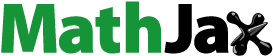
Abstract
Satellite-based monitoring provides insights on synergies and trade-offs between energy production and land use and land cover (LULC) changes (LULCC) around hydropower dams. Using Landsat data, we mapped LULCC related to hydropower expansion to understand change dynamics of cash crop production in the Magdalena River basin (Colombian Andes). We leveraged secondary map products and an active learning routine to produce thematically detailed LULC maps, for 2009, 2015, and 2020, including key cash crops (rice and oil palm). Our area-adjusted accuracy assessment revealed high overall and class-specific user’s and producer’s accuracies, exceeding 80% for oil palm, rice, non-agricultural vegetation, wetlands, and grasslands, and accuracies of ∼60% for other temporary and permanent crops. We identified substantial losses of rice (10,6%) and grasslands (25,9%) in El Quimbo due to reservoir flooding. For Hidrosogamoso, there was a loss of wetlands downstream. Our products provide the basis for balancing social, economic, and environmental trade-offs related to conflicting land uses.
1. Introduction
Land is a limited resource, underlying increasing pressure for multiple competing uses, including agriculture, extractive activities, energy production, built-up environments, but also for protection of the natural environment, or biodiversity conservation (Munroe and Müller Citation2020; Xie et al. Citation2020; Turner et al. Citation2021; Deteix et al. Citation2023). Global energy demand has increased rapidly in the last decade (IEA Citation2022). At the same time, the climate crisis and emerging tipping point discussions (Dakos et al. Citation2019) have triggered a resurgence of large hydropower projects for renewable energy production, with hotspots of future dam construction clustering in the Global South (Zarfl et al. Citation2015). Dams induce land system changes, from their construction to the operational phase, altering the access to and the use of land and water resources (Rufin et al. Citation2019). These changes play different roles downstream and upstream of dams, with drastic changes of local livelihoods (Castro-Diaz et al. Citation2023) and major ecosystem changes, such as depletion of riparian vegetation and wetlands (Rosenberg et al. Citation2000). The land surrounding reservoirs is often subject to increased deforestation or agricultural intensification, triggered by irrigation (Rufin et al. Citation2018; Lohani et al. Citation2020; Velastegui-Montoya et al. Citation2020) while at the same time traditional fishing and agriculture are negatively affected (Richter et al. Citation2010). Already prevailing inequalities may increase, such as disputes over land ownership, water distribution and energy resources (Boelens et al. Citation2016). Understanding land use and land cover change (LULCC) around dams is hence one of the crucial impacts for monitoring dam-related changes. Such information is also core for disentangling diverse impacts on agricultural land use and productivity, threats to biodiversity and habitats, and carbon sequestration potential (Domínguez and Rivera Citation2010; Woldemichael et al. Citation2012; De Oliveira Serrão et al. Citation2020; De Andrade et al. Citation2021; Zhang et al. Citation2022).
The Colombian Andes constitute a prime example of growing pressure on land due to conflicting land uses. The region is a hot-spot of dam construction, while at the same time being a key production area for cash crops. Colombia is planning to increase its current electricity production by 135% until 2050 (UPME Citation2020) and has a remaining hydropower potential to be explored (Xu et al. Citation2023), making it a hotspot of future hydropower expansion. Most hydropower dams are concentrated in the Magdalena River basin and the Andes region (Craven et al. Citation2017; Angarita et al. Citation2018), where an expansion of the hydropower sector is likely due to the existing infrastructure and the suitable topography of the region (Angarita et al. Citation2018). The Magdalena basin is home to 70% of the Colombian population (DANE Citation2022), responsible for 80% of the national energy production, and 70% of national agricultural production (Craven et al. Citation2017), mostly linked to the production of ‘cash crops’, or ‘export crops’ such as coffee, rice, and oil palm (Agronet Citation2021). The basin is a biodiversity hotspot (Myers et al. Citation2000), consisting of wetlands and paramos (Ricaurte et al. Citation2017; Patiño et al. Citation2021). Between 2005 and 2010, the Magdalena River basin experienced a loss of 40% of its natural land cover, while agriculture and pasture increased 65% (Restrepo and Escobar Citation2018). Despite a regrowth of forests in marginal agricultural areas (Rubiano et al. Citation2017), deforestation linked to the production of cash crops such as coffee and oil palm is one of the major drivers of change in the tropical forests of Colombia (Pendrill et al. Citation2019b, Citation2019a; Treanor and Saunders Citation2021). The growing demand for renewable energy and cash crops (Raihan Citation2023) requires an improved understanding of the trade-offs between energy production, agricultural production, and the natural ecosystems in this dynamic region.
Land use and land cover (LULC) maps derived from Earth observation data are an important tool to monitor changes and provide timely and standardized information for policies and environmental management (Chowdhury et al. Citation2021). Data acquired from the Landsat sensor family offers unique data continuity since the 1970s (Chowdhury et al. Citation2017; Wulder et al. Citation2019). The Landsat Global Archive Consolidation effort, along with standardized pre-processing schemes and improved processing capabilities now allows using all available data for long and dense time series analyses (Zhu and Woodcock Citation2014; Wulder et al. Citation2022). In some world regions, such as in parts of Africa and Latin America, Landsat data availability can be limited, due to persistent cloud cover and limited observation capacity in previous decades (Wulder et al. Citation2016). As such, integrating time series information across multiple years can be a necessity to obtain gap-free coverage for historic time slices and for separating spectrally similar LULC classes (Griffiths et al. Citation2013; Müller et al. Citation2015; Potapov et al. Citation2021). Multiple studies have investigated the use of aggregating time series information using statistical aggregation functions on distribution and variance - also referred to as spectral temporal metrics (STMs) - in LULC mapping. STM have been successfully used for applications targeting the mapping of agricultural land use across large regions and long time frames (Waldner et al. Citation2015; Rufin et al. Citation2022b), involving data-scarce periods and regions (Pflugmacher et al. Citation2019; Tang et al. Citation2021; Nill et al. Citation2022), while maintaining the temporal information that allows for creating thematically detailed maps, e.g. on particular tree species, crop types or management practices (Deines et al. Citation2017; Hemmerling et al. Citation2021; Ibrahim et al. Citation2021).
Multiple map products exist for Colombia, but limitations in thematic and spatial detail, and temporal coverage hamper assessments of LULCC related to hydropower expansion and cash crops. Global maps can provide information on forests (Hansen et al. Citation2013), water surfaces (Pickens et al. Citation2020), croplands (Potapov et al. Citation2021), or specific crop types, such as oil palm (Descals et al. Citation2021). Such maps can provide a valuable resource for identifying spatial patterns and distribution at the national to global level, but they do not provide the thematic detail for capturing regionally relevant change trajectories and may suffer region-specific inaccuracies (Tulbure et al. Citation2021). At the national level, the Instituto de Hidrología, Meterología y Estudios Ambientales (IDEAM) provides LULC maps based on the CORINE methodology (IDEAM Citation2010a). These maps were generated for specific years (2002, 2009, 2012, and 2018) with a minimum mapping unit of 25 ha (IDEAM Citation2023). Maps created by the SINCHI Institute (SINCHI Citation2022) and MapBiomas (Mapbiomas Citation2021) are only available for the Colombian Amazon. González-González et al. (Citation2022) provide a LULC map for the Colombian Andes and Amazon at high spatial resolution for 2018, but provide limited thematic detail in the agriculture domain, which does not allow for in-depth assessments of land change trajectories relevant in the context of dam construction and the dynamics of cash crops in the Magdalena River basin.
In this study, we mapped LULC around two of the major hydropower reservoirs located in the Magdalena River basin (Colombia) with a specific focus on understanding the dynamics of cash crops and dam construction. The Magdalena River basin is particularly cloud-prone due to advective cloud cover from the Andes mountains, resulting in data-scarce periods and regions, which further challenge assessments in these heterogeneous and dynamic landscapes (Ennen et al. Citation2021). We, therefore, used STMs to aggregate multi-year data into three periods for change assessment, relating to the construction phase of the dams, the operation onset, and the current state after 5 years of operation. The dam licensing inadequately addresses the potential impacts of the dams, considering only adjacent municipalities as the impacted ‘area of influence’ (AOI), using a single map representing the pre-construction phase at coarse spatial resolution and low thematic detail, and does not monitor LULCC for better alignment with other territorial planning (ENEL Citation2008; ISAGEN Citation2018). Recognizing that dams can have much broader impacts (Rufin et al. Citation2019), we select a larger area of interest that allows us to identify potential LULCC at larger spatial scales. We seek to answer three main research questions:
How well can we map complex LULC classes in the Magdalena River basin based on sparse Landsat time series?
What are the key LULCC trajectories in the reservoir area, in the dam’s area of influence (AOI), and beyond?
What are the dynamics of cash crops and how did the dam construction affect these?
2. Data & methods
2.1. Study area
We focus on two hydroelectric dams, El Quimbo, and Hidrosogamoso, in the Magdalena River basin in the Colombian Andes (). The region is characterized by a complex topography with gradients from southwest to northeast descending from 5,337 m.a.s.l. in the Colombian Andes to sea level in floodplain areas (Farr et al. Citation2007). Annual mean temperature and precipitation depend largely on the complex topography, varying from less than 8 to more than 28 °C (IDEAM Citation2014), and 1000 mm/year in the eastern to 5000 mm/year in the western part of the basin, respectively (IDEAM Citation2010b). The northern and central parts of the Magdalena basin experience a bimodal annual precipitation cycle with two wet periods (March – May, and October – November) (Restrepo and Escobar Citation2018). The basin is frequently impacted by El Niño - Southern Oscillation (ENSO) and La Nina events (Hoyos et al. Citation2013; Poveda et al. Citation2020). Unique natural vegetation types in the region include the paramos (Patiño et al. Citation2021) and numerous wetlands (Patino and Estupinan-Suarez Citation2016; Ricaurte et al. Citation2017).
Figure 1. Region of interest, (a) El Quimbo dam and (b) Hidrosogamoso, with AOI, Sub-basins upstream and downstream Magdalena River basin, and Andes region.

The agricultural land uses of the Magdalena basin are mainly extensive pastures of low productivity (Etter et al. Citation2006; Zuluaga et al. Citation2021), palm oil, coffee, and rice producing (IDEAM Citation2023). El Quimbo and Hidrosogamoso, located in Huila and Santander departaments, are dominated by different cash crops. In the El Quimbo region, coffee and rice are the most dominant. While rice is mainly produced for the domestic market, coffee targets the domestic and export markets. Hidrosogamoso features extensive oil palm plantations for the international market (Agronet Citation2021; USDA Citation2023a).
The El Quimbo and Hidrosogamoso dams were built after the introduction of a new legal framework in 2000 that introduced new guidelines for environmental and social sustainability in dam construction in Colombia. The construction of both dams started in 2009, Hidrosogamoso was commissioned in 2014 and El Quimbo in 2015, and both dams were in full operation in 2015. El Quimbo and Hidrosogamoso are together responsible for 16% of the national energy supply. The license documents of the dams define an AI comprising the municipalities where the dam is installed and the neighboring municipalities (ENEL Citation2008; ISAGEN Citation2018). Because downstream impacts of hydropower dams are often neglected due to the frequently long distances between dams and impacted areas, and the less obvious nature of downstream impacts (Baird et al. Citation2021), we here consider the catchment area that drains towards the dam (dams upstream) and the drainage basins downstream of the dam, including all eastern Andean municipalities in the area downstream (IDEAM Citation2013) summing up to 3.164.598,82 ha for El Quimbo, and 4.996.476,97 ha for Hidrosogamoso ().
2.2. Workflow
The methodology consists of seven main steps (), including pre-processing of the Landsat archive into STM, combining secondary map products and an active learning scheme to compile training data for model training, LULC classification, and area-adjusted accuracy assessment.
2.3. Landsat data preprocessing
We used all Landsat 5 TM, 7 ETM+, and 8 OLI Level 2, Collection 2, Tier 1 images available in Google Earth Engine (GEE) between 2007 and 2021. We utilized the Landsat quality assessment (QA) band to mask clouds, cloud shadows and dilated clouds based on the Fmask cloud detection (Qiu et al. Citation2019). Due to the high cloud cover and limited image availability in parts of the region, we aggregated observations across several years to reach a sufficient number of clear-observation counts for each period of interest. As the focus of our study is on the changes brought about by construction and operation of the hydropower reservoirs, we defined three periods aligning with major developments of these infrastructures, representing before, after and during construction. We integrated the years 2007–2011 for the target year 2009, the years 2013–2017 for the target year 2015 and the years 2018–2022 for the target year 2020. This resulted in 974 images for 2009, 2,075 for 2015, and 1,743 for 2020, leading to varying pixel-level clear observation counts across the study period and region ().
Figure 3. Pixel-wise count of clear observations for each aggregation period for (A) Hidrogamoso and (B) El Quimbo.

For each period, we calculated eight spectral-temporal metrics (maximum, minimum, median, standard deviation, 10th, 25th, 75th, and 90th percentile) from eight spectral indices (), three linear band transformations, and six Landsat spectral bands. We selected these indices as previous studies showed that they have the potential to pick up different aspects of data distributions in the feature space regarding our classes of interest. To incorporate pre-existing knowledge of crop distribution depending on topography (Zuluaga et al. Citation2021), we included elevation, slope, and aspect from the shuttle radar topography mission (SRTM) data (Farr et al. Citation2007). Overall, this resulted in a total of 139 features for each period.
Table 1. Spectral indices and authors.
See supplementary material (Fig. S1) for the variable importance of the final model.
2.4. Model training and classification
Following the regional characteristics of the land system and the expected change trajectories, we concentrated our analysis on nine classes: rice, oil palm plantations, other permanent crops, other temporary crops, grassland, non-agricultural vegetation, wetlands, water surfaces, and others ( and ).
Figure 4. Field photographs with examples of (a) rice cultivation, (b) oil palm cultivation, (c) grasslands, (d) non-agricultural vegetation, (e) water surface, (f) others, (g) other temporary crops, (h) other permanent crops and (i) wetlands were obtained by the authors during fieldwork in 2023.

Table 2. Class definitions.
To collect training points, we generated suitability maps for each class using secondary maps on forest cover (Hansen et al. Citation2013), water surface (Pickens et al. Citation2020), cropland (Potapov et al. Citation2021), palm oil (Descals et al. Citation2021), wetlands (SIAC Citation2020a), paramos (SIAC Citation2020b), and multiple LULC classes (González-González et al. Citation2022; IDEAM Citation2023) (Figs. S2 to S9 in the supplementary material). We further considered physical variables, such as the distance to water bodies, limiting suitable rice areas to regions of no more than 50 km distance, and slope in the case of grasslands used as pastures, which we assumed occur on slopes not exceeding 40%. (Zuluaga et al. Citation2021). We drew a stratified random sample (total n = 3,121) based on these maps and interpreted every point visually using very-high-resolution (VHR) images in Google Earth (GE). Uncertain or incorrect samples were removed, resulting in 1,623 labeled observations for 2020, 1,094 for 2015, and 404 for 2009. The suitability maps were used exclusively for model training, not for validation.
We extracted the STMs at our training locations and trained an initial Random Forest (RF) model in GEE using all 139 features (with 1,000 trees and trying variables at each split) (Belgiu and Drăguţ Citation2016; Probst et al. Citation2019). The RF model was used to predict LULC classes across the study regions due to its documented performance in numerous remote sensing studies (Belgiu and Drăguţ, Citation2016; Rodriguez-Galiano et al., Citation2012). Random Forest classifiers are robust in high dimensional and multicollinear feature spaces, their parametrization is straightforward, and they allow estimation of uncertainty at the pixel-level, which enabled the use of active learning for improving model performance in our study (Belgiu and Drăguţ, Citation2016). We iteratively added points in selected locations (e.g. misclassified areas) manually and complemented the training sample with two runs of an active learning routine. Active learning helps to add highly informative training samples based on the calculation of model-based uncertainty (Stumpf et al. Citation2014). Following the routine described in Rufin et al. (Citation2022a), we calculated probability margins derived from RF class probabilities as sampling strata to collect uncertain locations for training. Probability margins are calculated at the pixel level as the difference between the maximum class probability (i.e. class probability of the predicted class) and the second highest class probability. As such, large margins express high confidence scores, and low margins point to low model confidence. For each iteration, we used the class-wise 25th percentile of the distribution of probability margins in the study region to sample additional training points (20 points for each class) that were labeled using GE VHR imagery and PlanetScope mosaics provided through Norway’s International Climate and Forest Initiative (NICFI) data program (Planet Labs Inc Citation2020).
2.5. Area-adjusted accuracy assessment and area estimation
We followed the good practice recommendations for independent area-adjusted accuracy assessment based on a stratified random sample (Olofsson et al. Citation2014). We calculated the required sample size targeting a standard error of the overall accuracy (OA) of 1%, assuming user’s accuracies (UA) of 0.90 for the dominant classes, non-agricultural vegetation, and grasslands, and 0.85 for the other classes (Cochran Citation1977), yielding 937 samples. As we expected that generating reference labels for the classes of interest may be challenging given the limited availability of VHR imagery, we sampled a larger number of points (n = 2,559) for all years. We assigned at least 50 points per class and distributed the remaining samples according to class proportions, resulting in a higher number of samples for non-agricultural vegetation (n = 454), grasslands (n = 195), and others (n = 99).
All samples were interpreted using VHR imagery in GE and NICFI PlanetScope mosaics for the maps of 2015 and 2020. Samples were labeled independently by one experienced interpreter noting levels of confidence for the interpretation of each point for a second check by another experienced interpreter. Points flagged with high uncertainty were reexamined and labeled or deleted if no label could be assigned with certainty. We complemented the sample in four rounds of stratified random sampling to reach a sufficient number of validation samples. In total, we collected 1,396 for 2020, 1,392 for 2015, and 494 labeled samples for 2009. The relatively low number of labeled samples for the year 2009 was due to the scarcity of VHR imagery in parts of the study region, which increased standard errors in the estimation of accuracy and area. We generated a confusion matrix and derived area-adjusted OA, class-wise UA and PA, 95% confidence intervals, and error-adjusted area estimates from the reference data, using the mapac package for R v0.11 (Pflugmacher et al. Citation2019) using the estimators described in Stehman (Citation2014).
2.6. Quantifying LULCC
We quantified the overall net LULCC based on the error-adjusted area estimates and conducted a spatial analysis in different subsets of the study region to investigate change trajectories. First, we considered all the changes that occur just in the area flooded by the reservoir of the dams to capture the immediate (direct) effects of reservoir flooding. Second, we considered all the municipalities that appear on the license as AOI (, red area). Third, we analyzed LULCC trajectories in all municipalities in the upstream and downstream sub-basins of both dams, excluding the AOI (, blue and brown area).
3. Results
3.1. Accuracy assessment and area estimates
The area-adjusted UA, PA, and OA with corresponding standard errors were calculated for each year and each class (). The maps achieved satisfactory OA in 2020 (80.7%, ± 2.0%) and 2015 (80.0% ± 2.1%) and a medium OA (71.6% ± 3.9%) in 2009. UA ranging above 70% and partly exceeding 90% were registered for rice, palm oil, grasslands, non-agricultural vegetation, others, wetlands, and water surfaces. Producer’s accuracies higher than 70% and partly above 90% were registered for rice, palm oil, non-agricultural vegetation, water surface, and wetlands. On the contrary, temporary and permanent crops had low UA and PA across the years, indicating high omission errors for both classes.
Table 3. Confusion matrix for 2020 populated with probability scores, including UA and PA reported with standard errors.
Table 4. Confusion matrix for 2015 populated with probability scores, including UA and PA reported with standard errors.
Table 5. Confusion matrix for 2009 populated with probability scores, including UA and PA reported with standard errors.
The confusion matrices reveal the dominant error types for each map (). We observed high rates of confusion between permanent crops and non-agricultural vegetation, causing the above-mentioned omission errors for permanent crops. The dominant error types were similar across the three periods, except for the confusion between temporary crops and non-agricultural vegetation, which was particularly high in 2009, translating into the low producer’s accuracy for temporary crops.
Our error-adjusted area estimates reveal the extent of the target classes across the entire study region. Between the years the LULC classes changed dynamically but considering the whole period (2009–2020) the net changes were the decrease of wetlands, rice, grassland, temporary crops, and permanent crops, and an increase of water surface, non-agricultural vegetation, and palm oil ().
3.2. Spatial analysis of LULCC
The final LULC maps show the respective land cover according to our class catalog for the years 2009, 2015, and 2020 ().
According to our maps, the flooded area replaced by water surface in 2020 covered a total of 5,078.03 ha for Hidrosogamoso and 5,820.86 ha in the El Quimbo study region. For Hidrosogamoso, the flooded area dominantly replaced non-agricultural vegetation (77,9%) and grassland (14,3%) (). The classes with the largest losses in the El Quimbo study region were rice (10,6%), grassland (25,9%), non-agricultural vegetation (47,7%), other (8,0%), and temporary crops (7,1%).
Figure 7. Alluvial plots showing the LULC trajectories for the reservoir areas hidrosogamoso (left) and El Quimbo (right) in 2020 for both study regions. The colors of the connecting flows correspond to the class in 2009.

LULCC in the AOI covered 0.7 Mha, or 14% of the Hidrosogamoso study area, and 0.2 Mha, or 6,3%, of the El Quimbo study region. Beyond the AOI, the changes in the Hidrosogamoso study area covered 4,2 Mha, and 2,7 Mha for the El Quimbo. The distribution of each LULC per year can be observed in .
Figure 8. Alluvial plots showing the LULC trajectories of all changing pixels for (A) inside and (B) outside the AOI for the hidrosogamoso (left) and El quimbo (right) study region. Change trajectories below 10,000 pixels are omitted due to visualization purposes. The colors of the connecting flows correspond to the class in 2009.

In Hidrosogamoso, oil palm and grasslands suffered a loss inside and outside the AOI in both periods. Water surface decreased inside and outside the AOI in the first period, and increased in the second period. The other class decreased in both periods and spaces. Temporary and permanent crops increase and then decrease inside and outside the AI. Wetlands lost area in all regions and periods, despite in 2009–2015 in the AOI (). For El Quimbo, the rice areas suffered a decrease in the two periods and areas (inside and outside the AOI). The same happened for the other class. Grassland suffered decreases in all spaces and periods, despite 2009–2015. Temporary and permanent crops increase and then decrease for the influence area, and outside the influence area the first one decreases and then increases, and the second increases in both periods.
Table 6. Map-based LULC % change between the maps inside and outside the influence area.
3.3. Spatial analysis of cash crops
According to our maps, agricultural areas (i.e. the combination of temporary crops, permanent crops, rice, palm oil, and grassland) occupied 25% of the area in El Quimbo and 28% in the case of Hidrosogamoso region in 2020. This percentage increased between 2009–2015, mainly in the El Quimbo region, and maintained stable afterwards. For both cases, the predominant agricultural use was grassland (65% in El Quimbo and 81% in Hidrosogamoso), but note that this class includes unmanaged grassland as well. The grasslands were replaced over the three years mainly by palm oil in the case of the Hidrosogamoso and permanent crops in the case of El Quimbo. At the same time, our maps revealed a substantial decline in temporary crops. In the El Quimbo region, both downstream and upstream, this decrease reached 51,0%, between 2015 and 2020, and temporary crops were mainly replaced by water surfaces and grasslands ().
Figure 9. Examples of (A) areas flooded by the Hidrosogamoso reservoir, (B) areas flooded by the El Quimbo reservoir, (C) expansion of palm oil plantations at the expense of wetlands and grasslands, and D) palm oil areas affected by the disease. VHR imagery for 2009 in A (2009/12/05), B (2013/02/15), and C (2009/10/09) provided by ©maxar 2023 in Google Earth pro TM, VHR imagery for 2015 and 2020 in A (2016/06-11, 2020/03), B (2015/12, 2020/08), C (2016/12-2017/05, 2020/06-08), and D (2017/01/25, 2020/01) provided by Planet Labs Inc. ©2020.

The flooding of the Hidrosogamoso reservoir did not impact cash crops substantially, with most flooded agricultural lands being grasslands, and few temporary crop fields (). Palm oil covered the largest share of the agricultural area in the Hidrosogamoso region comprising 69.0% of the agricultural area in 2020. The palm oil area decreased between 2009 and 2015 but increased between 2015 and 2020. Palm oil has mainly replaced wetlands and grasslands (), with the former losing a substantial portion of their area between 2009 and 2015. On the other hand, palm oil has been replaced by non-agricultural vegetation (). Regarding cash crops in the El Quimbo region, rice and permanent crops dominated, occupying 37.2% and 50.3% respectively of the agricultural area in 2020. Rice areas decreased throughout the analysis period, particularly in the areas flooded by the reservoir ().
4. Discussion
4.1. Dam construction and land use/cover changes
We assessed LULC maps for three different periods to capture changes at different phases (construction, operation, present) and regions (flooded areas, licensed AOI, and beyond). We analyzed the changes in different spatial subsets of the region, to assess the changes beyond the areas of direct influence, and at the basin level.
The predominant classes in both study areas were non-agricultural vegetation and pastures. The presence of non-agricultural vegetation in much of both areas was also a pattern highlighted by González-González et al. (Citation2022) in their mapping of the Andes region in 2018. Tendencies for forest regrowth or secondary vegetation succession were also found by Rubiano et al. (Citation2017) in other parts of the Magdalena River basin. In this study, secondary vegetation mainly replaced marginal agricultural areas, while clearing dynamics were observed close to roads and urban areas. Pastures represented more than 70% of agricultural land uses, further reflecting the relevance of this extensive land use across Colombia (Etter et al. Citation2006; Zuluaga et al. Citation2021). In El Quimbo, reservoir flooding strongly affected agricultural land uses, contrary to Hidrosogamoso where non-agricultural vegetation occupied more than 75% of the flooded area; a pattern which is also confirmed by the license documents (ENEL Citation2008). The El Quimbo dam was constructed only 15 km upstream of the Betania dam, which was built as a multi-purpose dam in the 1960s. The joint use for irrigation and hydropower may have fostered the development of cash crops in this region as compared to the area surrounding Hidrosogamoso, where non-agricultural vegetation dominated, demonstrating how historic infrastructure expansion influences contemporary changing dynamics (De Andrade et al., Citation2021; De Oliveira Serrão et al., Citation2020).
Reservoir operation is linked to maintaining reservoir water levels according to the national energy demand. Thus, floodgates are closed or opened at different time periods and across seasons, affecting the river discharge and consequently water availability and accessibility downstream of the dams. As a consequence, we reason that the observed changes in wetlands and agricultural uses may relate to changes in the river flow controlled by the floodgates, providing additional insights into the interplay between dam commissioning, wetland conditions (Angarita et al. Citation2018) and downstream livelihoods (Baird et al. Citation2021). For Hidrosogamoso, wetlands areas decreased drastically (42,6%) between 2009 and 2015. This class was mostly replaced by grasslands downstream of the dams and in the influence area. Grassland expansion has been identified as an additional driver of wetland loss (Patino and Estupinan-Suarez Citation2016; Ricaurte et al. Citation2017), especially since this conversion is economically encouraged at the department level (Castiblanco et al. Citation2015; Ocampo-Peñuela et al. Citation2018).
For Hidrosogamoso, in 2015, a reservoir planning tool regulating competing land use interests around the reservoirs was published (‘Embalse Topocoro: Plan de Ordenamiento Central Hidroelétrica Sogamoso’). The document was elaborate by Fundación Humedales and ISAGEN, with the participation of social organizations. This instrument was required by Autoridad Nacional de Licencias Ambientales-ANLA (Resolution 1497/2009). LULC maps, as produced in this study, provide means to directly support management in the absence of planning instruments for El Quimbo, and enable modelling of related social, cultural, and economic drivers and consequences.
4.2. Cash crops, natural resources, and drivers of change
Colombia is the largest palm-oil producer in Latin America and the fourth-largest in the world (USDA Citation2023a). Palm oil dominates agricultural production in the Hidrosogamoso region, where oil palm cultivation was affected by a disease (pudrición del cogollo) starting in 2007 until 2013 (Rincón-Romero et al. Citation2021). Affected plantations were abandoned, until, in 2016, the disease could be controlled following technological developments and breeding of resistant species (Cabrera et al. Citation2020). The activation of formerly abandoned plantations has been captured by our maps with oil palm areas in 2009 changing to non-agricultural vegetation in 2015, followed by a partial change back to palm oil in 2020 (Figure 9D). Moreover, we identified municipalities with an increase in palm oil in the AOI, such as San Vicente de Chucuri, possibly related to the drastic price increase of palm oil in recent years (FEDEPALMA Citation2022). Currently, even small farmers have started to invest in palm oil. The creation and expansion of FUNDEPALMA, a federation dedicated only to smallholders, attest to these dynamics (Cabrera et al. Citation2020). These new oil palm plantations are established in proximity to rivers and are partly replacing wetlands, which is a new change trajectory as compared to the previous epoch, where oil palm mostly replaced grassland (Castiblanco et al. Citation2015).
Rice is among the most important cash crops in the El Quimbo region, occupying 34% of the agricultural area in 2020, which confirms the importance of Huila to national rice production (Agronet Citation2021). The areas concentrated near the main rivers and the dams remained stable during the three years. The retraction of rice areas evidenced in the maps occurred in the reservoir areas and areas at longer distances from water bodies, which are more vulnerable to changes in terms of water access. In 2010/2011, 23,759 ha of rice fields were impacted by La Nina, with a total loss of US$34.3 million, and in mid-2014, 33,938 ha were affected by El Niño-induced drought that led to crop losses valued at US$10.2 million (FEDEARROZ Citation2017). Recently, a retraction of rice areas has been documented in the Colombian Andes, which aligns with our findings (Castro-Llanos et al. Citation2019; Parra-Peña et al. Citation2022). Some of the remote rice areas were converted into water surfaces, which were visually identified as aquaculture areas. The Betania reservoir downstream of El Quimbo has been exploited by fish farming, following the initiatives by the departmental government to stimulate aquaculture production (Gobernación Huila Citation2006).
4.3. Uncertainties and limitations
Despite the data scarcity and dynamic nature of the Magdalena River basin, using multi-year STMs from Landsat images proved to be valuable for mapping LULC, even considering a complex class catalog. Our unbiased area estimates can be used for robust quantification of overall change dynamics in the region, while our maps are timely and due to the enhanced thematic detail in the agriculture domain, allow for more nuanced analyses of LULCC processes in the water-energy-food nexus, as compared to existing global and regional LULC products. Despite these advances, we identified remaining limitations, relating to limitations in data availability, the Landsat spatial resolution, and our multi-year aggregation strategy, which may have compromised the quality of our maps in certain areas. For specific periods and regions, we noted a low observation count and a seasonally clustered distribution of cloud-free observations, which likely reduced the distinguishability of the class-specific spectral-temporal behavior, mostly outside of the dams’ AOI.
Our area-adjusted accuracy assessment allowed for identifying the key error types, which in our case were commission errors of non-agricultural vegetation at the expense of temporary and permanent crops. The main permanent crop in the study region is coffee, which is mainly planted in oldgrown plantations (USDA Citation2023b), in agroforestry systems (Gomes et al. Citation2020), often in conjunction with other permanent crops such as banana or avocado (see ), which makes it appear similar to non-agricultural vegetation in the spectral-temporal feature space. Similarly, temporary crops have a high intra-class variability, while at the same time being spatially fragmented, and thus difficult to identify at Landsat spatial resolution. The limited data density required a multi-year aggregation strategy, which may have caused misclassifications for dynamic LULC types, including cropland-grassland rotations. On the contrary, the multi-year aggregation strategy is unlikely to affect rather stable LULC classes, such as rice. Such land uses require substantial land preparation and are often bound to the availability of surface water from rivers and streams for irrigation (Ochoa-Brito et al., Citation2023).
5. Conclusion
This study demonstrated that even in a data-scarce context like in Colombia, regional LULC maps derived from Landsat time series revealed changes across large regions and decadal time frames at high thematic detail. Integrating existing LULC products and iterative active learning proved to be a valuable tool to enhance training data collection, especially for complex classes in the agricultural domain. Our study provides further evidence that hydropower dams can directly affect LULC in different phases (construction and operation). Indirect effects can occur at spatial scales beyond the direct areas of influence, however, linking observed LULCC to the construction or operation of dams remains non-trivial, considering the interactions between multiple drivers of change (climate, social, cultural, economic). We thus argue that direct and indirect LULCC impacts need to be assessed and explicitly internalized in the dam’s environmental impact assessment and monitoring to be aligned with other territorial and policy instruments in order to decrease possible environmental and social inequalities.
Supplemental Material
Download PDF (1.9 MB)Acknowledgements
This work was supported by the project ‘Water Security for Whom’, a collaboration between Humboldt-Universität zu Berlin (Germany), Pontificia Universidad Javeriana (Colombia), and Universidade Federal de Minas Gerais (Brazil). The project is funded by VolkswagenStiftung as part of the initiative ‘Global Issues – Integrating Different Perspectives on Social Inequality’ (project n. 96955). The authors would like to thank the ‘Water Security for Whom’ team, and the agricultural federations Federación Nacional de Arroceros (FEDEARROZ), Federación Nacional de Cultivadores de Palma de Aceite (FEDEPALMA), Federación Nacional de Cacaoteros (FEDECACAO) and Federación Nacional de Cafeteros de Colombia for meetings and checkings on the LULC maps during the fieldwork in Huila and Santander departments in Colombia in 2023. PR acknowledges funding by the F.R.S.-FNRS, grant no. T.0154.21.
Disclosure statement
No potential conflict of interest was reported by the author(s).
Additional information
Funding
Notes on contributors
Caroline Salomão
Caroline Salomão contributed to Conceptualization, Methodology, Data curation, Software, Validation, Formal analysis, Investigation, Writing- Original draft preparation. Jonas Alsleben contributed to Methodology, Data curation, Software, Validation, Formal analysis, Writing-Review & Editing. Philippe Rufin contributed to Methodology, Writing-Review & Editing. Patrick Hostert contributed to Conceptualization, Writing-Review & Editing, Supervision.
Jonas Alsleben
Caroline Salomão contributed to Conceptualization, Methodology, Data curation, Software, Validation, Formal analysis, Investigation, Writing- Original draft preparation. Jonas Alsleben contributed to Methodology, Data curation, Software, Validation, Formal analysis, Writing-Review & Editing. Philippe Rufin contributed to Methodology, Writing-Review & Editing. Patrick Hostert contributed to Conceptualization, Writing-Review & Editing, Supervision.
Philippe Rufin
Caroline Salomão contributed to Conceptualization, Methodology, Data curation, Software, Validation, Formal analysis, Investigation, Writing- Original draft preparation. Jonas Alsleben contributed to Methodology, Data curation, Software, Validation, Formal analysis, Writing-Review & Editing. Philippe Rufin contributed to Methodology, Writing-Review & Editing. Patrick Hostert contributed to Conceptualization, Writing-Review & Editing, Supervision.
Patrick Hostert
Caroline Salomão contributed to Conceptualization, Methodology, Data curation, Software, Validation, Formal analysis, Investigation, Writing- Original draft preparation. Jonas Alsleben contributed to Methodology, Data curation, Software, Validation, Formal analysis, Writing-Review & Editing. Philippe Rufin contributed to Methodology, Writing-Review & Editing. Patrick Hostert contributed to Conceptualization, Writing-Review & Editing, Supervision.
References
- Agronet. 2021. Participación Departamental en la Producción y en el Área Cosechada. https://agronet.gov.co/estadistica/Paginas/home.aspx?cod=2.
- Angarita H, Wickel AJ, Sieber J, Chavarro J, Maldonado-Ocampo JA, Herrera-R GA, Delgado J, Purkey D. 2018. Basin-scale impacts of hydropower development on the Mompós Depression wetlands, Colombia. Hydrol Earth Syst Sci. 22(5):2839–2865. doi:10.5194/hess-22-2839-2018.
- Baird IG, Silvano RAM, Parlee B, Poesch M, Maclean B, Napoleon A, Lepine M, Hallwass G. 2021. The Downstream Impacts of hydropower dams and indigenous and local knowledge: examples from the peace–Athabasca, Mekong, and Amazon. Environ. Environ Manage. 67(4):682–696. doi:10.1007/s00267-020-01418-x.
- Belgiu M, Drăguţ L. 2016. Random forest in remote sensing: a review of applications and future directions. ISPRS J Photogramm Remote Sens. 114:24–31. doi:10.1016/j.isprsjprs.2016.01.011.
- Boelens R, Hoogesteger J, Swyngedouw E, Vos J, Wester P. 2016. Hydrosocial territories: a political ecology perspective. Water Int. 41(1):1–14. doi:10.1080/02508060.2016.1134898.
- Cabrera OA, Rincon-Romero VO, Ospina ME, Arias NA. 2020. Palma Aceite En Puerto Wilches Actores Procesos Transform (1960–2016). Anuario de Historia Regional y de las Fronteras. 26(1). doi:10.18273/revanu.v26n1-2021004.
- Castiblanco C, Etter A, Ramirez A. 2015. Impacts of oil palm expansion in Colombia: what do socioeconomic indicators show? Land Use Policy. 44:31–43. doi:10.1016/j.landusepol.2014.10.007.
- Castro-Diaz L, García MA, Villamayor-Tomas S, Lopez MC. 2023. Impacts of hydropower development on locals’ livelihoods in the Global South. World Dev. 169:106285. doi:10.1016/j.worlddev.2023.106285.
- Castro-Llanos F, Hyman G, Rubiano J, Ramirez-Villegas J, Achicanoy H. 2019. Climate change favors rice production at higher elevations in Colombia. Mitig Adapt Strateg Glob Change. 24(8):1401–1430. doi:10.1007/s11027-019-09852-x.
- Chowdhury S, Chao DK, Shipman TC, Wulder MA. 2017. Utilization of Landsat data to quantify land-use and land-cover changes related to oil and gas activities in West-Central Alberta from 2005 to 2013. GIScience Remote Sens. 54(5):700–720. doi:10.1080/15481603.2017.1317453.
- Chowdhury S, Peddle DR, Wulder MA, Heckbert S, Shipman TC, Chao DK. 2021. Estimation of land-use/land-cover changes associated with energy footprints and other disturbance agents in the Upper Peace Region of Alberta Canada from 1985 to 2015 using Landsat data. Int. J. Appl. Earth Obs. Geoinformation. 94:102224. doi:10.1016/j.jag.2020.102224.
- Cochran WG. 1977. Sampling techniques. 3rd ed. Newyork (NY): John Wiley & Sons.
- Craven J, Angarita H, Corzo Perez GA, Vasquez D. 2017. Development and testing of a river basin management simulation game for integrated management of the Magdalena-Cauca river basin. Environ Model Softw. 90:78–88. doi:10.1016/j.envsoft.2017.01.002.
- Crist EP. 1985. A TM Tasseled cap equivalent transformation for reflectance factor data. Remote Sens Environ. 17(3):301–306. doi:10.1016/0034-4257(85)90102-6.
- Dakos V, Matthews B, Hendry AP, Levine J, Loeuille N, Norberg J, Nosil P, Scheffer M, De Meester L. 2019. Ecosystem tipping points in an evolving world. Nat Ecol Evol. 3(3):355–362. doi:10.1038/s41559-019-0797-2.
- DANE. 2022. Boletín Técnico – Cuentas departamentales -Producto Interno Bruto por departamento preliminar 2021. https://www.dane.gov.co/files/investigaciones/pib/departamentales/B_2015/Bol_PIB_dptal_2021preliminar.pdf.
- De Andrade BCC, De Andrade Pinto EJ, Ruhoff A, Senay GB. 2021. Remote sensing-based actual evapotranspiration assessment in a data-scarce area of Brazil: a case study of the Urucuia Aquifer System. Int J Appl Earth Obs Geoinformation. 98:102298. doi:10.1016/j.jag.2021.102298.
- De Oliveira Serrão EA, Silva MT, Ferreira TR, De Paulo Rodrigues Da Silva V, De Salviano De Sousa F, De Lima AMM, De Ataide LCP, Wanzeler RTS. 2020. Land use change scenarios and their effects on hydropower energy in the Amazon. Sci Total Environ. 744:140981. doi:10.1016/j.scitotenv.2020.140981.
- Deines JM, Kendall AD, Hyndman DW. 2017. Annual Irrigation Dynamics in the U.S. Northern High Plains Derived from Landsat Satellite Data. Geophys. Res Lett. 44(18):9350–9360. doi:10.1002/2017GL074071.
- Descals A, Wich S, Meijaard E, Gaveau DLA, Peedell S, Szantoi Z. 2021. High-resolution global map of smallholder and industrial closed-canopy oil palm plantations. Earth Syst Sci Data. 13(3):1211–1231. doi:10.5194/essd-13-1211-2021.
- Deteix L, Salou T, Drogué S, Loiseau E. 2023. The importance of land in resource criticality assessment methods: a first step towards characterising supply risk. Sci Total Environ. 880:163248. doi:10.1016/j.scitotenv.2023.163248.
- Domínguez E, Rivera H. 2010. A Fokker–Planck–Kolmogorov equation approach for the monthly affluence forecast of Betania hydropower reservoir. J Hydroinformatics. 12(4):486–501. doi:10.2166/hydro.2010.083.
- ENEL. 2008. Estudio de Impacto Ambiental. https://www.enel.com.co/content/dam/enel-co/espa%C3%B1ol/sobre_enel/generaci%C3%B3n/central-hidroelectrica-el-quimbo/Estudio_de_impacto_ambiental.pdf.
- Ennen JR, Agha M, Sweat SC, Matamoros WA, Lovich JE, Iverson JB, Rhodin AGJ, Thomson RC, Shaffer HB, Hoagstrom CW. 2021. A watershed moment: analysis of sub-basins refocuses the geography of turtle conservation across the globe. Biol Conserv. 253:108925. doi:10.1016/j.biocon.2020.108925.
- Etter A, McAlpine C, Wilson K, Phinn S, Possingham H. 2006. Regional patterns of agricultural land use and deforestation in Colombia. Agric Ecosyst Environ. 114(2–4):369–386. doi:10.1016/j.agee.2005.11.013.
- Farr TG, Rosen PA, Caro E, Crippen R, Duren R, Hensley S, Kobrick M, Paller M, Rodriguez E, Roth L, et al. 2007. The Shuttle Radar Topography Mission. Rev Geophys. 45(2):RG2004. doi:10.1029/2005RG000183.
- FEDEARROZ. 2017. IV CENSO NACIONAL ARROCERO 2016. https://fedearroz.s3.amazonaws.com/media/documents/Libro_Censo_General.pdf.
- FEDEPALMA. 2022. Statistical Year Book 2022 - The Oil Palm Agroindustry in Colombia and the World 2017-2021. https://publicaciones.fedepalma.org/index.php/anuario/issue/view/1620/178.
- Gamon JA, Huemmrich KF, Wong CYS, Ensminger I, Garrity S, Hollinger DY, Noormets A, Peñuelas J. 2016. A remotely sensed pigment index reveals photosynthetic phenology in evergreen conifers. Proc Natl Acad Sci U S A. 113(46):13087–13092. doi:10.1073/pnas.1606162113.
- Gao B-C. 1996. NDWI a normalized difference water index for remote sensing of vegetation liquid water from space. Remote Sens Environ. 58(3), 257–266.
- Gobernación Huila C. 2006. Plano de Ordenamiento Pesquero de Betania-POPA. https://www.bing.com/ck/a?!&&p=65e066e05bc2fb85JmltdHM9MTY5MDg0ODAwMCZpZ3VpZD0xNGRmYTlhZC0xMzg2LTY5M2QtMDM5NC1iOGNmMTI4ZTY4NWEmaW5zaWQ9NTE3OQ&ptn=3&hsh=3&fclid=14dfa9ad-1386-693d-0394-b8cf128e685a&psq=(Plano+de+Ordenamiento+Pesquero+de+Betania-POPA%2c+2006).&u=a1aHR0cHM6Ly93d3cuaHVpbGEuZ292LmNvL2xvYWRlci5waHA_bFNlcnZpY2lvPVRvb2xzMiZsVGlwbz1kZXNjYXJnYXMmbEZ1bmNpb249ZGVzY2FyZ2FyJmlkRmlsZT03MzI2&ntb=1.
- Gomes LC, Bianchi FJJA, Cardoso IM, Fernandes RBA, Filho EIF, Schulte RPO. 2020. Agroforestry systems can mitigate the impacts of climate change on coffee production: a spatially explicit assessment in Brazil. Agric Ecosyst Environ. 294:106858. doi:10.1016/j.agee.2020.106858.
- González-González A, Clerici N, Quesada B. 2022. A 30 m-resolution land use-land cover product for the Colombian Andes and Amazon using cloud-computing. Int J Appl Earth Obs Geoinformation. 107:102688. doi:10.1016/j.jag.2022.102688.
- Griffiths P, Van Der Linden S, Kuemmerle T, Hostert P. 2013. A pixel-based Landsat compositing algorithm for large area land cover mapping. IEEE J Sel Top Appl Earth Observations Remote Sensing. 6(5):2088–2101. doi:10.1109/JSTARS.2012.2228167.
- Hansen MC, Potapov PV, Moore R, Hancher M, Turubanova SA, Tyukavina A, Thau D, Stehman SV, Goetz SJ, Loveland TR, et al. 2013. High-resolution global maps of 21st-century forest cover change. Science. 342(6160):850–853. doi:10.1126/science.1244693.
- Hemmerling J, Pflugmacher D, Hostert P. 2021. Mapping temperate forest tree species using dense Sentinel-2 time series. Remote Sens Environ. 267:112743. doi:10.1016/j.rse.2021.112743.
- Hoyos N, Escobar J, Restrepo JC, Arango AM, Ortiz JC. 2013. Impact of the 2010–2011 La Niña phenomenon in Colombia, South America: the human toll of an extreme weather event. Appl Geogr. 39:16–25. doi:10.1016/j.apgeog.2012.11.018.
- Huang C, Wylie B, Yang L, Homer C, Zylstra G. 2002. Derivation of a tasselled cap transformation based on Landsat 7 at-satellite reflectance. Int J Remote Sens. 23(8):1741–1748. doi:10.1080/01431160110106113.
- Huang S, Tang L, Hupy JP, Wang Y, Shao G. 2021. A commentary review on the use of normalized difference vegetation index (NDVI) in the era of popular remote sensing. J for Res. 32(1):1–6. doi:10.1007/s11676-020-01155-1.
- Huete A, Didan K, Miura T, Rodriguez EP, Gao X, Ferreira LG. 2002. Overview of the radiometric and biophysical performance of the MODIS vegetation indices. Remote Sens. Environ. 83:195–213. doi:10.1016/S0034-4257(02)00096-2.
- Huete AR. 1988. A soil-adjusted vegetation index (SAVI). Remote Sens Environ. 25(3):295–309. doi:10.1016/0034-4257(88)90106-X.
- Huete AR, Didan K, Shimabukuro Y, Ratana P, Saleska S, Hutyra L, Yang W, Nemani R, Myneni R. 2006. Amazon rainforest green-up with sunlight in dry season. Geophys. Res. Lett. 33(6):L06405. doi:10.1029/2005GL025583.
- Ibrahim ES, Rufin P, Nill L, Kamali B, Nendel C, Hostert P. 2021. Mapping crop types and cropping systems in Nigeria with Sentinel-2 imagery. Remote Sens. 13(17):3523. doi:10.3390/rs13173523.
- IDEAM. 2023. Monitoreo y seguimiento de suelos y tierras. Estado Coberturas Tierra. Cobertura de la Tierra 100K Periodo. http://www.ideam.gov.co/capas-geo.
- IDEAM. 2014. Distribución de la temperatura media anual (°c). Promedio multianual 1981 – 2010. http://atlas.ideam.gov.co/basefiles/Temp_Med_Anual.pdf.
- IDEAM. 2013. Zonificación y codificación de uniades hidrográficas e hidrogeológicas de Colombia. http://documentacion.ideam.gov.co/openbiblio/bvirtual/022655/MEMORIASMAPAZONIFICACIONHIDROGRAFICA.pdf.
- IDEAM. 2010a. Leyenda Nacional de Coberturas de la Tierra. Metodología CORINE Land Cover adaptada para Colombia Escala 1:100.000. Instituto de Hidrología, Meteorología y Estudios Ambientales. Bogotá, D. C. https://www.researchgate.net/publication/303960063_LEYENDA_NACIONAL_DE_COBERTURAS_DE_LA_TIERRA_METODOLOGIA_CORINE_LAND_COVER_ADAPTADA_PARA_COLOMBIA_ESCALA_1100000.
- IDEAM. 2010b. Atlas Climatológico da Colombia (1981–2010). http://atlas.ideam.gov.co/visorAtlasClimatologico.html.
- IEA. 2022. International energy agency (IEA). France: World Energy Outlook 2022.
- ISAGEN. 2018. https://livejaverianaedu-my.sharepoint.com/personal/laura-pulgarin_javeriana_edu_co/_layouts/15/onedrive.aspx?ga=1&id=/personal/laura%2Dpulgarin%5Fjaveriana%5Fedu%5Fco/Documents/Universidad%20Javeriana/Licencia%20Ambiental%20Hidrosogamoso/2%2E%20Complemento%20EIA%5FAnexos/Complemento%5FEIA%5FLAM0237%5FJun%5F2019%5FVF%2Epdf&parent=/personal/laura%2Dpulgarin%5Fjaveriana%5Fedu%5Fco/Documents/Universidad%20Javeriana/Licencia%20Ambiental%20Hidrosogamoso/2%2E%20Complemento%20EIA%5FAnexos.
- Jakimow B, Griffiths P, Van Der Linden S, Hostert P. 2018. Mapping pasture management in the Brazilian Amazon from dense Landsat time series. Remote Sens Environ. 205:453–468. doi:10.1016/j.rse.2017.10.009.
- Kaufman YJ, Tanre D. 1992. Atmospherically resistant vegetation index (ARVI) for EOS-MODIS. IEEE Trans Geosci Remote Sens. 30(2):261–270. doi:10.1109/36.134076.
- Key CH, Benson NC. 2006. Landscape Assessment (LA) Sampling and Analysis Methods. USDA Forest Service Gen. Tech Rep. RMRS-GT R-164-CD.
- Kowalski K, Okujeni A, Brell M, Hostert P. 2022. Quantifying drought effects in Central European grasslands through regression-based unmixing of intra-annual Sentinel-2 time series. Remote Sens Environ. 268:112781. doi:10.1016/j.rse.2021.112781.
- Lohani S, Dilts T, Weisberg P, Null S, Hogan Z. 2020. Rapidly Accelerating Deforestation in Cambodia’s Mekong River Basin: a Comparative Analysis of Spatial Patterns and Drivers. Water. 12(8):2191. doi:10.3390/w12082191.
- Mapbiomas. 2021. Colecciones de mapbiomas amazonía. https://amazonia.mapbiomas.org/mapas-de-la-coleccion.
- Müller H, Rufin P, Griffiths P, Barros Siqueira AJ, Hostert P. 2015. Mining dense Landsat time series for separating cropland and pasture in a heterogeneous Brazilian savanna landscape. Remote Sens Environ. 156:490–499. doi:10.1016/j.rse.2014.10.014.
- Munroe DK, Müller D. 2020. Land-system science to support achieving the sustainable development goals. J Land Use Sci. 15(4):477–481. doi:10.1080/1747423X.2020.1783085.
- Myers N, Mittermeier RA, Mittermeier CG, Da Fonseca GAB, Kent J. 2000. Biodiversity hotspots for conservation priorities. Nature. 403(6772):853–858. doi:10.1038/35002501.
- Nill L, Grünberg I, Ullmann T, Gessner M, Boike J, Hostert P. 2022. Arctic shrub expansion revealed by Landsat-derived multitemporal vegetation cover fractions in the Western Canadian Arctic. Remote Sens Environ. 281:113228. doi:10.1016/j.rse.2022.113228.
- Ocampo-Peñuela N, Garcia-Ulloa J, Ghazoul J, Etter A. 2018. Quantifying impacts of oil palm expansion on Colombia’s threatened biodiversity. Biol Conserv. 224:117–121. doi:10.1016/j.biocon.2018.05.024.
- Ochoa-Brito JI,Ghosh A,Hijmans RJ. 2023. Cropland expansion in Ecuador between 2000 and 2016. PLoS One. 18(9):e0291753. doi:10.1371/journal.pone.0291753.
- Olofsson P, Foody GM, Herold M, Stehman SV, Woodcock CE, Wulder MA. 2014. Good practices for estimating area and assessing accuracy of land change. Remote Sens Environ. 148:42–57. doi:10.1016/j.rse.2014.02.015.
- Parra-Peña R, Flórez S, Rodriguez D. 2022. La competitividad de la cadena del arroz en Colombia: un compromiso con el bienestar del agricultor. http://hdl.handle.net/11445/4237.
- Patino JE, Estupinan-Suarez LM. 2016. Hotspots of Wetland Area Loss in Colombia. Wetlands. 36(5):935–943. doi:10.1007/s13157-016-0806-z.
- Patiño S, Hernández Y, Plata C, Domínguez I, Daza M, Oviedo-Ocaña R, Buytaert W, Ochoa-Tocachi BF. 2021. Influence of land use on hydro-physical soil properties of Andean páramos and its effect on streamflow buffering. CATENA. 202:105227. doi:10.1016/j.catena.2021.105227.
- Pendrill F, Persson UM, Godar J, Kastner T. 2019a. Deforestation displaced: trade in forest-risk commodities and the prospects for a global forest transition. Environ Res Lett. 14(5):055003. doi:10.1088/1748-9326/ab0d41.
- Pendrill F, Persson UM, Godar J, Kastner T, Moran D, Schmidt S, Wood R. 2019b. Agricultural and forestry trade drives large share of tropical deforestation emissions. Glob Environ Change 56:1–10. doi:10.1016/j.gloenvcha.2019.03.002.
- Pflugmacher D, Rabe A, Peters M, Hostert P. 2019. Mapping pan-European land cover using Landsat spectral-temporal metrics and the European LUCAS survey. Remote Sens Environ. 221:583–595. doi:10.1016/j.rse.2018.12.001.
- Pickens AH, Hansen MC, Hancher M, Stehman SV, Tyukavina A, Potapov P, Marroquin B, Sherani Z. 2020. Mapping and sampling to characterize global inland water dynamics from 1999 to 2018 with full Landsat time-series. Remote Sens Environ. 243:111792. doi:10.1016/j.rse.2020.111792.
- Planet Labs Inc. 2020. Norway’s International Climate and Forest Initiative (NICFI) data program. https://www.planet.com/nicfi/.
- Potapov P, Turubanova S, Hansen MC, Tyukavina A, Zalles V, Khan A, Song X-P, Pickens A, Shen Q, Cortez J. 2021. Global maps of cropland extent and change show accelerated cropland expansion in the twenty-first century. Nat Food. 3(1):19–28. doi:10.1038/s43016-021-00429-z.
- Poveda G, Espinoza JC, Zuluaga MD, Solman SA, Garreaud R, Van Oevelen PJ. 2020. High Impact Weather Events in the Andes. Front Earth Sci. 8:162. doi:10.3389/feart.2020.00162.
- Probst P, Wright MN, Boulesteix A. 2019. Hyperparameters and tuning strategies for random forest. WIREs Data Min Knowl Discov. 9:e1301. doi:10.1002/widm.1301.
- Qiu S, Zhu Z, He B. 2019. Fmask 4.0: improved cloud and cloud shadow detection in Landsats 4–8 and Sentinel-2 imagery. Remote Sens Environ. 231:111205. doi:10.1016/j.rse.2019.05.024.
- Raihan A. 2023. The influences of renewable energy, globalization, technological innovations, and forests on emission reduction in Colombia. Innov Green Dev. 2(4):100071. doi:10.1016/j.igd.2023.100071.
- Restrepo JD, Escobar HA. 2018. Sediment load trends in the Magdalena River basin (1980–2010): anthropogenic and climate-induced causes. Geomorphology. 302:76–91. doi:10.1016/j.geomorph.2016.12.013.
- Ricaurte LF, Olaya-Rodríguez MH, Cepeda-Valencia J, Lara D, Arroyave-Suárez J, Max Finlayson C, Palomo I. 2017. Future impacts of drivers of change on wetland ecosystem services in Colombia. Glob Environ Change. 44:158–169. doi:10.1016/j.gloenvcha.2017.04.001.
- Richter BD, Postel S, Revenga C, Scudder T, Lehner B, Churchill A, Chow M. 2010. Lost in Development’s Shadow: the Downstream Human Consequences of Dams 3.
- Rincón-Romero V, Cabrera OA, Ospina ME, Arias NA. 2021. Revista Ciencia Tecnología Sociedad y Ambiente, 13. Spatial dynamics of oil palm crop in Puerto Wilches (1977–2017).
- Rodriguez-Galiano VF,Ghimire B,Rogan J,Chica-Olmo M,Rigol-Sanchez JP. 2012. An assessment of the effectiveness of a random forest classifier for land-cover classification. ISPRS J. Photogramm. Remote Sens. 67:93–104. doi:10.1016/j.isprsjprs.2011.11.002.
- Rosenberg DM, Mccully P, Pringle CM. 2000. Global-scale environmental effects of hydrological alterations: introduction. BioScience. 50(9):746. (2000)050[0746: GSEEOH]2.0.CO;2 doi:10.1641/0006-3568.
- Rubiano K, Clerici N, Norden N, Etter A. 2017. Secondary forest and shrubland dynamics in a highly transformed landscape in the Northern Andes of Colombia (1985–2015). Forests. 8(6):216. doi:10.3390/f8060216.
- Rufin P, Bey A, Picoli M, Meyfroidt P. 2022a. Large-area mapping of active cropland and short-term fallows in smallholder landscapes using PlanetScope data. Int J Appl Earth Obs Geoinf. 112:102937. doi:10.1016/j.jag.2022.102937.
- Rufin P, Gollnow F, Müller D, Hostert P. 2019. Synthesizing dam-induced land system change. Ambio. 48(10):1183–1194. doi:10.1007/s13280-018-01144-z.
- Rufin P, Levers C, Baumann M, Jägermeyr J, Krueger T, Kuemmerle T, Hostert P. 2018. Global-scale patterns and determinants of cropping frequency in irrigation dam command areas. Glob Environ Change. 50:110–122. doi:10.1016/j.gloenvcha.2018.02.011.
- Rufin P, Peña-Guerrero MD, Umirbekov A, Wei Y, Müller D. 2022b. Post-Soviet changes in cropping practices in the irrigated drylands of the Aral Sea basin. Environ Res Lett. 17(9):095013. doi:10.1088/1748-9326/ac8daa.
- SIAC. 2020a. Humedal Versión 3. https://siac-datosabiertos-mads.hub.arcgis.com/datasets/MADS::humedal-versi%C3%B3n-3/about.
- SIAC. 2020b. Páramos Delimitados Junio 2020. https://siac-datosabiertos-mads.hub.arcgis.com/datasets/9631ed8c44274baa824e6277276de48f/about.
- SINCHI. 2022. Coberturas de la Tierra de la Amazonia colombiana para el año 2022. Escala: 1:100.000. https://sinchi.maps.arcgis.com/home/item.html?id=775fe099cc974a868dd7463eb1e69474.
- Stehman SV. 2014. Estimating area and map accuracy for stratified random sampling when the strata are different from the map classes. Int J Remote Sens. 35(13):4923–4939. doi:10.1080/01431161.2014.930207.
- Stumpf A, Lachiche N, Malet JP, Kerle N, Puissant A. 2014. Active learning in the spatial domain for remote sensing image classification. IEEE Trans Geosci Remote Sens. 52(5):2492–2507. doi:10.1109/TGRS.2013.2262052.
- Tang Z, Adhikari H, Pellikka PKE, Heiskanen J. 2021. A method for predicting large-area missing observations in Landsat time series using spectral-temporal metrics. Int J Appl Earth Obs Geoinformation. 99:102319. doi:10.1016/j.jag.2021.102319.
- Treanor NB, Saunders J. 2021. Tackling (illegal) deforestation in coffee supply chains: what impact can demand-side regulations have? Forest Policy Trade and Finance Initiative Report.
- Tucker CJ. 1979. Red and photographic infrared linear combinations for monitoring vegetation. Remote Sens. Environ. 8(2):127–150. doi:10.1016/0034-4257(79)90013-0.
- Tulbure HP, Kuemmerle T, Broich M. 2021. Regional matters: On the usefulness of regional land-cover datasets in times of global change. Remote Sens. Ecol. Conserv. 8(3):272–283. doi:10.1002/rse2.248.
- Turner BL, Lambin EF, Verburg PH. 2021. From land-use/land-cover to land system science: this article belongs to Ambio’s 50th Anniversary Collection. Theme: Agricultural land use. Ambio. 50(7):1291–1294. doi:10.1007/s13280-021-01510-4.
- UPME. 2020. Plan Energético Nacional 2020-2050 La transformación energética que habilita el desarrollo sostenible. https://www1.upme.gov.co/DemandayEficiencia/Documents/PEN_2020_2050/Plan_Energetico_Nacional_2020_2050.pdf.
- USDA. 2023a. Graphical Query: stats By Commodity. https://apps.fas.usda.gov/psdonline/app/index.html#/app/statsByCommodity.
- USDA. 2023b. USDA - Required Report: required - Public Distribution - Coffe Annual - Colombia. https://apps.fas.usda.gov/newgainapi/api/Report/DownloadReportByFileName?fileName=Coffee%20Annual_Bogota_Colombia_CO2023-0012.
- Velastegui-Montoya A, Lima AD, Adami M. 2020. Multitemporal analysis of deforestation in response to the construction of the Tucuruí Dam. IJGI. 9(10):583. doi:10.3390/ijgi9100583.
- Waldner F, Canto GS, Defourny P. 2015. Automated annual cropland mapping using knowledge-based temporal features. ISPRS J Photogramm Remote Sens. 110:1–13. doi:10.1016/j.isprsjprs.2015.09.013.
- Woldemichael AT, Hossain F, Pielke R, Beltrán‐Przekurat A. 2012. Understanding the impact of dam‐triggered land use/land cover change on the modification of extreme precipitation. Water Resour Res. 48(9):2011WR011684. doi:10.1029/2011WR011684.
- Wulder MA, Loveland TR, Roy DP, Crawford CJ, Masek JG, Woodcock CE, Allen RG, Anderson MC, Belward AS, Cohen WB, et al. 2019. Current status of Landsat program, science, and applications. Remote Sens Environ. 225:127–147. doi:10.1016/j.rse.2019.02.015.
- Wulder MA, Roy DP, Radeloff VC, Loveland TR, Anderson MC, Johnson DM, Healey S, Zhu Z, Scambos TA, Pahlevan N, et al. 2022. Fifty years of Landsat science and impacts. Remote Sens Environ. 280:113195. doi:10.1016/j.rse.2022.113195.
- Wulder MA, White JC, Loveland TR, Woodcock CE, Belward AS, Cohen WB, Fosnight EA, Shaw J, Masek JG, Roy DP. 2016. The global Landsat archive: status, consolidation, and direction. Remote Sens Environ. 185:271–283. doi:10.1016/j.rse.2015.11.032.
- Xie H, Zhang Y, Zeng X, He Y. 2020. Sustainable land use and management research: a scientometric review. Landscape Ecol. 35(11):2381–2411. doi:10.1007/s10980-020-01002-y.
- Xu R, Zeng Z, Pan M, Ziegler AD, Holden J, Spracklen DV, Brown LE, He X, Chen D, Ye B, et al. 2023. A global-scale framework for hydropower development incorporating strict environmental constraints. Nat Water. 1(1):113–122. doi:10.1038/s44221-022-00004-1.
- Zarfl C, Lumsdon AE, Berlekamp J, Tydecks L, Tockner K. 2015. A global boom in hydropower dam construction. Aquat Sci. 77(1):161–170. doi:10.1007/s00027-014-0377-0.
- Zhang C, Kuai S, Tang C, Zhang S. 2022. Evaluation of hydrological connectivity in a river floodplain system and its influence on the vegetation coverage. Ecol Indic. 144:109445. doi:10.1016/j.ecolind.2022.109445.
- Zhu Z, Woodcock CE. 2014. Continuous change detection and classification of land cover using all available Landsat data. Remote Sens Environ. 144:152–171. doi:10.1016/j.rse.2014.01.011.
- Zuluaga A, Etter A, Nepstad D, Chará J, Stickler C, Warren M. 2021. Colombia’s pathway to a more sustainable cattle sector: A spatial multi-criteria analysis. Land Use Policy. 109:105596. doi:10.1016/j.landusepol.2021.105596.