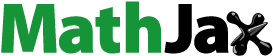
Abstract
The purpose of the presented study is to evaluate the comprehensive impact of different land cover types on the temperature development in the entire Shandong Province by a 20-year-long (MODIS) LST time series from 2003 to 2022. To find out the primary influencing factors, methods such as the Pearson correlation, stepwise analysis, and best-subset selection were applied. The results revealed that the average temperatures had been rising in summer during day- and night-time by 2.32 °C and 1.22 °C, respectively and in winter during day- and night-time by 3.25 °C and 1.33 °C, whereby a significant contribution can be attributed to the period 2014-2022. Substantial variations in LSTs emerge between built-up and vegetated areas and landlocked and coastal regions. Moreover, we could identify a contribution of 0.089 °C, caused merely by the extension of built-up areas of 1.65% in the entire Shandong Province. Modeling the combined effects of further relevant variables/factors, the percentage of cropland area (crop-per) and the number of landscape patches (NPl) indicate considerable influence on the daytime temperature in the temporal domain.
1. Introduction
In recent years, rapid urban sprawl and progressive population growth have led to the replacement of land cover, further affecting the rise in land surface temperatures (Barman et al. Citation2024, Chen et al. Citation2023, Tuholske et al. Citation2021). Land surface temperature (LST) is a primary key parameter influencing the physical, chemical and biological processes of the Earth. It plays a crucial role in climate change, evapotranspiration, hydrological cycle, vegetation monitoring, and thus, in urban climate and environmental studies (Peng et al. Citation2018; Hansen et al. Citation2010; Ye et al. Citation2017). Against the background of global warming and frequent extreme weather, the changes of LST are of great concern not only to social life and public health, but also to the stability of ecosystems (Fida et al. Citation2024; Zhang et al. Citation2023). Owing to the high heterogeneity of land surface features comprising build-up areas, vegetation canopies, topography, and different types of soils (Liu et al. Citation2016; Neteler Citation2010), LST varies in space and time (Prata et al. Citation1995; Li et al. Citation2011). Therefore, it is of great importance to further consider the influencing factors of the LST distribution and its seasonal/temporal evolution.
There are many studies on land surface temperature that can roughly be classified into three categories. First, there is quite a large number of publications on LST retrieval algorithms and methods like those presented by Prata et al. (Citation1995) and Dash et al. (Citation2002). Second, there are numerous studies dealing with the dynamic changes of the urban thermal environment. They focus primarily on the distribution of spatial-temporal changes of LST, heat islands, the development of morphological features, and the environmental impacts they cause (Luintel et al. Citation2019, Zhang et al. Citation2023). A third emphasis is on researching the relationship between LSTs and their influencing factors as well as possible interactions. In general, there are three major and widely focused factors concerning this subject. (1) Surface biophysical parameters such as land use and land cover information (Fida et al. Citation2024; Liu et al. Citation2016) that show an acceptable linear relationship with LSTs. (2) Landscape metrics, especially urbanization and different types or proportions of landscape components that have been studied by Chen et al. (Citation2023) and Zhou et al. (Citation2017). (3) Socio-economic factors such as population density and GDP have also proven a significant effect on LSTs and, especially, on the formation of urban heat islands (UHI) (Kotharkar and Surawar Citation2016; Huang and Cadenasso Citation2016).
For China, as one of the world’s fastest growing urbanized countries, it is also of great interest to consider the spatial, temporal and trend variations on annual and seasonal time-scales in order to better understand the associated processes. Previous studies such as the ones by Liu et al. (Citation2016) and Peng et al. (Citation2018) have achieved good results, but failed to integrate the overall influencing effects of land cover, urban sprawl, vegetation space, waterbody, build-up metrics, landscape metrics and socio-economic factors on LST. It is, therefore still difficult to determine the predominant factors influencing LSTs in the various provinces of China. Apart from that, there is still a lack of research concerning the detailed quantification and interpretation of temperature variations, including their driving factors.
One key topic in exploring the relationship between LSTs and their driving factors is the choice of the most suitable model. In order to quantitatively investigate this issue, the most commonly used methods include Pearson correlation analysis and general least square regression analysis. (Chen et al. Citation2023; Du et al. Citation2022; Guo et al. Citation2020). To measure spatial correlations, some studies used geographically weighted regressions (Guo et al. Citation2020), while stepwise regressions were often applied in multivariate analysis to find an optimal model (Asgarian et al. Citation2015). However, these models do not capture dominant factors neither a ranking of all the influencing factors.
Thus, the motivation for our study resulted from a missing holistic approach to analyze spatiotemporal variations of an extended area over a long time period and find and investigate the most significant driving forces for the extracted variations. For that purpose, the entire province of Shandong was selected, as a long time series of thermal observations are available here, recorded by the MODIS sensor from 2003 to 2022. Thereby, our study focused on the following aspects. The investigation of the spatial and temporal variations of the LSTs within this time period and the trends that can be derived from them and the interrelations to changes of land cover, urban sprawl and LSTs by utilizing correlation techniques and methods of best-subset selections. Thereby, association between the LST trends and various factors such as surface biophysical parameters, built-up metrics, landscape metrics and socio-economic factors can be derived. This knowledge can provide a theoretical basis for rational urban development planning, improving the ecological environment and promoting sustainable development in the future.
2. Materials and methods
2.1. Study area
The Shandong Province () covers an area of 157,100 km2 and is located on the east coast of China (114°36′-122°43′E, 34°25′-38°23′N). The western part is landlocked, while the eastern part of the province is shaped like a peninsula protruding into the Yellow Sea. In the central part of the province, the mountainous areas are ascending, where Mount Tai represents the highest elevation in the Shandong Province with an altitude of up to 1524 meters. In the southwest and northwest, more low-lying and flat areas are located. The region is characterized by a warm temperate monsoon climate with concentrated precipitation and heat during the short spring and autumn, and long winter and summer periods. The duration of sunshine is long, and the annual average precipitation ranges between 550 mm and 950 mm. The Shandong Province is one of the most important economic regions of China. Its population ranks second and the gross domestic product (GDP) third.
2.2. Data source
1840 MODIS 11A2 recordings of day and night data of the period from 2003 to 2022 were obtained from NASA’s website. MODIS 11A2 is an 8-day LST level3 product providing data at a spatial resolution of 1 km, fully calibrated and corrected for atmospheric and aerosol attenuations. Different methods are used to process single data takes, for e.g. monthly average temperatures by cropping, numerical conversion and synthesis. Further MODIS data at a spatial resolution of 0.5 km (12Q1) were obtained from satellite composite products (Friedl et al. Citation2002) as the source for estimating the land use area. Additional information about the population density and the GDP data was taken from the Shandong Statistical Yearbook. For the comparative analysis of meteorological data and recorded LSTs, we combined the daily average temperature with monthly average temperatures. (https://gis.ncdc.noaa.gov/maps/ncei/summaries/daily).
2.3. Methods
The research flowchart of this study is depicted in . After retrieving the LSTs of all data from the years 2003 to 2022, the respective land cover area was extracted and landscape metrics were calculated. Then, various models were applied to investigate the relative importance and marginal effects of all possible influencing factors.
2.3.1. Retrieval of LSTs
The main process of surface temperature inversion includes: cropping the study area, batch processing using MODIS Reprojection Tool (MRT), converting data of geographic coordinates, reprojection, and converting surface temperature data. According to the MODIS documentation, the pixel brightness values are converted to surface temperature in degrees Celsius using the formula 1.
(1)
(1)
2.3.2. Reclassification of land cover areas
MCD12Q1 is a level-3 product acquired by the MODIS Terra + Aqua satellites, with a GSD of 500 m. It provides five land classification types, derived by employing a supervised decision tree classification. This study primarily adopts the type 1 of land classification.
2.3.3. Urban sprawl
The following equation published by Qiao et al. (Citation2013) was used to calculate the temperature rise caused by built-up areas.
(2)
(2)
where
is the contribution of the functional zone or landscape to the regional LST,
is the average LST difference between the functional zone or the landscape and the entire area, and
is the proportion of the area.
2.3.4. Factors influencing the LST
We selected a number of variables (indices and factors), assumed to be influencing the LST, to estimate their impact on temperature development in relation to different land use classes ( and ). For details of the used equations and comments, see Barman et al. (Citation2024), Chen et al. (Citation2023), Li et al. (Citation2011) and Zhou (Citation2018).
Table 1. Factors influencing the LST.
Table 2. Calculation of biophysical surface parameters.
2.3.5. Statistical analysis
To investigate the relationship between temperature and potential impact factors, several statistical analysis methods were used (Asgarian et al. Citation2015; Peng et al. Citation2018; Han et al. Citation2023). A Pearson correlation analysis was applied to study the relationship of the recorded temperatures with the chosen variables and to identify distinct influencing factors on the LST. To avoid the collinearity of multiple significant influencing factors, a multiple stepwise regression was applied to calculate the comprehensive explanation rate of each stratification factor on LST variations. To consider all possible combinations of the variables, and to accurately predict the relationship between the temperature and its impact with small multicollinearity, the best-subset selection was chosen.
3. Results
3.1. Overall change of LSTs in space and time
To cope with the huge amount of temperature data we calculated mean values for the summer and winter time. gives an overview of the recorded average temperatures for the entire Shandong Province during 2003–2022 based on diurnal recordings in winter and summer. In general, the violin plot depicts minor temperature variations during summer, both day and night, as compared to the winter recording. In June, we see the highest temperature values during daytime which also depict the largest temperature difference during the diurnal circle. From July to November, the day-night temperature differences are less strong than in the period from December to June. There is also a significant rising trend in LSTs during both day and night within the time-frame of the investigated 20 years. The temperatures of the entire Shandong Province in summer increased by an average of 0.116 °C per year during the day, and by an average of 0.061 °C per year, at night. The successive winter recordings depict a temperature increase per year by an average of 0.162 °C during day and 0.067 °C at night. Generally, the rise in the average annual temperature in winter is higher than in summer and the rate of increase is higher during the day than at night for the study period. The total temperature increase for the entire Shandong Province during this period added up to 2.32 °C and 1.22 °C in summer during day- and night-times respectively and to 3.25 °C and 1.33 °C in winter during day- and night-times respectively.
Figure 3. Violin plot of the monthly average temperatures in winter (December, January and February) and summer (June, July and August) for the Shandong Province during the years 2003–2022.

The temperature distribution throughout the entire province is illustrated in detail in and . For the summer day recordings (), the temperatures are in general lower at the peninsula in the east along the coastline to the south and in the districts located NNW of the province. From 2011 on, we see a dramatic change where temperatures increased more significantly, especially along an E-W central trail from Liaocheng city to Zibo, Weifang and Qingdao. The summer night recordings show lower temperatures at the peninsula part of the province (). The winter day records () also show lower temperatures at the peninsula in the east and frequently for the cities located in the NNW of the study area. The temperature distribution in 2012 and 2013 indicates a phase of strong cooling followed by increasing temperatures especially for the SSW. Night recordings during winter () depict colder temperatures for the northern districts including the peninsula and are somewhat pronounced for the years 2010–2012. Only the year 2017 shows a clear increase in the overall temperatures. Thus, our findings indicate a reliable increase of LSTs over the course of two decades in summer as well as in winter.
3.2. Influence of different land cover types on variations of LSTs
To study the temporal changes in dependency of different land cover types, pixels/areas were selected that maintained the same cover class over the 18-year period. It is found that, in general, the temperatures of all investigated land use types show an increasing trend during day and night, in summer as well as winter (). To be more specific, during daytime in summer, built-up areas exhibit the highest LSTs and the steepest gradient (∼3.2 °C), followed by croplands (∼2.5 °C). Inland water bodies (lakes) followed by forests, show the lowest temperatures but similar temperature gradients (∼2 °C). During daytime in winter, all land cover types depict a similar temperature increase of about 2.1 °C in the recent 20 years. Concerning night temperatures, waterbodies with their very high thermal capacity show the highest temperatures followed by buildings. Croplands with a growth rate of 0.06 °C/y, and forests display the lower temperatures at night.
3.3. The impact of sprawling built-up areas on LST
illustrates the increase in temperature in relation to the annual areal increment of built-up areas. During the period under review, Liaocheng city sprawled by a further 113 km2 (561 km2 to 674 km2 which corresponds to a share of 16.7%) and Heze city grew by an area of 112 km2 (9.8%). The coastal cities of Qingdao and Rizhao expanded by 270 km2 (15.9%) and 116 km2 (31.2%) respectively. The mean temperature increases for the cities of Liaocheng and Heze was 2.73 °C and 3.35 °C and for the cities of Qingdao and Rizhao 2.30 °C and 2.14 °C respectively. These four cities show the highest temperature increase relative to the highest expansion areas. also enhances the most active periods of urbanization comparing the increase in built-up areas with the respective time frames. Liaocheng and Heze display a higher sprawl from 2003 to 2006, and again during 2016 and 2017. Qingdao grew mostly from 2003 to 2007 and from 2012 to 2017 while Rizhao expanded vigorously from 2013 to 2015. To estimate the overall impact of urban sprawl on the temperature development for the entire Shandong Province, the areas of the classification layers of building areas were surveyed. The entire Shandong Province covers an area of 157,100 km2, where the built-up area amounted to 12,450 km2 in 2003 and 15,047 km2 in 2020. This corresponds to an areal accumulation of built-up areas of 2,597 km2 in 18 years, resulting in a measurable average temperature increase of 3.33 °C. Using Equationequation 1(1)
(1) published by Qiao et al. (Citation2013), a contribution of 0.089 °C to the general temperature rise is calculated, which is solely attributable to the expansion of built-up areas in Shandong Province. The rise in temperatures in recent years is primarily the result of the generally recognized global warming, triggered by the release of increasing amounts of greenhouse gases into the atmosphere. But it could be unequivocally demonstrated that the continuous urban sprawl, with its related replacement of natural surfaces and vegetated areas by man-made materials with unfavorable thermal properties, has a measurable share of this issue (Qiao et al. Citation2013).
3.4. Analysis of the combined influence of further variables on LSTs
To explore the relationship between the temperature trends and the combined influence of other potential impact factors grouped in , a variety of statistical analysis methods was applied. There, calculated R or R2 values shows how exactly each factor can explain the respective LST. The R column display the results of the Pearson correlation of LSTs with the corresponding factors. Thereby, all factors except water-per, LSIc, and PDl passed the significance test for summer daytime recordings and, thus, indicated that most of the factors show a high association with LSTs (). The Adj. R2 gives the result of a stepwise regression between each factor layer and LSTs, also indicating that all sections obtained nearly equal explanation rates (). In detail, the ‘landscape’ metrics category shows the highest explanation rate of 75.5%, followed by the ‘socioeconomics’ category with 74.6%. It implies that these two layers best explain the spatial differentiation of LSTs in summer daytime. The category ‘waterbody’ exhibits the lowest explanation rate among all categories, reaching only 23%.
Table 3. Pearson correlation and stepwise regression results for factors affecting LSTs.
depicts the results of the subset regression conducted to determine which model can best fit the LSTs. The selected impact factors were crop-per, ED, NPl, LSIl, pop-den and GDP, all of which entered the stepwise regression test. Based on these six significant impact factors for summer daytime recordings we established the 11 best regression models for our calculations. The resulting optimal subset regression model yields two best regression models for every change in the number of independent variables. The higher the R2 value, the better the model fits the data. S represents the standard deviation of the error of the regression model. The lower the S value, the higher the model describes the response. The adjusted R2 was taken an indicator for judging the accuracy of the model fitting. The GDP gives the highest explanation rate for all variables (R2 = 67.1%; S = 0.57) followed by the crop-per layer (R2 = 66.2%; S = 0.58). This is consistent with the results of the Pearson regression analysis presented in . Compared to the 11 models calculated, the best model has the highest R2 value of 79.8%, and the lowest S value of 0.45. These two variations are crop-per and NPl. It can be deduced that of all tested variations, these two had the most impact on the LST in summer during these 18 years. shows that each layer of variations affects the temperature to a greater or lesser extent. The spatial pattern of LSTs is usually affected by the combined influence with multiple factors rather than only a single factor.
Table 4. Results of the optimal subset regression.
4. Discussion
4.1. Potential impact of land use dynamics on LST
In our study, forests exhibit the lowest temperatures during summer, whereas the farmlands depict the lowest temperatures in winter. The reason for this inversion has already been described by Qiao et al. (Citation2013) and is explained by their different thermal inertia that is much lower for cropland than for forests. Thermal inertia is the tendency of a material to resist changes in temperature. The LSTs of cropland also show a higher variability as compared to that of forests, which is due to the strongly varying seasonal phenology. The temperature increase rate for each land cover type is higher during the day as compared to that at nighttime (). The surface temperature of forests during the day is significantly lower than that of farmlands (Gillies and Carlson Citation1995). During the day, the evapotranspiration of the forests is higher than that of the croplands, and thus, it induces a stronger cooling effect. The built-up areas are mainly composed of concrete, asphalt, steel and other man-made materials that are usually characterized by high thermal mass and inertia. They are usually able to heat up strongly during the day but have only a moderate capacity to store heat for a longer time (see night temperatures). The nighttime average temperature is highest for water and lowest for forests. In general, the temperature of forests is lowest, whether recorded at day or at night. Waterbodies exhibit the lowest temperature difference between day and night, as water has by far the highest thermal capacity and therefore absorbs and releases heat very slowly.
4.2. Selection of factors
Many authors have investigated the influence of land cover (Fida et al. Citation2024) and landscape metrics (Barman et al. Citation2024; Han et al. Citation2023) on the LST, while little attention has been paid to surrounding factors. In our study, five types of surrounding factors, including vegetation, water, built-up areas, landscape and socioeconomics, were used to investigate their impacts on the LST. Landscape metrics such as NP, PD, LSI, COHESION, and SHDI have been applied in ecological and environmental research (An et al. Citation2022). These metrics can describe surrounding landscapes from the perspective of quantity, shape complexity, and spatial aggregation, comprehensively measuring the composition and spatial configuration characteristics (Han et al. Citation2023). Moreover, built-up metrics like PD, ER, LPI, LSI, and AI were used to describe building morphology. The above indicators can characterize anthropogenic heat flux, solar shortwave radiation, and sensible and latent heat, which can comprehensively characterize the urban energy balance process (Han et al. Citation2023; Yang et al. Citation2021).
4.3. Validation and limitations
The importance of LST information derived from remotely sensed thermal infrared data (TIR) via the MODIS instrument mounted on the TERRA and AQUA platforms, has already been proven by many scientists (Wan et al. Citation2004). For validation purposes, the temperature records of several evenly distributed meteorological ground stations () have been evaluated. Although, the absolute temperatures given by local ground stations (mean values of day and night temperatures) and those from satellite data cannot be directly compared, both show a comparable increase for the period.
As far as the limitations of our study are concerned, there are still a few points that need to be mentioned. First, the spatial resolution of the used land cover data is relatively low. The integration of satellite data with higher resolution is promising, but difficult and extremely time-consuming. Secondly, the effects of environmental factors were only investigated for the summer period. Further studies are planned for the future that examine more seasonal data in order to gain a more comprehensive understanding of their influence. Finally, the impact of surface waters on LST should be further investigated, preferably in regions where there is more surface water than in the Shandong Province, where water areas are scarce.
5. Conclusion
In our study we examined the annual, seasonal, temporal and spatial variations of MODIS LSTs during day and night in the Shandong Province from 2003 to 2022. Our results show that there has been a clearly recognizable rise in LSTs during the past 20 years, whereby the magnitude of temperature change for forests, crops, and water is lower in summer as compared to winter. In our study, we took into account 15 influencing factors in five dimensions. We found that all types of land cover classes influence temperatures differently in certain seasons, explaining that the factors affecting temperature are multi-layered. Crop-per and NPl are the most impact on the LST in summer which account for 79.8% during these 18 years. Therefore, these findings provide a basis for rational and sustainable planning for urban development in the future and may induce a more careful deliberation over how to balance ecological and economic issues.
Author contributions
All authors contributed to the study conception and design. Material preparation and original draft preparation were completed by Tingting Chen, Methodology and data collection were performed by Shanyu Zhou and Yu Wang. Review and editing were written by Ran Kang and Hang Chen. Supervised by Hermann Kaufmann. All authors commented on previous versions of the manuscript. All authors read and approved the final manuscript.
Acknowledgements
We would like to thank the anonymous reviewers for their time and efforts to review this paper and improve our work. This research did not receive any specific grant from funding agencies in the public, commercial, or not-for-profit sectors.
Disclosure statement
No potential conflict of interest was reported by the author(s).
Data availability statement
The data will be available upon reasonable request through corresponding authors.
Additional information
Funding
References
- An H, Cai H, Xu X, Qiao Z, Han D., 2022. Impacts of urban green space on land surface temperature from urban block perspectives. Rem Sens. 14(18):4580. doi:10.3390/rs14184580.
- Asgarian A, Amiri BJ, Sakieh Y. 2015. Assessing the effect of green cover spatial patterns on urban LSTs using landscape metrics approach. Urban Ecosyst. 18(1):209–222. doi:10.1007/s11252-014-0387-7.
- Barman S, Roy D, Chandra Sarkar B, Almohamad H, Abdo HG. 2024. Assessment of urban growth in relation to urban sprawl using landscape metrics and Shannon’s entropy model in Jalpaiguri urban agglomeration, West Bengal, India. Geocarto Int. 39(1):2306258. doi:10.1080/10106049.2024.2306258.
- Chen Y, Yang J, Yu W, Ren J, Xiao X, Xia JC. 2023. Relationship between urban spatial form and seasonal land surface temperature under different grid scales. Sustainable Cities and Soc. 89:104374. doi:10.1016/j.scs.2022.104374.
- Dash P, Göttsche F-M, Olesen F-S, Fischer H. 2002. Land surface temperature and emissivity estimation from passive sensor data: theory and practice-current trends. Int J Remote Sens. 23(13):2563–2594. doi:10.1080/01431160110115041.
- Du C, Jia W, Chen M, Yan L, Wang K. 2022. How can urban parks be planned to maximize cooling effect in hot extremes? Linking maximum and accumulative perspectives. J Environ Management. 317:115346.
- Fida GT, Baatuuwie BN, Issifu H. 2024. Potential impact of future land use/cover dynamics on the habitat quality of the Yayo Coffee Forest Biosphere Reserve, southwestern Ethiopia. Geocarto Int. 39(1):2278327. doi:10.1080/10106049.2023.2278327.
- Friedl MA, McIver DK, Hodges JCF, Zhang XY, Muchoney D, Strahler AH, Woodcock CE, Gopal S, Schneider A, Cooper A, et al. 2002. Global land cover mapping from MODIS: algorithms and early results. Remote Sens Environ. 83(1–2):287–302. doi:10.1016/S0034-4257(02)00078-0.
- Gillies RR, Carlson TN. 1995. Thermal remote sensing of surface soil water content with partial vegetation cover for incorporation into climate models. J Appl Meteor. 34(4):745–756. doi:10.1175/1520-0450(1995)034<0745:TRSOSS>2.0.CO;2.
- Guo A, Yang J, Sun W, Xiao X, Xia Cecilia J, Jin C, Li X. 2020. Impact of urban morphology and landscape characteristics on spatiotemporal heterogeneity of land surface temperature. Sustainable Cities and Soc. 63:102443. doi:10.1016/j.scs.2020.
- Han D, Xu X, Qiao Z, Wang F, Cai H, An H, Jia K, Liu Y, Sun Z, Wang S, et al. 2023. Roles of surrounding 2D/3D landscapes in park cooling effect: analysis from extreme hot and normal weather perspectives. Build Environ. 231:110053. doi:10.1016/j.buildenv.2023.
- Hansen J, Ruedy R, Sato M, Lo K. 2010. Global surface temperature change. Rev Geophys. 48(4):RG4004. doi:10.1029/2010RG000345.
- Huang GL, Cadenasso ML. 2016. People, landscape, and urban heat island: dynamics among neighborhood social conditions, land cover and surface temperatures. Landscape Ecol. 31(10):2507–2515. doi:10.1007/s10980-016-0437-z.
- Kotharkar R, Surawar M. 2016. Land use, land cover, and population density impact on the formation of canopy urban heat islands through traverse survey in the Nagpur urban area, India. J Urban Plann Dev. 142:1. doi:10.1061/(ASCE)UP.1943-5444.0000277.
- Li JX, Song CH, Cao L, Zhu FG, Meng XL, Wu JG. 2011. Impacts of landscape structure on surface urban heat islands: a case study of Shanghai, China. Remote Sens Environ. 115(12):3249–3263. doi:10.1016/j.rse.2011.07.008.
- Liu YX, Wu CY, Peng DL, Xu SG, Gonsamo A, Jassal RS, Arain MA, Lu LL, Fang B, Chen JM. 2016. Improved modeling of land surface phenology using MODIS land surface reflectance and temperature at evergreen needleleaf forests of central North America. Remote Sens Environ. 176:152–162. doi:10.1016/j.rse.2016.01.021.
- Luintel N, Ma W, Ma Y, Wang B, Subba S., 2019. Spatial and temporal variation of daytime and nighttime MODIS land surface temperature across Nepal. Atmos Oceanic Sci Lett. 12(5):305–312. doi:10.1080/16742834.2019.1625701.
- Neteler M. 2010. Estimating daily LSTs in mountainous environments by reconstructed MODIS LST data. Remote Sens. 2(1):333–351. doi:10.3390/rs1020333.
- Peng J, Jia J, Liu Y, Li H, Wu J. 2018. Seasonal contrast of the dominate factors for spatial distribution of land surface temperature in urban areas. Remote Sens Environ. 215:255–267. doi:10.1016/j.rse.2018.06.010.
- Prata AJ, Caselles V, Coll C, Sobrino JA, Ottlé C. 1995. Thermal remote sensing of land surface temperature from satellites: current status and future prospects. Remote Sens Rev. 12(3–4):175–224. doi:10.1080/02757259509532285.
- Qiao Z, Tian GJ, Xiao L. 2013. Diurnal and seasonal impacts of urbanization on the urban thermal environment: a case study of Beijing using MODIS data. ISPRS, J Photogrammetr Remote Sens. 85:93–101. doi:10.1016/j.isprsjprs.2013.08.010.
- Tuholske C, Caylor K, Funk C, Verdin A, Sweeney S, Grace K, Peterson P, Evans T. 2021. Global urban population exposure to extreme heat. Proc Natl Acad Sci U S A. 118(41):e2024792118. doi:10.1073/pnas.2024792118.
- Wan Z, Zhang Y, Zhang Q, Li ZL. 2004. Quality assessment and validation of the MODIS global land surface temperature. Int J Remote Sens. 25(1):261–274. doi:10.1080/0143116031000116417.
- Yang J, Yang Y, Sun D, Jin C, Xiao X., 2021. Influence of urban morphological characteristics on thermal environment. Sustainable Cities and Soc. 72:103045. doi:10.1016/j.scs.2021.103045.
- Ye X, Ren H, Liu R, Qin Q, Liu Y, Dong J. 2017. Land surface temperature estimate from Chinese Gaofen-5 satellite data using split-window algorithm. Transac Geosci Remote Sens. 55(10):5877–5888. doi:10.1016/j.rse.2003.11.005.
- Zhang R, Yang J, Ma X, Xiao X, Xia J. 2023. Optimal allocation of local climate zones based on heat vulnerability perspective. Sustainable Cities and Soc. 99:104981. doi:10.1016/j.scs.2023.104981.
- Zhou WQ, Wang J, Cadenasso ML. 2017. Effects of the spatial configuration of trees on urban heat mitigation: a comparative study. Remote Sens Environ. 195:1–12. doi:10.1016/j.rse.2017.03.043.
- Zhou ZG. 2018. Analysis of evolution characteristics of Nanchang’s ecological spatial landscape pattern based on RS and GIS. China: Jiangxi Normal University; p. 1–55.