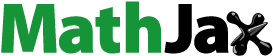
Abstract
The present research is conducted in the southern region of Khyber Pakhtunkhwa, Pakistan, to identify groundwater potential zones (GWPZ). We used three models including Weight of Evidence (WOE), Frequency Ratio (FR), and Information Value (IV) with twelve parameters (elevation, slope, aspect, curvature, drainage network, LULC, precipitation, geology, Lineament, NDVI, road, and soil texture, that have been prepared and integrated into ArcGIS 10.8. The reliability of the applied models’ results was validated using Area Under the Receiver Operating Characteristics (AUROC). The GWPZ were reclassified into five classes, i.e. very low, low, medium, high, and very high zone. The area occupied by mentioned classes using WOE are very low (10.14%), low (19.58%), medium (26.75%), high (27.10%), very high (16.40%), while using FR are very low (20.93%), low (32.38%), medium (18.92%), high (13.13%), very high (14.61%) and using IV are very low (14.41%), low (17.17%), medium (29.01%), high (25.85%), and very High (13.53%). The Success Rate Curve of WOE, FR, and IV were 0.86, 0.91, and 0.87, while the Predicted Rate Curve values were 0.89, 0.93, and 0.90, respectively. The results revealed that all applied statistical models performed very well to delineate GWPZ. However, use of the FR technique is strongly encouraged to evaluate the GWPZ, and its findings are especially useful for managing groundwater resources in urban planning. Our approaches for assessing the GWPZ mapping can be applied in any region with similar scenarios and are recommended as a helpful tool for policymakers to manage groundwater.
1. Introduction
The population of developing countries has increased substantially since the early 1970s and is expected to grow slowly in the ensuing decades (Gerland et al. Citation2014). Human population growth will presumably necessitate expanded farming operations and as expected, exert a new stress level on groundwater and surface water reservoirs. Excessive water extraction can lead to groundwater level decline, ground subsidence, salinity, decreased well production, enhanced pumping costs, and increased saltwater intrusion (M.Y.A. Khan et al. Citation2021). In several parts of the world, 60% of significant aquifer’ surfaces were reduced due to all these changes (Khan, ElKashouty, Zaidi, et al. 2023). Moreover, a significant problem of the twenty-first century is climate change, which increases stresses and influences groundwater (Yawar Ali Khan and ElKashouty Citation2023). Growing demands for water, especially obtaining enough for public demands, is a critical but complicated issue that needs improvement. Emerging water needs, such as the need to obtain enough water to satisfy public expectations, represents another identified as needing improvement (Khan et al. Citation2022).
Groundwater is the prime source of water storage and provides a means to cleverly help combat water scarcity (Khan, ElKashouty, Subyani, et al. 2023). In many nations, groundwater is a crucial natural resource for drinking water, irrigation, and business supply (Das et al. Citation2021; Sutradhar et al. Citation2022). Because of the limited availability of freshwater sources and the overexploitation of most of the world’s groundwater supplies, a severe water crisis is anticipated worldwide by 2025 (Xu et al. Citation2022; Zhu et al. Citation2022). Groundwater is more economically significant than surface water because it is easier to access locally, can adjust its fluctuation in response to consumption, provides an extremely consistent source during droughts, and has excellent quality. The dry and semi-arid regions globally, which have seen multiple drought occurrences in recent years due to irregular inadequate precipitation, are especially affected by water scarcity (Venkatesan et al. Citation2019; Ghosh et al. Citation2021).
In this water stressed region of Karak, GWPZ mapping is a significant mechanism for agriculture development because rain dependent agriculture is the primary revenue source for the District of Karak. Important crops include wheat, oats, mustard, gramme, and other rainfed varieties. Water scarcity results when there is insufficient water to simultaneously meet the demands of ecosystems and humans. Physical water scarcity essentially creates this basic lack of water. There is also economic water scarcity, or the lack of appropriate infrastructure to enable access to what would otherwise be deemed adequate available water resources. Our current study was conducted in an area deemed water scarce and lacking proper water recharge zonation to recharge the aquifer system. Therefore, the objective of our study was to identify the GWPZ of the region that would ultimately enhance sustainable groundwater management for agriculture and domestic purpose. Particularly, the primary purposes were to recharge an aquifer system to a sustainable level and make the community and help them with long-term water problems.
The GWPZ mapping approach has been used in field investigations in recent decades but can be costly and laborious (Li et al. Citation2020; Zhuo et al. Citation2022). Recent technological advances, however, have combined Geographic Information Systems (GIS) and Remote Sensing (RS) to help collect, manipulate, and display various types of data that ultimately generate themed maps (Zhou et al. Citation2022; Zhou and Liu Citation2022). Groundwater availability varies among locations depending on a region’s hydrodynamics, rainfall, landscape, geology, soil texture, gradient, etc. (Karimi-Rizvandi et al. Citation2021). Therefore, these types of parameters are used in a GIS environment to generate the GWPZ.
Scientists worldwide have employed several techniques to understand GWPZs (Islam et al. Citation2023). Among these, Analytical Hierarchy Process (AHP) (Dong, Zhao, et al. Citation2023), Logistic Regression (LR) (Dong, Yang, et al. Citation2023), Frequency Ratio (FR)(Yang et al. Citation2022), Weight of Evidence (WOE) (Tahmassebipoor et al. Citation2016), Random Forest (RF) (Prasad et al. Citation2020), Support Vector Machine (SVM) (Lee et al. Citation2018), Boosted Regression Tree (BRT) (Naghibi et al. Citation2016), Linear Discriminant Analysis (LDA) (Lautz et al. Citation2014), Classification and Regression Tree (CART) (Yin, Wang, T. Li, et al. Citation2022), Machine learning, Artificial Intelligence and Artificial Neural Network (ANN) (Ahmad et al. Citation2022). These techniques can generally be divided into qualitative and quantitative variants to provide an extensive GWPZ of a region. Qualitative methods like AHP are utilized frequently because they eliminate the numerical difficulty in decision-making (Abijith et al. Citation2020). Researchers use the qualitative approach arbitrarily based on their expertise and perspective. A quantitative method is an objective-based approach that generates GWPZ maps by evaluating the dependent and independent variables using mathematical and computational algorithms (Yin, Wang, J. Li, et al. Citation2022). These GIS-based quantitative methods compute the impacts and association of each class of thematic layers with groundwater (Prasad et al. Citation2020; Islam et al. Citation2023).
Herein, we computed the mathematical correlation between groundwater parameters using bivariate statistical techniques including WOE, FR, and IV. The WOE method is the GIS-based quantitative model used for the numerical association of dependent and independent variables to predict GWPZ mapping. This approach has been used for different purposes, including groundwater mapping (Tian et al. Citation2019). Frequency ratio is a widely used and effective mathematical technique for assessing the probabilistic link between several variables in a geographic manner (Tian et al. Citation2020), and it also has been used to map GWPZ (Y. Li et al. Citation2022). The Information Value (IV) technique is a statistical method employed frequently in risk analysis and credit scoring to evaluate the propensity of variables in a dataset (J. Li et al. Citation2022).
These models are significant for the GWPZ mapping because they compute the numerical-based association between dependent and independent variables. Despite the numerical nature and advantages, these algorithms have yet to be investigated by researchers in our study area. The main aims of this research are to (1) compare the bivariate statistical models to generate GWPZ maps of the study area and (2) provide a scientific means for policymakers and stakeholders to manage the water resources in this region.
2. Materials and Methodology
2.1. Study area
Our study was conducted in District Karak of Khyber Pakhtunkhwa (KPK), Pakistan (). Geographically, the Karak is located at latitude and longitude range of 32° 46′ 00′'_ 33° 22′ 00′' North and 70° 43′ 00′' _ 71° 33′ 00′' East (Ahmad et al. Citation2022). Topographically, the study area is part of the southern Kohat plateau of sub-Himalayas, while climatically, the region is arid to semi-arid (Javed et al. Citation2019). The climate is dry and non-monsoonal, With cold winters and hot summers. Winter temperatures can reach 0 °C to 15°Cand hot summertime temperatures range from 25 °C to 40 °C. Briefly, in June and July, the temperature may approach 48 °C. The region also receives substantial winter rainfall as clouds move from Afghanistan in a west-to-east direction. The monsoon season produces summertime precipitation, and an annual precipitation ranges from 13 to 37 cm. Rainfall amounts tend to be greatest in summer (July and September) and also parts of winter (December).
Figure 1. Study area map (a) Pakistan association with internal boundaries (b) Study area occurrence in the province of Khyber Pakhtunkhwa (c) Study area with inventory points and elevation in meters.

Geologically, the research area is located in the Southern Kohat Plateau and is influenced by the Himalaya orogenic deformational event (Rehman et al. Citation2009). The geological age of the regions spans the Plio-Pleistocene to Holocene as indicated by tilting and uplift of the recent terraces along its Southern Topographic front (Bai et al. Citation2019). Geological records suggest that our study area is bordered to the north by the Karak Fault Zone and to the west by the Bannu Basin; to the east, there are the Shakardara Mountains and the southernmost point is bound by the Shinghar-Surghar ridges (U. Khan et al. Citation2021). The region’s Siwalik Group is stratigraphically divided into the Lower, Middle, and Upper Siwaliks, respectively (Nazir-Ur-rehman et al. Citation2020). The Siwalik Group in the region is basically comprised of conglomerates, sandstones, and shales (Nazir-Ur-rehman et al. Citation2020; Li et al. Citation2022). The Plateau is the most twisted portion of the Himalayan Foreland Fold and Thrust Belt to the west.
2.2. Datasets
For this research, we used various types of data to produce the thematic layers in GIS environment. The applied data consists of both ground data and satellite imageries. The ground data were acquired from various national research organizations and field visits in the study area. However, the satellite data were acquired from various international open-source platforms to generate parameters for this study. The comprehensive details of both ground and satellite datasets are demonstrated in ().
Table 1. The comprehensive details of data, its source and purpose.
2.3. Methodology
We depict details of the approach used in the present study in , which consists of the following stages: Initially, the dependent variable (inventory data) map is generated from the ground data (field surveys) and RS data (Sentinel-2, Google Earth). For inventory generation, we first used Google Earth Pro to identify hydrological springs in the study area and exported the KML file of springs to a layer form using KML, and subsequently to a layer tool in GIS platform. In addition to using Google Earth Pro, we used satellite imageries in the Google Earth Engine (GEE) platform using Modified Normalized Difference Water Index (MNDWI). Then, the 12 thematic layers (elevation, slope, aspect, curvature, lineament, drainage, LULC, precipitation, soil, geology, road and NDVI map) were generated using various ground and satellite datasets. Additionally, we computed the associations of both variables. Furthermore, the GWPZ maps were generated using three approaches (WOE, FR and IV). Finally, the accuracy assessment of all algorithms has been performed using the AUROC technique.
2.3.1. Inventory map of the study area
Several previous studies have employed the precise positions of springs, wells, and reservoirs as inventory data for the GWPZ mapping (Prasad et al. Citation2020). In our research here, both ground and satellite data were considered for the analysis to produce GWPZ maps of the study area. One hundred sixteen inventory points across the area of interest were detected using Google Earth Pro, Senitnel-2 data, validated during field visits, and generated the updated inventory of District Karak (). Furthermore, the 116 points-based inventory data were divided into two inventory datasets, i.e. training and testing datasets, using a subset tool in ArcGIS 10.8. The training and testing class consisted of 80% and 20% data. For the training, 80% and 20% of the data were used for modelling and applied for validation, respectively.
2.3.2. Thematic layers preparation for GWPZ mapping
It is crucial to employ the appropriate criteria when generating a GWPZ map for a specific region (Naghibi et al. Citation2018). Based on previous literature (Rahmati et al. Citation2016; Golkarian et al. Citation2018; Bui et al. Citation2019; Chen et al. Citation2019; Jaafarzadeh et al. Citation2021) and our field observations, we considered 12 parameters as influential (elevation, slope, aspect, curvature, lineament, drainage, LULC, precipitation, soil, geology, road and NDVI map) to generate a GWPZ map of the study area using a GIS platform. For modelling purposes, each parameter was reclassified and normalized to the spatial resolution of 12.5 m. Details of these parameters used in this research follow.
2.3.2.1. Elevation
Elevation is the potential indicator and it can high influence the approach to GWPZ mapping (Naghibi et al. Citation2018). Elevation might directly affect rainfall, the amount, frequency, and process of localized precipitation, and thus the recharging of groundwater (Berhanu and Hatiye Citation2020). The elevation map of the current study was generated from ALOS DEM with a 12.5 m resolution. Additionally, the generated elevation map was reclassified into five classes, i.e. <500 m, 500–700 m, 700–900 m, 900–110 m and >1100 m ().
2.3.2.2. Slope
Slope angle is considered the most significant topographic factor affecting groundwater potential (Rahmati et al. Citation2015; Lee et al. Citation2018). Aquifers that store groundwater are replenished more frequently under gentle slopes because they allow rainwater to infiltrate and recharge beneath the surface. Infiltration may be impeded by steeper slopes, increasing surface runoff and decreasing recharge (Maskooni et al. Citation2020). In normal circumstances, more groundwater percolates into the earth, surface water flow velocity is slower, and slopes are less steep. However, the steeper the slope the greater the surface runoff, which leads to reduced groundwater percolation. The slope map was generated in the GIS platform from ALOS DEM, and the slopes were categorized into five classes using the natural break algorithm ().
2.3.2.3. Aspect
Aspect is a fundamental variable for successful GWPZ mapping. Aspect designates the slope’s orientation, which is determined by the exposure to sunshine, wind, and precipitation (Pande et al. Citation2021). Aspect also simulates the vegetation, moisture, air, rock bedding’s structure, and moisture retention, all of which can impact the physical components of soil structure, including sloped materials and possible groundwater zones. Moreover, particularly in dry, arid regions devoid of vegetation, the slope aspect substantially influences hydrological parameters such as evapotranspiration, precipitation direction, and weather process (Ercanoglu and Gokceoglu Citation2002; Benjmel et al. Citation2022). The aspect map was prepared to identify the groundwater possession at various slope orientations. The aspect map was generated from ALOS DEM and reclassified into nine classes using the natural break algorithm in the ArcGIS platform ().
2.3.2.4. Curvature
Curvature is a significant topographic parameter for GWPZ mapping because it influences surface water and subsurface aquifer systems (Regmi et al. Citation2015). The curvature of peak slopes, which represents the topography of the area, is the most important feature to measure to observe possible groundwater areas, gauge water hydrology, and estimate terrain instability. Curvatures provide greater precision in describing the dynamics of subsurface hydrology, soil formation, and soil accumulation. The primary functions of curvature are to influence flow convergence and divergence, and flow acceleration and deceleration (Ercanoglu and Gokceoglu Citation2002; Al-Abadi et al. Citation2016). The curvature map of the current research is computed from ALOS DEM having 12.5 m resolution and reclassified into three classes, i.e. concave, flat and convex (). Convex terrains, characterized by elevated topography, often create conditions favourable for groundwater occurrence. The collection of rainwater and enhanced infiltration in concave terrains, featuring lower elevations, promotes groundwater recharge. Both terrains contribute to groundwater availability, but convex terrains, with their potential for increased recharge, are particularly conducive to groundwater occurrence.
2.3.2.5. Proximity to drainage network
Drainage networks are directly associated with surface runoff and are inversely associated with groundwater storage (Magesh et al. Citation2012). Drainage density, an important metric for assessing the infiltration rate of a location, can significantly impact groundwater potential (Arulbalaji et al. Citation2019). High drainage density values promote runoff and hence signify a zone with little groundwater potential (Muhammad and Khalid Citation2017). Conversely, low drainage density denotes high infiltration, which can significantly raise the potential of groundwater (Prasad et al. Citation2008; Arulbalaji et al. Citation2019). The drainage network of the Karak was extracted from the ALOS DEM and five buffers of <200 m, 200–400 m, 400–600 m, 600–800 m and >800 m were applied on the drainage lines using ArcGIS 10.8 ().
2.3.2.6. Precipitation
Precipitation is considered the most influential parameter for GWPZ mapping. The period and magnitude of precipitation significantly influences the surface flow, recharge, and infiltration (Magesh et al. Citation2012). Rainfall is the primary source of surface and groundwater in this region, thus the volume of the basin’s recharge is greatly influenced by the amount and distribution of that rainfall (Zahir et al. Citation2020). The precipitation map of the present study is computed from the CHIRPS data of 2010 to 2023 using a 33-kernel machine learning algorithm in Google Earth Engine (GEE). The rainfall map was finally reclassified into five classes ().
2.3.2.7. LULC
The LULC of any area governs the transportation of groundwater. LULC is significantly responsible for controlling evapotranspiration, surface water runoff, and replenishment of aquifers (Karimi-Rizvandi et al. Citation2021). Groundwater is crucial for regulating land usage and land cover in an area, and it is also vital for the establishment and accessibility of this resource. It controls several hydrogeological processes, such as surface runoff, evapotranspiration, and infiltration. In addition, groundwater offers valuable information on soil conditions, such as surface and groundwater levels, soil moisture content, and potential indications of groundwater availability (Nguyen et al. Citation2020). Deeper plant roots loosen the ground and cause rocks to collapse, increasing water penetration and subsequently raising groundwater levels (Fatema et al. Citation2023). In the urbanized part of our study area, the surface of the region is impervious which leads to surface runoff and reduced infiltration. In the crop cultivated parts of the region, the land is suitable for water infiltration. The LULC map of the study area was generated using machine learning techniques, i.e. Random Forest (RF) in GEE. The prepared map in GEE was exported into ArcGIS 10.8 for further analysis. The LULC map of the present study consists of 7 classes: tree, shrubland, grassland, cropland, built-up, barren, and water ().
2.3.2.8. Geology
Lithology is the general physical characteristics of rocks and its formations affects groundwater and regulates the aquifer’s permeability and porosity because of its conductivity and penetrability (Islam et al. Citation2023). Geology is the most significant determinant of groundwater potential because penetration is mostly determined by the permeability or conductivity of various rock types (Bera et al. Citation2020). Information on the subsurface geological formations of our study area is provided by the geological map. These material properties impact groundwater presence, storage, and transportation (Muavhi et al. Citation2022). The lithological map of the current research was digitized and prepared from the Northern map of Pakistan using ArcGIS 10.8 ().
2.3.2.9. Lineament
Lineaments are tectonic features that are linear, rectilinear, and curvilinear. They are easily visible in satellite imagery and may be used to characterize a variety of attributes, including faults, master joints, cover of vegetation, topographic linearity and formation, roads, bridges, valleys, straight rivers, and lines between different lithological units (Berhanu and Hatiye Citation2020). Secondary porosity is considered just as important as primary porosity in determining hydrogeological conditions. Lineaments are linear structures of tectonic origin that symbolize secondary porosity and are critical for hydro-geological regulation of groundwater flow and storage (Magesh et al. Citation2012). Lineaments can be located on the globe’s surface in a linear or curved pattern using DEM, and fieldwork data (Magesh et al. Citation2012; Lee et al. Citation2018). The lineament map of the present study was calculated from ALOS DEM hill shades and field survey in the study area, and the buffers were applied on lineament features ().
2.3.2.10. Soil map
Soil mapping is also a significant factor for GWPZ mapping. Soil composition influences water storage because soil characteristics govern the area’s permeability (Karimi-Rizvandi et al. Citation2021). Generally, soil texture affects the soil’s moisture content, infiltration rate, hydraulic conductivity, soil permeability, grain size, and unique composition, all of which can significantly affect recharge potentiality (Lee et al. Citation2017). Our study area is comprised of three types of soil groups including loamy clay, loamy, and mainly loamy ().
2.3.2.11. Normalized Difference vegetation Index (NDVI)
The NDVI has a considerable impact on both groundwater retention and availability. A higher NDVI value indicates dense coverage of plants because vegetation both slows runoff and assists in recharging the groundwater reservoir (Hasanuzzaman et al. Citation2022). The amount of rainfall, temperature, soil adoption, vegetation water content, soil humidity, and evaporation are all related to the change in depth to groundwater. Because evapotranspiration rises during the dry months and precipitation and groundwater recharge in fall, the soil’s fresh water capacity diminishes which may cause related drought stress and restricted growth (Pourtaghi and Pourghasemi Citation2014). The NDVI map of the study () was prepared in GEE and exported to ArcGIS for further analysis.
2.3.2.12. Road distance
A network of roads can adversely impact groundwater. During road construction, the surface porosity and permeability within the strata are reduces because of the lithification phenomenon (Islam et al. Citation2023). Road structure facilitates surface runoff, which in turn are reduced infiltration rate. According to laboratory permeability measurements, loading caused by road embankments greatly decreases the permeability of the alluvial sediments, resulting in an impermeable groundwater system boundary. Numerical modelling in our study demonstrated that groundwater levels rose in the upstream region but decreased downstream when a road embankment served as an impermeable boundary. Furthermore, the intensity of this impact can change with the distance from the road embankment, while the radius of influence of road embankment construction on the upstream area groundwater supplies is determined by the geological and hydraulic conditions (Kim et al. Citation2023). The road map was prepared from the Khyber Pakhtunkhwa Highway authority data, and five buffers were applied in ArcGIS 10.8 ().
3. GWPZ mapping methods
In the present study, three GIS-based bivariate models (WOE, FR and IV) were used to compute the relationship between the dependent variable (inventory data) and independent parameters (groundwater influencing parameters). These models were used to produce GWPZ mapping for the study area. The overview of the models is as follows.
3.1. Weight of Evidence (WOE)
The WOE is a numerical, ‘data-driven’ Bayes rule-based algorithm that forecasts the likelihood of occurrences (Tahmassebipoor et al. Citation2016). The predictive ability of an independent variable with respect to the dependent variable is indicated by WOE. This approach previously was used to generate maps and investigate possible gold dispersion (Kim et al. Citation2018). Since then, the WOE method has been widely used in studies of landslides. Compared to field observation and other deterministic approaches, this method is currently considered dependable and economical (Naghibi and Pourghasemi Citation2015). We used this approach in our study to determine the prospect of groundwater (Corsini et al. Citation2009). The WOE approach was initially considered to assess mineral prospective mapping using GIS-based modelling (Cheng et al. Citation2011). The mathematical formulation of the WOE model is described in depth in Bonham-Carter (Bonham-Carter Citation1994). This approach determines the weight for each class of groundwater precondition parameter’s existence or absence (M or M*) depending on the presence or absence of the inventory pixels (N or N*) as mentioned in Bonham–Carter EquationEquations (1)(1)
(1) and Equation(2)
(2)
(2) (Keller Citation1995) as follow:
(1)
(1)
(2)
(2)
where P is the probability while M is the presence of groundwater parameters, M* is the absence of groundwater pixels, N is the presence of inventory, and N* is the absence of a well. When a component is present or absent, the weights of the evidence are positive weight (W+) and negative weight (W-), respectively EquationEquation (2)
(2)
(2) (Corsini et al. Citation2009).
The magnitude of these comparisons represents the entire spatial relationship between each class of parameter and the groundwater. Positive and negative contrast values imply favourable and unfavourable spatial associations, respectively. The comparison can be computed using EquationEquations (3)(3)
(3) and Equation(4)
(4)
(4) (Tahmassebipoor et al. Citation2016).
(3)
(3)
(4)
(4)
The Final GWPZ delineation is computed through EquationEquation (5)(5)
(5) (Getachew and Meten Citation2021).
(5)
(5)
3.2. Frequency Ratio model (FR)
A FR model is the most frequently used bivariate statistical techniques for research on hazards associated with nature, such as floods, landslides, and droughts (Sarkar et al. Citation2022). In this study FR, a GIS-based bivariate method, was established to identify the GWPZ by analysing the associations between the influencing parameters (Oh et al. Citation2011; Naghibi et al. Citation2015). This algorithm has been used to identify the numerical relationship between dependent variables and independent. The EquationEquation (6)(6)
(6) (Ozdemir Citation2011) has been used to compute the FR:
(6)
(6)
where FR = Frequency, R is the number of pixels from each thematic map that are well connected, and TR is the total number of pixels in the region of interest. Pixel counts for each thematic map and the region of concern are represented by AP and TA, respectively. Greater ratios typically signify stronger correlations between conditioning factors and dependent factors, and vice versa. The average value of an area’s groundwater pixels throughout the entire area is 1. An FR value >1 indicates high probability of groundwater pixel occurrence. Comparatively, values of <1 indicate little relationship in the probabilities of groundwater pixel occurrence. The raster calculator tool in ArcGIS 10 was used to integrate all the reclassified parameters to estimate the GWPZ in our current research using the EquationEquation (7)
(7)
(7) (Guru et al. Citation2017).
(7)
(7)
where
is the groundwater potential zone using FR while
in EquationEquation (7)
(7)
(7) represent elevation, slope, aspect, curvature, drainage network, rainfall, LULC, lineament, soil, Normalized difference vegetation index and road, respectively. The final map using the FR process is presented in .
3.3. Information value (IV)
The IV model is a bivariate statistical technique used to forecast spatial association between groundwater inventory data and groundwater parameters (Yin and Yan Citation1988). Yin and Yan designed this approach, and subsequently modified by Sarkar et al. (Citation2013). The estimated IV helps identify each independent variable class’s contribution to the dependent variables’ occurrence. The IV for each class of a factor map in our study were established based on the existence of groundwater in a particular map unit. The same coordinate system was used to convert each factor map into a raster map and then were divided into distinct classes despite having the same pixel size (12.5 m × 12.5 m). Considering their spatial distribution, the GWPZ map was randomly split into training wells and springs and testing data. Using ArcGIS software, the rasterized training GWPZ map was superimposed over the rasterized groundwater factor maps, and the information value model was used to determine the information values for each factor map’s classes.
The log ratio of conditional probability to prior probability was used to determine the IV of a specific parameter class (Wubalem and Meten Citation2020). The conditional probability was calculated by dividing the groundwater pixels in a single parameter class by the pixel of a subclass of groundwater parameters. However, the prior probability was estimated by dividing the entirety of groundwater pixels of our study area by the total pixels in the entire region (EquationEquation (8)(8)
(8) ).
(8)
(8)
The NSLpix is the number of groundwater pixels in a given class, is the number of pixels in the class, Nts
is the total number of groundwater pixels, and
is the total number of pixels in the study area. In this study, all parameters were reclassified, rasterized, normalized to 12.5 m resolution and converted to WGS_1984, UTM Zone, 43 N projected system.
The EquationEquation (9)(9)
(9) (Farooq and Akram Citation2021) is used to determine GWPZ by summing each variable category.
(9)
(9)
where, M = total number of classes, and
= 1 if i class present in parameter j and 0 if i class is absent in variable j (see for the IV generated map). A class’s weight for each factor was determined by dividing the density of springs and wells by the total area’s density of wells and springs; alternatively, the information value could be used to determine the probability of groundwater in each class and overall. The factor classes with the greatest likelihood of groundwater potential are those whose IV value exceed 0.1. Factor classes with negative values, in contrast, suggest the presence of a component that has no appreciable impact on groundwater potential.
3.4. Validation
Validation is the most significant process for validating the accuracy and reliability of the data used in models. Several techniques have been used to evaluate model performance including common approaches of Area Under the Curve Receiving Operating Characteristic (AUCROC), graphic analysis using Success Rate Curve (SRC) and Predicted Rate Curve (PRC). The values associated with these methods vary from 0.5 to 1.0, with values close to 1.0 denoting the greatest degree of accuracy; values close to 0.5 indicate model inaccuracy (Das and Pardeshi Citation2018). The following classifications distinguish the link between the AUC value and the precision of the predictions: 0.9–1.0 (excellent), 0.8–0.9 (very good), 0.7–0.8 (good), 0.6–0.7 (average) and 0.5–0.6 (poor) (Naghibi et al. Citation2015). SRC is computed using the training dataset, which has been used to run and train the model for GWPZ. Therefore, SRC alone is not a reliable technique to assess any model’s capacity for prediction (Zabihi et al. Citation2016). Hence, Predicted Rate Curve is used based on testing data for the prediction. The PRC represents the model’s ability to predict GWPZ as well as its predictor factors (Lee and Pradhan Citation2007).
4. Results
Understanding how an area’s inventory and GWPZ mapping-effective parameters relate is important. Several predisposing factors can affect groundwater areas’ potentiality (Mukherjee et al. Citation2021). In the present research, we applied 12 parameters including elevation, slope, aspect, curvature, drainage network, LULC, precipitation, geology, NDVI, road and soil texture, using ArcGIS 10.8, utilizing ground data and satellite imageries. We also developed three GIS-based techniques to produce GWPZ for the research region. The association of parameters with inventory data is included in .
Table 2. The statistical analysis of dependent and independent variables.
4.1. Application of WOE for GWPZ mapping
The GWPZ was generated using WOE technique and the output map was further reclassified into five classes i.e. very low, low, medium, high and very high (Jenks Citation1967) natural break algorithm in ArcGIS. In this study the classes occupied 262.09 km2 (10.14%), 506.00 km2 (19.58%), 691.10 km2 (26.75%), 700.18 km2 (27.10%), 423.75 km2 in (16.40%), respectively ().
Table 3. Showing the results of WOE, FR and IV in area km2 (% age).
All factors of the WOE technique were computed for each groundwater conditioning variable ( and ). The spatial relationships between the classes of each conditioning factor and groundwater occurrence are also shown (), together with the numeric value of each applicable variable. A positive or negative spatial correlation indicates a positive or negative contrast value for the WOE model.
We determined that the elevations of our study region consisted of five classes, i.e. <500 m, 500–700 m, 700–900 m, 900–1100 m and >1100 m (). The WOE value for these classes was −2.27, 0.63, 2.91, −0.22 and −0.44, respectively. The results indicated that the greatest groundwater potential zone occurred at 700–900 m, followed by 500–700 m, while the lowest value occurred in elevations <500 m and then those >1100 m. Slopes <10° are the best class for GWPZ, followed by 10–20°, while 30–40° and those >40° demonstrate unreliable associations with inventory data. The WOE for <10° was 0.41, and the association between WOE for >40° was −2.70. These results illustrated a strong positive association between variables using WOE for NE when the value was 0.53 and suggests that NE is the most significant class variable for our study area when using the WOE technique. We also determined that a concave structure is the most feasible class for groundwater potential, while a convex structure did not influence groundwater (). The results suggest that the <200 m drainage class was the least significant class, whereas the >800 m class was influential when WOE was −1.12 and 1.05, respectively. The precipitation factor was subdivided into five classes i.e. <700 mm/year, 700–800, 800–900, 900–1000 and >1000mm/year. The greatest rainfall class i.e. >1000mm/year, demonstrated the most positive correlation between both variables, while the low precipitation class <700 mm/year, had the least association between variables (). The WOE for >1000mm/year was 1.43, followed by 900–1000 mm/year, while <700mm/year has the least association of −2.71. The LULC parameter of this study was classified into seven classes having different associations with dependent variables. We found that forest class strongly associated with the dependent variable, as this class had 2.37 for WOE (). We found that urban and grassland regions had no influential role or association. However, the lithology parameter significantly influenced the GWPZ. The most significant class associated with this factor was Quaternary rocks, with a WOE model value of 0.75, followed by the Rawalpindi group. The lineament predisposing factor had five classes (), and the WOE <500 m class was 0.88, followed by 1500 m. Simultaneously, the >5000 m class had no significant association of −0.70. The WOE for the Loamy class was 0.51 (), suggesting that loamy clayey is the least significant class of this parameters in this study. We determined that the NDVI is an essential signal for subsurface water perspective (). The WOE for the high NDVI class was 0.22. Lastly, the road parameter in this study was categorized into five buffer zones, i.e. <200 m, 200–400 m, 400–600 m, 600–800 m and >800 m classes. Results concluded that road surface restricts water infiltration and adversely impacts GWPZ.
4.2. Application of FR for GWPZ mapping
The FR model was used to generate GWPZ maps and then reclassified into potential zones ranging from very low, low, medium, high, and very high using Natural break algorithm in GIS environment. The zones that we studied contained the following values: very low zone (538.90 km2, or 20.93%), the low zone (833.53 km2, or 32.38%), the medium (487.14 km2, or 18.92%), the high (337.94 km2, or13.13%), and the very high zone (376.07 km2, or, 14.61%) ().
Values reported in indicate each factor’s FR value calculations using the number of pixels and wells in each contributing variable. The FR is calculated using EquationEquations (6)(6)
(6) and Equation(7)
(7)
(7) (). To define GWPZ, 12 parameters that affect groundwater occurrence were combined in the ArcGIS environment. The computed GWPZ using the FR technique was further categorized into five classes, i.e. very low, low, medium, high and very high (). The association of elevation class with inventory data using FR revealed that the greatest potential zone occurred at 500–700 m, followed by 700–900, while the least value occurred in elevations <500 and followed by >1100 m. The FR value for <10° and >40° were 1.22 and 0.07, respectively. The FR for concave and convex structures were 2.05 and 0.42, respectively. The drainage network was categorized into five classes, each with a different association with the dependent variable. The results revealed that the >800 m class was most significant, while <200 m had no influential impact. The WOE for >1000mm/year was 1.43 and for <700mm/year, −2.71. The results demonstrate that <500 m lineament buffer is the most significant class for GWPZ, strongly associating with the dependent variable, while >5000 m class has no significant association with inventory data. Our findings demonstrate that NDVI is an essential sign for groundwater presence (). The FR for the high NDVI class was 0.53. The results conclude that road surface restricts water infiltration and adversely impacts GWPZ.
4.3. Application of IV for GWPZ mapping
The IV technique is a theory largely applied in credit scoring and risk assessment to examine the ability of various factors to forecast various outcomes. The five classes of final map were generated using Natural Break Algorithm in ArcGIS 10.8. The area of 372.46 Km2 (14.41%), 443.67 Km2 (17.17%), 749.52 Km2 (29.01%), 667.97 Km2 (25.85%), and 349.50 Km2 (13.53%) were occupied by very low, low, medium, high, and very high zone class of GWPZ map of IV model (). The statistical results associated with the dependent and independent variables are also provided (). Results revealed that elevation consisted of five classes, i.e. <500, 500–700, 700–900, 900–1100 and >1100, and the IV value for mentioned classes area were −2.04, 0.30, 2.36, −0.20 and −0.43. Results suggested that the greatest potential zone occurred at 500–700 m, followed by 700–900, while the least value occurred in elevation <500 m and followed by >1100 m. The GWPZ used IV and revealed that slope <10° was the best class for GWPZ, followed by 10–20°, while >40° and 30–40° had deleterious association with inventory data. The IV for <10° are 0.20 and −2.69, respectively. The results illustrated that the strong positive association between variables using IV for NE are 0.46, respectively, followed by SE and SW ().
The drainage network was categorized into five classes, each with a different association with the dependent variable. The analytical results of >800 m class for IV were 0.96. We illustrate the greatest rainfall class with the most positive correlation between both variables, and vice versa, in . The IV for >1000mm/year and <700 m was 1.01 − 2.47, respectively. The lithology parameter significantly influenced the GWPZ in this study, where Siwalics, Quaternary, Rawalpindi group and Paleocene occurred in the study area and have a diverse relationship with inventory data. For example, the most significant class of this factor was Quaternary rocks with an IV model values of 0.54, followed by the Rawalpindi group. We found that a < 500 m lineament buffer was the most significant class for GWPZ, strongly associating with the dependent variable. The IV model for <500 m and >5000 m class was 0.84 and −0.11, respectively. Moreover, the loamy sediment had a positive relationship with inventory data, while loamy clayey was an insignificant class (). The WOE, FR and IV for high NDVI were 0.22, 0.53 and 0.21, respectively. For low NDVI scores, they were −1.58, −0.07, and −1.37 respectively. Our research results suggested that the > 800m class was the most significant class for GWPZ map, while the <200 m class had useless association with the dependent variable ().
4.4. Validation
We generated GWPZ in this study using three bivariate models. The model’s validation was essential for authenticating GWPZ mapping (Prasad et al. Citation2020). The ROC curve was frequently utilized in research to statistically verify the models with high predictive values (Golkarian et al. Citation2018; Chen et al. Citation2018). We were able to validate the WOE, FR and IV models using the AUROC curve. The AUC values representing the poorest to the best forecasting models for GWPZ vary from lesser (0) to greater (1). According to the AUCROC graphical display, the accuracy of the forecast values can be categorized into five categories: 0.5–0.6 (poor), 0.6–0.7 (average), 0.7–0.8 (good), 0.8–0.9 (very good), and 0.9–1 (excellent) (Yesilnacar Citation2005). In this study, the SRC for WOE, FR and IV models were 0.86 (86%), 0.91 (91%) and 0.87 (87%), respectively (). The PRC rate for WOE, FR and IV models were 0.89 (89%), 0.93 (93%) and 0.90 (90%), respectively (). The validation results illustrated that all three models perform very well for the current study, but the FR model performed very well compared to WOE and IV models.
5. Discussion
The possibility for high groundwater production is often indicated by springs and wells within specific portions of study areas (Oh et al. Citation2011; Naghibi et al. Citation2017). However, many scholars have tended to apply statistical and machine learning techniques with positive outcomes to evaluate an entire region’s aquifer potential (Oh et al. Citation2011). In this research we used three models (WOE, FR, and WOE) to potentially improve on analyses of to produce GWPZ maps of the study area. In this study, a map with 12 parameters served as an independent variable to compute and subsequently understand its association with groundwater inventory and generate the GWPZ. A poor statistical correlation between dependent and independent variables indicates low potential zones, whereas a strong positive correlation indicates highly potential zones for groundwater. Moreover, the geographic distribution of water wells as indicated by the WOE, FR, and IV, enhances the estimation of corresponding weights of every category of precondition parameter.
Because elevations can significantly influence GWPZ mapping (Pham et al. Citation2021; Zhou et al. Citation2021; Rui et al. Citation2023), the fact that our final GWPZ maps contained five groups (very low, low, medium, high and very high zones) aided our understanding of the effect of this variable. For example, the most significant class for GWPZ mapping was 700–900 m elevation range, which strongly correlated with the dependent variable. Because of such a steep gradient, water cannot pool on the ground’s surface for an extended period and penetrate. Additionally, the low slope regions, i.e. <10° showed a strong association with groundwater potential while >40° showed a poor relationship with groundwater (Luo et al. Citation2022; Cheng et al. Citation2023). High-slope regions support runoff and consequently affect groundwater recharge. High drainage network in basins show that most rainfall is lost as runoff, while low drainage supports infiltration (Mandal et al. Citation2016). The current research observed that low drainage networks exhibited a strong association with groundwater potential and vice versa. Rainfall is the most significant parameter for GWPZ and the primary source of groundwater recharge. As a result, the water table rises in the region, mostly because it receives 1200–1400 mm/year rainfall.
In our study, the greatest association class of rainfall occurred in the highest category (>1000mm/year). Presence and flow of groundwater are facilitated by weathered and fractured strata. Movement and existence of groundwater are also influenced by geological factors, including lithology and lineament (Li et al. Citation2020; Maskooni et al. Citation2020; Qiu et al. Citation2023). As a fault or fracture line, a lineament is a large linear surface feature on a planet that promotes secondary porosity and permeability and supports groundwater recharge (Naghibi et al. Citation2018). In the present research, <500 m buffer zone of lineament showed the greatest correlation with groundwater, while >5000 m buffer was insignificant. Increased NDVI levels raise the probability of finding groundwater, and deep water, for example, is indicated by negative NDVI values (values close to 1). This study observed high associations between groundwater and a high NDVI range. While NDVI can provide valuable insights into vegetation health and its potential relationship with groundwater, we advise that is merely one variable of potentially many influences. Integrating NDVI data with other hydrological and environmental information, such as groundwater monitoring wells, precipitation data, soil properties, and land use patterns, is essential to understand groundwater dynamics and its interactions with the environment comprehensively. Urbanization or impervious surfaces increase surface runoff and decrease infiltration (U. Khan et al. Citation2021). In this study, urbanization, such as buildings and roads, adversely influenced the GWPZ. The GIS-based statistical models have frequently been used (Rahmati et al. Citation2015; Tariq, Mumtaz, et al. Citation2023; Tariq, Hashemi Beni, et al. Citation2023), and results overwhelmingly support use of the FR model for application in the same study when in similar regions.
5.1. Limitations
The precision and dependability of groundwater potential mapping can be impacted by several data and resolution-related limitations. It is essential to comprehend these constraints to evaluate the outcomes and generate plausible knowledge and recommendations. In the current study, we recognized the typical limitations were the data and resolution in GWPZ mapping i.e. the lack of groundwater data (wells) in various regions, high authentic geological data, and insufficient high advance RS data for monitoring. Moreover, our results were also constrained because of missing geophysical data. As a result, the accuracy of the findings could have been enhanced by incorporating additional thematic layers, such as aquifer thickness, groundwater depth before and after monsoon, recharge rate, and the volume of water used for domestic and agricultural uses. Unfortunately, this study could only consider a small number of thematic layers because of the restricted availability of data at the spatial scale. Furthermore, human activities that were not considered in this study but may have a substantial impact on groundwater availability and recharge rates include pumping groundwater for home or agricultural purposes.
A comprehensive strategy including enhanced data collecting, cutting-edge technologies, interdisciplinary cooperation, and continual modelling technique improvement is needed to overcome these constraints. Improving groundwater potential mapping accuracy and dependability requires frequent changes to datasets and models based on fresh data and research discoveries.
6. Conclusion
The current research attempted to evaluate the groundwater potential in the Karak District of Khyber Pakhtunkhwa, Pakistan, using GIS and RS based modelling. In the present study, groundwater has been investigated using three GIS-based bivariate to generate GWPZ map. According to the literature review and field visit of the area, 12 conditioning parameters including elevation, slope, aspect, curvature, drainage network, LULC, rainfall, lithology, lineament, NDVI, soil and road network were considered for computing their association with groundwater inventory data. These 12 parameters were integrated with inventory data in the GIS platform using WOE, FR and IV models to generate GWPZ map and classify it into five zones. The results of all applied models were validated using the AUROC technique to evaluate the reliability of the techniques. The SRC of WOE, FR and IV were 86%, 91% and 87%, while the PRC rate for WOE, FR and IV models were 89%, 93% and 90%, respectively. The current research findings can be helpful for public and commercial organizations engaged in managing groundwater resources, planning land uses, and safeguarding the environment in the studied region. Additionally, the approach of the current study can be used to examine other watersheds with similar hydro-geologic, topographic, and climatic characteristics. However, some measures can be made to improve the replenishment of groundwater, boost water supply, develop rainwater harvesting projects in urban households, etc. Additionally, advanced innovative geospatial techniques are further required to enhance the management of water resources and control overexploitation and unresearched exploration. Sustainably managed water resources can be supported by more multidisciplinary study in these areas, which can lead to more precise, reliable, and useful groundwater potential zone mapping techniques. In the future, researchers must investigate the impacts of climatic change and urbanization on groundwater recharging and potential. The current research recommends use of advanced RS data such as LIDAR, machine learning algorithms, groundwater-surface water interaction, real time monitoring, capacity building and, integrated approach in future to map GWPZ map for sustainable system. The present investigation emphasizes the need for more research that will include socioeconomic aspects, monitor over an extended period, evaluate the findings in the field, and expand the study to other regions. Future projects like these would help manage water resources sustainably, satisfy the growing demand for water in many industries, and improve the understanding of groundwater potential worldwide.
Author contributions
Abdur Rehman: methodology, software, formal analysis, visualization, data curation, writing—original draft. Fakhrul Islam: methodology, software, formal analysis, visualization, data curation, writing—original draft, investigation, validation, writing—review and editing. Ijaz Ul Islam: investigation, validation, writing review and editing. Aqil Tariq: formal analysis, visualization, data curation, writing—review and editing, Supervision. Davis J. Brain: writing—review and editing. Tehmina Bibi: writing review and editing. Waqar Ahmad: writing—original draft, investigation, validation, writing—review and editing. Liaqat Ali Waseem: writing review and editing. Shankar Karuppannan: writing review and editing. Saad Al-Ahmadi: writing review and editing, Funding. All authors have read and agreed to the published version of the manuscript.
Acknowledgements
The authors extend their appreciation to the Researchers supporting project number (RSP2024R384) King Saud University, Riyadh, Saudi Arabia. We also thank the Forest and Wildlife Research Center, and James C. Kennedy Waterfowl and Wetlands Endowed Chair, Mississippi State University, for support.
Disclosure statement
No potential conflict of interest was reported by the authors.
Data availability statement
Data available on the reasonable request from the 1st author of this article.
Additional information
Funding
References
- Abijith D, Saravanan S, Singh L, Jennifer JJ, Saranya T, Parthasarathy KSS. 2020. GIS-based multi-criteria analysis for identification of potential groundwater recharge zones - a case study from Ponnaniyaru watershed, Tamil Nadu, India. HydroRes. 3:1–14. doi: 10.1016/j.hydres.2020.02.002.
- Ahmad K, Banerjee A, Rashid W, Xia Z, Karim S, Asif M. 2022. Assessment of long-term rainfall variability and trends using observed and satellite data in central Punjab, Pakistan. Atmosphere (Basel). 14(1):60. doi: 10.3390/atmos14010060.
- Al-Abadi AM, Al-Temmeme AA, Al-Ghanimy MA. 2016. A GIS-based combining of frequency ratio and index of entropy approaches for mapping groundwater availability zones at Badra–Al Al-Gharbi–Teeb areas, Iraq. Sustain Water Resour Manag. 2(3):265–283. doi: 10.1007/s40899-016-0056-5.
- Arulbalaji P, Padmalal D, Sreelash K. 2019. GIS and AHP techniques based delineation of groundwater potential zones: a case study from Southern Western Ghats, India. Sci Rep. 9(1):2082. doi: 10.1038/s41598-019-38567-x.
- Bai B, Rao D, Chang T, Guo Z. 2019. A nonlinear attachment-detachment model with adsorption hysteresis for suspension-colloidal transport in porous media. J Hydrol. 578:124080. doi: 10.1016/j.jhydrol.2019.124080.
- Benjmel K, Amraoui F, Aydda A, Tahiri A, Yousif M, Pradhan B, Abdelrahman K, Fnais MS, Abioui M. 2022. A multidisciplinary approach for groundwater potential mapping in a fractured semi-arid terrain (Kerdous Inlier, Western Anti-Atlas, Morocco). Water. 14(10):1553. doi: 10.3390/w14101553.
- Bera A, Mukhopadhyay BP, Barua S. 2020. Delineation of groundwater potential zones in Karha river basin, Maharashtra, India, using AHP and geospatial techniques. Arab J Geosci. 13(15):1–21. doi: 10.1007/s12517-020-05702-2.
- Berhanu KG, Hatiye SD. 2020. Identification of groundwater potential zones using proxy data: case study of Megech Watershed, Ethiopia. J Hydrol Reg Stud. 28:100676. doi: 10.1016/j.ejrh.2020.100676.
- Bonham-Carter G. 1994. Geographic information systems for geoscientists: modelling with GIS. doi: 10.1016/C2013-0-03864-9.
- Bui DT, Shirzadi A, Chapi K, Shahabi H, Pradhan B, Pham BT, Singh VP, Chen W, Khosravi K, Ahmad BB, et al. 2019. A hybrid computational intelligence approach to groundwater spring potential mapping. Water. 11(10):2013. doi: 10.3390/w11102013.
- Cheng Q, Bonham-Carter G, Wang W, Zhang S, Li W, Qinglin X. 2011. A spatially weighted principal component analysis for multi-element geochemical data for mapping locations of felsic intrusions in the Gejiu mineral district of Yunnan, China. Comput Geosci. 37(5):662–669. doi: 10.1016/j.cageo.2010.11.001.
- Cheng Y, Lan S, Fan X, Tjahjadi T, Jin S, Cao L. 2023. A dual-branch weakly supervised learning based network for accurate mapping of woody vegetation from remote sensing images. Int J Appl Earth Obs Geoinf. 124:103499. doi: 10.1016/j.jag.2023.103499.
- Chen W, Li H, Hou E, Wang S, Wang G, Panahi M, Li T, Peng T, Guo C, Niu C, et al. 2018. GIS-based groundwater potential analysis using novel ensemble weights-of-evidence with logistic regression and functional tree models. Sci Total Environ. 634:853–867. doi: 10.1016/j.scitotenv.2018.04.055.
- Chen W, Panahi M, Khosravi K, Pourghasemi HR, Rezaie F, Parvinnezhad D. 2019. Spatial prediction of groundwater potentiality using ANFIS ensembled with teaching-learning-based and biogeography-based optimization. J Hydrol. 572:435–448. doi: 10.1016/j.jhydrol.2019.03.013.
- Corsini A, Cervi F, Ronchetti F. 2009. Weight of evidence and artificial neural networks for potential groundwater spring mapping: an application to the Mt. Modino area (Northern Apennines, Italy). Geomorphology. 111(1-2):79–87. doi: 10.1016/j.geomorph.2008.03.015.
- Das S, Pardeshi SD. 2018. Integration of different influencing factors in GIS to delineate groundwater potential areas using IF and FR techniques: a study of Pravara basin, Maharashtra, India. Appl Water Sci. 8(7):197. doi: 10.1007/s13201-018-0848-x.
- Das N, Sutradhar S, Ghosh R, Mondal P, Islam S. 2021. The response of groundwater to multiple concerning drivers and its future: a study on Birbhum District, West Bengal, India. Appl Water Sci. 11(4):79. doi: 10.1007/s13201-021-01410-8.
- Dong W, Yang Y, Qu J, Xiao S, Li Y. 2023. Local Information-enhanced graph-transformer for hyperspectral image change detection with limited training samples. IEEE Trans Geosci Remote Sens. 61:1–14. doi: 10.1109/TGRS.2023.3269892.
- Dong W, Zhao J, Qu J, Xiao S, Li N, Hou S, Li Y. 2023. Abundance matrix correlation analysis network based on hierarchical multihead self-cross-hybrid attention for hyperspectral change detection. IEEE Trans Geosci Remote Sens. 61:1–13. doi: 10.1109/TGRS.2023.3235401.
- Ercanoglu M, Gokceoglu C. 2002. Assessment of landslide susceptibility for a landslide-prone area (north of Yenice, NW Turkey) by fuzzy approach. Environmental Geol. 41(6):720–730. doi: 10.1007/s00254-001-0454-2.
- Farooq S, Akram MS. 2021. Landslide susceptibility mapping using information value method in Jhelum Valley of the Himalayas. Arab J Geosci. 14(10):1–16. doi: 10.1007/s12517-021-07147-7.
- Fatema K, Joy MAR, Amin FMR, Sarkar SK. 2023. Groundwater potential mapping in Jashore, Bangladesh. Heliyon. 9(3):e13966. doi: 10.1016/J.HELIYON.2023.E13966.
- Gerland P, Raftery AE, Sevčíková H, Li N, Gu D, Spoorenberg T, Alkema L, Fosdick BK, Chunn J, Lalic N, et al. 2014. World population stabilization unlikely this century. Science. 346(6206):234–237. doi: 10.1126/SCIENCE.1257469.
- Getachew N, Meten M. 2021. Weights of evidence modeling for landslide susceptibility mapping of Kabi-Gebro locality, Gundomeskel area, Central Ethiopia. Geoenviron Disasters. 8(1):1–22. doi: 10.1186/S40677-021-00177-Z/FIGURES/11.
- Ghosh R, Sutradhar S, Mondal P, Das N. 2021. Application of DRASTIC model for assessing groundwater vulnerability: a study on Birbhum district, West Bengal, India. Model Earth Syst Environ. 7(2):1225–1239. doi: 10.1007/s40808-020-01047-7.
- Golkarian A, Naghibi SA, Kalantar B, Pradhan B. 2018. Groundwater potential mapping using C5.0, random forest, and multivariate adaptive regression spline models in GIS. Environ Monit Assess. 190(3):1–16. doi: 10.1007/s10661-018-6507-8.
- Guru B, Seshan K, Bera S. 2017. Frequency ratio model for groundwater potential mapping and its sustainable management in cold desert, India. J King Saud Univ Sci. 29(3):333–347. doi: 10.1016/j.jksus.2016.08.003.
- Hasanuzzaman M, Mandal MH, Hasnine M, Shit PK. 2022. Groundwater potential mapping using multi-criteria decision, bivariate statistic and machine learning algorithms: evidence from Chota Nagpur Plateau, India. Appl Water Sci. 12(4):1–16. doi: 10.1007/s13201-022-01584-9.
- Islam F, Tariq A, Guluzade R, Zhao N, Shah SU, Ullah M, Hussain ML, Ahmad MN, Alasmari A, Alzuaibr FM, et al. 2023. Comparative analysis of GIS and RS based models for delineation of groundwater potential zone mapping. Geomatics, Nat Hazards Risk. 14(1):27. doi: 10.1080/19475705.2023.2216852.
- Jaafarzadeh MS, Tahmasebipour N, Haghizadeh A, Pourghasemi HR, Rouhani H. 2021. Groundwater recharge potential zonation using an ensemble of machine learning and bivariate statistical models. Sci Rep. 11(1):5587. doi: 10.1038/s41598-021-85205-6.
- Javed T, Sarwar T, Ullah I, Ahmad S, Rashid S. 2019. Evaluation of groundwater quality in district Karak Khyber Pakhtunkhwa, Pakistan. Water Sci. 33(1):1–9. doi: 10.1080/11104929.2019.1626630.
- Jenks GF. 1967. The data model concept in statistical mapping. Int Yearb Cartogr. 7(1):186–190.
- Karimi-Rizvandi S, Goodarzi HV, Afkoueieh JH, Chung IM, Kisi O, Kim S, Linh NTT. 2021. Groundwater-Potential mapping using a self-learning Bayesian network model: a comparison among metaheuristic algorithms. Water. 13(5):658. doi: 10.3390/w13050658.
- Keller CP. 1995. Geographic information systems for geoscientists: modelling with GIS. Comput Geosci. 21(9):1110–1112. doi: 10.1016/0098-3004(95)90019-5.
- Khan MYA, ElKashouty M, Subyani AM, Tian F. 2023. Morphometric determination and digital geological mapping by RS and GIS techniques in Aseer–Jazan contact, Southwest Saudi Arabia. Water. 15(13):2438. doi: 10.3390/w15132438.
- Khan MYA, ElKashouty M, Subyani AM, Tian F, Gusti W. 2021. GIS and RS intelligence in delineating the groundwater potential zones in arid regions: a case study of southern Aseer, southwestern Saudi Arabia. Appl Water Sci. 12(1):3. doi: 10.1007/s13201-021-01535-w.
- Khan MY, ElKashouty M, Tian F. 2022. Mapping groundwater potential zones using analytical hierarchical process and multicriteria evaluation in the central Eastern Desert, Egypt. Water. 14(7):1041. doi: 10.3390/w14071041.
- Khan MYA, ElKashouty M, Zaidi FK, Egbueri JC. 2023. Mapping aquifer recharge potential zones (ARPZ) using integrated geospatial and analytic hierarchy process (AHP) in an arid region of Saudi Arabia. Remote Sens. 15(10):2567. doi: 10.3390/rs15102567.
- Khan U, Faheem H, Jiang Z, Wajid M, Younas M, Zhang B. 2021. Integrating a GIS-based multi-influence factors model with hydro-geophysical exploration for groundwater potential and hydrogeological assessment: a case study in the Karak Watershed, Northern Pakistan. Water. 13(9):1255. doi: 10.3390/w13091255.
- Kim D, Cha BG, Yeo IW. 2023. Impact of road embankment construction on groundwater system in alluvial aquifers. Geosci J. 27(1):89–99. doi: 10.1007/S12303-022-0024-Z/METRICS.
- Kim JC, Jung HS, Lee S. 2018. Groundwater productivity potential mapping using frequency ratio and evidential belief function and artificial neural network models: focus on topographic factors. J Hydroinformatics. 20(6):1436–1451. doi: 10.2166/hydro.2018.120.
- Lautz LK, Hoke GD, Lu Z, Siegel DI, Christian K, Kessler JD, Teale NG. 2014. Using discriminant analysis to determine sources of salinity in shallow groundwater prior to hydraulic fracturing. Environ Sci Technol. 48(16):9061–9069. doi: 10.1021/ES502244V.
- Lee S, Hong SM, Jung HS. 2017. GIS-based groundwater potential mapping using artificial neural network and support vector machine models: the case of Boryeong city in Korea. Geocarto International. 33(8):847–861. doi: 10.1080/10106049.2017.1303091.
- Lee S, Hong SM, Jung HS. 2018. GIS-based groundwater potential mapping using artificial neural network and support vector machine models: the case of Boryeong city in Korea. Geocarto Int. 33(8):847–861. doi: 10.1080/10106049.2017.1303091.
- Lee S, Pradhan B. 2007. Landslide hazard mapping at Selangor, Malaysia using frequency ratio and logistic regression models. Landslides. 4(1):33–41. doi: 10.1007/s10346-006-0047-y.
- Li Q, Lu L, Zhao Q, Hu S. 2022. Impact of Inorganic Solutes’ release in groundwater during oil shale in situ exploitation. Water. 15(1):172. doi: 10.3390/w15010172.
- Li Y, Qian J, Feng S, Chen Q, Zuo C. 2022. Deep-learning-enabled dual-frequency composite fringe projection profilometry for single-shot absolute 3D shape measurement. OEA. 5(5):210021–210021. doi: 10.29026/oea.2022.210021.
- Li J, Wang Z, Wu X, Xu CY, Guo S, Chen X. 2020. Toward monitoring short-term droughts using a novel daily scale, standardized antecedent precipitation evapotranspiration index. J Hydrometeorol. 21(5):891–908. doi: 10.1175/JHM-D-19-0298.1.
- Li J, Zhou N, Sun J, Zhou S, Bai Z, Lu L, Chen Q, Zuo C. 2022. Transport of intensity diffraction tomography with non-interferometric synthetic aperture for three-dimensional label-free microscopy. Light Sci Appl. 11(1):2–14. doi: 10.1038/s41377-022-00815-7.
- Luo J, Niu F, Lin Z, Liu M, Yin G, Gao Z. 2022. Abrupt increase in thermokarst lakes on the central Tibetan Plateau over the last 50 years. CATENA. 217:106497. doi: 10.1016/j.catena.2022.106497.
- Magesh NS, Chandrasekar N, Soundranayagam JP. 2012. Delineation of groundwater potential zones in Theni district, Tamil Nadu, using remote sensing, GIS and MIF techniques. Geosci Front. 3(2):189–196. doi: 10.1016/j.gsf.2011.10.007.
- Mandal U, Sahoo S, Munusamy SB, Dhar A, Panda SN, Kar A, Mishra PK. 2016. Delineation of groundwater potential zones of coastal groundwater basin using multi-criteria decision making technique. Water Resour Manage. 30(12):4293–4310. doi: 10.1007/S11269-016-1421-8/TABLES/3.
- Maskooni EK, Naghibi SA, Hashemi H, Berndtsson R. 2020. Application of advanced machine learning algorithms to assess groundwater potential using remote sensing-derived data. Remote Sens. 12(17):2742. doi: 10.3390/rs12172742.
- Muavhi N, Humphrey K, Mulalo T, Mutoti I, Thamaga KH, Mutoti MI. 2022. Mapping groundwater potential zones using relative frequency ratio, analytic hierarchy process and their hybrid models: case of Nzhelele-Makhado area in South. MutotiGeocarto Int. 37(21):6311–6330. doi: 10.1080/10106049.2021.1936212.
- Muhammad S, Khalid P. 2017. Hydrogeophysical investigations for assessing the groundwater potential in part of the Peshawar basin. Pakistan. Environ Earth Sci. 76(14):1–12. doi: 10.1007/S12665-017-6833-0/FIGURES/8.
- Mukherjee A, Sarkar S, Chakraborty M, Duttagupta S, Bhattacharya A, Saha D, Bhattacharya P, Mitra A, Gupta S. 2021. Occurrence, predictors and hazards of elevated groundwater arsenic across India through field observations and regional-scale AI-based modeling. Sci Total Environ. 759:143511. doi: 10.1016/j.scitotenv.2020.143511.
- Naghibi SA, Moghaddam DD, Kalantar B, Pradhan B, Kisi O. 2017. A comparative assessment of GIS-based data mining models and a novel ensemble model in groundwater well potential mapping. J Hydrol. 548:471–483. doi: 10.1016/j.jhydrol.2017.03.020.
- Naghibi SA, Pourghasemi HR. 2015. A comparative assessment between three machine learning models and their performance comparison by bivariate and multivariate statistical methods in groundwater potential mapping. Water Resour Manage. 29(14):5217–5236. doi: 10.1007/s11269-015-1114-8.
- Naghibi SA, Pourghasemi HR, Abbaspour K, Naghibi SA, Pourghasemi HR, Abbaspour K. 2018. A comparison between ten advanced and soft computing models for groundwater qanat potential assessment in Iran using R and GIS. Theor Appl Climatol. 131(3-4):967–984. doi: 10.1007/s00704-016-2022-4.
- Naghibi SA, Pourghasemi HR, Dixon B. 2016. GIS-based groundwater potential mapping using boosted regression tree, classification and regression tree, and random forest machine learning models in Iran. Environ Monit Assess. 188(1):44. doi: 10.1007/s10661-015-5049-6.
- Naghibi SA, Pourghasemi HR, Pourtaghi ZS, Rezaei A. 2015. Groundwater qanat potential mapping using frequency ratio and Shannon’s entropy models in the Moghan watershed, Iran. Earth Sci Inform. 8(1):171–186. doi: 10.1007/s12145-014-0145-7.
- Nazir-Ur-Rehman Ahmad S, Faisal S, Ali F, Ullah S, Ullah R, Khan MA, Afrasiab, Waqar Azeem M. 2020. Assessment of drinking water quality and human health risks in karak and adjoining areas, Southeastern Kohat Basin, Pakistan. J Himal Earth Sci. 53(1):126–139.
- Nguyen PT, Ha DH, Jaafari A, Nguyen HD, Van Phong T, Al-Ansari N, Prakash I, Le HV, Pham BT. 2020. Groundwater potential mapping combining artificial neural network and real adaboost ensemble technique: the DakNong province case-study, Vietnam. Int J Environ Res Public Health. 17(7):2473. doi: 10.3390/ijerph17072473.
- Oh H-J, Kim Y-S, Choi J-K, Park E, Lee S. 2011. GIS mapping of regional probabilistic groundwater potential in the area of Pohang City, Korea. J Hydrol. 399(3-4):158–172. doi: 10.1016/j.jhydrol.2010.12.027.
- Ozdemir A. 2011. GIS-based groundwater spring potential mapping in the Sultan Mountains (Konya, Turkey) using frequency ratio, weights of evidence and logistic regression methods and their comparison. J Hydrol. 411(3-4):290–308. doi: 10.1016/j.jhydrol.2011.10.010.
- Pande CB, Moharir KN, Panneerselvam B, Singh SK, Elbeltagi A, Pham QB, Varade AM, Rajesh J. 2021. Delineation of groundwater potential zones for sustainable development and planning using analytical hierarchy process (AHP), and MIF techniques. Appl Water Sci. 11(12):1–20. doi: 10.1007/s13201-021-01522-1.
- Pham BT, Jaafari A, Phong TV, Mafi-Gholami D, Amiri M, Van Tao N, Duong V-H, Prakash I. 2021. Naïve Bayes ensemble models for groundwater potential mapping. Ecol Inform. 64:101389. doi: 10.1016/j.ecoinf.2021.101389.
- Pourtaghi ZS, Pourghasemi HR. 2014. GIS-based groundwater spring potential assessment and mapping in the Birjand Township, southern Khorasan Province, Iran. Hydrogeol J. 22(3):643–662. doi: 10.1007/s10040-013-1089-6.
- Prasad P, Loveson VJ, Kotha M, Yadav R. 2020. Application of machine learning techniques in groundwater potential mapping along the west coast of India. GISci Remote Sens. 57(6):735–752. doi: 10.1080/15481603.2020.1794104.
- Prasad RK, Mondal NC, Banerjee P, Nandakumar MV, Singh VS. 2008. Deciphering potential groundwater zone in hard rock through the application of GIS. Environ Geol. 55(3):467–475. doi: 10.1007/S00254-007-0992-3/FIGURES/9.
- Qiu D, Zhu G, Bhat MA, Wang L, Liu Y, Sang L, Lin X, Zhang W, Sun N. 2023. Water use strategy of nitraria tangutorum shrubs in ecological water delivery area of the lower inland river: based on stable isotope data. J Hydrol. 624:129918. doi: 10.1016/j.jhydrol.2023.129918.
- Rahmati O, Nazari Samani A, Mahdavi M, Pourghasemi HR, Zeinivand H. 2015. Groundwater potential mapping at Kurdistan region of Iran using analytic hierarchy process and GIS. Arab J Geosci. 8(9):7059–7071. doi: 10.1007/s12517-014-1668-4.
- Rahmati O, Pourghasemi HR, Melesse AM. 2016. Application of GIS-based data driven random forest and maximum entropy models for groundwater potential mapping: a case study at Mehran Region, Iran. Catena. 137:360–372. doi: 10.1016/j.catena.2015.10.010.
- Regmi NR, Giardino JR, McDonald EV, Vitek JD. 2015. A Review of mass movement processes and risk in the critical zone of earth. In: Dev Earth Surf Process. Vol. 19 [place unknown]; p. 319–362. doi: 10.1016/B978-0-444-63369-9.00011-2.
- Rehman G, Ahmad S, Ali F, Zahid M, Journal P. 2009. Structural Geology of the Shakar Khel Area, Karak District, Khyber Pakhtunkhwa, Pakistan. Pakistan J Hydrocarb Res. 19:11–17.
- Rui S, Zhou Z, Jostad HP, Wang L, Guo Z. 2023. Numerical prediction of potential 3-dimensional seabed trench profiles considering complex motions of mooring line. Appl Ocean Res. 139:103704. doi: 10.1016/j.apor.2023.103704.
- Sarkar S, Roy AK, Martha TR. 2013. Landslide susceptibility assessment using information value method in parts of the Darjeeling Himalayas. J Geol Soc India. 82(4):351–362. doi: 10.1007/s12594-013-0162-z.
- Sarkar D, Saha S, Mondal P. 2022. GIS-based frequency ratio and Shannon’s entropy techniques for flood vulnerability assessment in Patna district, Central Bihar, India. Int J Environ Sci Technol. 19(9):8911–8932. doi: 10.1007/s13762-021-03627-1.
- Sutradhar S, Sarkar D, Bhuimali A, Mondal P. 2022. Integration of different geospatial factors to delineate groundwater potential zones using multi-influencing factors under remote sensing and GIS environment: a study on Dakshin Dinajpur district, West Bengal, India. Sustain Water Resour Manag. 8(1):37. doi: 10.1007/s40899-022-00630-3.
- Tahmassebipoor N, Rahmati O, Noormohamadi F, Lee S. 2016. Spatial analysis of groundwater potential using weights-of-evidence and evidential belief function models and remote sensing. Arab J Geosci. 9(1):1–18. doi: 10.1007/s12517-015-2166-z.
- Tariq A, Hashemi Beni L, Ali S, Adnan S, Hatamleh WA. 2023. An effective geospatial-based flash flood susceptibility assessment with hydrogeomorphic responses on groundwater recharge. Groundw Sustain Dev. 23(8):100998. doi: 10.1016/j.gsd.2023.100998.
- Tariq A, Mumtaz F, Majeed M, Zeng X. 2023. Spatio-temporal assessment of land use land cover based on trajectories and cellular automata Markov modelling and its impact on land surface temperature of Lahore district Pakistan. Environ Monit Assess. 195(1):114. doi: 10.1007/s10661-022-10738-w.
- Tian H, Huang N, Niu Z, Qin Y, Pei J, Wang J. 2019. Mapping winter crops in China with multi-source satellite imagery and phenology-based algorithm. Remote Sens. 11(7):820. doi: 10.3390/rs11070820.
- Tian H, Pei J, Huang J, Li X, Wang J, Zhou B, Qin Y, Wang L. 2020. Garlic and winter wheat identification based on active and passive satellite imagery and the Google earth engine in Northern China. Remote Sens. 12(21):3539. doi: 10.3390/rs12213539.
- Venkatesan G, Pitchaikani S, Saravanan S. 2019. Assessment of groundwater vulnerability using GIS and DRASTIC for Upper Palar River Basin, Tamil Nadu. J Geol Soc India. 94(4):387–394. doi: 10.1007/s12594-019-1326-2.
- Wubalem A, Meten M. 2020. Landslide susceptibility mapping using information value and logistic regression models in Goncha Siso Eneses area, northwestern Ethiopia. SN Appl Sci. 2(5):1–19. doi: 10.1007/S42452-020-2563-0/TABLES/6.
- Xu Z, Li X, Li J, Xue Y, Jiang S, Liu L, Luo Q, Wu K, Zhang N, Feng Y, et al. 2022. Characteristics of source rocks and genetic origins of natural gas in deep formations, Gudian depression, Songliao Basin, NE China. ACS Earth Space Chem. 6(7):1750–1771. doi: 10.1021/acsearthspacechem.2c00065.
- Yang M, Wang H, Hu K, Yin G, Wei Z. 2022. IA-Net: an inception–attention-module-based network for classifying underwater images from others. IEEE J Oceanic Eng. 47(3):704–717. doi: 10.1109/JOE.2021.3126090.
- Yawar Ali Khan M, ElKashouty M. 2023. Watershed prioritization and hydro-morphometric analysis for the potential development of Tabuk Basin, Saudi Arabia using multivariate statistical analysis and coupled RS-GIS approach. Ecol Indic. 154:110766. doi: 10.1016/j.ecolind.2023.110766.
- Yesilnacar EK. 2005. The application of computational intelligence to landslide susceptibility mapping in Turkey.
- Yin L, Wang L, Li J, Lu S, Tian J, Yin Z, Liu S, Zheng W. 2023. YOLOV4_CSPBi: enhanced land target detection model. Land. 12(9):1813. doi: 10.3390/land12091813.
- Yin L, Wang L, Li T, Lu S, Yin Z, Liu X, Li X, Zheng W. 2023. U-Net-STN: a novel end-to-end lake boundary prediction model. Land. 12(8):1602. doi: 10.3390/land12081602.
- Yin KL, Yan TZ. 1988. Statistical prediction models for slope instability of metamorphosed rocks. Landslides Proc 5th Symp Lausanne, 1988, Vol 2. p. 1269–1272. doi: 10.1016/0148-9062(90)90358-9.
- Zabihi M, Pourghasemi HR, Pourtaghi ZS, Behzadfar M. 2016. GIS-based multivariate adaptive regression spline and random forest models for groundwater potential mapping in Iran. Environ Earth Sci. 75(8):2–19. doi: 10.1007/s12665-016-5424-9.
- Zahir M, Ahmedl Z, Ansari MT, Shakir U, Subhan M. 2020. Hydrogeophysical investigation for groundwater potential through electrical resistivity survey in Islamabad, Pakistan. J Geogr Soc Sci. 2(2):147–163. http://jgssjournal.uob.edu.pk/journal/index.php/jgss/article/view/16.
- Zhou G, Deng R, Zhou X, Long S, Li W, Lin G, Li X. 2022. Gaussian Inflection point selection for LiDAR hidden echo signal decomposition. IEEE Geosci Remote Sens Lett. 19:1–5. doi: 10.1109/LGRS.2021.3107438.
- Zhou G, Liu X. 2022. Orthorectification model for extra-length linear array imagery. IEEE Trans Geosci Remote Sens. 60:1–10. doi: 10.1109/TGRS.2022.3223911.
- Zhou G, Li W, Zhou X, Tan Y, Lin G, Li X, Deng R. 2021. An innovative echo detection system with STM32 gated and PMT adjustable gain for airborne LiDAR. Int J Remote Sens. 42(24):9187–9211. doi: 10.1080/01431161.2021.1975844.
- Zhu G, Liu Y, Shi P, Jia W, Zhou J, Liu Y, Ma X, Pan H, Zhang Y, Zhang Z, et al. 2022. Stable water isotope monitoring network of different water bodies in Shiyang River basin, a typical arid river in China. Earth Syst Sci Data. 14(8):3773–3789. doi: 10.5194/essd-14-3773-2022.
- Zhuo Z, Du L, Lu X, Chen J, Cao Z. 2022. Smoothed Lv distribution based three-dimensional imaging for spinning space debris. IEEE Trans Geosci Remote Sens. 60:1–13. doi: 10.1109/TGRS.2022.3174677.