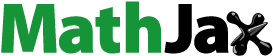
Abstract
The study of dynamic changes in vegetation coverage has significant implications for urban clusters in terms of ecological environment protection, climate change research, water resource management and urban planning. A thorough understanding of the driving mechanisms behind vegetation coverage helps provide scientific basis and policy recommendations for the ecological environment protection, sustainable development and decision-making of urban clusters. Taking the central Yunnan urban cluster as an example, this study utilizes long-term Landsat remote sensing image data from 2000 to 2020 on the Google Earth Engine platform. The fractional vegetation cover (FVC) is estimated using a pixel-based binary model. The Sen’s slope, Mann–Kendall trend analysis and Hurst index methods are employed to investigate the spatiotemporal dynamic characteristics of FVC. The impacts of climate change and human activities on vegetation coverage are explored through partial correlation analysis and residual analysis. Finally, considering natural factors such as topography and climate, as well as socioeconomic factors, the geographic detector is used to quantitatively analyze the driving factors behind FVC changes. The results indicate that: (1) From 2000 to 2020, the FVC in the central Yunnan urban cluster changed significantly, showing an overall improvement trend. The average FVC is 0.496 with a growth rate of 0.0024 per year, and it exhibits a distribution pattern of higher values in the west and lower values in the east. (2) In terms of percentage distribution, areas with low FVC and high FVC account for a relatively high proportion. The trend of FVC changes is as follows: improvement (49.6%) > degradation (26.4%) > no change (24%). The average Hurst value is 0.45, indicating that future FVC changes in the study area will be opposite to the past. (3) FVC shows a positive correlation with precipitation and a negative correlation with temperature. Human activities have a positive impact on FVC in the study area, accounting for 55.1% of the regional proportion. (4) Slope and nighttime light contribute the most to FVC. The explanatory power of the interaction between slope and precipitation/temperature is the most significant.
1. Introduction
Fractional vegetation cover (FVC) refers to the extent or proportion of an area or spatial scope covered by vegetation objects, serving as a descriptor for vegetation distribution and density within a specific area. Typically, it is represented as the ratio between the vertical projection area of the vegetation canopy onto the ground and the total land area (Gan et al. Citation2011). FVC serves as a reliable indicator reflecting vegetation growth conditions and biomass, holding significance as a parameter for assessing surface vegetation (Gu et al. Citation2020); it stands as a vital metric for analyzing regional environmental shifts, socio-economic development and the advancement of ecological civilization. Moreover, it constitutes a crucial component in the evaluation of soil and water conservation (Zhang and Liang Citation1996), soil erosion (Zhang et al. Citation2011), land desertification (Fu Citation2020), atmospheric composition, climate change, ecological environment, etc. Given the current precarious state of the global ecological environment, compounded by climate upheavals and intensified human activities, studying vegetation cover assumes paramount importance in ecological preservation, water resource management, soil conservation, climate change appraisal and agricultural productivity. This research not only aids in comprehending the spatial distribution and evolving trends of vegetation cover, as well as the health of ecosystems but also forms the groundwork for decision-making processes and sustainable development across related domains.
FVC monitoring predominantly relies on remote sensing technology, ground observation and field surveys. Among these methods, remote sensing stands out as a comprehensive approach (Pei et al. Citation2018), offering real-time, precise data, wide coverage, strong continuity and thoroughness (Liu et al. Citation2021). This technology has found extensive application in monitoring surface FVC across diverse spatial and temporal scales (Jia et al. Citation2013; Van De Voorde et al. Citation2008; Zhang et al. Citation2013). The continuous advancements in remote sensing big data technology have enabled the swift acquisition of high-quality, large-scale, long time-series remote sensing images through platforms like Google Earth Engine’s cloud platform. These datasets facilitate in-depth studies of FVC and enable large-scale remote sensing data analysis. Key remote sensing indices, such as the Normalized Difference Vegetation Index (Konatowska et al. Citation2023)(NDVI), are commonly utilized for monitoring vegetation cover. NDVI, renowned for estimating and tracking vegetation cover, provides insights into photosynthetic activity and vegetation growth status. While sensitive to changes in vegetation cover stability, NDVI exhibits limitations in distinguishing between high and low cover, sometimes failing to accurately estimate extreme vegetation cover or reflect its precise status. Enhanced Vegetation Index (Nepita-Villanueva et al. Citation2019)(EVI) emerges as an alternative remote sensing index for evaluating vegetation cover and productivity. It excels in areas with low vegetation cover, including non-vegetated terrains like bare soil and rocks. EVI boasts a superior dynamic range and differentiation ability compared to NDVI. Unlike NDVI, EVI's performance remains less saturated in heavily vegetated regions, offering a more accurate estimation of vegetation cover. However, computing EVI necessitates specific band data from remote sensing images, EVI may be limited when specific band data or multispectral band data are unavailable, potentially affecting the accuracy of vegetation change analysis by extracting pixels akin to vegetation’s spectral characteristics (Yang et al. Citation2020). Fraction of Vegetation Cover (FVC), another crucial index for assessing vegetation status and ecological conditions, is compared with NDVI and EVI. FVC, estimated via the image element dichotomous method, exhibits a linear relationship with vegetation productivity. This index accurately estimates vegetation cover, effectively portrays surface vegetation growth (Liu et al. Citation2021;Zhang et al. Citation2016), and facilitates continuous monitoring across extensive geographical areas and extended time-series within remote sensing images (Chen et al. Citation2021).
Currently, many methods are employed to study the spatiotemporal evolution of vegetation characteristics. The Theil-Sen slope estimate (Sen Citation1968), Mann-Kendall trend analysis (Mann Citation1945; Kendall Citation1975) and simple linear regression (Krieger et al. Citation2003) have been widely used for long-term vegetation change monitoring. LandTrendr (Kennedy et al. Citation2010), DBEST (Jamali et al. Citation2015) and Hurst index (Koutsoyiannis Citation2003) are utilized to detect whether long time series vegetation changes are continuous. Residual analysis (Anscombe and Tukey Citation1963) and partial correlation analysis (Lawrance Citation1976) quantitatively analyze the impact of influencing factors on the spatial variation of vegetation coverage. Wang and Xu (Citation2017) proposed the Geo-detector model in 2017. This model does not require a linear assumption and can reveal the impact of influencing factors and the synergistic effects between different factors on the spatial distribution of factors.
The central Yunnan urban agglomeration is located in the southwestern part of China, with unique geographical and climatic characteristics and a diverse range of vegetation types. As one of the regions with the most diverse existing vegetation types in China, providing scientific basis for effective resource management and ecological environment protection of vegetation resources has been a focus of attention for scholars both domestically and internationally. For example, research by He et al. (He et al. Citation2018) explored the relationship between vegetation in Yunnan and climatic factors, indicating that regions heavily influenced by precipitation are mainly distributed in the central part of Yunnan Province. Feng and Dong (Citation2022) investigated the spatiotemporal changes of FVC in Yunnan Province in relation to topographical effects, revealing an overall improvement trend of FVC, which is significantly negatively correlated with elevation and slope. Fan et al. (Fan et al. Citation2023) studied the impact of human and climate factors on vegetation changes in Yunnan Province, highlighting temperature and precipitation as important factors influencing vegetation changes. Li et al. (Li et al. Citation2024) explored the spatiotemporal changes of FVC in the central Yunnan urban agglomeration under the influence of climate change and human activities, indicating that nighttime light have become one of the major factors influencing FVC since 2010. Research by Peng et al. (Citation2021) and Li and Liu (Citation2023) provided evidence that the FVC of the central Yunnan urban agglomeration has shown an overall upward trend. Chen et al. (Chen et al. Citation2023) discussed the spatiotemporal change patterns of vegetation in Yunnan Province and the influence of climatic factors, revealing a positive correlation between precipitation and FVC. From the existing research, it can be observed that over the past 20 years, the rapid urbanization development of the central Yunnan urban agglomeration has been significant (Pan et al. Citation2023). However, there is still a relatively limited amount of long-term remote sensing monitoring studies on the changes in vegetation coverage in the central Yunnan urban agglomeration during this period. Additionally, there is a lack of research on the county-scale spatiotemporal analysis of vegetation changes and their driving factors in the central Yunnan urban agglomeration. Therefore, leveraging the extensive remote sensing data and computational capabilities provided by the Google Earth Engine remote sensing cloud platform, we utilized Landsat series images as the primary data source. Estimation of FVC was conducted using the pixel bisection model. Subsequently, a quantitative analysis of the temporal and spatial variations in FVC within the central Yunnan urban agglomeration from 2000 to 2020 was carried out. This analysis encompassed Sen’s slope, MK trend analysis, the Hurst index, partial correlation analysis, residual analysis and geo-detector method. The objective was to explore the response characteristics of FVC to climate change and human activities, as well as to dissect the driving forces underlying FVC changes, understanding the evolutionary patterns of FVC in both temporal and spatial dimensions within this study area, along with gaining profound insights into the causes and mechanisms driving vegetation changes, holds immense significance. These findings serve as crucial scientific evidence, providing a basis for fields such as ecological preservation, disaster management, climate change and sustainable development. They further contribute to propelling the central Yunnan urban agglomeration towards a green transformation in economic and social development, fostering ecologically friendly advancements.
2. Materials and methods
2.1. Study area
The central Yunnan urban agglomeration is located in the central and eastern part of Yunnan Province, China (Ding et al. Citation2023). It is situated between 100°43′ to 104°49′ east longitude and 23°01′ to 27°04′ north latitude (Yang and Liu Citation2024). As shown in , it comprises seven counties/cities including Kunming, Qujing, Yuxi, Chuxiong Yi Autonomous Prefecture and Honghe Hani and Yi Autonomous Prefecture, totaling 49 counties (cities, districts). This region possesses Yunnan’s most advantageous land resources, with vegetation mainly consisting of broadleaf forests, coniferous forests, grasslands and shrubs. It is one of the most vegetation-rich areas in Yunnan Province and a pioneer in Yunnan’s ecological civilization construction (Zhi et al. Citation2021). In recent years, with the continuous growth in demand for natural resources due to socio-economic development, issues such as vegetation destruction in the central Yunnan urban agglomeration have become increasingly prominent. The rapid development of urbanization and the urgent need for vegetation protection present serious challenges. The ‘Development Plan of central Yunnan urban agglomeration (2019–2035)’ explicitly states that the central Yunnan urban agglomeration is a key support area for building the most beautiful province in China. It emphasizes that a high-quality ecological environment is the core competitiveness of the central Yunnan urban agglomeration. Research on the vegetation coverage of the central Yunnan urban agglomeration and its response to climate change and human activities is of great significance.
2.2. Data sources
This article utilizes LANDSAT data within the Google Earth Engine platform to synthesize FVC (Fraction of Vegetation Cover) remote sensing images employing the annual average synthesis method, ensuring cloud cover remains below 10% and a spatial resolution of 30 meters. Temperature data is derived from ECMWF/ERA5/DAILY, while precipitation data sources from UCSB-CHG/CHIRPS/DAILY. The DEM (Digital Elevation Model) is obtained from the SRTMGL1_003 image data set by USGS, offering a spatial resolution of 30m. For GDP data spanning 2000 to 2015, it is acquired from the Resource and Environment Science and Data Center. However, the GDP data for 2020 is retrieved from the China Kilometer Grid GDP Data set (Chen et al. Citation2022;Xu et al. Citation2023), available at the ‘Yangtze River Delta Science Data Center,’ a subset of the National Earth System Science Data Center and National Science & Technology Infrastructure of China (http://geodata.nnu.edu.cn/). Population data are gathered from the WorldPop data website, and nighttime light data stems from the NPP-VIIRS-like NTL Data set (Chen et al. Citation2021), accessible through the ‘Yangtze River Delta Science Data Center,’ as mentioned previously (http://geodata.nnu.edu.cn/). Refer to for comprehensive details regarding the datasets used in this study.
Table 1. Source and description of research data.
2.3. Methods
2.3.1. Technology roadmap
This article utilizes annual Landsat remote sensing images from 2000 to 2020 in the central Yunnan urban agglomeration through the Google Earth Engine (GEE) platform. The Fraction of Vegetation Cover (FVC) index is obtained using a pixel-based binary model to reflect the vegetation coverage of the central Yunnan urban agglomeration. Spatial analysis methods, including Sen’s slope, Mann–Kendall (MK) trend and Hurst index, were employed to analyze the temporal and spatial trends of vegetation coverage from 2000 to 2020. Future trends were predicted, and residual analysis, correlation analysis and significance tests were conducted on FVC in the years 2000, 2005, 2010, 2015 and 2020, along with corresponding temperature and precipitation data for those years. This meticulous exploration aims to uncover the intricate interplay between human activities and vegetation dynamics. Ultimately, the study embarks on a detailed exploration of the principal driving factors contributing to the spatial differentiation of vegetation coverage, employing the geo-detector method; refer to .
2.3.2. FVC estimation
This article uses the pixel bisection model (Yan et al. Citation2022) to calculate FVC, and the calculation formula is:
(1)
(1)
NDVI of completely bare soil or non-vegetated areas;
NDVI of completely covered by vegetation.
2.3.3. Sen’s slope analysis and Mann-Kendall (M-K) trend test
Sen’s slope (Gocic and Trajkovic Citation2013) analysis is a method for estimating the trend of time series (Liang et al. Citation2023). The calculation formula is as follows:
(2)
(2)
In the formula, is the median function;
and
are the FVC observation values at the ith and jth moments in the time series; when
>0, FVC in the time series shows an increasing trend and vice versa.
The MK (Da Silva et al. Citation2015) test is a rank-based non-parametric (Yue and Wang Citation2004) test, it is often combined with Sen’s slope analysis to determine the significance of changing trends (Wang et al. Citation2022). It performs trend testing through the test statistic Z (Wang et al. Citation2013). The specific calculation method is as follows:
(3)
(3)
In the formula, is the variance of
and the calculation formula of
is:
(4)
(4)
The calculation formula of is:
(5)
(5)
In EquationEq. (4)(4)
(4) , the expression of
is as follows:
(6)
(6)
The method used in this article to judge trend significance is as follows ().
Table 2. Mann–Kendall test trend categories.
2.3.4. Hurst index
The Hurst index is an effective method to quantitatively describe the long-range dependence of time series (Hou et al. Citation2010), the calculation formula is as follows:
For any positive integer define the mean series:
(7)
(7)
The cumulative deviation is:
(8)
(8)
The range is:
(9)
(9)
The standard deviation is:
(10)
(10)
For the ratio if the following relationship
exists. It means that the analyzed time series has the Hurst phenomenon, and H is called the Hurst index.
Diverse Hurst index values, denoted by H (0<H < 1), delineate varying scenarios as follows:
H = 0.5: This signifies complete independence within the FVC data, demonstrating no interdependence between elements and erratic changes in FVC over time.
0.5 < H < 1 indicates long-term correlation within the time series and the process is continuous. In terms of FVC, this implies a consistency between future and past trends. For instance, a historical increasing trend in FVC portends a continuing upward trajectory in the future and vice versa. Moreover, a closer proximity of the H value to 1 signifies heightened sustainability.
0 < H < 0.5 indicates anti-persistence within the time series. Future trends oppose past patterns. Specifically, a historical increase suggests an impending decrease in the future and conversely. Furthermore, a closer proximity of the H value to 0 signifies stronger anti-sustainability tendencies.
2.3.5. Pearson partial correlation analysis
When analyzing the impact of climate factors on FVC, one other variable can be regarded as a constant. Studying the relationship between FVC and precipitation or FVC and temperature separately is called a partial correlation (Sun et al. Citation2015). Use the single correlation coefficient to calculate the Pearson partial correlation coefficient. The calculation formula of the single correlation coefficient is as follows:
(11)
(11)
In the formula, a and b are the average values of the two variables in n years respectively; c is the simple correlation coefficient between the two elements; n is the number of samples.
The calculation formula of the Pearson partial correlation coefficient is as follows:
(12)
(12)
The significance test of the Pearson partial correlation coefficient generally uses the t test method, and its statistical calculation formula is:
(13)
(13)
In the formula, is the partial correlation coefficient; n is the number of samples; m is the number of independent variables (Yue et al. Citation2023).
2.3.6. Residual analysis
Residual (John et al. Citation2016) analysis is one of the most effective methods to determine the impact of human activities on FVC (Guo et al. Citation2023). This article is based on the assumption that the dynamic change of FVC mainly depends on the influence of climate and establishes a multiple regression model of FVC, precipitation and temperature to obtain the prediction value of FVC. The residual is the difference between the measured value and the predicted value of FVC, that is, the impact of human activities on FVC. The specific calculation formula is as follows:
(14)
(14)
(15)
(15)
In the formula, is the annual precipitation data;
is the annual temperature data; a, b, c are the regression coefficients;
is the predicted value of FVC;
is the measured value of FVC;
is the residual value,
indicates that human activities play a positive role;
indicates that human activities play a negative role. The residual value grading standards used in this article are as follows ().
Table 3. Impact categories of human activities on FVC.
2.3.7. Geo-detector
Spatial heterogeneity refers to the geographical phenomenon that the intra-layer variance is smaller than the inter-layer variance. That is, the same geographical phenomenon shows similarity in the same sub-region, but its distribution among different sub-regions shows differences. Geo-detector is a tool for detecting and utilizing spatial variations (Wang and Xu Citation2017). It is commonly used to detect the correlation of geographical phenomena and environmental factors (Wang et al. Citation2010). Factor detection is mainly employed to identify spatial correlation relationships between geographical features and other characteristics. It analyzes the contribution and interaction of different geographical features, determines the differences in geographical features between different regions and provides scientific basis for regional planning and spatial decision-making. Interaction detection of Geo-detector is primarily used to analyze the interaction processes among multiple elements in geographical phenomena. It helps reveal the ways, intensity and direction of interactions between geographical elements, thus providing a deeper understanding of the formation mechanisms and influencing factors of geographical phenomena.
In this article, the determining force q of FVC differentiation introduced by Geo-detector into counties of central Yunnan urban agglomeration was used to detect and analyze the degree of influence of each element on the FVC. q is calculated as:
(16)
(16)
In the formula: h = 1, …, L is the stratification of the independent or dependent variable, that is, categorization or partitioning; and N are the number of cells in layer h and the whole area, respectively;
and
are the variance in layer h and the whole area, respectively. This article selected eight factors: temperature, precipitation, elevation, slope, aspect, GDP, population and nighttime lighting for research on spatial differentiation driving of FVC.
This article utilizes the interaction detection in the geo-detector to thoroughly reveal the interactions and influence patterns among the multiple driving factors of FVC change, determines the characteristics of the interaction between two variables by comparing the q value of a single factor and the q value of a two-factor interaction (Huang et al. Citation2015). The specific interaction types and classification criteria are as follows ().
Table 4. Interaction types and judgment criteria.
3. Results
3.1. Characteristics of spatial and temporal distribution of FVC
In order to reflect the vegetation coverage more intuitively, the vegetation coverage is graded according to the following relevant standards ().
Table 5. FVC classification standard.
As shown in , there exists distinct spatial and temporal heterogeneity in vegetation cover in central Yunnan urban agglomerations from 2000 to 2020. The FVC exhibits uneven spatial distribution characteristics. From a spatial perspective, the FVC in the western region is, on average, higher than that in the eastern region. High FVC is predominantly found in Chuxiong Yi Autonomous Prefecture, Yuxi City, the western part of Kunming City and the southern region of Qujing City, benefiting from favorable water and thermal conditions. The primary land use in these areas is characterized by extensive forestland. Conversely, low FVC is prevalent in regions marked by heightened human activity, including urban zones in Kunming City, Qujing City and most areas within the Honghe Hani and Yi Autonomous Prefecture. The predominant land use types in these locales encompass grassland, cultivated land, construction land and urban areas. Human activities such as urbanization and agricultural cultivation may contribute to vegetation degradation, destruction or removal, thereby altering the spatial distribution of FVC.
To delve deeper into the evolving dynamics of FVC (Fraction of Vegetation Cover) within the central Yunnan urban agglomeration from 2000 to 2020, statistical analyses were conducted on the alterations in the mean FVC values and the distribution of different FVC grades, as depicted in . Over the past two decades, the average FVC of the central Yunnan urban agglomeration is 0.496. showing an overall upward trend with an average annual growth rate of 0.0024a-1, the FVC reached its pinnacle in 2017 at 0.533, while the nadir occurred in 2010 at 0.467.From the statistics of different levels of FVC in , it is apparent that both low and high FVC proportions are relatively substantial. Meanwhile, the proportions of medium-low, medium and medium-high FVC remain relatively steady, with medium-low FVC consistently at 15%–18.9%, medium FVC at 16.4%–18.3% and medium-high FVC at 13.6%–17%. The trend for low FVC indicates reduced volatility, peaking at 31.7% in 2010 and hitting its lowest point at 22.8% in 2017. In contrast, high FVC demonstrates a fluctuating growth pattern, climbing from 21.6% in 2000 to 25.7% in 2020. With the exceptions of 2001, 2003 and 2005, when the proportion of high FVC dipped below 20%, the remaining years all exceeded this threshold. The aforementioned analysis underscores a significant improvement in vegetation quality in the study area over the past two decades.
3.2. FVC trend analysis
Sen’s slope estimation and the Mann-Kendall (M-K) significance test were employed to scrutinize the spatio-temporal variations in FVC (Fraction of Vegetation Cover) within the central Yunnan urban agglomeration from 2000 to 2020, illustrated in . From 2000 to 2020, the FVC in the central Yunnan urban agglomerations of experienced an overall increase, reaching approximately 49.6%, while about 26.4% showed a decline. In specific terms, 25.8% of the areas exhibited a significant increase, while most of the decreasing areas showed an insignificant decrease. Spatially, the regions with extremely significant and significant increases accounted for 13% and 8.5%, respectively, dispersed across various states and cities, This positive trend is attributed to the successful implementation of ecological and environmental protection measures in recent years, such as the rocky desertification control project in the Honghe Hani and Yi Autonomous Prefecture, the establishment of a nature reserve in Qujing City and the execution of ecological restoration projects in Yuxi City. The eastern part of Qujing City, in particular, has experienced a more pronounced increase in vegetation coverage. The areas with extremely significant and significant decrease accounted for 3.8% and 3.6%, respectively, primarily situated in the northern and southern parts of Chuxiong Yi Autonomous Prefecture, the central region of Kunming City and the southeastern part of Honghe Hani and Yi Autonomous Prefecture. Rapid urbanization has adversely affected the vegetation growth conditions in these areas. The proportions of slightly significant increase, no significant increase, no change, no significant decrease and slightly significant decrease were 4.3%, 23.8%, 24%, 17% and 2.1%, respectively, distributed across all states and cities in the study area. The no changed areas typically have sparse vegetation year-round, barren land and pose management challenges, so there is no change. This comprehensive analysis distinctly signifies a general upward trend in vegetation activities within the central Yunnan urban agglomerations over the past two decades.
3.3. Persistence analysis of changes in FVC
Based on the findings from the Hurst index analysis, illustrated in , the maximum value of the Hurst index in the central Yunnan urban agglomeration from 2000 to 2020 is 0.9275, the minimum value is 0.0961, with an average value of 0.45. 72.5% of the areas exhibit a Hurst index below 0.5, indicating anti-sustainable vegetation changes – an increase in the past suggests a general decrease in the future and vice versa, it is widely distributed in space; 27.5% of the areas have a Hurst index greater than 0.5, signaling weak persistence in FVC and suggesting a degradation trend in future changes. The distribution of these areas is scattered, with a concentration in the western part of the study area. This outcome may be attributed to irregular climate characteristics in different years or seasons, intense human activities such as urban expansion and land use changes, and the intrinsic instability and dynamics of certain ecosystems. In summary, the analysis indicates that a significant proportion of regions will experience contrasting trends in FVC changes in the future compared to past changes.
3.4. The relationship between FVC and climate factors
3.4.1. Pearson correlation analysis between FVC and precipitation
Using Pearson correlation analysis, the significance of the correlation between precipitation and vegetation coverage was tested. Precipitation is a crucial factor for vegetation growth, playing a vital role in maintaining vegetation coverage. As shown in , the region with a positive correlation between FVC and precipitation from 2000 to 2020 accounts for 54.8%, primarily distributed in the eastern part of the study area. The region with a significantly positive correlation covers 2.9%, including the southwest and northwest of Chuxiong Yi Autonomous Prefecture, the southern part of Kunming City, the central part of Qujing City, the eastern and southwest parts of Yuxi City, and the central part of Honghe Hani and Yi Autonomous Prefecture. This may be attributed to increased precipitation, enhanced vegetation water use efficiency, and promoted vegetation growth. The area with a negative correlation in the Central Yunnan urban agglomeration constitutes 40.1%, mainly distributed in the eastern and central parts of Chuxiong Yi Autonomous Prefecture, the western part of Yuxi City, the central and southwest parts of Qujing City, and the northeastern part of Honghe Hani and Yi Autonomous Prefecture. The significantly negative correlation region covers 2.2%, distributed in the central, southeastern and eastern parts of Chuxiong Yi Autonomous Prefecture, the southern part of Kunming City, the northern and southwest parts of Yuxi City, the central and southwest parts of Qujing City. The reasons may be the steep slopes and concentrated, intense rainfall in these areas, making them prone to soil erosion.
3.4.2. Pearson correlation analysis between FVC and temperature
There is a close association between vegetation coverage and temperature, with temperature influencing the entire process of vegetation growth and development. As depicted in , the region with a positive correlation between FVC and temperature in the central Yunnan urban agglomeration from 2000 to 2020 accounts for 40.8%, primarily distributed in the northeastern and northern parts of Qujing City, the southwestern and northeastern parts of Kunming City, the western part of Chuxiong Yi Autonomous Prefecture and the northern part of Yuxi City. The significantly positive correlation region covers 1.9%, including the western part of Chuxiong Yi Autonomous Prefecture, the southwestern and northeastern parts of Kunming City, the northeastern and southwestern parts of Qujing City, the northern and southwestern parts of Yuxi City and the southern part of Honghe Hani and Yi Autonomous Prefecture. These areas have a suitable temperature, favorable for vegetation growth, promoting an increase in FVC. The region with a negative correlation covers 54.1%, mainly distributed in the southern part of Qujing City, the northwestern and southeastern parts of Kunming City, the northern and southern parts of Chuxiong Yi Autonomous Prefecture, the southern part of Yuxi City and most areas of Honghe Hani and Yi Autonomous Prefecture. The significantly negative correlation region covers 3.2%, including the central and southern parts of Chuxiong Yi Autonomous Prefecture, the central and southeastern parts of Kunming City, the southwestern part of Yuxi City, the southern part of Qujing City and the southern part of Honghe Hani and Yi Autonomous Prefecture. The reasons may be that these regions have relatively high temperatures, and an increase in temperature will increase surface evapotranspiration, which is not conducive to vegetation absorbing water. Excessively high temperatures will reduce available water for vegetation and impact vegetation growth.
3.5. Impact of human activities on FVC
sheds light on the impact of human activities on FVC (Fraction of Vegetation Cover) within the central Yunnan urban agglomeration during the period spanning 2000 to 2020. The proportion of regions depicting an improvement due to human activities amounts to 55.1%. Within this category, regions showcasing significant improvement and slight improvement constitute 42.0% and 13.1%, respectively. These areas are notably distributed across the eastern and southern sectors of Qujing City, the central and southwestern precincts of Kunming City, the western, central and northern expanses of Yuxi City, the northern and northeastern reaches of Honghe Hani and Yi Autonomous Prefecture and the southwestern and eastern territories of Chuxiong Yi Autonomous Prefecture. Conversely, areas displaying stable account for 6.5% of this category, mainly encompassing lakes and rivers. The portion of areas where human activities have caused a detrimental impact on FVC accounts for 38.4%. Regions experiencing significant deterioration and slight deterioration represent 26.3% and 12.1%, respectively. These areas are predominantly situated in the northern sector of Qujing City, the northern and central parts of Chuxiong Yi Autonomous Prefecture, the northwestern zone of Yuxi City, the southeastern sector of Kunming City, and the southern, eastern and central precincts of Honghe Hani and Yi Autonomous Prefecture.
Human activities exert a dual impact on FVC, creating a complex interplay. On one hand, activities such as land cultivation expansion and urbanization tend to disrupt FVC. Conversely, practices such as afforestation and ecological initiatives contribute to enhancing vegetation levels. Within this study, a residual value >0 signifies a positive role of human activities, while a residual value <0 indicates a negative impact. illustrates that in 2000, 2015 and 2020, the areas where human activities positively influenced FVC outweighed those where they had a negative impact. However, in 2005 and 2010, the scenario was reversed, with the areas depicting a negative effect from human activities surpassing those exhibiting a positive impact on FVC. Through residual analysis, we can pinpoint regions where human activities detrimentally affected FVC, providing valuable insights crucial for environmental considerations within the central Yunnan urban agglomerations. While residual analysis offers a directional understanding of areas impacted by human-induced damage to FVC, it’s essential to note that comprehensive comprehension regarding the underlying causes of ecosystem impairment demands further on-site investigations and research initiatives, these endeavors are pivotal for developing a holistic understanding of FVC ramifications of human activities and formulating targeted vegetation conservation strategies within the central Yunnan urban agglomerations.
3.6. Analysis of driving factors of FVC
3.6.1. Factor influence detection
The factor influence detection results depicted in unveil the pivotal factors shaping the spatial distribution of FVC across different years. In the year 2000, key influential factors included slope and nighttime light, other factors ranked in descending order of q-values: temperature, elevation, slope, GDP, precipitation and population. Moving to 2005, the foremost driving forces impacting the spatial distribution of FVC were precipitation and temperature. The remarkably high q-value of precipitation underscored its paramount importance in limiting FVC during that year. By 2010, nighttime light emerged as the predominant factor dictating the spatial distribution of FVC, evident from its remarkably high q-value of 0.297. Factors like slope , nighttime light and aspect exerted a secondary influence on FVC during this period. In 2015, nighttime light maintained its prime influence on FVC distribution, evidenced by a q-value of 0.361, the impact of slope comes second, with a q value of 0.29. Finally, by 2020, factors including slope, elevation and nighttime light emerged as the primary influencers on the spatial distribution of FVC, population exhibited the least influence, denoted by a q-value of 0.02. Throughout the 2000–2020 period, the spatial distribution of FVC bore the imprint of various influencing factors. Slope notably wielded the most pronounced impact on FVC distribution, closely trailed by nighttime light, while the impact of other factors fluctuated over time.
3.6.2. Factor interaction detection analysis
The interaction detection method has unveiled significant interconnectedness among the driving factors influencing FVC changes. As depicted in , an evident interplay among the impacting factors reflects a discernible interactive effect on FVC, outlined in through terms like ‘enhance,bi-’ and ‘enhance,nonlinear’, within this study, the ‘enhance,nonlinear’ effect surpasses the ‘enhance,bi-’ impact. The study found that the interaction factors that play a leading role in FVC in the central Yunnan urban agglomeration are mainly temperature ∩ nighttime light, temperature ∩ slope, precipitation ∩ slope and show ‘enhance,nonlinear’, indicating that the explanatory power of the interactions between precipitation and slope, temperature and nighttime light, as well as slope, is greater than the sum of their individual explanatory powers; the double interaction of slope ∩ nighttime light shows ‘enhance,bi-’, indicating that the explanatory power of the interaction of slope and nighttime light is greater than the explanatory power of each single factor; aspect ∩ nighttime light, population ∩ nighttime light, slope ∩ GDP, elevation ∩ GDP, elevation ∩ nighttime light, slope ∩ population, GDP ∩ population, GDP ∩ nighttime light, elevation ∩ population, temperature ∩ precipitation, slope ∩ aspect, aspect ∩GDP shows ‘enhance,bi-’ and ‘enhance,nonlinear’ after pairwise interaction; the remaining factors all show ‘enhance,nonlinear’ after pairwise interaction. The influences of diverse factors on FVC in the central yunnan urban agglomeration are interrelated and operate collaboratively. The amalgamation of topographic and climatic factors exerts a more pronounced impact on FVC. After the interaction between natural and human activity factors, the impact has been significantly enhanced.
Table 6. Ranking of interaction influence by year.
4. Discussions
4.1. Changes in spatial and temporal patterns of FVC
The overall FVC within the central Yunnan urban agglomeration demonstrated a consistent upward trend from 2000 to 2020, aligning with existing research findings (Qiu Citation2022; Feng and Dong, Citation2022; Xiong et al. Citation2018). During this period, the average FVC reached 0.496. Notably, the proportions of low, medium-low and medium FVC steadily declined, while the ratios of high and medium-high FVC consistently increased. This trend primarily correlates with the area’s subtropical monsoon climate, conducive to vegetation growth and the successful implementation of measures such as afforestation, the ‘Grain for Green’ program and robust ecosystem protection initiatives. The spatial distribution of FVC across the central Yunnan urban agglomeration reveals a pattern of high FVC in the western region and lower FVC in the eastern region. The western part is distinguished by natural reserves like Ailao Mountain, characterized by lofty mountains, dense forests and abundant vegetation. Conversely, the eastern region features a karst landscape (Xu et al. Citation2023), marked by slow soil formation rates and limited soil water-holding capacity (Deng et al. Citation2020), resulting in lower FVC. The analysis of FVC trends highlights that 49.6% of the area in the central Yunnan urban agglomeration experienced increased FVC. Notably, vegetation in eastern Qujing City exhibited a substantial improvement in FVC due to key projects like natural forest protection, rocky desertification control and public welfare forest replanting. However, certain areas, including the northern and southern sections of Chuxiong Yi Autonomous Prefecture, the central part of Kunming City, and the southeastern part of Honghe Hani and Yi Autonomous Prefecture, witnessed decreased FVC due to urban expansion and ecological disruptions. Hurst analysis indicated that approximately 72.5% of the pixels within the central Yunnan urban agglomeration exhibited indices ranging from 0 to 0.5, this provides evidence that the future changes in FVC in these areas will exhibit an opposite trend to that observed in the past 20 years.
4.2. Impact of climate change and human activities on changes in FVC
The correlation analysis between the FVC and average annual temperature, as well as annual precipitation in the central Yunnan urban agglomeration spanning from 2000 to 2020, underscores a substantial relationship between FVC and these climatic factors. A positive correlation emerges between FVC and precipitation, aligning with existing research findings (Xu and Liu Citation2022; Chen et al. Citation2023), its pivotal role as the primary water source for vegetation growth, has a delayed impact on vegetation growth. Conversely, an overall negative correlation surfaces between FVC and temperature. This association can be attributed to the central Yunnan urban agglomeration’s location in the southwest plateau mountainous region, characterized by relatively high average temperatures. Scholarly research has consistently revealed that heightened temperatures tend to accelerate plant transpiration, elevate evaporation rates and inhibit photosynthesis (Zuidema et al. Citation2022). These temperature-induced effects also reduce soil moisture content and can potentially lead to soil desiccation, impeding plant growth (Liang et al. Citation2022). The findings of this study validate that temperature and precipitation stand as the primary climate influencers shaping the spatial distribution and dynamic alterations in FVC within central Yunnan urban agglomerations.
Residual analysis of FVC within the central Yunnan urban agglomeration spanning from 2000 to 2020 reveals relationship between human activities and FVC dynamics, human activities exhibited a positive influence on FVC in 2000, 2015 and 2020. However, adverse impacts were observed in 2005 and 2010, potentially attributed to numerous highland lakes within the area, the consequences of engineering projects, urban expansion, mineral extraction and intensified human activities have disrupted the environment essential for vegetation growth. The proportional area affected by human activities on FVC in the central Yunnan urban agglomeration is 55.1% (improved) >38.4% (degraded) >6.5% (unchanged). Contextualizing these findings within regional development, the factors contributing to vegetation degradation encompass improved living standards, population growth, urban expansion and the limited expanse of native vegetation; the factors leading to the enhancement of vegetation encompass establishing natural ecological reserves, implementing ecological protection policies such as returning farmland to forests and grasslands, continual artificial grass planting and intensified afforestation efforts. These measures have notably expanded vegetation cover area and consistently enhanced its quality.
Over the period from 2000 to 2020, the analysis on influential factors underscores that slope stands as the primary driver significantly impacting FVC within the central Yunnan urban agglomeration. Nevertheless, it’s essential not to overlook the enduring shifts in other variables such as temperature, nighttime light, among others, as these factors also wield substantial influence on FVC. Regarding factor interactions, the outcomes following pairwise interactions revealed ‘enhance,nonlinear’ and ‘enhance,bi-’, signifying that the interaction positively strengthened the driving force of the single factor on the spatial and temporal evolution of FVC. In particular, interactions involving slope demonstrate the most robust effects, notably the interactive impact of slope with temperature and precipitation. These interactions denote that, against the backdrop of slope, climate factors such as precipitation and temperature play a crucial role in dictating the growth and development of vegetation within the central Yunnan urban agglomeration.
Therefore, when implementing initiatives for the preservation of vegetation and eco-environment in the central Yunnan urban agglomeration, it is essential to tailor appropriate protective policies in accordance with the diverse topography, climatic variations and human activity intensities across distinct zones. For instance, as elevation rises, human activities tend to diminish, leading to more pronounced vertical climate distinctions, which favor the proliferation and maturation of vegetation. This situation allows for the establishment of ecological protection zones and an expansion of vegetation cultivation. Conversely, regions characterized by low annual precipitation levels might face exacerbated water resource depletion due to excessive tree planting, potentially causing water scarcity. In such cases, large-scale ecological management would prove inappropriate. Furthermore, in areas grappling with severe vegetation degradation, concentrated efforts should concentrate on bolstering management practices and reinstating vegetation coverage. Moreover, fostering public awareness regarding ecological environmental protection becomes pivotal in curbing the adverse impacts of human activities on vegetation cover. In conclusion, the urban agglomeration in central Yunnan ought to optimize diverse ecological engineering measures, considering the nuanced interplay between natural conditions and human activities in distinct localities. Continuous carry out natural resource surveys and ecological tests to support and promote the scientific use of natural resources, thereby advancing the pursuit of sustainable development.
4.3. Disadvantages and prospects
This study conducts a quantitative analysis of the spatial and temporal dynamics of FVC within the central Yunnan urban agglomeration. It comprehensively investigates the interplay among temperature, precipitation and FVC, delves into the impact of human activities on FVC and scrutinizes the underlying driving factors influencing FVC dynamics, but several limitations are worth noting. First, while this study employed Sen’s slope analysis and the Mann–Kendall (M–K) trend test and analyzed the Hurst index separately, the Hurst analysis results yielded relatively weak results. Future research could enrich the study outcomes by integrating Sen’s, Mann–Kendall and Hurst methods for a more comprehensive analysis. Secondly, although the study focused on temperature and precipitation as primary climatic factors, it is important to note that climatic factors encompass a broader spectrum, including elements like wind speed, sunshine duration, solar radiation (Sun et al. Citation2023), among others. Future research can expand the analysis by incorporating additional climate factors, providing a more nuanced understanding of FVC changes within the study area. Lastly, the study utilized county-level administrative regions as the primary research unit for conducting the driving force analysis. However, refining the research scale by employing smaller units such as towns might offer enhanced insights into understanding the driving forces behind FVC changes within the region.
5. Conclusion
This research leveraged remote sensing data spanning from 2000 to 2020 to meticulously examine the spatial and temporal dynamics of FVC within the central Yunnan urban conglomeration. Furthermore, it delved into understanding the intricate interplay between FVC and variables such as climate, topography and human activities. The principal conclusions from this study are outlined below:
Between 2000 and 2020, the mean FVC value within the central Yunnan urban agglomeration stood at 0.496. The spatial distribution predominantly exhibits a pattern of high in the western regions and low in the eastern areas. There’s a discernible upward trend over time, marked by an increase at a rate of 0.0024 per annum. Increasing in the proportion of regions characterized by high and medium-high FVC, while witnessing a decline in the proportion of areas featuring medium, medium-low and low FVC values.
The FVC within the central Yunnan urban agglomeration demonstrates an overarching positive trend, marked by expanded coverage in 49.6% of the total area. Additionally, approximately 26.4% of regional vegetation indicates signs of degradation, while 24% of the regional FVC remains unchanged. The average Hurst value for FVC in this area stands at 0.45, about 72.5% of the pixels across the region exhibit anti-persistent changes in vegetation.
Within the central Yunnan urban agglomeration, the FVC displays a positive correlation with precipitation and an inverse relationship with temperature. Around 57.7% of the total area exhibits a positive correlation between FVC and precipitation, while 42.3% of the area manifests a negative correlation. Areas demonstrating positive and negative correlations between FVC and temperature constitute 42.7% and 57.3% of the study area respectively.
Over the past two decades, human activities have significantly influenced the Vegetation Cover (FVC) within the central Yunnan urban agglomeration, resulting in an overall improvement. Specifically, areas showcasing improved areas (55.1%) > damaged areas (38.4%) > unchanged areas (6.5%). In 2000, 2015 and 2020, the areas where human activities positively affected FVC in the central Yunnan urban agglomeration outnumbered those where negative impacts were observed. Human activities notably propelled vegetation growth. However, during 2005 and 2010, the converse was evident: areas negatively impacted by human activities exceeded those positively affected, human activities resulting in an adverse effect on the vegetation growth of the central Yunnan urban agglomeration.
The geo-detector’s factor analysis revealed that slope significantly impacts the spatial distribution of FVC within the central Yunnan urban agglomeration, followed closely by nighttime light. The primary influencing factors underwent changes over time. Moreover, the interaction detector identified two distinct types of interactions each factor had on FVC changes in this region: ‘enhance,bi-’ and ‘enhance,nonlinear’. There were no isolated interaction factors or elements that exhibited diminished explanatory power for FVC alterations. Among these interactions, slope and temperature, precipitation provided the most robust explanatory insights into FVC changes.
Disclosure statement
There is no potential conflict of interest.
Additional information
Funding
References
- Anscombe FJ, Tukey JW. 1963. The examination and analysis of residuals. Technometrics. 5(2):141–160. doi: 10.1080/00401706.1963.10490071.
- Chen H, Guo Z, He P. 2021. Spatial and temporal change characteristics of vegetation coverage in Erhai Lake basin during 1988—2018. Rem Ote Sens Land Res. 33(2):116–123. doi: 10.6046/gtzyyg.2020283.
- Chen C, Wang JX, Gan S, Yuan XP, Luo WD. 2023. Spatial-temporal change patterns of vegetation and the influence of climatic factors in Yunnan Province, China from 2000 TO 2020. Appl Ecol Env Res. 21(6):5265–5283. doi: 10.15666/aeer/2106_52655283.
- Chen Y, Wu G, Ge Y, Xu Z. 2022. Mapping gridded gross domestic product distribution of China using deep learning with multiple geospatial big data. IEEE J Sel Top Appl Earth Observations Remote Sens. 15:1791–1802. doi: 10.1109/JSTARS.2022.3148448.
- Chen Z, Yu B, Yang C, Zhou Y, Yao S, Qian X, Wang C, Wu B, Wu J. 2021. An extended time series (2000–2018) of global NPP-VIIRS-like nighttime light data from a cross-sensor calibration. Earth Syst Sci Data. 13(3):889–906. doi: 10.5194/essd-13-889-2021.
- Da Silva RM, Santos CAG, Moreira M, Corte-Real J, Silva VCL, Medeiros IC. 2015. Rainfall and river flow trends using Mann–Kendall and Sen’s slope estimator statistical tests in the Cobres River basin. Nat Hazards. 77(2):1205–1221. doi: 10.1007/s11069-015-1644-7.
- Deng Y, Wang S, Bai X, Luo G, Wu L, Chen F, Wang J, Li Q, Li C, Yang Y, et al. 2020. Spatiotemporal dynamics of soil moisture in the karst areas of China based on reanalysis and observations data. J Hydrol. 585:124744. doi: 10.1016/j.jhydrol.2020.124744.
- Ding X, Feng J, Huang Y, Shi J, Wang J. 2023. Dynamic monitoring of ecological environmental quality and spatial pattern evolution of urban agglomeration in Central Yunnan from 2000 to 2020. Bull Soil Water Conserv. 43(3):96–104. doi: 10.13961/j.cnki.stbctb.2023.03.013.
- Fan F, Xiao C, Feng Z, Yang Y. 2023. Impact of human and climate factors on vegetation changes in mainland Southeast Asia and Yunnan province of China. J Cleaner Prod. 415:137690. doi: 10.1016/j.jclepro.2023.137690.
- Feng B, Dong Y. 2022. Spatial-temporal changes of vegetation coverage and topographic effect in Yunnan Province from 2010 to 2020. J Zhejiang for Sci Technol. 42(3):23–32. doi: 10.3969/j.issn.1001-3776.2022.03.004.
- Fu J. 2020. Coordinating management of the eco-environmental systems in the Yellow River Basin. J Irrigation Drainage. 39(10):130–138. doi: 10.13522/j.cnki.ggps.2020326.
- Gan C, Wang X, Li B, Liang Z, Li Z, Wen X. 2011. Changes of Vegetation Coverage During Recent 18 Years in Lianjiang River Watershed. Scientia Geographica Sinica. 31(8):1019–1024. doi: 10.13249/j.cnki.sgs.2011.08.013.
- Gocic M, Trajkovic S. 2013. Analysis of changes in meteorological variables using Mann-Kendall and Sen’s slope estimator statistical tests in Serbia. Global Planet Change. 100:172–182. doi: 10.1016/j.gloplacha.2012.10.014.
- Gu L, Yue C, Zhao X, Xu H. 2020. Analysis of vegetation coverage change in Yunnan Province from 1999—2018 based on Google Earth Engine. Journal of West China Forestry Science. 49(5):74–80. doi: 10.16473/j.cnki.xblykx1972.2020.05.012.
- Guo J, Sang H, Zhai L. 2023. Spatiotemporal variations and driving factors of vegetation coverage on the Qinghai-Tibet Plateau. Chinese Journal of Ecology. 42(11):2665–2674. doi: 10.13292/j.1000-4890.202311020.
- He Y, Li T, Xiong Q, Yu L. 2018. Spatio-temporal patterns of vegetation coverage and response to hydrothermal factors in Yunnan Province. Acta Ecologica Sinica. 38(24):8813–8821. doi: 10.5846/stxb201806211364.
- Hou X, Ying L, Gao M, Bi X, Lu X, Zhu M. 2010. Character of vegetation cover change in China’s Eastern Coastal Areas 1998–2008. Scientia Geographica Sinica. 30(5):735–741. doi: 10.13249/j.cnki.sgs.2010.05.001.
- Huang M, Yue W, Fang B, Feng S. 2015. Scale response characteristics and geographic exploration mechanism of spatial differentiation of ecosystem service values in Dabie Mountain area, central China from 1970 to 2019. Acta Geographica Sinica. 74(9):1904–1920. doi: 10.11821/dlxb201909015.
- Jamali S, Jönsson P, Eklundh L, Ardö J, Seuist J. 2015. Detecting changes in vegetation trends using time series segmentation. Remote Sens Environ. 156:182–195. doi: 10.1016/j.rse.2014.09.010.
- Jia K, Yao Y, Wei X, Gao S, Jiang B, Zhao X. 2013. A review on fractional vegetation cover estimation using remote sensing. Adv Earth Sci. 28(7):774–782.
- John R, Chen J, Kim Y, Ou-Yang Z, Xiao J, Park H, Shao C, Zhang Y, Amarjargal A, Batkhshig O, et al. 2016. Differentiating anthropogenic modification and precipitation-driven change on vegetation productivity on the Mongolian Plateau. Landscape Ecol. 31(3):547–566. doi: 10.1007/s10980-015-0261-x.
- Kendall MG. 1975. Rank Correlation Methods. London: Charles Griffin
- Kennedy RE, Yang Z, Cohen WB. 2010. Detecting trends in forest disturbance and recovery using yearly Landsat time series: 1. LandTrendr—temporal segmentation algorithms. Remote Sens Environ. 114(12):2897–2910. doi: 10.1016/j.rse.2010.07.008.
- Konatowska M, Młynarczyk A, Kowalewski W, Rutkowski P. 2023. NDVI as a potential tool for forecasting changes in geographical range of sycamore (Acer pseudoplatanus L.). Sci Rep. 13(1):19818. doi: 10.1038/s41598-023-46301-x.
- Koutsoyiannis D. 2003. Climate change, the Hurst phenomenon, and hydrological statistics. Hydrol Sci J. 48(1):3–24. doi: 10.1623/hysj.48.1.3.43481.
- Krieger AM, Pollak M, Yakir B. 2003. Surveillance of a simple linear regression. J Am Stat Assoc. 98(462):456–469. doi: 10.1198/016214503000233.
- Lawrance AJ. 1976. On conditional and partial correlation. Am Statistic. 30(3):146–149. doi: 10.2307/2683864.
- Liang H, Kasimu A, Zhang X, Zhao Y, Reheman· R. 2023. Spatio-temporal change and influencing factors of land surface temperature in oasis urban agglomeration in arid region: a case study in the urban agglomeration on the northern slope of Tianshan Mountains. Acta Ecologica Sinica. 43(9):3650–3664. doi: 10.5846/stxb202205151368.
- Liang H, Meng Z, Li Z, Liu G. 2022. The effect of Robinia pseudoacacia plantation on soil desiccation across different precipitation zones of the Loess Plateau, China. Forests. 13(2):321. doi: 10.3390/f13020321.
- Li J, Liu L. 2023. Analysis of the spatiotemporal change and influencing factors of vegetation in Yunnan Province from 2000 to 2020. Acta Agrestia Sinica. 31(11):3503.
- Li Y, Song Y, Cao X, Huang L, Zhu J. 2024. Temporal—spatial changes in vegetation coverage under climate change and human activities: a case study of Central Yunnan Urban Agglomeration, China. Sustainability. 16(2):661. doi: 10.3390/su16020661.
- Liu Y, Lei S, Chen X, Chen M, Yang Y, Li X, Zhang X, Long L, Bian Z. 2021. Temporal variation and driving factors of vegetation coverage in Shendong central mining area based on the perspective of guided restoration. J China Coal Soc. 46(10):3319–3331. doi: 10.13225/j.cnki.jccs.2020.1387.
- Liu H, Li X, Mao F, Zhang M, Zhu D, He S, Huang Z, Du H. 2021. Spatiotemporal evolution of fractional vegetation cover and its response to climate change based on MODIS data in the subtropical region of China. Remote Sens. 13(5):913. doi: 10.3390/rs13050913.
- Mann HB. 1945. Non-parametric test against trend. Economet-rica. 13(3):245–259. doi: 10.2307/1907187.
- Nepita-Villanueva MR, Berlanga-Robles CA, Ruiz-Luna A, Morales Barcenas JH. 2019. Spatio-temporal mangrove canopy variation (2001–2016) assessed using the MODIS enhanced vegetation index (EVI). J Coast Conserv. 23(3):589–597. doi: 10.1007/s11852-019-00689-9.
- Pan Y, Wang J, Liu M LIJ, Wei X, Zhi L. 2023. Spatial and temporal evolution and driving analysis of green space ecosystem service value of urban agglomeration in Central Yunnan. Res Soil Water Conserv. 30(2):352–360. doi: 10.13869/j.cnki.rswc.2023.02.048.
- Pei J, Niu Z, Wang L, Huang N, Gao J. 2018. Monitoring to variations of vegetation cover using long-term time series remote sensing data on the Google Earth Engine cloud platform. Carsologica Sinica. 37(4):608–616. doi: 10.11932/karst20180415.
- Peng Z, Yang K, Luo Y, Yang J. 2021. Based on MOD13Q1 data to analyze the characteristics of vegetation changes in central Yunnan from 2000 to 2019. IOP Conf Ser: earth Environ Sci. 658(1):12007. doi: 10.1088/1755-1315/658/1/012007.
- Qiu L. 2022. Study on spatiotemporal variations and influencing factors of fractional vegetation coverage in Central Yunnan urban agglomeration. No.768, Juxian Street, Chenggong District, Kunming: Yunnan Normal University. https://kns.cnki.net/KCMS/detail/detail.aspx?dbcode=CMFD&dbname=CMFD202301&filename=1022611723.nh&v=.
- Sen PK. 1968. Estimates of the regression coefficient based on Kendall’s tau. J Am Stat Assoc. 63(324):1379–1389. doi: 10.1080/01621459.1968.10480934.
- Sun L, Li H, Wang J, Chen Y, Xiong N, Wang Z, Wang J, Xu J. 2023. Impacts of climate change and human activities on NDVI in the Qinghai-Tibet Plateau. Remote Sens. 15(3):587. doi: 10.3390/rs15030587.
- Sun W, Song X, Mu X, Gao P, Wang F, Zhao G. 2015. Spatiotemporal vegetation cover variations associated with climate change and ecological restoration in the Loess Plateau. Agric for Meteorol. 209-210:87–99. doi: 10.1016/j.agrformet.2015.05.002.
- Van De Voorde T, Vlaeminck J, Canters F. 2008. Comparing different approaches for mapping urban vegetation cover from landsat ETM + data: a case study on Brussels. Sensors (Basel). 8(6):3880–3902. doi: 10.3390/s8063880.
- Wang J‐F, Li X‐H, Christakos G, Liao Y‐L, Zhang T, Gu X, Zheng X‐Y, et a. 2010. Geographical detectors-based health risk assessment and its application in the neural tube defects study of the Heshun region, China. Int J Geograph Info Sci. 24(1):107–127. doi: 10.1080/13658810802443457.
- Wang D, Liu W, Huang X. 2013. Trend analysis in vegetation cover in Beijing based on Sen + Mann-Kendall method. Comp Eng Appl. 49(5):13–17. doi: 10.3778/j.issn.1002-8331.1206-0282.
- Wang J, Xu C. 2017. Geodetector: principle and prospective. Acta Geographica Sinica. 72(1):116–134. doi: 10.11821/dlxb201701010.
- Wang S, Zhang L, Lin W, Huang Q, Song Y, Ye M. 2022. Study on vegetation coverage and land-use change of Guangdong Province based on MODIS-NDVI. Acta Ecologica Sinica. 42(6):2149–2163. doi: 10.5846/stxb202104261100.
- Xiong J, Peng C, Cheng W, Li W, Liu Z, Fan C, Sun H. 2018. Analysis of vegetation coverage change in Yunnan Province based on MODIS-NDVI. J Geoinfo Sci. 20(12):1830–1840. doi: 10.12082/dqxxkx.2018.180371.
- Xu X, Jiao F, Lin D, Liu J, Zhang K, Yang R, Lin N, Zou C. 2023. Carbon sink trends in the karst regions of Southwest China: impacts of ecological restoration and climate change. Land. 12(10):1906. doi: 10.3390/land12101906.
- Xu H, Liu Q. 2022. Analysis of vegetation NDVI dynamic and its relationship with climatic factors in yunnan province during 2001–2019. Res Soil Water Conserv. 29(1):162–168. doi: 10.13869/j.cnki.rswc.2022.01.018.
- Xu Z, Wang Y, Sun G, Chen Y, Ma Q, Zhang X. 2023. Generating gridded gross domestic product data for China using geographically weighted ensemble learning. IJGI. 12(3):123. doi: 10.3390/ijgi12030123.
- Yan K, Gao S, Chi H, Qi J, Song W, Tong Y, Mu X, Yan G. 2022. Evaluation of the vegetation-index-based dimidiate pixel model for fractional vegetation cover estimation. IEEE Trans Geosci Remote Sens. 60:1–14. doi: 10.1109/TGRS.2020.3048493.
- Yang L, Liu F. 2024. Landscape ecological risk assessment and driving factors of urban agglomeration in Central Yunnan from the perspective of production-living-ecological space. J Southwest Forest Univ (Nat Sci). 44(3):1–14. doi: 10.11929/j.swfu.202304017.
- Yang J, Zhang Y, Wang J, Cao J. 2020. Vegetation index algorithm using NDVI and EVI resynthesis. Remot Sens Info. 35(5):127–133. doi: 10.3969/j.issn.1000-3177.2020.05.016.
- Yue M, Geng G, Wang T, Yang R, Gu Q. 2023. Spatiotemporal variation of vegetation NDVI and its driving factors in the Shaanxi section of the Yellow River Basin from 2000 to 2019. Res Soil Water Conserv. 30(2):238–246. doi: 10.13869/j.cnki.rswc.2023.02.022.
- Yue S, Wang C. 2004. The Mann-Kendall test modified by effective sample size to detect trend in serially correlated hydrological series. Water Resour Manage. 18(3):201–218. doi: 10.1023/B:WARM.0000043140.61082.60
- Zhang G, Liang Y. 1996. A summary of impact of vegetation coverage on soil and water conservation benefit. Res Soil Water Conserv. 3(2):104–110.
- Zhang X, Liao C, Li J, Sun Q. 2013. Fractional vegetation cover estimation in arid and semi-arid environments using HJ-1 satellite hyperspectral data. Int J Appl Earth Obs Geoinf. 21:506–512. doi: 10.1016/j.jag.2012.07.003.
- Zhang B, Wu P, Zhao X. 2011. Detecting and analysis of spatial and temporal variation of vegetation cover in the Loess Plateau during 1982–2009. Transac CSAE. 27(4):287–293. doi: 10.3969/j.issn.1002-6819.2011.04.051.
- Zhang Y, Zhu Z, Liu Z, Zeng Z, Ciais P, Huang M, Liu Y, Piao S. 2016. Seasonal and interannual changes in vegetation activity of tropical forests in Southeast Asia. Agric for Meteorol. 224:1–10. doi: 10.1016/j.agrformet.2016.04.009.
- Zhi L, Wang J, Liu M, Wang Z. 2021. Multi-scale dynamic change of green spatial pattern of urban agglomeration in Central Yunnan. J Southwest Forest Univ (Nat Sci). 41(5):88–97. doi: 10.11929/j.swfu.202009064.
- Zuidema PA, Babst F, Groenendijk P, Trouet V, Abiyu A, Acuña-Soto R, Adenesky-Filho E, Alfaro-Sánchez R, Aragão JRV, Assis-Pereira G, et al. 2022. Tropical tree growth driven by dry-season climate variability. Nat Geosci. 15(4):269–276. doi: 10.1038/s41561-022-00911-8.