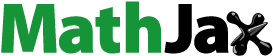
Abstract
The increasing soil erosion (SE) and the associated problems for society, economy, and environment sparked a lot of interest in estimating and mapping SE at different basin scales. The estimation of SE exhibits that SE ranges from 10 to 50 t ha−1 yr−1, with a mean SE of 20 t ha−1 yr−1. The very steep slopes account for 54.21% of total soil loss. The SRB areas where soil loss rates are >10 t ha−1 yr−1 are considered the target areas which account for 27% of the study area and 96% of the soil loss). The high SY is concentrated only in the first-order basins located in a higher slope zone in the northern part of the river. Besides, basin morphometry (basin shape, relative relief) and anthropogenic activities (agricultural land) are retained in the PSLR model as significant factors contributing to SY in the entire river basin.
1. Introduction
In the twenty first century, the exposure of soils to water erosion is increasing, directly leading to soil erosion (SE), sediment yield (SY) and degradation of land (Renschler and Harbor Citation2002; Napoli et al. Citation2016; Singh and Panda Citation2017; Zhang et al. Citation2017; García-Soriano et al. Citation2020; Quesada-Román et al. Citation2021). Climate change, coupled with direct and indirect human innervations, has made the SE phenomenon a serious environmental challenge across the world. Rapid land use and land cover (LULC) changes especially in the forms of deforestation and expansion of agricultural and pasture lands are responsible for acute SE (García-Ruiz et al. Citation2015; Gessesse et al. Citation2015; Quesada-Román and Mata-Cambronero Citation2021; Quesada-Román and Campos-Durán Citation2022). Bhattacharyya et al. (Citation2015) and Pal (Citation2016) mentioned that ∼1964.4 Mha of global soils have faced human-induced erosion, while 1903 Mha have been exacerbated by the work of water. Consequently, the Anthropocene era has observed negative effects of accelerated SE on a variety of topics, including crop production (Balthazar et al., Citation2013), hydro-morphology (Thomas et al. Citation2018), reservoir capacity (John et al. Citation2022), landslides (Kirkby Citation1987), economic loss (Van Pelt et al. Citation2017; Zerihun et al. Citation2018), ecosystem health (Fujaco et al. Citation2016), and many more.
A traditional approach involves estimating the rate of soil erosion (SE) and comprehending their nature, processes, and consequences at a river basin scale. The basic basin morphometric characteristics like shape, size, and relief are explored to understand their direct and indirect influence on the nature of erosion (Das Citation2014; Kadam et al. Citation2019). Previous studies (Jose and Das Citation1982) developed a number of empirical models based on the geomorphological parameter for measuring soil erosion. Using morphometric criteria, Chaudhary and Sharma (Citation1998) assessed and prioritized erosion hazards at sub-watershed level. Numerous empirical techniques, such as the Revised Universal Soil Loss Equation or RUSLE (Wischmeier and Smith Citation1978), and the sediment yield index (Lu et al. Citation2003), are widely utilized in watershed prioritization at the basin level. Garde and Kothari (Citation1987) created an empirical equation for determining average annual sediment output considering catchment area, catchment slope, drainage density, plant cover factor, and yearly precipitation. The equation was based on data from 50 catchments in the plain region of India.
There are many numerical models for estimating soil loss. Though European SE Model (EuroSEM) (Morgan et al. Citation1990), Erosion Productivity Impact Calculator (EPIC), Limburg SE Model (LSEM) (Hudson Citation1981), Areal Nonpoint Source Watershed Environment Response Simulation (ANSWERS) (Beasley et al. Citation1980), Chemicals, Runoff and Erosion from Agricultural Management System (CREAMS) (Knisel Citation1980) are popular and highly capable models for estimating the soil loss, they are rarely found to fit them with the small-scale river basin. Contrarily, the empirical models, for example, the universal soil loss equation (USLE) and its revised version (RUSLE) are well-suited for the estimation of soil loss of small-scale river basins and therefore these models are popularly used across the world (Shamshad et al. Citation2008; Brooks et al. Citation2014; Shivhare et al. Citation2018; Lin et al. Citation2020). Since the USLE and RUSLE techniques do not account for the transportation and deposition of the eroded sediments, they only reflect the overall rate of soil erosion. Therefore, both the sediment delivery ratio (SDR) and the USLE/RUSLE concepts can be coupled to assess soil erosion/deposition and sediment yield (Jain and Das Citation2010; Thomas et al. Citation2018). This coupling approach has been well-acknowledged in recent studies for an in-depth investigation of soil erosion at different spatiotemporal scales. For example, Demir et al. (Citation2022) estimated the soil loss of the Capakcur watershed (Turkey) by applying the RUSLE method and compared the results with the predicted SY and SDR. The watershed is estimated to have lost 96916.20 tons of soil annually, whereas the Capakcur stream transports 68656.09 tons of soil annually. A value of 0.78 was determined for the SDR.The morphometric, hydrologic, soil and LULC factors of a drainage basin have a major role in controlling the processes of soil erosion and sediment delivery. To comprehend the relative influences of these factors, several multivariate regression approaches are being applied. Though Principal Component Analysis (PCA) and Multiple Linear Regressions (MLR) are sophisticated techniques in this arena, PLSR has been most popularly used because it can handle the high inter-predictor multicollinearity (Beusen et al. Citation2005).
Moreover, geospatial techniques are also widely used to address the issue of basin-scale SE (Rahman et al. Citation2009; Aher et al. Citation2014; Chatterjee et al. Citation2014; Gayen et al. Citation2019). Research on multi-criteria decision-making (MCDM) methods for handling complicated decision-making has received a lot of attention in recent years. Instead of utilizing only one criterion, multiple relevant criteria are utilized in this decision-making process to optimize the model, which improves the accuracy of the decision-making process (Chitsaz and Banihabib Citation2015). Using several morphometric characteristics, the application of the MCDM techniques of TOPSIS, SAW, and VIKOR, aids in determining the sub-basin prioritization in a drainage basin based on the degree of susceptibility to SE (Ameri et al. Citation2018; Bhattacharya et al. Citation2020).
The humid subtropical region has been experiencing the acute problem of SE and researchers from diversified disciplines are working relentlessly to understand the dynamics of SE and develop management options. For example, Senanayake et al. (Citation2022) estimated an increase in SE from 9.08 Mg ha−1 yr−1 to 11.08 Mg ha−1 yr-1 during 2000–2019 in Sri Lank and linked it to land degradation and sustainable food production. The National Bureau of Soil Survey (NBSS) estimated that ∼45% (146.8 Mha) area of India exhibits higher susceptibility to SE causing the loss of nutrients of ∼74 million tons every year correspondent to the annual loss of ∼68 billion INR (India national rupees) due to lower crop productivity (Bhattacharyya et al. Citation2015; Lal Citation2015). Eastern India is also experiencing high SE in the wake of the rapid expansion of agricultural land and deforestation. For example, Rajbanshi and Bhattacharya (Citation2020) studied the Konar River basin located in eastern India and observed mean soil loss as 4.42 t ha−1 yr−1. Das et al. (Citation2022) studied the representative catchment of the Chota Nagpur Plateau located in eastern India and estimated and predicted the range of mean erosion rates for cultivated lands (26–35 t ha−1 yr−1), built-up areas (43–48 t ha−1 yr−1), and bare lands (30–36 t ha−1 yr−1) during 2001–2040. Bag et al. (Citation2022) detected 14.48% of the Sobha watershed areas undergoing severe erosion. The study area, located in eastern India, exhibits the problems of SE-induced land degradation. The SRB is principally an agriculture-dominated basin with more than 70% of its total population engaged in agrarian pursuits. Moreover, the intensive agricultural practices without proper management coupled with the accelerated rate of human activities, i.e. deforestation, settlement expansion, and other construction works have exacerbated the recent SE of the SRB. However, the river basin is completely unexplored from the standpoint of SE, land degradation and management. So far, no earlier studies have been focused on the coupling relationship between SE rate and SY in a tropical plateau fringe river basin. Thus, it would be a novel attempt to identify the morphological characteristics of each sub-basin order as well as their unique responses to SE in this small river basin. Analysing soil erosion in small basins offers crucial insights into the environmental, agricultural, hydrological, and economical effects of erosion. The management of water resources, strategies for preventing flooding, land-use planning, and actions to adapt to climate change are all influenced by this comprehension. The study would help the regional planners and other stakeholders to better manage the soil erosion of the agro-based region based on the findings of the severely susceptible SE-prone basins. Therefore, the objectives of the current investigation are (1) to estimate the sub-basin scale SE and SY using the RUSLE-SDR, and sediment production rate (SPR) models, and (2) to find out the underlying processes controlling the SE and sediment production using the PLSR model. The present study would reinstate the central argument that SE and SY decrease with increasing basin order. The novelty is that the current investigation represents a ground-breaking effort to characterize basin order-wise SE potential, and SY using an integrated novel approach.
2. Materials and methods
2.1. Study area
The Sankha River basin (SRB) is situated in eastern India, especially in the Purulia district of West Bengal (). The basin is also a part of the Chota Nagpur Plateau region, which is known for its undulating topography and numerous plateaus. The river originates from Ajodhya Hill (669 m above mean sea level) and joins with the Subarnarekha River at the Chandil Reservoir (23°01′58ʺ N and 86°01′21ʺ N). The river is 35 km long covering basin area 194 km2 (). The tropical humid climate prevails in SRB where the maximum rainfall is received in the monsoon months (June to September) and the average rainfall is observed as 240 mm per year. The geo-physical setting of the basin, i.e. the steep and unstable slope, and less vegetation cover collectively lead to the development of a poor soil profile that has low resistance to external forces like the action of running water. As the basin in its catchment area also maintains high drainage density with the first order streams, therefore, the geo-physical settings provide the ideal environment for immature soil to be eroded (Mahala Citation2018). The LULC dynamics of the basin portray a declining trend of vegetation (55.62 km2 or 28.6% in 2021 from 68.13 km2 or 35.11% in 2011); cultivation land 26.79% in 2021 from 38.30% in 201; and waterbodies (0.2%) (). In contrast, the fallow land has increased by 13%, from 2011 (12.15%) to 26.14% in 2021. Overall, the basin has experienced rapid deforestation, development of settlements, and transportation networks during the last decade. Therefore, all the anthropogenic activities operating in this basin are escalating the SE process.
2.2. Database
Morphometric analysis of the SRB was carried out for 236 first-order, 65 s-order, 17 third-order and 4 fourth-order sub-basins (). The morphometric parameters considered for the present investigation and computed using ArcGIS software (v.10.6) are presented in . First, data from the Shuttle Radar Topography Mission (SRTM) with a resolution of 30 m were obtained from the USGS Earth Explorer (USGS Citation2022). Subsequently, the basin area was demarcated from the DEM and the drainage networks were extracted after the Strahler method. The results have been validated with the Survey of India (SOI) toposheet (73I/4) which has a 1:50,000 scale. In addition, rainfall grid data encompassing 0.5° of geographical resolution from the Climate Research Unit (CRU) were extracted and resampled on 30 m for preparing the SE map based on the RUSLE model (CRU Citation2022). The Food and Agriculture Organisation of the United Nations (FAO) produced a digital soil map of the world at a scale of 1:5.000.000, which was used to collect soil-related data, such as the amount of sand, silt, clay, and organic matter (FAO Citation2022). The LANDSAT TM (November 2011) and OLI (November 2021) satellite images (30 m spatial resolution), acquired from http://earthexplorer.usgs.gov, were utilized to create the LULC map of the basin. Using maximum likelihood algorithms and supervised image classification, the basin’s LULC map was created.
Table 1. Morphometric measurements of the river basin.
2.3. Methods of data processing
depicts the current study, which was carried out using a sound methodology. The first section pertains to the delineation of the watershed area along with numbers, orders, and lengths of streams. The second section covers the morphometric measurements of the river basin (). These measurements lead to an understanding of the hydro-morphological pattern and behaviour of the sub-watersheds and, of course, the nature, mechanism and magnitude of SE in the respective sub-watersheds. Moreover, in the third section, an attempt has also been made to apply the RUSLE model for SE potential followed by SDR and SY models for comparison with the SPR. The next section is concerned with the application of Jose and Das’s (Citation1982) morphometry-based experimental model for quantifying the SPR of the respective sub-watersheds. Finally, the nature of SE of the entire basin has been studied dealing with the underlying processes triggering the SE in varying magnitude.
Figure 2. Methodological flow chart representing the processes followed for the present investigation. The process starts with building the RUSLE model database. Following that, the model’s outcome was validated. The PLSR method has been used to discover probable causes of soil erosion if the results accurately reflect reality. In the event that the validation fails to accurately reflect reality, the RUSLE database has been examined and improved.

2.4. SE estimation by RUSLE model
The model is efficiently used for estimating soil loss of a specific region based on five basic model parameters as presented in EquationEquation (1)(1)
(1) .
(1)
(1)
Where SE is denoted as estimated average SE potential (t ha−1 yr−1), R as a factor of rainfall erosivity measured in MJ mm ha−1 h−1 yr−1, K as a factor of soil erodibility measured in t h M J−1 mm−1, LS as a factor of dimensionless slope length and steepness, C as a factor of land use specific cover management, and P as conservation support practice factor.
The degree of uncertainty in the model depends on the selection of the parameters as model inputs. DEM is one of the important factors that, together with rainfall and soil erodibility parameters, is likely to induce uncertainty in predictions of soil erosion. When estimating soil loss using the RUSLE model, the SRTM-DEM-derived parameters performed better than the Cartosat-DEM and ASTER-DEM generated values and comparable results have been seen in many river basins of India (Mondal et al. Citation2017; Pandey et al. Citation2021). Soil erosion estimates based on SRTM-DEM are thus expected to have less uncertainty. Particularly, the total energy of the rainfall and the maximum 30-minute rainfall intensity (I30) of storm events are rarely available in developing countries, which raises the uncertainty of the conclusions of the model. However, for India’s climatic zones, soil erosion estimates utilising the correlation between the annual rainfall and EI30 values (a rainfall erosivity index based on 30-minutes rainfall intensity) proposed by Singh et al. (Citation1981) produced better findings. Therefore, the same has been followed for deriving the R-factor of SRB. Thus, uncertainties in the present computation tend to be minimal.
2.4.1. Derivation of RUSLE parameters
The derivations of the model parameters followed for the present study have been illustrated in the following sections.
2.4.1.1. Rainfall erosivity factor (R)
R-factor indicates the likelihood of erosion capability of rainfall which is entirely dependent upon the physical characteristics of rainfall e.g. quantity and intensity of rainfall, size and terminal velocity of the raindrop, and kinetic energy (Behera et al. Citation2020). However, the lack of the above mention parameters in the rainfall dataset leads to the adoption of an indirect method for calculating the R-factor (Diodato and Bellocchi Citation2007). EquationEquation (2)(2)
(2) suggested by Wischmeier and Smith (Citation1978) and later on revised by Arnoldus (Citation1980) has been considered for the R factor. The method is widely applied for estimating soil erosion of the small (Prasannakumar et al. Citation2012) and large river basins (Ganasri and Ramesh Citation2016) located particularly in the tropical region. Therefore, SRB located in the tropical region is also suited to this method.
(2)
(2)
where Pi and P, respectively, stand for the monthly and yearly rainfall (mm). For the present study, monthly time series of rainfall data has been used from 1990 to 2021.
2.4.1.2. Soil erodibility factor (K)
It represents the potentiality of the intrinsic erodibility of soil particles by the means of separation and transit of soil particles. Therefore, K-factor is derived considering the physicochemical properties of the soil (top layer) of the studied region. In the RUSLE model the value of K-factor is derived followed by the equation of Wischmeier and Smith (Citation1978). In this method the value of K is derived using the percentage of sand, silt, clay and organic matter present in every soil type (). This method is widely used in the tropical region, predominately in India (Chatterjee et al. Citation2014; Mahala Citation2018). Therefore, we have considered the same methods for estimating this factor (EquationEquation 3–4).
(3)
(3)
where M is the textural factor computed using EquationEquation (4)
(4)
(4) .
(4)
(4)
where OM represents the soil organic matter (%); msilt is the percentage fraction content of silts (0.002–0.5 mm), mvfs is the percentage fraction content of fine sand (0.05–0.1 mm), mc is the percentage fraction content of clay, csoilstr is soil structure code given by USDA, cperm is profile permeability class of USDA.
Table 2. K factor values of soil of SRB.
2.4.1.3. Slope length factor (L)
The L factor denotes the linear distance measured between the initiation of overland flow and the point where the slope is imperceptible leading to the depositional process. Measurement of the L factor in the entire region is both costly and time-consuming. Therefore, remote sensing as an indirect method is widely used for this measurement. Desmet and Govers (Citation1996) first suggested DEM-based measurement for the USA condition. The present study also followed the remote sensing approach and applied EquationEquation (5)(5)
(5) for the derivation of the L factor.
(5)
(5)
where Lij is the L factor having the coordinates of i and j. Aij is the area contributed to the inlet of the respective grid cell with their coordinates (i, j) measured as square metre, and D is the cell size of the respective grid. Xi,j = sin ai,𝑗 + cos ai,𝑗, where the ai,𝑗 indicates the aspect direction of the respective grid cell (i,j), and m is the ratio of β representing the rill to inter-rill erosion and calculated using EquationEquations (6)
(6)
(6) and Equation(7)
(7)
(7) .
(6)
(6)
(7)
(7)
where θ represents the slope angle in degrees. The m has a range of 0 to 1, and it gets close to 0 when the ratio of rill to inter-rill erosion is almost zero.
2.4.1.4. Slope steepness factor (S)
The empirical findings of McCool et al. (Citation1987) work confirmed faster soil loss with a slope steeper than 9%. Following this observation, Renard (Citation1997) adopted an algorithm for computing the S factor for RUSLE (EquationEquations 8(8)
(8) and Equation9
(9)
(9) ).
(8)
(8)
(9)
(9)
where θ represents the slope gradient measured in degrees. Moreover, EquationEquation (7)
(7)
(7) is applied when the slope is <0.09 whereas Eq. has been fitted for the slope ≥ 0.09. The ‘L’ and ‘S’ factors jointly represent the topographic factor that has a direct influence on the process of SE.
2.4.1.5. Crop cover management factor (C)
The ratio of soil loss under a particular crop to that of the base soil is represented by the cover and management factor (Morgan et al. Citation1990). The C-factor indicates how different factors, such as crops, different soil covers, availability of subsurface bio-mass, etc., affect the rate of SE Kouli et al. (Citation2009) and Zhou et al. (Citation2008). Since plot-level identification of crop types with course resolution satellite images is becoming less useful, NDVI has been employed in many research as an alternative for determining C-factor (De Jong et al. Citation1999; Zhou et al. Citation2008; Kouli et al. Citation2009). By lowering the energy of the raindrops during rainfall, vegetation cover stops soil erosion. Nonetheless, the types of vegetation and their density determine how much energy is absorbed by the vegetation. Therefore, NDVI-based C-factor has been determined using (EquationEquation 10(10)
(10) ).
(10)
(10)
where α and β denote the shape parameters used to determine the shape of the NDVI-C curve where α and β are considered as 2 and 1, respectively, according to Van der Knijff et al. (Citation2000).
2.4.1.6. Conservation practices factor (P)
The ratio of soil loss from a field with a certain conservation technique to one where none is used is known as the support practice or P-factor. According to Morgan et al. (Citation1990), the value of P is 1.0 in the absence of conservation measures. By altering the concentration and velocity of runoff, conservation strategies aim to reduce the likelihood of erosion (Renard Citation1997). Therefore, the P-factor values are derived using information about the slope steepness and soil management practice of SRB. It is pertinent to mention here that the land use map has been prepared using the Landsat OLI images (2021) processing while the slope classes have been prepared by ASTER DEM processing. Then, different weights have been assigned to different slope classes and management strategies. The management strategies that significantly lessen SE have been given higher weights, and vice versa as proposed by USDA-SCS (1972). Similarly, different weights are assigned to different slope classes, with higher weights assigned to those reducing soil erosion (Behera et al. Citation2020) (). Finally, in ArcGIS 10.6, a raster overlay process has been used to build the P-factor map integrating the land use and slope maps. The P value ranges from 0 to 1, with values close to 1 indicating areas more vulnerable to erosion (Chatterjee et al. Citation2014).
Table 3. P factor values of different land-use categories of SRB.
2.5. Estimation of SDR and SY
All the individual RUSLE parameters are computed and developed as thematic layers with the help of the ArcGIS 10.6 software. Afterward, all the layers are multiplied using the ‘raster calculator’ to obtain the output the model. The model portrays the spatial variation of the SE map of the SRB. However, it is more important to compute the actual amount of soil leaving the catchment (SY). Therefore, many researchers have already addressed this point and attempted to quantify SDR-based SY in the Indian context following the recommendation of USDA SCS (Vigiak et al. Citation2012; Behera et al. Citation2020; Rajbanshi and Bhattacharya Citation2020). USDA SCS recommendation for SY estimation is very popular, reliable, and simple to address (Behera et al. Citation2020; Rajbanshi and Bhattacharya Citation2020). Therefore the present study has followed the same for estimating the SDR and SY (EquationEquations 11(11)
(11) And Equation12
(12)
(12) ).
(11)
(11)
(12)
(12)
where A denotes the order-wise area of the SRB (km2).
2.6. Estimation of SPR
Many researchers have studied drainage basin morphometric analysis to understand the complexity of the erosion process mechanisms (Kadam et al. Citation2019; Kulimushi et al. Citation2021). For the present study, Jose and Das (Citation1982) morphometry-based experimental model has been followed to estimate the capacity and actual sediment production of the SRB. The model was developed based on the empirical relationship involving specific morphometric parameters such as form factor (Ff), Circularity ratio (Rc), and Compactness coefficient (Cc) (Kulimushi et al. Citation2021). The reasons behind adopting this method are (i) it is suitably applied across the world to a small-scale (Kadam et al. Citation2019; Sofi et al. Citation2021) and large-scale (Kulimushi et al. Citation2021) river basin for estimating SPR, and (ii) the parameters involved in this method can be measured easily. Moreover, as Jose and Das’s (Citation1982) morphometry-based experimental model is very popular in India, the present study also applied it to every stream order (first to fourth) of the SRB (EquationEquation 13(13)
(13) ).
(13)
(13)
where Ff indicates the form factor, Rc represents the circularity ratio, and Cc represents the compactness coefficient ().
2.7. Estimation of runoff rate
The amount of water that is transported via rivers from their sources to other regions is measured as run-off. It was reliant on the rates of precipitation, evapotranspiration, and percolation, each of which was regulated by lithology, relief, climate, and other factors. Because of their low relief, gentle slopes, and consequently good terrain permeability, plain areas typically have low run-off rates, whereas hilly areas typically have high run-off rates because of their high relief as well as steep slopes. The run-off rate of the SRB has been using the Jose and Das (Citation1982) model, which is represented by EquationEq. 14(14)
(14) . The method followed uses logarithmic functions and variables like Ff, Rc, and Cc, and the availability and accuracy of data for these variables influence the choice of the method.
(14)
(14)
2.8. Investigating the influencing factors of erosion using partial least square regression (PLSR)
Partial Least Squares Regression (PLSR) is a statistical technique commonly used for analyzing the relationships between a set of independent variables and a dependent variable, especially when there are high collinearity or multicollinearity issues (Beusen et al. Citation2005). The advantages of principal component analysis (PCA) and multiple linear regression (MLR) are combined in PLSR. (Zhang et al. Citation2011). Therefore, PLSR has been applied to identify the influencing factors of soil erosion. The PLS tool from OriginPro software (v. 2016) was used for the present investigation. Therefore, a total of 26 variables under morphometric, LULC, climate and soil characteristics are considered as the predictors of sediment yield at every sub-basin order of SRB. Finally, PLSR determines which independent variables (predictors) have a significant influence on the dependent variable (erosion) by considering their contributions to both the dependent and independent variance.
3. Results and discussion
3.1. Estimation of SE potential using RUSLE
3.1.1. Spatiality of RUSLE parameters
illustrates the regional variation in the total amount of rainfall (rainfall depth) and its erosivity for the subbasins of the SRB. The rainfall erosivity value correlates directly and linearly with the magnitude of rainfall depth for the majority of the places showing the greatest value. In , an inverse distance weighting (IDW) approach to interpolation has been used to create a map showing the spatial distribution of the R-factor using the point rainfall erosivity data. The mean reading of the rainfall erosivity (measured in MJ mm ha−1 h−1 yr−1) is found to be 738.76 with a standard deviation (SD) of 2.58 and a range of 733.39 to 746.21. The highest value is restricted to the basin’s lower part and while the minimum value is recorded in the upper part of the basin. The spatial variation of the K-factor (measured in t ha MJ−1 ha−1 mm−1) is portrayed in . The mean concentration of the K-factor is recorded as 0.13. The upper part of the SRB exhibits an elevated concentration of the K-factor (0.15) and while it is low in the lower portions of the basin (0.11). AS the SRB is situated on the fringe of the Chotonagpur Plateau and has a tropical humid environment, it is the ideal place for the formation of lithosol soil, which makes up 85% of the study area. The little variation in K-factor values is caused by the uniform geo-climatic conditions of the small river basin. The L and S factors were originally calculated separately, and the topographic factor (LS-factor) was generated by multiplying the LS-factor. The spatial pattern of the LS-factor shown in varied from 0.00 for level terrain to 167.13 for steep slopes. Furthermore, the LS-factor, which has values between 1 and 5, covers more than 80% of the catchment area, suggesting that the topography of the basin is not as variable. The spatial pattern of the C-factor exhibited in ranged from 0.27 recorded in the lower part of the basin to 0.58 located in the upper part of the basin. The P-factor relates to the decreased SE risk brought on by the protective management practices or structures implemented in a particular area. Therefore, the spatial variation of the P-factor was created by incorporating these data into the raster calculator (). The mean P-factor value was revealed to be 0.12 with a range between 0 and 0.75, with 0 representing locations with the lower SE potential as a result of adequate adaptation measures and 0.75 denoting extremely erosion-prone regions as a consequence of a lack of appropriate management approaches.
3.1.2. RUSLE based-SE analysis
3.1.2.1. SE analysis of different erosion severity classes
We employed the raster calculator of the ArcGIS software (v. 10.1) to overlay the six components of RUSLE to calculate the average SE. The annual SE for the entire basin is shown in as a spatial map that shows the variation in SE at the pixel level for the entire river basin. The mean SE is 20 t ha−1 yr−1 ranging from 10 t ha−1 yr−1 to 50 t ha−1 yr−1. Additionally, SE values have been classified into 5 categories – very low erosion area (<10 t ha−1 yr−1), low (10–20 t ha−1 yr−1), moderate (20–30 t ha−1 yr−1), high (30–40 t ha−1 yr−1) and very high (>40 t ha−1 yr−1). In detail, SE < 10 covers 89% of the SRB while SE > 40 covers only 3%. Moreover, 1.1% of the SRB falls into the category of moderate SE risk, while 2.3% falls into the category of low SE risk.
3.1.2.2. Order-wise SE analysis
The SE has also been computed order-wise in t ha−1 yr−1. Regarding the first-order basin, the mean SE has been observed as 173 t ha−1 yr−1 with the range: 0–1792; standard deviation (SD): 280. Out of 236 first order river basins, 31,44,30, 13, and 118 basin record SE in the categories of very low with SE <10 t ha−1 yr−1 (mean: 6.41 t ha−1 yr−1); low SE: 10-20 (mean: 14.35 t ha−1 yr−1); moderate SE: 20-30 t ha−1 yr−1 (mean: 24.81 t ha−1 yr−1); high SE: 30-40 t ha−1 yr−1 (mean: 33.32 t ha−1 yr−1), and very high SE> 40 t ha−1 yr−1 (mean: 330.31 t ha−1 yr−1), respectively. This classification is made based on the rage of SE values and following the classification of SE done by others researches in the Indian context and adjacent to our study area (Dutta et al. Citation2015; Samanta et al. Citation2016; Behera et al. Citation2020) For the classification of SE, A total of 3, 6, 8, and 48 second order subbasins, respectively, exhibit low (mean: 15.27), moderate (mean: 25.91), high (mean: 35.32), and very high (mean: 440.32) SE. Furthermore, only one basin falls into the high (30-40 t ha−1 yr−1) category, while the rest fall into the very high (SE > 40 t ha−1 yr−1, mean: 816.94) category, while one basin falls into the very low (SE <10 t ha−1 yr−1) and moderate (SE: 20-30 t ha−1 yr−1) categories, while the other two fall into the very high (SE> 40 t ha−1 yr−1) category.
3.1.2.3. SE analysis of different slope classes and LULC types
The classes of very gentle and gentle slopes make up about 84% of the basin’s total surface. The average SE from very gentle slopes is 2.22 t ha−1 yr−1 with an SD of 7.08 while the average soil loss rate from gentle slopes is 5.38 t ha−1 yr−1 with an SD of 12.79. Moreover, both slope classes account for 1.63% of the total soil loss. Furthermore, very steep slopes account for about 2.5% of the total basin area () and mainly concentrate in the north portion of the river basin. The very steep slopes account for 54.21% of total soil loss (mean: 267.43 t ha−1 yr−1, SD: 249.21), whereas steep slopes (5.1% of the total SRB) account for 35.40% of total soil loss (mean: 174.63 t ha−1 yr−1, SD: 185.25). Therefore, very steep and steep slopes jointly contribute the lion’s share (71%) of erosion of the basin. Besides, the moderate slopes mainly concentrate in the southern portion of the river basin (7.5% of the total SRB) accounting for 8.75% of the total soil loss (mean: 43.16 t ha−1 yr−1, SD: 72.42) (). The forest land accounts for around 28% of the total basin area followed by agricultural land (27%), fallow land (26%), settlement (13%), and waterbody (4%) (). In the forest land area of the SRB higher soil loss is recorded as high. The increased slope steepness may be the key cause of the higher soil loss rate in the forest. Furthermore, mean soil loss follows the same pattern, with forest land accounting for 58.39%, fallow land accounting for 11.97%, settlement accounting for 8.55%, and agricultural land accounting for 4.06%. The contrasting observations are commonly recorded that human interventions like agricultural land intensify high rates of soil erosion (Rahman et al. Citation2009; Farhan and Nawaiseh Citation2015; Mukanov et al. Citation2019). For example, Ganasri and Ramesh (Citation2016), Nethravathi Basin, India, observed that due to an increase in agricultural area, the gross quantity of erosion had increased to 488,012.6 t yr−1 from 473,339 t yr−1. However, the observation similar to our study has also been recorded by others. For examples, Nyesheja et al. (Citation2019) studied Congo Nile Ridge region of Rwanda and observed that forestland had the highest soil loss rate of 104.93 t ha−1 yr−1, compared to the agricultural land 19.10 t ha−1 yr−1. They also argued that elevated terrain with steep slopes causes the high soil erosion in the forest area.
Table 4. Erosion potential classes and their distribution based on the LULC and slope categories.
3.1.3. RUSLE model findings and other similar studies
Soil erosion models try to approximate the reality, however, there may be some degree of uncertainty in these models. Even though uncertainties related to the model are not shown to be larger than some of the complicated process-oriented models, soil erosion predictions from SRB need to be validated. The outcomes of research related to the study region conducted by others considering similar geo-climatic variables have been used to validate our findings. The estimated rate of soil erosion in the SRB in comparison with other river basins in India with similar geo-climatic conditions indicates a good fit (70–85%). For example, Rajbansi and Bhattacharya assessed SE in the Konar River basin located in a similar geo-climatic setting (physiography, temperature, soil, and vegetation) with human interventions (land use patterns) that closely correspond to the SRB. Their estimate of 4.42 t ha−1 yr−1 for the mean SE rate represents parallel nature of soil erosion. Samanta et al. (Citation2016) used the RUSLE model to study SE along the Subarnarekha River, a distributary of the Sankha River. They found that 26% of the basin area belonged to the very low SE class (less than 5 t ha−1 yr−1), followed by 20% of moderate erosion (between 10 and 20 t ha−1 yr−1). Their findings concur with those of the current study with a similarity of ∼80%. A similar model was used by Mahala (Citation2018) on the Kangsabati River basin (adjacent to SRB) and found that 82% of the basin area experienced low SE with SE 2.5 t ha−1 yr−1. Besides, a large portion of the Kangsabati River basin falls into the low (0.57-0.83 mt ha−1) and medium (0.84–1.08 mt ha−1) SY classes, according to Bhattacharya et al. (Citation2020). Another comparable study conducted by Dutta et al. (Citation2015) on the Sanjal watershed of the Kharkai River sub-basin (in the West Singbhum district of Jharkhand and near the Sankha River) explained that 49 of the basin area fell into the 0–5 t ha−1 yr−1 class. Therefore, their estimation supports the validity of our findings.
3.2. SDR and SY analysis
The SDR values varied from 0.44 to 0.74 (mean: 0.60, SD: 0.05) for first order, from 0.41 to 0.63 (mean: 0.51, SD: 0.04) for second order, from 0.38 to 0.51 (mean: 0.42, SD: 0.04) for third order, and from 0.36 to 0.39 (mean: 0.37, SD: 0.01) for fourth order. Therefore, value indicates SDR in SRB in general for all sub-basin order. However, it is classified into high, moderate and low based on the range of SRD. The high SDR values of first-order basins have been found in hillslope zones, the northern part of SRB (). In 100 basins of the 236 first-order river basins, the SDR was observed high (SDR > 0.62 and above) with a mean 0.65. besides moderate SDR (0.54-0.62) was observed for 111 first-order subbasins scatted in the western part of the basin. Low SDR (<0.54) was recorded for 25 first-order subbasins (mean= 0.51) scattered in the central part of the basin. Regarding second order basins, 18 basins exhibit high SDR (mean: 0.63) located in the northern part of SRB. In contrast, moderate SDR (mean: 0.55) has been recorded for 16 basins located in the north-western portion of SRB. Additionally, 31 basins show the lowest SDR (mean: 0.47) located in the eastern and central parts of SRB (). Additionally, the entire third order (mean: 0.42) and fourth order (mean: 0.37) sub-basins exhibit very low to low SDR (). The SDR values in the Brahmani River basin, which is adjacent to SRB, were discovered to be unevenly distributed and to range from 0.22 to 0.54. A SDR value ranging from 0.22 to 0.32 included roughly 95% of the basin. It was discovered that the minimum basin area was below the 0.32–0.42 SDR range.
Figure 5. Sub-basin order-wise estimation of sediment delivery ratio of the Sankha river. (a) SDR of first order basin, (b) SDR of second order basin, (c) SDR of third order basin, and (d) SDR of fourth order basin.

The values of SY in SRB are classified into six classes: very low (<10 t ha−1 yr−1), low (10-20 t ha−1 yr−1), moderate (20-40 t ha−1 yr−1), moderately high (40-80 t ha−1 yr−1), high (80–160 t ha−1 yr−1), and very high classes (>160 t ha−1 yr−1). depicts the spatial variation of SY of 236 first-order sub-basins. The first-order subbasins have an average SY of 102.37, with 64, 45, 24, 60, 32, and 55 basins falling into the very low (mean: 5.96 t ha−1 yr−1), low (mean: 14.14 t ha−1 yr−1), moderately low (mean: 27.6 t ha−1 yr−1), moderately high (mean: 60.06 t ha−1 yr−1), high (mean: 118.3 t ha−1 yr−1 1), and very high (mean: 322.64 t ha−1 yr−1) SY classes. The second-order subbasins have an average SY of 169.79, with 3, 15, 13, 15, 7, and 23 basins falling very low (mean: 8.51 t ha−1 yr−1), low (mean: 15.37 t ha−1 yr−1), moderately low (mean: 24.47 t ha−1 yr−1), moderately high (mean: 57.53 t ha−1 yr−1), high (mean: 113.44 t ha−1 yr−1), and very high (mean: 409.99 t ha−1 yr−1) SY classes (). The third-order subbasins have an average SY of 87.89 t ha−1 yr−1. The SY variation indicates that 2, 1, 1, 2, and 11 basins fall into low, moderate, moderately high, high, and very high classes (). The fourth-order sub-basins exhibit relatively low SY recording the average 12.08 t ha−1 yr−1 where 2 located in the northern part of the SRB are exhibiting relatively hight SY (). As water and sediment move downstream, they often encounter larger and deeper channels that allow for sediment deposition. Higher order sub-basins may have more significant sediment storage areas, which can reduce the overall sediment yield.
3.3. SPR analysis
The value of SPR of the study basin has been computed by applying the Jose and Das (Citation1982) method which combined the effects of form factor, compactness coefficient, and circulatory ratio in sediment production. As a result, before performing SPR analysis, the variation of the factors was investigated across the entire basin. The elongated basin generally exhibits lower Ff with low peak flow for a longer duration. For example, the Shivganga watershed (part of the Western Ghat region of Maharashtra state, India) has Ff value of 0.42, which indicates that it is a sub-circular to the somewhat elongated basin with low peak flows for a long duration (Magesh and Chandrasekar, Citation2016). For the present study, the mean values of Ff at first, second, third and fourth order basins are observed as 0.46 (range: 0.048-4.50), 0.40 (range: 0.18-0.78), 0.45 (range: 0.21-0.91) and 0.39 (range:0.32-0.48) respectively. Watersheds with low form factors, or 0.14, exhibit their elongated shape with streams running for longer durations and are thus least prone to SE. Besides, the high form factors indicate high peak flows of shorter duration and consequently high erosion. Generally, a basin looks circular in shape when Cc = 1. The basin deviates from its circular shape when Cc > 1. (Altaf et al. Citation2014). For the present study, the mean values of Cc at first, second, third and fourth order basins are observed to be 1.21 (range:1.02–1.71), 1.24 (range: 1.04–1.82), 1.33 (ranger:1.07–1.61) and 1.45 (range:1.26–1.65) respectively. Moreover, the mean values of Cr at first, second, third and fourth order basins are observed as the 0.71 (range:0.34-0.96), 0.66 (range: 0.30-0.92), 0.58 (ranger:0.38-0.86) and 0.48 (range:0.36-0.62) respectively. The inverse relationship between the compactness coefficient and circularity ratio arises because the metrics are essentially measuring opposite aspects of shape. As a shape becomes more compact (less elongated) and approaches a circular form, the compactness coefficient decreases, but the circularity ratio increases (Gravelius, Citation1914; Miller Citation1953). Conversely, as a shape becomes more elongated and deviates from a circular form, the compactness coefficient increases, but the circularity ratio decreases.
The erosion rate for the first-order basin ranges from 0.48 to 15.75 hm/100 km2/yr with an average value of 1.66 hm/100 km2/yr and an SD of 1.22. The first-order streams distributed in the upper portions of the basin portray a high erosion (). Besides, the moderate erosion zone was found in the middle sections of the basin. The SPR values are recorded as high in 4 subbasins (mean: 8.6 hm/100 km2/year), and moderate in 79 subbasins (mean: 2.18). and low in 153 subbasins (mean: 1.21 hm/100 km2/yr). The spatial variation of the erosion rate at the second-order sub-basin is shown in . With an average value of 1.68 hm/100 km2/yr and an SD of 0.54, the second-order basin’s erosion rate ranges from 0.26 to 2.44 hm/100 km2/yr. The upper part of the basin’s second-order streams shows a high potential for erosion. Additionally, the centre portions of the basin had a moderate erosion potential zone. In 33 subbasins, the likelihood of SE is high (mean: 2.12 hm/100 km2/yr), in 14 subbasins it is moderate (mean: 1.59 hm/100 km2/yr), and in 18 subbasins it is low (mean: 0.94 hm/100 km2/yr). The spatiality of the SE at the third-order sub-basin is shown in . Among the 17 third-order sub-basins, the chance of soil erosion is high in 8 subbasins (mean: 2.38 hm/100 km2/yr), moderate in 2 (mean: 2.04 hm/100 km2/yr), and low in 7 subbasins (mean: 1.32 hm/100 km2/yr). High soil erosion at third-order subbasins are mostly located in the north-eastern portion whereas moderate soil erosion are found in the western portion and low erosion are found in the southern portion. The four fourth-order subbasins exhibit an average erosion rate of 1.70 ranging from 0.76 to 2.77 (). In the SRB, the subbasin with the highest rate of SE is found in the western section, while the subbasin with the lowest rate is found in the eastern part.
3.4. Factors controlling soil erosion
The SE potential of the SRB was calculated using the RUSLE model with six commonly used parameters. The catchment area of the basin exhibits high SE with a low R factor, and vice-versa. This signifies that the other factors are strongly influencing the SE potential. Regarding the soil types of SEB the entire basin is dominated by two soil types: lithosol (I) and dystic nitosol (ND). The lithosol (sand: 58.9%; silt:16.2%; clay:24.9%; OC: 0.97% at topsoil sand: 65%; silt: 17%; clay:27%; OC: 0.4% at sub-soil) covers 85% area of the SRB. The soil type ND (sand: 38.9%; silt:17.6%; clay:43.6%; OC: 1.57% at top-soil and sand: 31.9%; silt:13.8%; clay:54.4%; OC: 0.44% at sub-soil) covers 14% of the SRB and highly concentrated in the southern part. Besides, LF is observed in a very negligible portion of the basin. The presence of FL on hills and hill slopes is a common occurrence in SRB. The soil type LF (sand:74.6%; silt:9.6%; clay:15.6%; OC:0.39% at top-soil and sand:67.7%; silt:8.9%; clay:23.4%; OC:0.25% at sub-soil) covers 1% of the SRB.
Lithosol having a shallow nature and limited soil development, often has poor structural stability and is prone to erosion, especially if the rock fragments provide pathways for water to move quickly over the surface. On moderately sloping stable land formations, ND develops. This soil containing high clay content indicates high soil erodibility and resists erosion. While it is inherently highly erodible, the erodibility can still be influenced by factors such as slope, vegetation cover, and land management practices. The FL is characterized by clay illuviation, indicating the movement of clay from upper horizons to lower horizons. The presence of clay can enhance the soil’s ability to retain water and nutrients. However, the erodibility of can still be influenced by factors such as slope steepness and land management practices.
Additionally, the run-off characteristics also support the erosion variability in the magnitude of SE among the sub-basins. For example, among the subbasin, the first-order basin has recorded a low average runoff rate (1.49 cm/km2) compared to the other sub-basins (1.50 cm/km2 for second-order, 1.53 cm/km2 for third order and 3.91 cm/km2 for fourth order basin). These opposite findings indicate that dynamic land use and land cover (especially the expansion of settlement and fallow land and reduction of waterbody area) increase the runoff rate causing significant SE. The majority of the basin’s agricultural land is situated on gentle to moderate slopes. Poor farming practices have an impact on the soil’s surface and structure, especially in agrarian areas with mild to moderate slopes. The influence of the C-factor on SE seems to be of significant relevance due to the greater concentration of agriculture in the basin.
The present investigation has also focused on the relationship between the determinants (independent variables) and SY (dependent variable) and the identification of leading factors at every basin order (first to fourth) using the PLSR model ( and ). Soil erosion potential was first thought to be predicted by 26 independent variables under 5 major categories (8 under basin geometry, 5 under drainage, 4 under topography and climate, and 5 under LULC and 4 under soil). Finally, influencing variables have been retrieved for each dependent variables depending on the proportion of variance explained. Cross-validation analysis, loading PLS factors, XY scores, X weights, and variable importance for the projection (VIP) are among the PLS outcomes. Therefore, this section explains the PLS model’s empirical findings in each basin order. Four elements in the PLSR model of the first order basin have an explained variance (jointly by F1 and F2) greater than 70% (A: 82%, P: 94%, RR: 89%, DI: 89%). Furthermore, we discovered that six elements: P (1.02), CR (1.38), CR (1.39), RR (2.54), DI (2.46), and vegetation (1.047) have VIP greater than 1, implying an influential impact on SY (). Besides, only the variables with weights greater than 0.5 are taken into account when interpreting X weights.
Figure 8. PLSR analysis for identifying the leading factors of SY. (a) Variable importance for the projection (VIP) of SY for the first-order basin, (b) PLSR loading plots for dimension reductions of the factors responsible for SE yield for the first-order basin, (c) Variable importance for the projection (VIP) of SY for second-order basin and (d) PLSR loading plots for dimension reductions of the factors responsible for SE yield for second-order basin.

Figure 9. PLSR analysis for identifying the leading factors of SY. (a) Variable importance for the projection (VIP) of SY for third-order basin, (b) PLSR loading plots for dimension reductions of the factors responsible for SE yield for third-order basin, (c) Variable importance for the projection (VIP) of SY for fourth-order basin and (b) PLSR loading plots for dimension reductions of the factors responsible for SE yield for fourth-order basin.

Table 5. Order-wise factor loading score of different parameters in the PSLR model.
The following are the PLS factor 1 weights for the dependent variable: RR (0.585), DI (0.570), CC, and (0.54) (). This implies that topography plays a significant role in SE. Additionally, the preservation of CR (0.504) and CC (05.07) in PLS factor 2 explains the significance of the basin geometry factor. Likewise, for second order basin, explained variances of more than 70% are retained by RR: 92% and, DI: 94%; VIP of more than 1 is recorded for RR (2.46), DI (2.35) and vegetation (1.27); and X significant weights are RR: −0.55, DI: −0.53 at F1. Therefore, it can be argued that relative relief and dissection index jointly have a significant influence on erosion at the second-order basin scale the correlation coefficient values also confirm the importance of the RR and DI and CC in SY of SRB (). Moreover, for third order basin, explained variances of more than 70% are retained by DF: 86%, DD: 79%, and CCM: 73% LOF: 73%, DT: 87% DI: 71% Cul: 74% and, Fallow: 76%; VIP greater than 1 are recorded for CR (1.02), EL (1.09), FF (1.12), ELL (1.41), DI (1.47) and Cul (1.06); and X significant weights are FF: 0.50, CC: −0.53, ELL: −0.59, RR: −0.57, and DI: −0.55 at F1.
Figure 10. Heatmap explaining the correlations among the dependent and independent variables of SE (L – basin length; A – basin area; P – basin perimeter; Cr – circularity ratio; RR – relative relief; DD – drainage density; DF – drainage frequency; Er – elongation ratio; Ff – form factor; CC – compact coefficient; Di – dissection index; HI – Hypsometric integral; SE – soil erosion; SY – sediment yield; SDR – sediment delivery ratio).

Therefore, it can be argued that basin shape, drainage characteristics, and land use (cultivation pattern) are the key factors contributing to the SE at the third order basin scale. Finally, for fourth order basin, explained variances of more than 70% are retained by L: 80%, FF: 76%, ELL: 78% ELL: 83%, DF: 79% DD: 81% CCM: 84% LOF: 83%, DT: 76, Cul: 93% and, Fallow: 98%, Sett: 88, sand: 70, silt: 70, clay: 70; VIP more than 1 are recorded for L (1.14), EL (1), ELL (1), DF (1), DD (1), EL (1), CCM (1), LOF (1), DT (1), and Cul (1.06); and X significant weights are FF: 0.50, CC: −0.53, ELL: −0.59, RR: −0.57, and DI: −0.55 at F1. and RR: −0.55%, DI: −0.53 at F2. The PLSR approach investigated how the number of components increases as the basin order increases. More importantly, manmade variables such as various land uses have evolved into influencing factors for SE in the higher basin order. In particular, the land use-specific analysis shows that compared to other LULCs, agricultural and fallow land contribute more to SE.
3.5. Implications of the study for land management
The findings of the current study will help the stakeholders for erosion control in the SRB. Selecting severe erosion-prone locations is a pre-requisite strategy for the efficient allocation of scarce resources because conserving all the basin areas suffering from soil loss of different magnitudes is neither economically feasible nor technically possible (Zhou et al. Citation2019). To enhance the health of the landscape ecology by boosting ecosystem diversity and productivity, many studies across the world have estimated the rate of SE at different spatio-temporal scales and focused on the regions that are most vulnerable to SE. For example, Elnashar et al. (Citation2021) proposed an urgent policy impact for the areas of the Blue Nile Basin where SE is occurring at a rate of more than 10 t ha−1 yr−1. Furthermore, it has been demonstrated that countries in Asia, Europe, Africa, and America focus their policy recommendations on areas with erosion rates higher than 20 t ha−1 yr−1 (Aneseyee et al. Citation2020; Majhi et al. Citation2021). On the other side, it has been observed that in tropical India, where SE rates exceed 20 t ha−1 yr−1 in large river basins, attention is being paid to SE mitigation through civil and bioengineering approaches. Small river basins with SE rates of greater than 20 t ha−1 yr−1, however, are planned as priority sites for SE control. SE is posing challenges to the small SRB in the tropical area. Therefore, in the areas of SRB where soil loss rates are more than 10 t ha−1 yr−1, we recommend urgent, focused implementation. These target regions make up 27% of the research area and represent 96% of the soil loss rate in the SRB.
To reduce soil loss in such target areas, appropriate land management techniques should be put into place. As the northern portion of the basin experiences high soil erosion due to steep slopes physical measures such as soil bunds, stone bunds and percolation ditches may be implemented. Besides, agronomic practices (changes in cropping and land management practices such as contour ploughing, and terracing can adopted to minimize soil erosion. Moreover, improved agronomic practices are recommended for the areas located in the middle and western parts of the SRB that have low to moderate SE so that future erosion may be checked. Therefore, to successfully address erosion issues, conservation planning might be improved in the SRB using the results of the current study. This could create chances for collaboration between the local government and the local population.
4. Conclusions
The basin-scale SE study of the Sankah River reveals that most of the river basin has low SE, and the upper portion of the SRB is more susceptible to erosion. With a range of 10 t ha−1 yr−1 to 50 t ha−1 yr−1, the mean SE is 20 t ha−1 yr−1. In particulars, low erosion potential (SE < 10 t ha−1 yr−1) covers 89% of the SRB, whereas ow erosion potential (SE > 40 t ha−1 yr−1) only accounts for 3% of the SRB. The forest land has experienced high soil loss due to steep slopes, accounting for 58.39% of the total. Besides, the results of sediment delivery ratio and sediment yield have also been integrated with SE. The average SDR for first, second, third and fourth order basins are 0.60, 0.63, 0.42, and 0.37 respectively while the average SY of the basin varies from 102.37 to 332.4 t ha−1 yr−1. The PSLR models reveal that circularity ratio, compactness coefficient, relative relief, and dissection index are the main factors controlling soil erosion for the first-order river basin. Besides, relative relief, dissection index, and vegetation cover for the second-order River basin. circularity ratio, ellipticity index, form factor, compactness coefficient, basin elongation, relative relief, dissection index, cultivation for third order and basin length, basin elongation, drainage frequency, drainage density, constant of channel maintenance fallow land, cultivation land, settlement for fourth order are the significant factors for promoting the soil erosion.
Therefore, the explored method shows that increasing the basin order increases the number of factors. More importantly, anthropogenic factors such as different land uses have become influential factors for SE in the higher basin order. Precisely, the land use-specific study indicates that fallow and agricultural land contribute more to SE than other LULCs. Besides, the slope-specific study indicates that the steeper slope, often found in the upper (north) part of the basin, is more prone to SE. Therefore, the present study clearly demonstrates that different basin orders require different management techniques to prevent SE. Especially, the lower-order basin demands slope stability through aforestation, systematic agricultural practice, controlling the headward erosion of gullies, etc. Overall, the higher-order basins demand controlled anthropogenic activities and sustainable restoration of degraded lands.
The present effort involves research to characterize soil erosion in light of the present-day situation. This approach aims to depict spatiotemporal variations more accurately, fostering the integration of basin-scale erosion dynamics with land management strategies. Furthermore, future land management would better be framed if social perspectives especially social needs and demand could be integrated. Another dimension emanating from the present investigation is the application of sophisticated geospatial and geostatistical models to simulate the future trend of erosion dynamics in different geo-climatic, physiographic environments.
Supplemental Material
Download MS Word (2 MB)Disclosure statement
There is no conflict of interest.
Data availability statement
Data available on request from the authors.
References
- Aher PD, Adinarayana J, Gorantiwar SD. 2014. Quantification of morphometric characterization and prioritization for management planning in semi-arid tropics of India: a remote sensing and GIS approach. J Hydrol. 511:850–860. doi:10.1016/j.jhydrol.2014.02.028.
- Altaf S, Meraj G, Romshoo SA. 2014. Morphometry and land cover based multi-criteria analysis for assessing the soil erosion susceptibility of the western Himalayan watershed. Environ Monit Assess. 186(12):8391–8412. doi:10.1007/s10661-014-4012-2.
- Ameri AA, Pourghasemi HR, Cerda A. 2018. Erodibility prioritization of sub-watersheds using morphometric parameters analysis and its mapping: a comparison among TOPSIS, VIKOR, SAW, and CF multi-criteria decision making models. Sci Total Environ. 613–614:1385–1400. doi:10.1016/j.scitotenv.2017.09.210.
- Aneseyee AB, Elias E, Soromessa T, Feyisa GL. 2020. Land use/land cover change effect on SE and sediment delivery in the Winike watershed, Omo Gibe Basin, Ethiopia. Sci Total Environ. 728:138776. doi:10.1016/j.scitotenv.2020.138776.
- Arnoldus HMJ. 1980. An approximation of the rainfall factor in the Universal Soil Loss Equation. In: De Boodt M, Gabriels D, editors. Assessment of erosion. New York: John Wiley and Sons; p.127–132.
- Bag R,Mondal I,Dehbozorgi M,Bank SP,Das DN,Bandyopadhyay J,Pham QB,Fadhil Al-Quraishi AM,Nguyen XC. 2022. Modelling and mapping of soil erosion susceptibility using machine learning in a tropical hot sub-humid environment. J. Cleaner Prod. 364:132428 doi:10.1016/j.jclepro.2022.132428.
- Balthazar V,Vanacker V,Girma A,Poesen J,Golla S. 2013. Human impact on sediment fluxes within the Blue Nile and Atbara River basins. Geomorphology. 180–181:231–241. doi:10.1016/j.geomorph.2012.10.013.
- Beasley DB, Huggins LF, Monke A. 1980. ANSWERS: a model for watershed planning. Trans ASAE. 23(4):938–944.
- Behera M, Sena DR, Mandal U, Kashyap PS, Dash SS. 2020. Integrated GIS-based RUSLE approach for quantification of potential SE under future climate change scenarios. Environ Monit Assess. 192(11):733. doi:10.1007/s10661-020-08688-2.
- Beusen AHW, Dekkers ALM, Bouwman AF, Ludwig W, Harrison J. 2005. Estimation of global river transport of sediments and associated particulate C, N, and P. Global Biogeochem Cycle. 19(4). doi:10.1029/2005GB002453.
- Bhattacharya RK, Chatterjee ND, Das K. 2020. Sub-basin prioritization for assessment of SE susceptibility in Kangsabati, a plateau basin: a comparison between MCDM and SWAT models. Sci Total Environ. 734:139474. doi:10.1016/j.scitotenv.2020.139474.
- Bhattacharyya R, Ghosh B, Mishra P, Mandal B, Rao C, Sarkar D, Das K, Anil K, Lalitha M, Hati K, et al. 2015. Soil degradation in India: challenges and potential solutions. Sustainability. 7(4):3528–3570. doi:10.3390/su7043528.
- Brooks A, Spencer J, Borombovits D, Pietsch T, Olley J. 2014. Measured hillslope erosion rates in the wet-dry tropics of Cape York, northern Australia: part 2, RUSLE-based modeling significantly over-predicts hillslope sediment production. Catena. 122:1–17. doi:10.1016/j.catena.2014.06.002.
- Chatterjee S, Krishna AP, Sharma AP. 2014. Geospatial assessment of SE vulnerability at watershed level in some sections of the Upper Subarnarekha river basin, Jharkhand, India. Environ Earth Sci. 71(1):357–374. doi:10.1007/s12665-013-2439-3.
- Chitsaz N, Banihabib ME. 2015. Comparison of different multi criteria decision-making models in prioritizing flood management alternatives. Water Resour Manage. 29(8):2503–2525. doi:10.1007/s11269-015-0954-6.
- Chorley RJ. 1969. The drainage basin as the fundamental geomorphic unit. In: Chorley RJ, editor. Water, earth and man, London: Methuen; p. 77–100.
- Chaudhary RS, Sharma PD. 1998. Erosion hazard assessment and treatment prioritization of Giri River catchment, North Western Himalayas. Indian J Soil Conserv. 26(1):6–11.
- CRU. 2022. CRU TS v4.06 Data Variables: PRE. University of East Anglia. [accessed 15 Jul 2022]. https://crudata.uea.ac.uk/cru/data/hrg/cru_ts_4.06/cruts.2205201912.v4.06/pre/.
- Das A, Remesan R, Chakraborty S, Gupta AK. 2022. Investigation of human-induced land use dynamics in a representative catchment on the Chota Nagpur Plateau, India: a spatiotemporal application of SE modeling with connectivity index studies. CATENA. 217:106524. doi:10.1016/j.catena.2022.106524.
- Das D. 2014. Identification of erosion prone areas by morphometric analysis using GIS. J Inst Eng India Ser A. 95(1):61–74. doi:10.1007/s40030-014-0069-8.
- De Jong SM, Paracchini ML, Bertolo F, Folving S, Megier J, De Roo APJ. 1999. Regional assessment of soil erosion using the distributed model SEMMED and remotely sensed data. Catena. 37(3-4):291–308. doi:10.1016/S0341-8162(99)00038-7.
- Demir Y, Meral A, Demir AD. 2022. Estimation of soil losses in Çapakcur watershed (Bingol, Turkey) using RUSLE method and comparison of predicted soil losses with sediment yield. Kahramanmaraş Sütçü İmam Üniversitesi Tarım ve Doğa Dergisi. 25(Ek Sayı 2):523–537. doi:10.18016/ksutarimdoga.vi.1059631.
- Desmet PJJ, Govers G. 1996. A GIS procedure for automatically calculating the USLE LS factor on topographically complex landscape units. J Soil Water Conserv. 51(5):427–433.
- Diodato N, Bellocchi G. 2007. EstimaIg monthly (R) USLE climate input in a Mediterranean region using limited data. J Hydrol. 345(3-4):224–236. doi:10.1016/j.jhydrol.2007.08.008.
- Dutta D, Das S, Kundu A, Taj A. 2015. SE risk assessment in Sanjal watershed, Jharkhand (India) using geo-informatics, RUSLE model and TRMM data. Model Earth Syst Environ. 1(4):1–9. doi:10.1007/s40808-015-0034-1.
- Elnashar A, Zeng H, Wu B, Fenta AA, Nabil M, Duerler R. 2021. SE assessment in the Blue Nile Basin driven by a novel RUSLE-GEE framework. Sci Total Environ. 793:148466. doi:10.1016/j.scitotenv.2021.148466.
- FAO. 2022. FAO Soils Portal, FAO/UNESCO Soil Map of the World. Accessed 20 Aug 2022. https://www.fao.org/soils-portal/data-hub/soil-maps-and-databases/faounesco-soil-map-of-the-world/en/.
- Farhan Y, Nawaiseh S. 2015. Spatial assessment of soil erosion risk using RUSLE and GIS techniques. Environ Earth Sci. 74(6):4649–4669. doi:10.1007/s12665-015-4430-7.
- Fujaco MAG, Leite MGP, Neves AHCJ. 2016. A GIS-based tool for estimating soil loss in agricultural river basins. REM Int Eng J. 69(4):417–424. doi:10.1590/0370-44672015690197.
- Ganasri BP, Ramesh H. 2016. Assessment of soil erosion by RUSLE model using remote sensing and GIS-A case study of Nethravathi Basin. Geosci Front. 7(6):953–961. doi:10.1016/j.gsf.2015.10.007.
- García-Ruiz JM, Beguería S, Nadal-Romero E, González-Hidalgo JC, Lana-Renault N, Sanjuán Y. 2015. A meta-analysis of soil erosion rates across the world. Geomorphology. 239:160–173. doi:10.1016/j.geomorph.2015.03.008.
- García-Soriano D, Quesada-Román A, Zamorano-Orozco JJ. 2020. Geomorphological hazards susceptibility in high-density urban areas: a case study of Mexico City. J South Am Earth Sci. 102:102667. doi:10.1016/j.jsames.2020.102667.
- Gayen A, Pourghasemi HR, Saha S, Keesstra S, Bai S. 2019. Gully erosion susceptibility assessment and management of hazard-prone areas in India using different machine learning algorithms. Sci Total Environ. 668:124–138. doi:10.1016/j.scitotenv.2019.02.436.
- Gessesse B, Bewket W, Bräuning A. 2015. Model‐based characterization and monitoring of runoff and SE in response to land use/land cover changes in the Modjo watershed, Ethiopia. Land Degrad Dev. 26(7):711–724. doi:10.1002/ldr.2276.
- Gravelius H. 1914. Grundriß der gesamten Gewässerkunde, Band 1: Flußkunde, Göschen Verlag. 1, 1.
- Garde RJ, Kothari UC. 1987. Sediment yield estimation. J Irrig Power (India). 44(3):97–123.
- Horton RE. 1932. Drainage-basin characteristics. Trans Am Geophys Union. 13:350–361.
- Horton RE. 1945. Erosional development of streams and their drainage basins: hydrophysical approach to quantitative morphology. Geol Soc America Bull. 56(3):275–370. doi:10.1130/0016-7606(1945)56[275:EDOSAT]2.0.CO;2.
- Hudson NW. 1981. Soil conservation. London: Batsford.
- Jain MK, Das D. 2010. Estimation of sediment yield and areas of soil erosion and deposition for watershed prioritization using GIS and remote sensing. Water Resour Manage. 24(10):2091–2112. doi:10.1007/s11269-009-9540-0.
- John J, Rosamma CN, Thampi SG. 2022. Assessment and prediction of SE and its impact on the storage capacity of reservoirs in the Bharathapuzha River Basin, India. Environ Model Assess. 27(1):77–103. doi:10.1007/s10666-021-09786-2.
- Jose CS, Das DC. 1982. Geomorphic prediction models for sediment production rate and intensive priorities of watersheds in Mayurakshi catchment. In: Proc. of International Symp. On Hydrological Aspects of Mountainous Watersheds, School of Hydrology, UOR, Roorkee (Vol. 1, p. 15–23).
- Kadam AK, Jaweed TH, Kale SS, Umrikar BN, Sankhua RN. 2019. Identification of erosion-prone areas using modified morphometric prioritization method and sediment production rate: a remote sensing and GIS approach. Geomatics Nat Hazards Risk. 10(1):986–1006. doi:10.1080/19475705.2018.1555189.
- Kirkby MJ. 1987. Modelling some influences of SE, landslides and valley gradient on drainage density and hollow development. Catena. Supp (Giessen) (10):1–14.
- Knisel WG. 1980. CREAMS: a field scale model for chemicals, runoff, and erosion from agricultural management systems (No. 26). United States: Department of Agriculture, Science and Education Administration.
- Kouli M, Soupios P, Vallianatos F. 2009. Soil erosion prediction using the revised universal soil loss equation (RUSLE) in a GIS framework, Chania, Northwestern Crete, Greece. Environ Geol. 57(3):483–497. doi:10.1007/s00254-008-1318-9.
- Kulimushi LC,Choudhari P,Mubalama LK,Banswe GT. 2021. GIS and remote sensing-based assessment of soil erosion risk using RUSLE model in South-Kivu province, eastern, Democratic Republic of Congo. Geomatics Nat. Hazards Risk. 12(1):961–987. doi:10.1080/19475705.2021.1906759.
- Lal R. 2015. Restoring soil quality to mitigate soil degradation. Sustainability. 7(5):5875–5895. doi:10.3390/su7055875.
- Lin J, Guan Q, Tian J, Wang Q, Tan Z, Li Z, Wang N. 2020. Assessing temporal trends of SE and sediment redistribution in the Hexi Corridor region using the integrated RUSLE-TLSD model. Catena. 195:104756. doi:10.1016/j.catena.2020.104756.
- Lu XX, Ashmore P, Wang J. 2003. Sediment yield mapping in a large river basin: the Upper Yangtze, China. Environ Model Softw. 18(4):339–353. doi:10.1016/S1364-8152(02)00107-X.
- Magesh NS,Chandrasekar N. 2016. Assessment of soil erosion and sediment yield in the Tamiraparani sub-basin, South India, using an automated RUSLE-SY model. Environ Earth Sci. 75(16). doi:10.1007/s12665-016-6010-x.
- Mahala A. 2018. SE estimation using RUSLE and GIS techniques—a study of a plateau fringe region of tropical environment. Arab J Geosci. 11(13):1–18. doi:10.1007/s12517-018-3703-3.
- Majhi A, Shaw R, Mallick K, Patel PP. 2021. Towards improved USLE-based SE modelling in India: a review of prevalent pitfalls and implementation of exemplar methods. Earth Sci Rev. 221:103786. doi:10.1016/j.earscirev.2021.103786.
- McCool DK, Brown LC, Foster GR, Mutchler CK, Meyer LD. 1987. Revised slope steepness factor for the Universal Soil Loss Equation. Trans ASAE. 30(5):1387–1396.
- Miller VC. 1953. A quantitative geomorphic study of drainage basin characteristics in the clinch mountain area virginia and tennessee, Defense Technical Information Center. 1, 1.
- Mondal A, Khare D, Kundu S, Mukherjee S, Mukhopadhyay A, Mondal S. 2017. Uncertainty of soil erosion modelling using open source high resolution and aggregated DEMs. Geosci Front. 8(3):425–436. doi:10.1016/j.gsf.2016.03.004.
- Morgan RPC, Quinton JN, Rickson RJ. 1990. Structure of the SE prediction model for the European community. In Proceedings of International Symposium on Water Erosion, Sedimentation and Resource Conservation (Vol. 913).
- Mukanov Y, Chen Y, Baisholanov S, Amanambu AC, Issanova G, Abenova A, Fang G, Abayev N. 2019. Estimation of annual average soil loss using the Revised Universal Soil Loss Equation (RUSLE) integrated in a Geographical Information System (GIS) of the Esil River basin (ERB), Kazakhstan. Acta Geophys. 67(3):921–938. doi:10.1007/s11600-019-00288-0.
- Napoli M, Cecchi S, Orlandini S, Mugnai G, Zanchi CA. 2016. Simulation of field-measured soil loss in Mediterranean hilly areas (Chianti, Italy) with RUSLE. Catena. 145:246–256. doi:10.1016/j.catena.2016.06.018.
- Nyesheja EM, Chen X, El-Tantawi AM, Karamage F, Mupenzi C, Nsengiyumva JB. 2019. Soil erosion assessment using RUSLE model in the Congo Nile Ridge region of Rwanda. Phys Geogr. 40(4):339–360. doi:10.1080/02723646.2018.1541706.
- Pal S. 2016. Identification of SE vulnerable areas in Chandrabhaga river basin: a multi-criteria decision approach. Model Earth Syst Environ. 2(5):1–11. doi:10.1007/s40808-015-0052-z.
- Pandey A, Gautam AK, Chowdary VM, Jha CS, Cerdà A. 2021. Uncertainty assessment in soil erosion modelling using RUSLE, multisource and multiresolution DEMs. J Indian Soc Remote Sens. 49(7):1689–1707. doi:10.1007/s12524-021-01351-4.
- Prasannakumar V, Vijith H, Abinod S, Geetha NJGF. 2012. Estimation of soil erosion risk within a small mountainous sub-watershed in Kerala, India, using Revised Universal Soil Loss Equation (RUSLE) and geo-information technology. Geosci Front. 3(2):209–215. doi:10.1016/j.gsf.2011.11.003.
- Quesada-Román A, Campos-Durán D. 2022. Natural disaster risk inequalities in Central America. Papers Appl Geogr. 9(1):36–48. doi:10.1080/23754931.2022.2081814.
- Quesada-Román A, Mata-Cambronero E. 2021. The geomorphic landscape of the Barva volcano, Costa Rica. Phys Geogr. 42(3):265–282. doi:10.1080/02723646.2020.1759762.
- Quesada-Román A, Castro-Chacón JP, Boraschi SF. 2021. Geomorphology, land use, and environmental impacts in a densely populated urban catchment of Costa Rica. J South Am Earth Sci. 112:103560. doi:10.1016/j.jsames.2021.103560.
- Rahman MR, Shi ZH, Chongfa C. 2009. SE hazard evaluation—an integrated use of remote sensing, GIS and statistical approaches with biophysical parameters towards management strategies. Ecol Modell. 220(13–14):1724–1734. doi:10.1016/j.ecolmodel.2009.04.004.
- Rajbanshi J, Bhattacharya S. 2020. Assessment of soil erosion, sediment yield and basin specific controlling factors using RUSLE-SDR and PLSR approach in Konar river basin, India. J Hydrol. 587:124935. doi:10.1016/j.jhydrol.2020.124935.
- Renard KG. 1997. Predicting SE by water: a guide to conservation planning with the Revised Universal Soil Loss Equation (RUSLE). Washington, DC: United States Government Printing.
- Renschler CS, Harbor J. 2002. Soil erosion assessment tools from point to regional scales—the role of geomorphologists in land management research and implementation. Geomorphology. 47(2-4):189–209. doi:10.1016/S0169-555X(02)00082-X.
- Samanta RK, Bhunia GS, Shit PK. 2016. Spatial modelling of SE susceptibility mapping in lower basin of Subarnarekha river (India) based on geospatial techniques. Model Earth Syst Environ. 2(99):1–13. doi:10.1007/s40808-016-0170-2.
- Schumm SA. 1956. Evolution of drainage systems and slopes in Badlands at Perth Amboy, New Jersey. Geol Soc America Bull. 67(5):597. doi:10.1130/0016-7606(1956)67[597:EODSAS]2.0.CO;2.
- Senanayake S, Pradhan B, Huete A, Brennan J. 2022. Spatial modeling of SE hazards and crop diversity change with rainfall variation in the Central Highlands of Sri Lanka. Sci Total Environ. 806(Pt 2):150405. doi:10.1016/j.scitotenv.2021.150405.
- Shamshad A, Azhari MN, Isa MA, Hussin WW, Parida BP. 2008. Development of an appropriate procedure for estimation of RUSLE EI30 index and preparation of erosivity maps for Pulau Penang in Peninsular Malaysia. Catena. 72(3):423–432. doi:10.1016/j.catena.2007.08.002.
- Shivhare N, Rahul AK, Omar PJ, Chauhan MS, Gaur S, Dikshit PKS, Dwivedi SB. 2018. Identification of critical SE prone areas and prioritization of micro-watersheds using geoinformatics techniques. Ecol Eng. 121:26–34. doi:10.1016/j.ecoleng.2017.09.004.
- Singh G, Panda RK. 2017. Grid-cell based assessment of SE potential for identification of critical erosion prone areas using USLE, GIS and remote sensing: a case study in the Kapgari watershed, India. Int Soil Water Conserv Res. 5(3):202–211. doi:10.1016/j.iswcr.2017.05.006.
- Singh G, Chandra S, Babu R. 1981. Soil loss and prediction research in India. New York: Central Soil and Water Conservation Research Training Institute
- Smith KG. 1950. Standards for grading texture of erosional topography. Am J Sci. 248(9):655–668. doi:10.2475/ajs.248.9.655.
- Sofi MS, Rautela KS, Bhat SU, Rashid I, Kuniyal JC. 2021. Application of geomorphometric approach for the estimation of hydro-sedimentological flows and cation weathering rate: towards understanding the sustainable land use policy for the Sindh Basin, Kashmir Himalaya. Water Air Soil Pollut. 232(7):280. doi:10.1007/s11270-021-05217-w.
- Strahler A. 1952. Hypsometric (area-altitude) analysis of erosional topography. Geol Soc America Bull. 63(11):1117–1142. doi:10.1130/0016-7606(1952)63[1117:HAAOET]2.0.CO;2.
- Thomas J, Joseph S, Thrivikramji KP. 2018. Estimation of SE in a rain shadow river basin in the southern Western Ghats, India using RUSLE and transport limited sediment delivery function. Int Soil Water Conserv Res. 6(2):111–122. doi:10.1016/j.iswcr.2017.12.001.
- USGS. 2022. Earth Explorer, Science for a Changing World, United States Department of the Interior, USA. [accessed 10 Jun 2022]. https://earthexplorer.usgs.gov/.
- Van der Knijff JM, Jones RJA, Montanarella L. 2000. SE risk: assessment in Europe. Belgium: European Commission.
- Van Pelt RS, Hushmurodov SX, Baumhardt RL, Chappell A, Nearing MA, Polyakov VO, Strack JE. 2017. The reduction of partitioned wind and water erosion by conservation agriculture. Catena. 148:160–167. doi:10.1016/j.catena.2016.07.004.
- Vigiak O, Borselli L, Newham LTH, McInnes J, Roberts AM. 2012. Comparison of conceptual landscape metrics to define hillslope-scale sediment delivery ratio. Geomorphology. 138(1):74–88. doi:10.1016/j.geomorph.2011.08.026.
- Wischmeier WH, Smith DD. 1978. Predicting rainfall erosion losses: a guide to conservation planning (No. 537). Maryland: United States Department of Agriculture.
- Zerihun M, Mohammedyasin MS, Sewnet D, Adem AA, Lakew M. 2018. Assessment of SE using RUSLE, GIS and remote sensing in NW Ethiopia. Geoderma Regional. 12:83–90. doi:10.1016/j.geodrs.2018.01.002.
- Zhang S, Fan W, Li Y, Yi Y. 2017. The influence of changes in land use and landscape patterns on SE in a watershed. Sci Total Environ. 574:34–45. doi:10.1016/j.scitotenv.2016.09.024.
- Zhang S, Lu XX, Sun H, Han J. 2011. Modeling catchment controls on organic carbon fluxes in a meso-scale mountainous river (Luodingjiang), China. Quat Int. 244(2):296–303. doi:10.1016/j.quaint.2010.11.008.
- Zhou M, Deng J, Lin Y, Belete M, Wang K, Comber A, Huang L, Gan M. 2019. Identifying the effects of land use change on sediment export: integrating sediment source and sediment delivery in the Qiantang River Basin, China. Sci Total Environ. 686:38–49. doi:10.1016/j.scitotenv.2019.05.336.
- Zhou P, Luukkanen O, Tokola T, Nieminen J. 2008. Effect of vegetation cover on soil erosion in a mountainous watershed. Catena. 75(3):319–325. doi:10.1016/j.catena.2008.07.010.