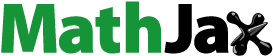
Abstract
Rapid urbanization currently poses a threat to cropland areas. Therefore, exploring the pattern of change in cropland areas and its driving mechanism is of great significance in safeguarding regional economic development and food security. In this study, data regarding cropland in the Changsha-Zhuzhou-Xiangtan city group (CZTCG) from 2000 to 2020 were collected, and dynamic changes in cropland in the past 20 years were analyzed. Based on this, the mechanisms that drive cropland change were explored in the context of multiple dimensions. Furthermore, an Inertial Development Scenario and a Cropland Priority Scenario were designed to simulate land use/land cover (LULC) changes in the CZTCG in 2025 and 2035, and, in particular, to analyze the characteristics of future spatiotemporal changes of cropland. The results demonstrated that the cropland area in the CZTCG in the past 20 years has shown a two-stage trend of increasing (2000–2015, increase of 8.25%) and decreasing (2015–2020, decrease of 2.91%), and the main reasons for cropland loss in the whole process of change were forest and building, with serious loss of cropland area in Changsha County and Wangcheng District. Between 2000 and 2020, socio-economic factors (GDP, total agricultural output), topography (elevation, slope), and climate change (average annual temperature, annual precipitation) were highly correlated with changes in cropland area, exhibiting significant temporal and regional variability. The simulation results indicate that until 2035, the Cropland Priority Scenario shows a significant increase in the area of cropland in the study area compared to the Inertial Development Scenario. Consequently, the implementation of a strategy that prioritizes cropland can effectively protect it, while helping to promote ecological development and regional socio-economic development.
1. Introduction
China is a large country dominated by agriculture, and cropland is an important part of the agroecosystem, playing an essential role in maintaining the ecological environment (Zhang et al. Citation2022). In recent years, due to the process of industrialization and urbanization in China, large areas of high quality cropland have been occupied, leading to the increasing fragmentation of contiguous cropland, reducing its area and destroying its ecosystem (Wu et al. Citation2021; Yan et al. Citation2022). This urban/industrial progress seriously constrains the sustainable development of cropland resources and even severely affects China’s food production and agricultural development (Qiu et al. Citation2020; S. Wang et al. Citation2021). As China’s economy shifts to high quality development, the pattern of urbanization development is ushering in a major change, with central cities and city groups becoming the main carrying space for the economy and population, and urban development attracting important opportunities (Bahar et al. Citation2020). However, cropland resources are facing major challenges due to the overloading of land resources in the central cities. Therefore, monitoring changes in cropland and modeling the future spatial distribution of cropland is crucial to local governments, especially in cities facing major reforms and in the midst of urban expansion, to formulate rational policies towards sustainable development.
Urbanization is achieved through the appropriation of cropland for urban development or by taking advantage of better non-farm economic opportunities (Hou et al. Citation2019). According to surveys, 60% of the world’s irrigated cropland is located near urban areas, and with agricultural production set to increase by 70% by 2050, the competition for prime land is expected to continue and even accelerate (Qiu et al. Citation2020). At the same time, China’s cropland continued to decline during the first decade of the twenty first century. Therefore, urbanization is always accompanied by the loss of cropland, and the growing demand for urban development is considered to be the main reason for the decline in cropland. Studies on cropland in Changsha-Zhuzhou-Xiangtan area mainly focused on quantitative evaluation of land use change and the reasons for its transfer, ecosystem service function and its influencing factors, etc., and few studies analyzed the driving mechanism of cropland change and its future dynamic change (Fan et al. Citation2022; Wang et al. Citation2023). Therefore, monitoring changes in cropland and simulating the future distribution of cropland is essential for local governments to formulate appropriate sustainable development policies. In the early stage of this research, scholars at home and abroad preliminarily discussed the driving factors of cropland changes by using mathematical and statistical analysis methods (Wu et al. Citation2021), linear regression models (Liu et al. Citation2019), and spatial analysis to study the relationship between cropland changes and economic growth, urban expansion and geographic location, etc. (Ullah and Uddin Citation2021; Zaveri et al. Citation2020). The results revealed that factors such as public services, transportation, and agricultural output value affect the amount of cropland and the non-agriculturalization of cropland. As the research developed, 3S technology and mathematical statistics were introduced, and with the help of spatial analysis methods (Xiao et al. Citation2018), empirical research was carried out at different spatial scales, such as the national, watershed, and regional scales, to characterize the spatiotemporal distribution of cropland. Furthermore, econometric methods (Cho and McCarl Citation2021), logistic models (Chen et al. Citation2021), geographically weighted regressions (Ren et al. Citation2020), geo-detectors (Zhao et al. Citation2022), and spatial econometric regression analysis (Du et al. Citation2023) were applied to cropland research to identify and analyze the driving mechanism of the changes in cropland in a single land category, realizing a gradual shift from qualitative research to a combination of qualitative and quantitative spatialization. Most of these models start from a unidirectional effect of the independent variable on the dependent variable, lacking the effect on the interlinking of multiple groups of dependent variables. Structural equation modeling unifies factor analysis, path analysis, analysis of variance, regression analysis, etc., in its system, and after comprehensive use and continuous improvement and enhancement, it has recently become a mainstream linear statistical model, with wide used in multidisciplinary fields.
Forecasting land use/land cover (LULC) is an important methodology for city development and policy formulation, which is particularly important for upgrading the level of spatial planning and management of the territory. Moreover, measuring, modeling, and understanding coupled human environment systems is instrumental in addressing the challenges posed by environmental change and promoting global sustainable development. Currently, most studies use land use models to develop land simulation predictions supported by multi-temporal and multi-source remote sensing data. Among them, the newly developed PLUS (Patch generating Land Use Simulation) model (Liang et al. Citation2021), compared with the commonly used Markov model, CLUE-S (Conversion of Land Use and its Effects at Small regional extent) model (Mohammady et al. Citation2018), SD (System Dynamics) model (Kim et al. Citation2020), and FLUS (Future Land Use Simulation) model (Liu et al. Citation2022), improves the analysis strategy of the relationship between land use changes and influencing factors, enhances the simulation performance of land use patch changes, and better realizes spatial optimization.
Changsha-Zhuzhou-Xiangtan city group (CZTCG) is the core growth pole of Hunan Province’s development and an important support for the province to be at the forefront of that growth (Deng et al. Citation2021). In addition, due to its advantageous geographical location, CZTCG has experienced rapid urbanization since 1984, when integration was formally proposed, resulting in rapid population and economic growth. This has been manifested in the region by the outward expansion of urban land, leading to an increased reduction in cropland area, the non-agricultural transfer of the agricultural population, and the conversion of cropland to non-cropland (Fan et al. Citation2022; Jiang et al. Citation2016). Meanwhile, relatively few studies have been conducted on the extraction and monitoring of cropland area in the CZTCG. Therefore, in this study, taking CZTCG as a typical region, we quantitatively evaluated the dynamic changes of cropland and explored the potential driving factors of its spatiotemporal changes in order to realize the corresponding regulation mechanism. Meanwhile, we designed different development scenarios and set the land transfer direction in combination with local land policies to promote the sustainable development of regional cropland. The main aims of this study are to: (1) to extract information on cropland in the study area and monitor its pattern of change over the past 20 years; (2) to explore the effects of different indicators on changes in the cropland area; and (3) to predict the future pattern of spatiotemporal changes in cropland in accordance with inertial development and cropland prioritization patterns. Based on the above objectives, the ultimate goal of this investigation is to develop information related to changes in the dynamics of cropland in the CZTCG, and to offer a reference for regional sustainable development from the perspective of sustainable development.
2. Materials and methods
2.1. Study area
Changsha-Zhuzhou-Xiangtan city group is located in the eastern-central part of Hunan Province, in the transition zone between eastern and central-western China, and lies between latitudes of 26°18′19″ and 28°41′72″N and longitudes of 111°58′38″ and 114°13′20″E, including the cities of Changsha, Zhuzhou, and Xiangtan. Changsha’s city limits mainly include the counties and districts of Yuelu District, Furong District, Tianxin District, Kaifu District; the districts of Tianyuan District, Hetang District, and Lusong District lie within the city limits of Zhuzhou; and Yuetang District, Yuhu District, and Xiangtan County are in Xiangtan City (). The region is located in the subtropical monsoon humid climate zone, with four distinct seasons, an average annual temperature of about 16 ∼ 19 °C, and an annual precipitation of about 1230 ∼ 1700 mm. The region is not only an important economic development center in Hunan Province but also an essential hub for industrial transfer from the eastern region, and for promoting the rise of the central region and boosting the development of industries in the western region.
2.2. Data sources and processing
Remote sensing data were mainly used to extract information on cropland. The information included time series Landsat 5 (2000, 2005, 2010) and Landsat 8 (2015, 2020) March-June 30 m resolution images acquired from the United States Geological Survey (https://earthexplorer.usgs.gov) to produce LULC maps based on Google Earth Engine. The image preprocessing steps included de-clouding and median synthesis. Cropland information was extracted by attribute from 2000 to 2020 through ArcGIS software.
Driving data were employed to analyze the correlation of driving factors with changes in cropland area and their impact on cropland changes. They mainly comprised meteorological data, natural factors, socio-economic data, soil properties data, and distance factor data. Among them, meteorological data included the annual mean temperature and annual precipitation, which were resampled to 30 m resolution. Natural factors included topographic conditions (elevation, slope), slope obtained through elevation using ArcGIS processing, and socio-economic data included urbanization (population, GDP, urbanization, total agricultural output value), The urbanization rate and total agricultural output value were obtained from county and district values through the Hunan Provincial Statistical Yearbook. The data (population, GDP, agricultural output and other statistics) were explored and analyzed using geostatistical methods (explored data), histogram distribution trends were observed, and the inverse distance weight method was selected for spatial interpolation analysis. The final raster data were obtained by extracting the generated results by mask using the vector data of the study area. The processed data were resampled to 30 m, and the clipping tool was applied to unify them into a dataset with the same row and column numbers and resolution. Soil properties data included soil organic matter, soil organic carbon, and soil clay. The distance factor was mainly taken as the distance from the national road, the distance from the county road, and the distance from the railway, which were obtained by calculating the Euclidean Distance based on the corresponding vector data, with a uniform resolution of 30 m ().
Table 1. Driving data and information.
Administrative boundary data were used for mapping, mainly including provincial and district boundaries and railway data. The data were obtained from the National Center for Basic Geographical Information (http://ngcc.sbsm.gov.cn). Details of the data are shown in .
2.3. Methodology
In this paper, we produced LULC classification data for the CZTCG based on remote sensing data, extracted cropland information, and analyzed the driving mechanism of cropland change, and dynamically simulated the future spatiotemporal distribution pattern of the cropland. The main workflow comprised three main steps: (1) extraction of cropland information and its spatiotemporal distribution characteristics; (2) exploration of the driving mechanisms of cropland change based on multiple groups of composite factors; and (3) dynamic modeling of future cropland changes under multiple scenarios ().
2.3.1. Extraction of LULC information
In this paper, the national standard of Classification of Land Use Status is adopted, which includes two levels of classification system, one level and two levels. According to the actual situation of the study area and the high-resolution image data, the land use classification was performed in five major one-level categories: Cropland, Forest, Grassland, Water and Building. The procedure was implemented on the Google Earth Engine (GEE) Cloud platform, allowing us to perform pixel-by-pixel analysis and ensuring that producers were free from data downloads and management. Public data on GEE, such as Landsat and MODIS data, provide long term terrestrial observations that help us synthesize temporal metrics and collect samples for training and validation. The specific process for LULC classification was as follows. (1) Input features. The initial dataset was constructed based on Landsat5/8 remote sensing images with 30 m resolution available during the target year, and the image preprocessing steps included de-clouding as well as median synthesis. Because spectral indices can effectively enhance the differences between different land types, as recommended by Li et al. (Citation2021), in order to enhance the recognition ability of LULC remote sensing imagery, we calculated the spectral indices of NDVI(Normalized Difference Vegetation Index), NDBI(Normalized Difference Built-up Index), and (MNDWI Modified Normalized Difference Water Index) to better differentiate between vegetated and non-vegetated, and building and water. This makes the image as clear as possible by minimizing clouds, cloud shadows, or other disturbances. At the same time, it is possible to reduce the dimensionality of the input features within the effective preservation time. (2) Sample data generation. An adequate sample size ensures the accuracy of supervised classification. Samples are collected through field survey data and high resolution images through visual interpretation, and it should be guaranteed that the training samples and validation samples are evenly distributed in the study area. In this paper, in order to facilitate the differentiation of land types, a total of 70 field survey samples were obtained, including 13 grassland samples, 35 cropland samples and 22 forest samples, with a sample size of 30 m × 30m. Each sample kept the relative purity of land types. The samples were collected in 2000, 2005, 2010, 2015 and 2020 by Google Earth’s historical high-resolution images and Landsat images for information extraction of long time series of cropland monitoring, and an average of 250 samples of cropland, 120 samples of forest, 70 samples of grassland, 160 samples of water, and 180 samples of building were obtained each year. Based on previous classification studies, each land cover type sample was divided in a 7:3 ratio in the established sample database, with 70% of the samples used as training samples and 30% of the samples used as validation samples. After determining the sample data, 70% of the sample data were randomly selected to be applied as the training dataset and 30% of the sample data as the validation set. (3) Classification and validation. The Random Forest classifier is widely used for LULC classification because it can handle both categorical and numerical features, as well as having the ability to handle high dimensional data. Therefore, the feature selection using Random Forest Classifier was applied to LULC classification. In this paper, the classification results and validation samples were validated using confusion matrix with overall accuracy, Kappa coefficient, and producer accuracy as evaluation indices to guarantee the accuracy and reliability of the classification results.
2.3.2. Net rate of change in cropland
The net rate of change in cropland is commonly used to measure the extent to which cropland in the study area is flowing in or out from the outside over a period of time, and it is generally expressed as the ratio of net change in cropland during the study period to the total cropland at the beginning of the study. The expression for it is
where N is the net rate of change in cropland; S is the total area of cropland at the beginning of the study period; Ub and Ua are the areas of cropland that increased or decreased at the end and beginning of the study period, respectively.
2.3.3. Structural equation modeling
Structural equation modeling (SEM) is a method of statistical analysis that analyzes the relationship between latent variables and between latent variables and observed indicators, and the method is also a model based on the covariance matrix between the variables. The model includes two categories: observed and latent variables (Edeh et al. Citation2023). Observed variables are those that are directly observable and measurable. Latent variables are variables that cannot be directly observed and characterized, thus requiring the measurement of latent variables with observed variables. Additionally, the setting of latent variables is usually based on some theoretical assumptions or research hypotheses about the research objectives based on existing research. The above two variables together constitute the measurement and structural models. The structural model is used to explore the correlation between different latent variables, while the measurement model is used to study the mechanism of latent and observed variables. The model expression is shown in .
According to the variety of changes in cropland, the study selected climate, soil, distance factor, urbanization, and terrain factor as the manifest variables. Mean annual temperature, annual precipitation, GDP, population, elevation, slope, soil organic matter, soil organic carbon, urbanization rate, total agricultural output value, and distance from provincial, railway, and county roads were used as observed variables. In this paper, we first used the Pearson correlation to analyze the comprehensive variables affecting the change of cropland area in the study area () and screened out the factors with a greater degree of influence as the subsequent driving data. To guarantee the feasibility of the model, the results of the correlation analysis were used to construct a structural equation model. During the modeling process, the model multicollinearity was analyzed by Variance Inflation Factor (VIF), and the results showed that the VIF values ranged from 1 to 5, indicating that there was no serious covariance problem among the variables. Convergent Validity (CV) was verified using mean variance extraction with a value greater than 0.5. Combined reliability was used to evaluate the reliability of the internal consistency of the model, and a CR value greater than 0.7 and not less than 0.6 proved that the model was reasonable. In conclusion, the model was proved to be reliable and feasible.
Figure 4. Correlation between drivers and changes in cropland area (elev: Elevation; popu: Population; temp: Temperature; prec: Precipitation; UR: urbanization, TAOV: total agricultural output value; DFR: distance from the railway; DFCR: distance from the county road; DFNR: distance from the national road).

2.3.4. Simulation of future cropland information
2.3.4.1. Plus model
The PLUS model is a patch generated land use change model based on meta-cellular automata. The model can mine the driving factor of land expansion and landscape change, and better simulate the generation and evolution of land use patches to accurately simulate LULC change (Liang et al. Citation2021). The specific steps are as follows. (1) Driving factor selection. Considering the availability of data and referring to the literature, the drivers were chosen mainly in terms of natural elements and socio-economic factors, which primarily comprised two natural factors, namely, average annual temperature and annual precipitation, and seven socio-economic factors, namely, elevation, slope, GDP, population, urbanization, distance from national roads, and distance from county roads (). (2) Land use expansion extraction. Firstly, the impact of driving factors on the expansion of different land use types was calculated based on 2010 and 2015 LULC data and using the Random Forest algorithm to predict the development potential of different land use types in the study area. Then, the model parameters were set through the CA model based on multi-type random patch seeds (CARS) module to predict LULC in 2020. (3) Neighborhood weight setting. The range of values of neighborhood weights in the PLUS model is 0 to 1, with higher values meaning greater neighborhood influence, indicating greater expansion capability. Combining the percentage of expansion area of different LULC types in the study area and references, the neighborhood weights of cropland, forest, grassland, water, and building were set to 0.214, 0.072, 0.033, 0.0131, and 0.449, respectively. (4) Accuracy test. In this paper, the 2010 LULC data were taken as the initial year data, and the 2020 simulation results were obtained based on the CARS module. When the model accuracy does not meet the requirements, the set of influencing factors with higher simulation accuracy is finally obtained by continuously adding and subtracting factors for model accuracy validation. Finally, the Kappa coefficient was calculated to be 0.87, the FoM coefficient was 0.23, and the RMSE value was in accordance with the requirements, so the results showed that the model predictions were more accurate.
2.3.4.2. Scenario setting
To comprehensively reflect future LULC changes in the study area, two scenarios were constructed to simulate the future LULC changes according to the ‘Outline of the Regional Integration and Development Plan of Changsha-Zhuzhou-Xiangtan’ and taking into account the actual situation in the study area. (1) Inertial Development Scenario (IDS): following the development direction under the historical trend of the study area, with no strict limitations on LULC conversions and no consideration of the impact of policies and planning, the simulation was conducted based on the probability of LULC conversions from 2010 to 2015. (2) Cropland Priority Scenario (CPS): cropland is the LULC type with the highest percentage in the study area, and cropland security is key to guaranteeing regional food security and sustainable development. This scenario limited the transfer of cropland to other land categories, with the aim of maximizing cropland and ecological protection (Zhou et al. Citation2019).
3. Results and analysis
3.1. Spatiotemporal distribution pattern of cropland in the CZTCG from 2000 to 2020
The classification results showed that the overall accuracy of LULC classification in the study area from 2000 to 2020 exceeded 90%, and the Kappa coefficients were higher than 0.88 (). Cropland information for 2000–2020 was obtained through the Extract by Attribute tool.
Changes in the cropland in the study area mainly underwent two stages. In the first stage, from 2000 to 2015, the cropland area showed an increasing trend, with a total increase of 349.8507 km2, an increase of 8.25%. The region with the largest increase in cropland area was Changsha County, followed by Xiangtan County. At the end of the 1990s, the policy of strengthening land management for the effective protection of cropland was issued, and the implementation of a system for the protection of basic farmland and the dynamic balance of the total amount of cropland was proposed, as well as the revision of the Land Management Law. In 2006, the binding target of a 1.8 billion mu red line for cropland was proposed for the first time. During this period, a provincial government cropland protection responsibility target appraisal system was also implemented, and an unprecedented level of attention was paid to the protection of cropland, which effectively protected and controlled the loss of cropland. In the second stage, from 2015 to 2020, the area of cropland slowly decreased, with a total decrease of 132.0156 km2, a decrease of 2.91%, and a serious decrease in the area of cropland in Changsha County and Wangcheng District (). Due to the release and implementation of the ‘Tenth Five-Year Plan for the Economic Integration of Changsha-Zhuzhou-Xiangtan’, the urbanization process has been greatly promoted, and the CZTCG has entered a phase of large scale development and construction, leading to an intensification of the occupation of cropland for non-agricultural construction and a greater degree of development of land resources. Meanwhile, with the implementation of the accelerated integration process of Changsha-Zhuzhou-Xiangtan and the approval of the two type society pilot zone in 2007, the rapid economic development started to occupy more cropland, and the pressure on cropland began to present an upward trend again after 2007. It demonstrates that changes in cropland and ecological protection are closely related to the implementation of construction projects, and the built-up area continues to spread out in all directions, resulting in a shrinking of the cropland area. At the same time, Wangcheng District, Ningxiang County, etc., represent an important agricultural product planting area in the Changsha-Zhuzhou-Xiangtan region and even in Hunan Province. In order to ensure food security, the government should pay sufficient attention to the protection of cropland resources while maintaining economic growth.
There are differences in the net change in cropland area between 2000 and 2020 by district and county. From 2000 to 2005, the net change rate of cropland in Kaifu District and Changsha County was the largest, at 12.21% and 11.22%, respectively. The areas with larger net changes in cropland from 2005 to 2010 were Tianxin and Yuhua districts, with net changes of 15.75% and 13.41%, respectively. The greatest net change in cropland from 2010 to 2015 was in the region represented by the Yuhu and Yuelu districts, which increased by 2.04% and 6.99%, respectively, compared to the previous two phases; however, the net rate of change in cropland decreased in 2015–2020 by 3.90% and 6.88%, respectively. It can be seen that during the period of 2000–2020, the most serious areas of cropland loss were in Kaifu, Furong, and Tianxin districts, which are located in the central urban areas and areas of rapid urbanization, and the cropland area of Changsha metropolitan area and other urban areas has reduced since 2015 under the pressure of the drastic increase in demand for land resources for economic development. Moreover, due to continuous development and construction, there is a shortage of reserve resources of cropland in the study area, which makes it difficult to realize the balance of cropland occupation and replenishment, thereby transferring the pressure of cropland in the study area to the neighboring areas ().
There are differences in the nature and socio-economic situation of the districts and counties in the region, and the magnitude of changes in the area of cropland and the direction of the transfer vary from region to region. From 2000 to 2020, the appearance of new cropland was mainly due to forest and building reclamation, located in the center of Furong District, Kaifu District, Yuhua District, and other urban areas. In contrast, the loss of cropland mainly occurred in the fallowing of farmland and the expansion of urban areas ( and ). The Dongting Lake Basin ecological conservation and construction program implemented since 1998, for example, focusing on the implementation of the project of returning farmland to the lake, returning farmland to forests, and cultivating wetlands, has increased the area of cropland. However, this has also been accompanied by regional construction, rapid expansion of urban areas along the Xiangjiang River, and serious loss of cropland area.
3.2. Influencing factors on changes in cropland area in the CZTCG from 2000 to 2020
In the SEM model, the path coefficients are used to evaluate the extent to which a variable affects its pointing variable, and the path coefficients can be positive, negative or zero. Positive path coefficients indicate a positive relationship between the independent and dependent variables, negative path coefficients show a negative relationship between the independent and dependent variables, and zero path coefficients indicate no relationship between the independent and dependent variables. The results showed that climate, topography, urbanization, soil conditions, and distance conditions had an effect on the distribution of carbon stocks. Among them, climate conditions, urbanization and topographic factors have a strong and positive influence on cropland change, the path coefficient distribution of climate conditions on cropland change were 0.179/-1.139/0.148/-0.102, the path coefficients of urbanization on cropland change were 0.083/0.247/-0.195/0.193, and the path coefficients of topographic conditions on cropland change were shown as 0.084/0.072/0.001/0.246 (). Based on the results of the path coefficients, it can be seen that there was variability in the impact of the drivers on changes in cropland area and their magnitude. Climate change, socio-economic factors, and topographical factors had the most significant impact on the change in cropland area, with the negative impact of socio-economic factors being more pronounced. In the context of climate change, on the one hand, a large area of cropland is covered by water, and surface waters extend significantly, making the area of cropland shift. On the other hand, the increase in water area affects the average temperature, soil humidity, and vegetation cover of the region, which not only plays a role in regulating the regional climate and maintaining the stability of the ecological environment but also promotes the growth and upgrading of the regional industry and boosts the local economic development. Changes in economic development and population numbers had a negative impact on changes in the area of cropland, which indicated that population size fluctuation and economic growth promoted the urbanization and industrialization of the region, resulting in an increase in the demand for various types of infrastructure, and contributed to the increase in the area of cropland. On the one hand, economic development has promoted scientific and technological progress, reducing the agricultural density of the region’s cropland, which is able to meet the food needs of the population, leading to an increase in abandoned land. At the same time, by combining the economic development of each prefecture level city in the CZTCG, it can be observed that the ultimate cause of the reduction in cropland area was manifested as a result of the outward expansion of urban land, the non-agricultural transfer of the agricultural population, and the transformation of cropland into non-cropland. Furthermore, population growth implies an ever increasing demand for food, so when considering the single linear relationship between population and cropland in isolation, the area of cropland ought to increase. On the other hand, the implementation of ecological protection and construction projects has brought about compensation for ecological benefits, making the development of forestry and pastoralism account for an increased proportion of agricultural output, taking up cropland resources and leading to a reduction in the area of cropland. Topographic factors showed a constant positive influence on the change in the area of cropland, with slope and elevation being the determining factors on whether or not to develop cropland. The distance factor determined the location of the cropland, and land resources that were located further away from the road required a large amount of labor and material resources, which was inversely proportional to the output. Soil properties were also an important factor affecting cropland, the quality of which is determined by the nature of the soil and indirectly affects crop output. Therefore, people usually choose to cultivate land that is nearby and has excellent soil properties. Generally, the drivers of changes in the cropland area were influenced by a combination of composite factors, such as climatic conditions, soil factors, distance factors, urbanization, and topographic factors, which directly or indirectly affect the area of cropland.
Figure 11. Structural equation modeling pattern diagram shows the relationship between variables and changes in cropland area over the period 2000–2020. Circles indicates latent variables and rectangular boxes indicate observed variables. The arrows indicate the influence relationship between the two. The red line represents a positive correlation between the factor and the change in cropland area, while the blue line indicates a negative correlation (abbreviations as in ).

3.3. Cropland analysis under multiple future scenarios in the CZTCG
The FLUS model and PLUS model were used to calculate the LULC in 2020. From the perspective of spatial distribution location and quantity prediction, there were relatively few incorrect patches in the simulated images of the PLUS model, and its simulation results were basically consistent with the actual images. Meanwhile, from the perspective of model simulation accuracy, the Kappa coefficient of the PLUS model (0.86) was higher than that of the FLUS model (0.82), and it was obvious that the simulation accuracy of the former was better than that of the latter at the same scale in the study area. Therefore, the PLUS model was selected in this study for LULC projections under multiple future scenarios ().
According to the outline of the integrated development plan for the CZTCG, 2025 and 2035 are important time points for CZTCG; thus, this study selected 2025 and 2035 as the simulation years for future LULC in the study area (). Under the Inertial Development Scenario, compared to 2020, the area of cropland in 2025 increased by 0.5%, and the area of building decreased; by contrast, the area of cropland in 2035 decreased by 1.3%, and the area of decrease was mainly concentrated in Furong District, Tianxin District, and Yuetang District. At the same time, the area of building under this scenario increased significantly, by 9.2%. Cropland loss showed a more pronounced pattern of change, with most of the loss of cropland likely to arise from the substitution of buildings and an increase in the use of cropland and basic farmland for afforestation and greening, planting of seedlings, construction of greenhouses, and human made landscapes. In the short term, such a phenomenon may bring economic benefits to the local community, but in the long term, the cultivation layer of the occupied cropland has been seriously damaged, changing the agricultural use of the cropland and the permanent basic farmland, and resulting in a great threat to the country’s food security. Under the Cropland Priority Scenario, cropland patches become larger, and patches of building land become more compact with fewer patches and being clustered towards the center, and the cropland area has increased to some extent. Compared to 2020, the area of cropland continued to grow until 2035, by which time it had increased by 129.95 km2. The area of cropland has increased more in areas represented by Yuetang and Kaifu districts (). The reduction in cropland can lead to threats to food security, making sustainable development less sustainable. This development is consistent with the opinions in the ‘Recommendations on the Future Science and Technology Development Strategy of Changsha-Zhuzhou-Xiangtan’, which focuses on emphasizing the construction of an ecological civilization and fully integrating the coordinated development of the three spatial patterns of towns, ecology, and agriculture in the preparation of spatial planning for the national territory. It illustrates that under the Cropland Priority Scenario, ecological land such as cropland and forest land were effectively protected, and the expansion of building land was slowed, which is applicable to the future development of the CZTCG.
4. Discussion
4.1. Impact of urbanization on changes in cropland area
Urbanization is considered to be a process of transformation from an agrarian based rural society to an industrial based urban society (Kipkulei et al. Citation2022). Since the Industrial Revolution, and especially since the development of modern cities, the contradiction between the protection of cropland and economic development has become more and more pronounced. Local economic development is closely related to land, and it plays a special role in the local economy and its financial revenue, and greatly promotes the process of industrialization and urbanization (Khawaldah et al. Citation2020; Wang et al. Citation2022). However, due to the accelerated process of industrialization and urbanization, there is a strong demand for land, whether for industry, agriculture, or urban development, leading to a prominent contradiction between the supply of and demand for land, which is also an important reason for the continued decline in cropland areas (Cai et al. Citation2021; Hu et al. Citation2020). Therefore, urbanization is invariably accompanied by a reduction in cropland, and there is always a conflict between urban expansion and cropland protection. It is worth noting that recent studies have identified a form of ‘gain/loss’, whereby cropland gains area from grasslands and forests while at the same time reducing it to built-up areas, which leads to a balance in the total (Xiong et al. Citation2022). This study emphasized the assumption that the building area mainly comes from the loss of cropland, and it can be seen that the change in the built-up area of each county and district in the CZTCG during the period of 2000–2020 was basically consistent with the change in the area of cropland and was at a stable level. The correlation results indicated that the change in cropland area between 2000–2005 (R2=0.267) and 2005–2010 (R2=0.004) was correlated with the change in built-up land, although the degree of fit was low. However, the two phases 2010–2015 (R2 = 0.531) and 2015–2020 (R2 = 0.851) showed a highly linear correlation ( and ). It indicated that the conclusion was consistent with the previous study (Hou et al. Citation2019). Thus, it was proved that urbanization was accompanied by a reduction in the area of cropland (Li et al. Citation2021). During the study period, there were sufficient cropland resources in the previous period, and the government issued a series of strict cropland protection policies, which ensured that the built-up area and land resources were well planned, resulting in a stable saturation of land resources. Nevertheless, when the cropland resources reached saturation, the reserve cropland resources were seriously insufficient, and the large-scale development and utilization method was no longer applicable. The paper argues that, under the process of rapid urbanization, the formulation of a policy for the protection of cropland that takes into account both the area and the amount of cropland can mitigate the threat of excessive loss of cropland. The unauthorized occupation of cropland resulting in the ‘de-farming’ and ‘non-food’ of cropland must be resolutely stopped and seriously treated.
4.2. Limitations and reflections
In this study, we first extracted the information of cropland in the CZTCG from 2000 to 2020 based on remote sensing data, and we analyzed the influencing factors of cropland changes in the past 20 years. Because the spatial distribution data of landscape elements used in this paper were obtained based on the ArcGIS platform after resampling, there may be certain errors and accuracy problems, which need to be further verified and optimized. The results showed that cropland change was governed by compounding factors. Natural factors, such as climate change, have an impact on cropland change, but anthropogenic factors have a more drastic impact on cropland change. Although the method used in this paper can reveal the mechanism of spatial heterogeneity of cropland changes and the dominant drivers, it does not consider the causal relationship between cropland changes and the drivers, and at the same time, it should take into account the feedback between the drivers, which needs to be further refined and improved. Finally, the PLUS model was used to simulate the change rule of cropland under the future scenario. While the PLUS model improves and enhances the simulation performance of LULC patch changes, it is prone to subjective bias in the parameter setting process, which may affect the accuracy of the prediction results.
We combined the future planning of the CZTCG and the results of this paper to put forward three points of reflection on the future direction of cropland (Guo et al. Citation2023; Li and Yi Citation2020). On the one hand, the CZTCG region has a good ecological base: the city was built with water, outstanding features, and at the same time, it is a national pilot area for the construction of a two type society, with a good trend of green development, and it should make full use of the superior geographic conditions and natural resources. Secondly, it is recommended that construction land indicators be tilted. In the preparation of national spatial planning, the three spatial patterns of townships, ecology, and agriculture should be fully integrated, and the tasks of construction land indices and basic farmland protection should be balanced, with appropriate increases in construction land indices for the CZTCG integration zone, reductions in basic farmland indices, and the vacating of township industrial parks and inefficient industrial zones in remote areas to restore arable land by means of comprehensive land remediation across the entire region. Thirdly, it is recommended that strategic research and planning pre-control be strengthened. The CZTCG integration city should implement a strategic white space and project access mechanism, strictly protect landscape ecological resources and riverfront resources, and provide spatial security and land reserves for the construction of a high-level Changsha-Zhuzhou-Xiangtan city group.
5. Conclusions
In this paper, we explored the spatiotemporal patterns of cropland changes in the CZTCG over the past 20 years and predicted cropland conditions under different scenarios in 2025 and 2035. The CZTCG, as an important regional economic center, has developed rapidly, causing cropland to undergo a profound transformation. Our results demonstrated that between 2000 and 2020, the area of cropland exhibited a phase change of increasing and then decreasing, with cropland patches gradually shrinking and shifting to a more regular distribution pattern. These observations indicated that changes in cropland were influenced by composite factors. Among them, socio-economic conditions and climate change had a significant impact on cropland change. Equally important, this study utilized the PLUS model to predict the land distribution in 2025 and 2035 in the CZTCG based on a multi-scenario model (Inertial Development Scenario, Cropland Priority Scenario). The results of the Inertial Development Scenario showed an increased risk of loss of existing basic cropland if LULC patterns are not controlled. Conversely, the Cropland Priority Scenario revealed that spatial and quantitative controls on LULC types are able to effectively slow the decline of cropland. In contrast, setting a target value for the amount of cropland for a specific area can better protect cropland; however, it may significantly mitigate the urbanization of the region. Therefore, the optimal policy is to take into account the context of rapid urbanization while focusing on the protection and planning of cropland by adopting a combination of strict delineation of protected areas and basic farmland zoning, as well as the setting of a wide range of total cropland areas for the protection of cropland.
Authors’ contributions
Wenbo Zhang: Conceptualization, Methodology, Software, Formal analysis, Project administration, Funding acquisition, Investigation, Writing-original draft, Writing-review and editing. Conceptualization, Methodology, Software, Formal analysis, Song Ji, Yang Dong, Dongzi Li and Ming Li. Dazhao Fan: Data curation, Investigation, Funding acquisition, Writing-original draft. All authors have read and agreed to the published version of the manuscript.
Acknowledgements
The National Natural Science Foundation of China (No.41971427); Program of Song Shan Laboratory (included in the management of the Major Science and Technology Program of Henan Province) (221100211000-5); and The High Resolution Remote Sensing, Surveying and Mapping Application Demonstration System (Phase II) (No. 42-Y30B04-9001-19/21).
Disclosure statement
The authors declared that they have no conflicts of interest to this work. We declare that we do not have any commercial or associative interest that represents a conflict of interest in connection with the work submitted.
References
- Bahar NHA, Lo M, Sanjaya M, Van Vianen J, Alexander P, Ickowitz A, Sunderland T. 2020. Meeting the food security challenge for nine billion people in 2050: what impact on forests? Glob Environ Chang. 62:102056. doi:10.1016/j.gloenvcha.2020.102056.
- Cai T, Zhang X, Xia F, Zhang Z, Yin J, Wu S. 2021. The process-mode-driving force of cropland expansion in arid regions of China based on the land use remote sensing monitoring data. Remote Sens. 13(15):2949. doi:10.3390/rs13152949.
- Chen Z, Huang M, Zhu D, Altan O. 2021. Integrating remote sensing and a Markov-FLUS model to simulate future land use changes in Hokkaido, Japan. Remote Sens. 13(13):2621. doi:10.3390/rs13132621.
- Cho SJ, McCarl B. 2021. Major united states land use as influenced by an altering climate: a spatial econometric approach. Land. 10(5):546. doi:10.3390/land10050546.
- Deng C, Liu J, Liu Y, Li Z, Nie X, Hu X, Wang L, Zhang Y, Zhang G, Zhu D, et al. 2021. Spatiotemporal dislocation of urbanization and ecological construction increased the ecosystem service supply and demand imbalance. J Environ Manage. 288:112478. doi:10.1016/j.jenvman.2021.112478.
- Du T, Li C, Wang Z. 2023. Spatial differentiation and influencing mechanisms of farmland transfer rents in mountainous areas: evidence from Chongqing and its surrounding areas. Land. 12(3):699. doi:10.3390/land12030699.
- Edeh E, Lo W-J, Khojasteh J. 2023. Review of partial least squares structural equation modeling (PLS-SEM) using R: a workbook. Struct Equat Model. 30(1):165–167. doi:10.1080/10705511.2022.2108813.
- Fan X, Quan B, Deng Z, Liu J. 2022. Study on land use changes in Changsha–Zhuzhou–Xiangtan under the background of cultivated land protection policy. Sustain. 14(22):15162. doi:10.3390/su142215162.
- Guo A, Yue W, Yang J, Xue B, Xiao W, Li M, He T, Zhang M, Jin X, Zhou Q. 2023. Cropland abandonment in China: patterns, drivers, and implications for food security. J Clean Prod. 418:138154. doi:10.1016/j.jclepro.2023.138154.
- Hou H, Wang R, Murayama Y. 2019. Scenario-based modelling for urban sustainability focusing on changes in cropland under rapid urbanization: a case study of Hangzhou from 1990 to 2035. Sci Total Environ. 661:422–431. doi:10.1016/j.scitotenv.2019.01.208.
- Hu Q, Xiang M, Chen D, Zhou J, Wu W, Song Q. 2020. Global cropland intensification surpassed expansion between 2000 and 2010: a spatio-temporal analysis based on GlobeLand30. Sci Total Environ. 746:141035. doi:10.1016/j.scitotenv.2020.141035.
- Jiang W, Chen Z, Lei X, He B, Jia K, Zhang Y. 2016. Simulation of urban agglomeration ecosystem spatial distributions under different scenarios: a case study of the Changsha-Zhuzhou-Xiangtan urban agglomeration. Ecol Eng. 88:112–121. doi:10.1016/j.ecoleng.2015.12.014.
- Khawaldah HA, Farhan I, Alzboun NM. 2020. Simulation and prediction of land use and land cover change using GIS, remote sensing and CA-Markov model. Glob J Environ Sci Manag. 6:215–232. doi:10.22034/gjesm.2020.02.07.
- Kim S, Chang S, Castro-Lacouture D. 2020. Dynamic modeling for analyzing impacts of skilled labor shortage on construction project management. J Manage Eng. 36(1):1–13. doi:10.1061/(asce)me.1943-5479.0000720.
- Kipkulei HK, Bellingrath-Kimura SD, Lana M, Ghazaryan G, Boitt M, Sieber S. 2022. Modelling cropland expansion and its drivers in Trans Nzoia County, Kenya. Model Earth Syst Environ. 8(4):5761–5778. doi:10.1007/s40808-022-01475-7.
- Li W, Yi P. 2020. Assessment of city sustainability—coupling coordinated development among economy, society and environment. J Clean Prod. 256:120453. doi:10.1016/j.jclepro.2020.120453.
- Li X, Suoerdahan G, Shi Z, Xing Z, Ren Y, Yang R. 2021. Spatial-temporal impacts of urban sprawl on ecosystem services: implications for urban planning in the process of rapid urbanization. Land. 10(11):1210. doi:10.3390/land10111210.
- Liang X, Guan Q, Clarke KC, Liu S, Wang B, Yao Y. 2021. Understanding the drivers of sustainable land expansion using a patch-generating land use simulation (PLUS) model: a case study in Wuhan, China. Comput Environ Urban Syst. 85:101569. doi:10.1016/j.compenvurbsys.2020.101569.
- Liu J, Shang J, Qian B, Huffman T, Zhang Y, Dong T, Jing Q, Martin T. 2019. Crop yield estimation using time-series MODIS data and the effects of cropland masks in Ontario, Canada. Remote Sens. 11(20):2419. doi:10.3390/rs11202419.
- Liu X, Wei M, Li Z, Zeng J. 2022. Multi-scenario simulation of urban growth boundaries with an ESP-FLUS model: a case study of the Min Delta region, China. Ecol Indic. 135:108538. doi:10.1016/j.ecolind.2022.108538.
- Mohammady M, Moradi HR, Zeinivand H, Temme AJAM, Yazdani MR, Pourghasemi HR. 2018. Modeling and assessing the effects of land use changes on runoff generation with the CLUE-s and WetSpa models. Theor Appl Climatol. 133(1-2):459–471. doi:10.1007/s00704-017-2190-x.
- Qiu B, Li H, Tang Z, Chen C, Berry J. 2020. How cropland losses shaped by unbalanced urbanization process? Land Use Policy. 96:104715. doi:10.1016/j.landusepol.2020.104715.
- Ren Y, Lü Y, Fu B, Comber A, Li T, Hu J. 2020. Driving factors of land change in China’s loess plateau: quantification using geographically weighted regression and management implications. Remote Sens. 12(3):453. doi:10.3390/rs12030453.
- Ullah KM, Uddin K. 2021. The relationships between economic growth and cropland changes in Bangladesh: an evidence based on annual land cover data. Environ Challenges. 5:100252. doi:10.1016/j.envc.2021.100252.
- Wang L, Zheng W, Tang L, Zhang S, Liu Y, Ke X. 2021. Spatial optimization of urban land and cropland based on land production capacity to balance cropland protection and ecological conservation. J Environ Manage. 285:112054. doi:10.1016/j.jenvman.2021.112054.
- Wang S, Bai X, Zhang X, Reis S, Chen D, Xu J, Gu B. 2021. Urbanization can benefit agricultural production with large-scale farming in China. Nat Food. 2(3):183–191. doi:10.1038/s43016-021-00228-6.
- Wang W, Li B, Su F, Jiang Z, Chen S. 2023. Identifying ecological security patterns meeting future urban expansion in Changsha–Zhuzhou–Xiangtan urban agglomeration, China. Remote Sens. 15(12):3141. doi:10.3390/rs15123141.
- Wang Z, Li X, Mao Y, Li L, Wang X, Lin Q. 2022. Dynamic simulation of land use change and assessment of carbon storage based on climate change scenarios at the city level: a case study of Bortala, China. Ecol Indic. 134:108499. doi:10.1016/j.ecolind.2021.108499.
- Wu H, Sipiläinen T, He Y, Huang H, Luo L, Chen W, Meng Y. 2021. Performance of cropland low-carbon use in China: measurement, spatiotemporal characteristics, and driving factors. Sci Total Environ. 800:149552. doi:10.1016/j.scitotenv.2021.149552.
- Xiao R, Liu Y, Huang X, Shi R, Yu W, Zhang T. 2018. Exploring the driving forces of farmland loss under rapidurbanization using binary logistic regression and spatial regression: a case study of Shanghai and Hangzhou Bay. Ecol Indic. 95:455–467. doi:10.1016/j.ecolind.2018.07.057.
- Xiong S, Baltezar P, Crowley MA, Cecil M, Crema SC, Baldwin E, Cardille JA, Estes L. 2022. Probabilistic tracking of annual cropland changes over large, complex agricultural landscapes using google earth engine. Remote Sens. 14(19):4896. doi:10.3390/rs14194896.
- Yan Z, Zhou D, Li Y, Zhang L. 2022. An integrated assessment on the warming effects of urbanization and agriculture in highly developed urban agglomerations of China. Sci Total Environ. 804:150119. doi:10.1016/j.scitotenv.2021.150119.
- Zaveri E, Russ J, Damania R. 2020. Rainfall anomalies are a significant driver of cropland expansion. Proc Natl Acad Sci U S A. 117(19):10225–10233. doi:10.1073/pnas.1910719117.
- Zhang J, Song Y, Wang J. 2022. Spatiotemporal patterns of gross ecosystem product across China’s cropland ecosystems over the past two decades. Front Ecol Evol. 10:959329. doi:10.3389/fevo.2022.959329.
- Zhao S, Fan Z, Gao X. 2022. Spatiotemporal dynamics of land cover and their driving forces in the Yellow River Basin since 1990. Land. 11(9):1563. doi:10.3390/land11091563.
- Zhou Y, Hartemink AE, Shi Z, Liang Z, Lu Y. 2019. Land use and climate change effects on soil organic carbon in North and Northeast China. Sci Total Environ. 647:1230–1238. doi:10.1016/j.scitotenv.2018.08.016.