.org/10.1093/icc/dth080
- Hoehne, O. (2016). Rail systems viewed from a system of systems perspective. Insight, 19(3), 36–38. https://doi.org/10.1002/inst.12105
- Hwang, J., & Jo, H. (2008). RAMS management and assessment of railway signaling system through RAM and safety activities. 2008 International Conference on Control, Automation and Systems (pp. 892–895). IEEE.
- IEEE. (2007). Design of reliable industrial and commercial power systems. IEEE Std 493–2007. IEEE.
- INCOSE. (2014). Systems engineering in transportation projects: A library of case studies (pp. 15). INCOSE.
- INCOSE. (2015). Systems Engineering Handbook (4th ed). INCOSE.
- Institute For Government. (2020). Major Projects. https://www.instituteforgovernment.org.uk/publication/whitehall-monitor-2020/major-projects
- Iwata, K., Hiraguri, S., & Watanabe, I. (2009). Evaluation methods for railway signalling systems from the viewpoint of availability. Quarterly Report of RTRI, 50(3), 152–157. https://doi.org/10.2219/rtriqr.50.152
- Ji-Liang, T., Rong-Xing, D., & Xing-Yuan, Z. (2011). Assessment model for the reliability of vehicle non-service brake system based on fault-tree analysis and meta-synthesis weight (pp. 138–143). IEEE.
- Jiang, L., Liu, Y., Wang, X., & Lundteigen, M. A. (2019). Operation-oriented reliability and availability evaluation for onboard high-speed train control system with dynamic Bayesian network. Proceedings of the Institution of Mechanical Engineers, 233(3), 455–469. https://doi.org/10.1177/1748006X18800630
- Jiang, L., Wang, X., & Liu, Y. (2018). Reliability evaluation of the Chinese Train Control System Level 3 using a fuzzy approach. Proceedings of the Institution of Mechanical Engineers, 232(9), 2244–2259. https://doi.org/10.1177/0954409718769193
- Johnson, D., Kenney, A., Rowe, A., Angus, M., Thomas, M., Drea, J., & Miller, A. (2017). Definitions of railway performance metrics definitions of railway performance metrics issue record. Network Rail.
- Ju, H., Xiang, W., Lu, Y., & Du, X. (2011). Integrating RAMS approach on the safety life cycle of rail transit. ICQR2MSE 2011 – Proceedings of 2011 International Conference on Quality, Reliability, Risk, Maintenance, and Safety Engineering (pp. 801–803). https://doi.org/10.1109/ICQR2MSE.2011.5976732
- Junfeng, W., & Xishi, W. (2001). Analysis about the reliability of wireless data transmission for high speed train in strong interference environment. IEEE Vehicular Technology Conference, 1(54ND) (pp. 168–172). IEEE. https://doi.org/10.1109/VTC.2001.956578
- Kazantseva, N. K., Kharlamov, E. P., Tkachuk, G. A., & Kazantseva, T. V. (2019). Assessment of the reliability of the locomotive based on statistical methods of quality management. IOP Conference Series: Materials Science and Engineering, 666(1), 012054. https://doi.org/10.1088/1757-899X/666/1/012054
- Kececioglu, D. (2002). Reliability engineering handbook (Vol. 1). DEstech Publications. http://www.jstor.org/stable/1270054?origin=crossref
- King, J., & Gugala, O. (2018). Effective reliability, availability, maintainability (RAM) and safety (S), principles and guidelines for large subsurface metro and main line projects. IET Conference Publications, 2018 (CP742) (pp. 1–8). https://doi.org/10.1049/cp.2018.0070
- Kitchenham, B. (2007). Guidelines for performing systematic literature reviews in software engineering. Keele University and University of Durham
- Kraus, S., Breier, M. y., & Dasí-Rodríguez, S. (2020). The art of crafting a systematic literature review in entrepreneurship research. International Entrepreneurship and Management Journal, 16(3), 1023–1042. https://doi.org/10.1007/s11365-020-00635-4
- Ku, B. H., & Cha, J. M. (2011). Reliability assessment of catenary of electric railway by using FTA and ETA analysis. EEEIC.EU 2011 – Conference Proceedings 2011, 10th International Conference on Environment and Electrical Engineering, (pp. 2–5). IEEE. https://doi.org/10.1109/EEEIC.2011.5874633
- Kuan, C. S., Kin, H. T., & Hua, M. B. (2007). Component reliability estimations without field data. HKIE Transactions Hong Kong Institution of Engineers, 14(3), 10–17. https://doi.org/10.1080/1023697X.2007.10668080
- Kwansup Lee, K. L., Jaechan Lee, J. L., & Ilhwan Kim, I. K. (2017). A study on strategy of condition based maintenance for Korea metro rolling stocks. 7th IET Conference on Railway Condition Monitoring 2016 (RCM 2016). IET. https://doi.org/10.1049/cp.2016.1192
- Lee, H. J., Seo, Y. J., Yang, H. K., Koh, D. J., & Kim, D. S. (2007). Basic study on RAMS applications to the urban maglev systems. Proceeding of International Conference on Electrical Machines and Systems, ICEMS 2007 (pp. 1985–1988). IEEE. https://doi.org/10.1109/ICEMS.2007.4412053
- Liu, J., Li, S., Jiang, Y., & Krishnamurthy, M. (2013). Reliability evaluating for traction drive system of high-speed electrical multiple units. 2013 IEEE Transportation Electrification Conference and Expo: Components, Systems, and Power Electronics – From Technology to Business and Public Policy, ITEC 2013 (pp. 10–15). IEEE. https://doi.org/10.1109/ITEC.2013.6574491
- Locatelli, G., Mancini, M., & Romano, E. (2014). Systems engineering to improve the governance in complex project environments. International Journal of Project Management, 32(8), 1395–1410. https://doi.org/10.1016/j.ijproman.2013.10.007
- Lu, D., Grasso Toro, F., & Schnieder, E. (2013). RAMS evaluation of GNSS for railway localisation. IEEE ICIRT 2013 – Proceedings: IEEE International Conference on Intelligent Rail Transportation (pp. 209–214). IEEE. https://doi.org/10.1109/ICIRT.2013.6696295
- Mabelo, P. B., & Sunjka, B. P. (2017). Application of systems engineering concepts to enhance project lifecycle methodologies. South African Journal of Industrial Engineering, 28(3), 40–55. https://doi.org/10.7166/28-3-1838
- MacChi, M., Garetti, M., Centrone, D., Fumagalli, L., & Piero Pavirani, G. (2012). Maintenance management of railway infrastructures based on reliability analysis. Reliability Engineering & System Safety, 104, 71–83. https://doi.org/10.1016/j.ress.2012.03.017
- Bititci, U. & Veiseth B. (2006). Performance measurement in railway operations – improvement of punctualiy and reliability. Proceedings of the PMA Conference (pp. 1–10).
- Milutinović, D., & Lučanin, V. (2005). Relation between reliability and availability of railway vehicles. FME Transactions, 33(3), 135–139.
- Mokhtarian, P., Namzi-Rad, M. R., Ho, T. K., & Suesse, T. (2013). Bayesian nonparametric reliability analysis for a railway system at component level. IEEE ICIRT 2013 – Proceedings: IEEE International Conference on Intelligent Rail Transportation (pp. 197–202). IEEE. https://doi.org/10.1109/ICIRT.2013.6696293
- Muruganandan, K., Davies, A., Denicol, J., & Whyte, J. (2022). The dynamics of systems integration: Balancing stability and change on London’s Crossrail project. International Journal of Project Management, 40(6), 608–623. https://doi.org/10.1016/j.ijproman.2022.03.007
- NASA. (2006). NASA preferred reliability and maintainability practices. http://www.hq.nasa.gov/office/codeq/rm/prefprac.htm
- Navas, M. A., Sancho, C., & Carpio, J. (2017). Reliability analysis in railway repairable systems. International Journal of Quality & Reliability Management, 34(8), 1373–1398. https://doi.org/10.1108/IJQRM-06-2016-0087
- Network Rail. (2017). Definitions of railway performance metrics. Network Rail
- Nguyen, T. P. K., Beugin, J., & Marais, J. (2015). Method for evaluating an extended Fault Tree to analyse the dependability of complex systems: Application to a satellite-based railway system. Reliability Engineering & System Safety, 133, 300–313. https://doi.org/10.1016/j.ress.2014.09.019
- O’Connor, P. D., & Kleyner, A. (2012). Practical reliability engineering (5th ed). John Wiley and Sons.
- ORR: Office of Rail and Road. (2021). Passenger Rail Performance: Quality and Methodology Report. In Passenger Rail Performance: Quality and Methodology Report. September 2021.
- Panchenko, S., Siroklyn, I., Lapko, A., Kameniev, A., & Buss, D. (2019). Critical failures of turnouts: Expert approach. Procedia Computer Science, 149, 422–429. https://doi.org/10.1016/j.procs.2019.01.157
- Panja, S. C., & Ray, P. K. (2007). Reliability analysis of a “point-and-point machine” of the Indian railway signaling system. Quality and Reliability Engineering International, 23(7), 833–848. https://doi.org/10.1002/qre.851
- Prencipe, A. (2003). Corporate strategy and systems integration capabilities: Managing networks in complex systems industries. In A. Prencipe, A. Davies, M. Hobday (Eds). The business of systems integration (pp. 114–132). Oxford University Press.
- Puntis, R., & Walley, D. M. (2007). The use of reliability techniques on traction and rolling stock. Proceedings of the Institution of Mechanical Engineers, 200(4), 295–304. https://doi.org/10.1243/PIME_PROC_1986_200_194_02
- Quanterion Solutions. (2015). System reliability toolkit V: New approaches and practical applications. Quanterion Solutions Incorporated.
- Rajabalinejad, M., van Dongen, L., & Ramtahalsing, M. (2020). Systems integration theory and fundamentals. Safety and Reliability, 39(1), 83–113. https://doi.org/10.1080/09617353.2020.1712918
- Renpenning, F. (2004). Relialbity prediction in railway signalling. 7th International Conference on Probabilistic Safety Assessment and Management/European Safety and Reliability Conference.
- Rezvanizaniani, S. M., Barabady, J., Valibeigloo, M., Asghari, M., & Kumar, U. (2009). Reliability analysis of the rolling stock industry: A case study. International Journal of Performability Engineering, 5(2), 167–175.
- Rowley, J., & Slack, F. (2004). Conducting a literature review. Management Research News, 27(6), 31–39. https://doi.org/10.1108/01409170410784185
- RSSB. (2009). Strategy support mapping current remote condition monitoring activities to the system reliability framework. Rail Safety and Standards Board Ltd.
- Saponara, S., Fanucci, L., & Falciani, A. (2015). Uninterruptible power supply systems for railway with predictive diagnostic against power transformer failure. 2015 IEEE 15th International Conference on Environment and Electrical Engineering, EEEIC 2015 – Conference Proceedings (pp. 2119–2123). IEEE. https://doi.org/10.1109/EEEIC.2015.7165505
- Shimohara, K. (2019). Boundary and relationality in systems design: Toward designing system of systems. CSDE 2019 IEEE Asia-Pacific Conference on Computer Science and Data Engineering. IEEE. https://doi.org/10.1109/CSDE48274.2019.9162423
- Smith, D. (2017). Reliability, maintainability and risk (9th ed). Butterworth-Heinemann. https://doi.org/10.1016/B978-0-7506-6694-7.X5000-5
- Stenström, C., Parida, A., & Kumar, U. (2016). Measuring and monitoring operational availability of rail infrastructure. Proceedings of the Institution of Mechanical Engineers F, 230(5), 1457–1468. https://doi.org/10.1177/0954409715592189
- Stone, B. (2005). Reliability data collection and analysis. Safety and Reliability, 25(1), 58–69. https://doi.org/10.1080/09617353.2005.11690798
- Su, H., & Che, Y. (2013a). Reliability assessment of CTCS-3 using Bayesian networks. QR2MSE 2013 - Proceedings of 2013 International Conference on Quality, Reliability, Risk, Maintenance, and Safety Engineering (pp. 284–288). https://doi.org/10.1109/QR2MSE.2013.6625585
- Su, H., & Che, Y. (2013b). Reliability assessment on CTCS-3 train control system using faults trees and bayesian networks. International Journal of Control and Automation, 6(4), 271–292.
- Toossi, A., Barson, L., Hyland, B., Fung, W., & Best, N. (2017). Infrastructure/train borne measurements in support of UK railway system performance—gaining insight through systematic analysis and modelling. In A. Shaw, L. Redding, & R. Roy (Eds.). Advances in through-life engineering services. Springer. https://doi.org/10.1007/978-3-319-49938-3
- Vintr, Z., & Vintr, M. (2008). Safety management for electromechanical systems of railway vehicles. Proceedings – Annual Reliability and Maintainability Symposium (pp. 155–160). https://doi.org/10.1109/RAMS.2008.4925787
- Vom Brocke, J., Simons, A., Niehaves, B., Riemer, K., Plattfaut, R., & Cleven, A. (2009). Reconstructing the giant: On the importance of rigour in documenting the literature search process [Paper presentation]. 17th European Conference on Information Systems, ECIS 2009.
- Wasson, C. S. (2006). System analysis, design, and development concepts, principles, and practices. A John Wiley & Sons.
- Weiqi, W., & Shenghua, D. (2016). Reliability evaluation of interlocking software based on improved NHPP model. 2016 IEEE International Conference on Intelligent Rail Transportation (ICIRT) (pp. 447–452). IEEE. https://doi.org/10.1109/ICIRT.2016.7588767
- Whyte, J., & Davies, A. (2021). Reframing systems integration: A process perspective on projects. Project Management Journal, 52(3), 237–249. https://doi.org/10.1177/8756972821992246
- Whyte, J., Davies, A., & Sexton, C. (2022). Systems integration in infrastructure projects: seven lessons from Crossrail. Proceedings of Institution of Civil Engineers, 175(3), 103–109. https://doi.org/10.1680/jmapl.21.00014
- Worsnop, T., Miraglia, S., & Davies, A. (2016). Balancing open and closed innovation in megaprojects: Insights from Crossrail. Project Management Journal, 47(4), 79–94. https://doi.org/10.1177/875697281604700407
- Wright, S., Palczynski, R., & ten Have, P. (2017). Crossrail programme organisation and management for delivering London’s Elizabeth line. Proceedings of the Institution of Civil Engineers – Civil Engineering, 170(6), 23–33. https://doi.org/10.1680/jcien.17.00016
- Xiaomin, W., Yiliu, L., & Lei, J. (2016). Reliability assessment of ZPW-2000A track circuit using Bayesian network. International Conference on Reliability, Maintainability and Safety, ICRMS (pp. 3–6). IEEE. https://doi.org/10.1109/ICRMS.2016.8050083[]
- Yan, J., & Wang, X. (2000). Reliability and safety analysis of automatic train protection system. IFAC Proceedings Volumes, 33(9), 615–619.(17)38212-5 https://doi.org/10.1016/s1474-6670
- Yang, Y., & Beijing, J. (2009). Reliability evaluation of a bulk power system for the traction power supply system of a high-speed railway. Annual Symposium on Reliability and Maintainability (RAMS). IEEE.
- Yilin, C., Zhongbei, T., Hillmansen, S., Roberts, C., & Zhao, N. (2019). DC traction power supply system reliability evaluation and robust design (pp. 1153–1158). IEEE.
- Yonghua, L., Pengpeng, Z., Bingzhi, C., & Yuedong, W. (2016). Reliability analysis of bearing system in high speed railway and software implementation. 2016 4th International Conference on Machinery, Materials and Information Technology Applications (763–767). (Icmmita 2016 https://doi.org/10.2991/icmmita-16.2016.142
- Yu, M., Yang, C., & Zhang, S. (2013). Reliability model for control center of railway signalling system based on SRN. Proceedings – 2013 4th International Conference on Digital Manufacturing and Automation, ICDMA (pp. 987–990). https://doi.org/10.1109/ICDMA.2013.231
- Zhen, W., Ding, F., Sheng, L., & Zhengyou, H. (2016). Research on reliability evaluation method of catenary of high speed railway considering weather condition. 2016 International Conference on Probabilistic Methods Applied to Power Systems, PMAPS (pp. 1–6). IEEE. https://doi.org/10.1109/PMAPS.2016.7764080