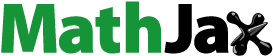
Abstract
Malaria is a serious, acute disease spread by female Anopheles mosquito bites. Malaria is an epidemic in the Wolaita Zone, affecting 85% of the population despite the implementation of effective prevention and control measures in the area’s public health services. The main objective of this study is to apply geospatial techniques to environmental, socioeconomic, and epidemiological parameters that increase certain conditions for malaria incidence to identify malaria vulnerability hotspots using a multi-criteria evaluation method. The malaria vulnerability hotspot map is identified by using AHP and the malaria hotspot identification algorithm. The findings show that the research area is 51% very high and highly vulnerable (224.14 km2), 26% moderate (116.560 km2) and 23% low vulnerable (103.600 km2). The western part of the area, which is near the Omo River and has low elevation, is highly vulnerable to malaria incidence. Reliable malaria vulnerability hotspot maps are critical for estimating the scope of the problem and, consequently, the resources required to combat malaria.
1. Introduction
Malaria is a severe, acute disease spread by the bites of infected female Anopheles mosquitoes, also known as ‘malaria vectors’. Malaria remains a major cause of illness and death in children and adults worldwide, with 87 countries reporting one or more malaria cases in 2017 (WHO Citation2019). Four parasite species cause malaria in humans, and two of these species, Plasmodium falciparum (Pf) and Plasmodium vivax, are the most common. Pf accounted for 99.7% of the total cases of malaria in the WHO African Region in 2018, 50% of cases in the South-East Asia Region and 71% of cases in the Eastern Mediterranean Region (WHO Citation2020). Pf is the most common of the four human malaria parasites across much of Sub-Saharan Africa (Feachem and Jamison 1991).
Ethiopia, one of the Sub-Saharan African countries, is a malaria victim (Kassaw et al. Citation2019). Malaria remains one of Ethiopia’s leading causes of illness and death (Eniyew Citation2018). It’s one of the most health problems within the country, during which its cases are one of the highest and increasing at an alarming rate. However, exposure to malaria varies markedly by location and season (Ahmed Citation2014). For Ethiopians living at altitudes −100 to >4220 m, the topography is a rich breeding ground for Mosquitoes (Ahmed Citation2014; Kassaw et al. Citation2019).
Elevation is an important factor in the transmission of malaria because it greatly determines the temperature, and the temperature, in turn, affects mosquito breeding as the length of the immature stage in the life cycle (Kebede et al. Citation2017). Accordingly, at high temperatures, the egg, larval and pupil stages are shortened so that turnover is increased and the length of the saprogenic cycle of the parasite within the mosquito host is also influenced, i.e. when the temperature rises, the period of the saprogenic cycle is short-circuited (Ahmed Citation2014).
The slope is another environmental parameter that may be associated with mosquito larval habitat formation, which is the measurement of the rate change of the land per unit distance, which may affect the stability of the aquatic habitat (Ahmed Citation2014; Kebede et al. Citation2017). Regarding the relationship between slope and malaria case, water appears to be logged in regions with low-lying slopes, which speeds up the possibilities of water stagnation. Ahmad et al. (Citation2017) state that this aspect is another important parameter that influences malaria transmission. The northern aspect ranges from NW340 to NE70, known as the shadow area. In the shadow area, the moisture content is high due to the lack of direct sunlight, and compared to the other aspects, there is relatively dense vegetation. Shadow influences the larval distribution for the risk of malaria, and other parameters also contribute to the malaria life cycle and diffusion in the country.
Malaria is a major burden of public health services in the Wolaita Zone. According to WZHD (Citation2019), about 85% of the population is at risk of malaria despite effective prevention and control interventions. The outbreak of malaria is continuous in three woredas: Kindo Koysha, Boloso Sore and Humbo. To overcome this problem, it is important to use cost and time-effective preventive and control practices. Prevention of malaria can be more cost-effective than treating the disease in the long run (Dana Citation2018). Experts, specialists or scientists working on vector-borne disease control are applying many traditional, conventional and modern scientific methods. However, conventional approaches are laborious, costly, erroneous and time-consuming (Manish Citation2017).
Kindo Koysha is a malarious district on the foot of the Omo River in the Wolaita Zone. Malaria is a serious problem in Kindo Koysha because of the large number of inpatient and outpatient cases. The data accessed from Kindo Koysha Woreda Health Office reveal that malaria is still epidemic in the area, with 3233, 2981 and 7008 consecutive data of malaria cases in the years 2017, 2018 and 2019 (WorHO, Citation2020). Currently, the problem is becoming greater due to time-consuming prevention and control activities for malaria. According to Yadav et al. (Citation2012), cited by Qayum et al. (Citation2015), Malaria control action plans are dying out due to improper implementation, inadequate surveillance and lack of georeferenced information to pin-point the trouble spots for timely preventive action. Effective vector control requires a vast knowledge of the ecology of breeding and resting habitats as well as the actions of the different mosquito species; it is crucial to develop a tool with such functionality and great performance (Okogun Citation2005; Pam et al. Citation2017).
According to Kassaw et al. (Citation2019), geospatial technologies, i.e. Geographic Information System (GIS), Remote Sensing (RS) and Global Positioning System (GPS), offer an opportunity for rapid assessment of malaria-endemic areas. These technologies, coupled with prevalence/incidence data from health centres, can provide reliable estimates of the population at risk, predict disease distributions in areas that lack baseline data and provide guidance for intervention strategies so that scarce resources can be allocated cost-effectively (Kumar et al. Citation2021; Naqvi et al. Citation2023). The GIS and RS are new technologies that have emerged as frontrunners in malaria epidemiology research. By applying GIS and RS techniques for mapping: vector environments, vector presence, abundance and density, it also assesses the risk of vector-borne diseases, disease transmission and spatial diffusion (Manish Citation2017).
Malaria continues to kill and harm many people of all ages throughout Kindo Koysha Woreda at any given moment. Furthermore, Kindo Koysha has a substantial number of inpatient and outpatient malaria cases; it is a serious problem. The woreda has suffered a great loss of life and money. As a result, research is necessary in Kindo Koysha; it is possible to model malaria cases linked to environmental and socioeconomic variables very important.
Several index-based malaria modelling studies have been conducted in Ethiopia. These studies have used a variety of approaches to model the relationship between malaria incidence and environmental factors, such as rainfall, temperature and vegetation (Ahmed Citation2014; Mestewat Citation2014; Kassaw et al. Citation2019; Muluken Citation2019). This study addresses the high spatial resolution data usage and applies more variables to identify malaria vulnerability.
Modelling these variables with the help of GIS and RS techniques is very valuable for defining focal points of malaria transmission (Kassaw et al. Citation2019). The research was conducted to identify malaria epidemic-prone areas’ hotspot risk maps using spatial multi-criteria evaluation (MCE) technique to improve malaria intervention and control program service in Majang zone, Gambella, Ethiopia. Finally, they concluded that a large area of the zone is found to be a risk area for malaria, and this research justified that combining GIS with MCE was capable of integrating all the malaria hazard causative factors and the components of malaria risk. MCE provides a foundation for selecting, sorting and prioritizing materials and helps in the overall assessment. It is crucial because it allows decision-makers to systematically evaluate and compare different options based on their relative importance and trade-offs.
Therefore, this study focuses on applying environmental, socioeconomic and epidemiological parameters that facilitate conditions for malaria incidence and developing a malaria vulnerability hotspot map of the study area using geospatial techniques with MCE. The output of this study considered will be used as input to stakeholders for the betterment of preventive and control practices.
2. Materials and methods
2.1. Description of the study area
The study area Kindo Koysha Woreda is one of the woredas in the Wolaita Zone of the South Nation Nationalities and People Regional state, which is located at 37°24′–37°38′ E longitude and 6°48′–7°04′ N latitude, 431 km southeast of Addis Ababa and 36 km west of Wolaita Sodo town. Woreda has a total area of 444.30 km2, administratively divided into 19 Kebeles and one town administration called Bele Town, with a total population of nearly 139,316. It is composed of 2 agro-ecological zones: 21% midland temperate (‘Woinadega’) and 79% lowland (‘Kolla’), with altitude ranging from 687 m at the foot of the Omo River valley to 2164 m above sea level (Buke and Gidago Citation2016).
The area has an average maximum and minimum temperature of 30.7 °C and 18.2 °C, respectively, and the annual rainfall has characteristic monthly variation, with peak rainy seasons usually observed during March through May (autumn), locally termed as ‘belg’, and July through September (summer to partially spring), locally termed as ‘kremt’ to partially ‘tsedey’. In woreda there are currently one primary hospital, 3 health centres and 28 health posts. The map in shows the location of the study area.
2.2. Materials and data collection
Different data from primary and secondary sources were collected from field surveys, online sources and respective institutions (). Instruments and materials used in current study are listed with their purpose in .
Table 1. Data source.
Table 2. Instruments and materials used.
The main parameters used in this study were identified through a literature review as well as a questionnaire, and they are environmental (elevation, slope, aspect, wetness, and also drainage buffer, proximity to a river, rainfall, temperature and land use/land cover), epidemiological (annual parasite incidence (API) and Pf cases) and socioeconomic parameters (population and hospital proximity). After the parameters were identified, buffering, interpolation, reclassification and zonal statistical processes were performed using ArcGIS tools, and land use/cover supervised classification was performed using ERDAS Imagine. Both geospatial techniques and the multi-criteria evaluation method were used to achieve the objectives of this study. These methods were chosen for the study because they are very effective and easy to apply, and a malaria hotspot identification algorithm was used to compute the parameters, and zonal statistics were used to determine to what extent vulnerability degree in each Kebele shares in the study area. The details of data analysis as per the conceptual framework flow chart are presented in .
2.2.1. Malaria hotspot identification algorithm
The hotspot identification was done by applying the multi-criteria evaluation method to weight the factor for weighted overlay, and finally, the vulnerability hotspot map was identified by the malaria hotspot identification algorithm. A hotspot refers to an area or geographical region of relatively higher importance that is based on parameters such as symptomatic cases and asymptomatic, Malaria hotspot identification algorithm used as done by Qayum et al. (Citation2015) and Ahmad et al. (Citation2017).
(1)
(1)
where MHS is a Malaria hotspot,
HSse is a hotspot for socioeconomic parameters,
HSepidemiology is a hotspot for epidemiology and
HSenv is a hotspot for environmental parameters.
2.2.2. Weight computation
All the parameters were rated based on their influence on mosquito breeding and suitability for mosquito habitat. Because each factor has a different degree of influence, it was necessary to determine the weight of each factor. Previous works and literature were used to assign weights to the factors (Mestewat Citation2014; Ahmad et al. Citation2017; Kassaw et al. Citation2019; Muluken Citation2019), and a discussion with malaria experts by using a questionnaire to get the appropriate weights for the parameters.
The analytical hierarchy process (AHP), which is one of the techniques for multi-criteria decision-making, is used to attain the weights by obtaining the main eigenvectors of the reciprocal square matrix of pairwise comparisons between criteria. The sum of the weights must be one in the multi-criteria evaluation process using a weighted linear combination (Eastman Citation1999; Saaty Citation2008). The comparison involves determining the relative importance of the two criteria involved in a given goal. Scores are provided on a continuous 9-point scale for pairwise comparison using this computation of this study.
2.3. Analysis
This study’s findings were a combination of thirteen factors classified into three categories based on their influence on the spread of malaria incidence and facilitation of mosquito breeding.
2.3.1. Relationship of environmental parameters with malaria incidences
The environmental factors, including elevation, slope, aspect, wetness index, drainage buffer, distance to rivers, rainfall, temperature and land use/land cover, were presented in this section for what extent each factor shares the degree of vulnerability in the study area.
2.3.1.1. Elevation
This study’s reclassified elevation map ((a)) shows from the total area coverage 58.357 km2 as very high, 118.997 km2 high, 127.889 km2 as moderate, 84.599 km2 low and 54.458 km2 as very lowly vulnerable to malaria. According to Federal Ministry of Health (FMOH), areas with elevations of less than 1500 m are intensively favourable for mosquito breeding, so 80% of the study area has elevations ranging from 687 to 1500 m amsl, which could be favourable for mosquito breeding.
2.3.1.2. Slope
To the assessment of identifying a suitable area for mosquito breeding, the reclassified slope map ((b)) reveals that approximately 22% are very highly vulnerable to malaria incidence, 28% are high, 31% are moderate, 18% are low and 1% are very low with area coverage of 99.002, 125.362, 137.674, 78.233 and 4.029 km2, respectively.
2.3.1.3. Aspect
The presence of shadow helps the soil to hold moisture that creates a favourable site for mosquito breeding; based on reclassification, the study area can be divided into two categories: high 15%, with an area coverage of 68.693 km2, which is suitable for breeding, and low 85% with an area coverage of 375.607 km2, which have a lesser influence on the breeding system.
2.3.1.4. Drainage buffer
The reclassified drainage buffer covers a total of 89% of the study area covered by two classes: very high (52%) covers an area of 231.828 km2, and highly vulnerable (37%), has 166.58 km2 area coverage. The remaining classes covered 11% of the study area. These are moderate 9% (41.220 km2), low 1% (3.728 km2) and very low 1% (0.944 km2). details the area coverage and classification for an environmental factor of this study.
Table 3. Environmental parameters classification and area coverage.
2.3.1.5. Proximity to the river
According to the classified proximity to river class, 40% of the study area is vulnerable to malaria incidence with the degree of extremely high and highly vulnerable, the area coverage of these two classes is about 135.134 km2, the moderately vulnerable area cover 13% of study area 57.646 km2, and both low and very lowly vulnerable classes cover about 56% about 251.52 km2 of the study area.
2.3.1.6. Wetness index
The reclassified wetness index map ((d)) reveals that approximately 59% of the study area is favourable for mosquito breeding, with the remaining percent shares being moderate, low and very low, with values of 28, 11 and 2, respectively. The study area covers the classes very high and high degrees, 262.78 km2, moderate 124.950 km2 and very low and low, about 56.57 km2 of the study area.
2.3.1.7. Rainfall
Based on the classification, 60% of the study area is highly vulnerable to malaria, with an area coverage of 266.953 km2, a moderate cover of about 54.292 km2, and a very low and low share of about 16% of 69.993 km2 of the study area.
2.3.1.8. Temperature
Temperature plays a key role in the breeding system of mosquitoes. According to the classification classes, about 57% (254.830 km2) of the study area is extremely highly vulnerable to malaria spread, and about only 1% (2.495 km2) is very low vulnerable to the Malaria epidemic.
2.3.1.9. Land use/cover
Land use/cover affects the breeding of the mosquitoes directly, supervised maximum likelihood classification is applied to get the four class feature. According to the classification, 23% (101.977 km2) of the total area shares extremely high and high rank, and about 7% (30.751 km2) of the study area falls in a moderate and very low and low degree of vulnerability with area coverage of 342.323 km2.
2.3.2. Relationship of socioeconomic parameters with malaria incidences
The population distribution between the study area and proximity to the hospital is the two factors considered in socioeconomic parameters. Both population map and proximity to hospital map are presented in respectively.
2.3.2.1. Population
Based on the classification, about 95% of the study area held on very low and low vulnerability degree with an area coverage of 421.921 km2; the rest classes share only 6% area of the woreda; these are Moderate (4%), high and very high (2%).
2.3.2.2. Proximity to hospital
Based on classification from a total land area of 444.30 km2 (75%) was identified as very highly vulnerable to malaria incidence in the case study area, with a proximity distance of above 6000 m from a hospital. A buffer distance of less than 1500 m from the hospital was classified as extremely low vulnerable, representing a total land area of 7.243 km2 (2%) and also about 34.596 km2 moderately vulnerable to malaria. shows socioeconomic parameter classification and area coverage.
Table 4. Socioeconomic parameters classification and area coverage.
2.3.3. Relationship of epidemiological parameters with malaria incidences
The epidemiological parameters of the study are classified into two groups API and Pf cases both contribute to their parties in the spread of malaria in the study area. maps reveal the extent of API and Pf cases in study area respectively.
2.3.3.1. Annual Parasite Incidence (API)
API, which is the number of new infections per year per 1000 population. The API obtained from the combination of both populations and confirmed positive cases on average of three consecutive years based on classification about 53% of the study area lives in very high and highly vulnerable and 30% lives in moderate rank also, only 17% share low and very low classes. shows the classification and area coverage.
Table 5. Epidemiological parameters classification and area coverage.
2.3.3.2. Plasmodium falciparum (Pf) cases
Pf is a unicellular protozoan parasite that causes malaria, a deadly human disease. It is one of the five species of Plasmodium that cause malaria in humans and is the most prevalent and deadly species. The reclassified Pf cases of the study cover about 79% very low and low with area coverage of 351.399 km2, and only about 8% of the study area falls in very high and a high degree of vulnerability. The moderately vulnerable to malaria area covers 55.675 km2 of the study area. depicts the classification and area coverage of Pf cases in the study area.
2.3.4. Malaria vulnerability analysis
Malaria vulnerability hotspots were identified using a weighted combination of three parameters: environmental (70%), epidemiological (20%) and socioeconomic parameter (10%). The weight was assigned to the parameters with the help of experts and a review of the literature.
- a.
Hotspot for Environmental Parameters
The malaria hotspot for environmental parameters was computed using the identified environmental variables such as elevation, aspect, slope, wetness and buffer distance to drainage, land use/cover, rainfall, temperature and breeding sites (Proximity to the river) developed, and weighted by using IDRISI, and the overlaying process performed by using the weighted linear combination.
(2)
(2)
shows that about 45% (200.397 km2) of the total area coverage is extremely high and highly vulnerable to malaria, 25% (109.710 km2) is moderate and 30% (174.253 km2) of the study area falls in a very low and low degree of vulnerability.
Table 6. Hotspot for environmental parameters classification and area coverage.
The overlay of the nine environmental parameters gives the following result for the study area the map in depicts the hotspot pattern for environmental factors in the study area.
- b.
Hotspot for Epidemiological parameters
The hotspot for Epidemiological parameters obtained from API and Pf cases by applying the Weighted linear combination;
(3)
(3)
The classification shows that about 47% (206.31 km2) of the study area has very low and low vulnerability degrees, and about 38% (168.219 km2) share very high and high vulnerability degrees. The area coverage and classification information for epidemiological factors hotspots are shown in .
Table 7. Epidemiological parameters hotspot classification and area coverage.
depicts an epidemiological parameters hotspot for malaria with their degree of vulnerability in the study area.
- c.
Hotspot for Socioeconomic Parameters
The socioeconomic parameters were calculated and weighted using socioeconomic variables such as population and hospital proximity.
(4)
(4)
According to classification, approximately 86% of the study area is extremely high and highly favourable to the spread of malaria. The classification and area coverage of the classes in the study area are shown in . The map in depicts the study’s socioeconomic hotspot distributions.
Table 8. Hotspot for socioeconomic parameters classification and area coverage.
3. Results and discussion
3.1. Results
The malaria vulnerability hotspot is calculated by the output of the three parameters based on their influence on the mosquito breeding system by applying a malaria hotspot identification algorithm. The output is classified into four by using natural break; according to the malaria hotspot map, approximately 23% of the study area 103.600 km2 is lowly vulnerable to malaria, 26% of the study area 116.560 km2 is moderately vulnerable and approximately 51% (224.14 km2) is extremely high and highly vulnerable to malaria ().
Table 9. Malaria hotspot classification and area coverage.
The map in depicts the malaria hotspots in the study area in four malaria incidence vulnerability degrees.
Some interrelated environmental, epidemiological and socioeconomic factors influence the probability of a malaria outbreak. All of these factors were taken into account in this study to identify mosquito-friendly regions. The final result was divided into four malaria hotspot categories:
3.1.1. Very high
The area that is very highly vulnerable to malaria in the study area was obtained based on the contributions of parameters like elevation, wetness index, proximity to the river, temperature, land use/cover, proximity to a hospital and API. The delineated area is very highly vulnerable and has low elevation with high temperature that affect the breeding cycle of mosquitoes also, it’s near to Omo River and water cover, which increases the wetness it makes suitable habitat for mosquito facilitation and the API value in this area is low to high, and also the area shares moderate to the high value of proximity to a hospital. Based on ranking, it covers 24% of 104. 029 km2 of total area.
3.1.2. High
The area is dominated by high elevation with suitable temperature for breeding, gentle slope, high drainage density, near to river with high rainfall and high API value. The area covers 27% (119.073 km2).
3.1.3. Moderate
These areas are covered by a moderate to high value of proximity to a hospital, moderate to high population with the coverage of forest, high temperature and rainfall value, and low to high value of distance to the river with a high value of wetness. The delineated area coverage for moderately vulnerable is 26% (116.041 km2).
3.1.4. Low
It was found that areas of low priority are more likely to be in the Northeast and Southeast part of the study area with a low population, moderate to high slope, low Pf cases and low proximity to river value, accounting for 23% (102.559 km2) of the study area.
3.2. Discussion
Based on the malaria vulnerability of this study, very highly vulnerable areas have low altitudes with high temperatures affecting the mosquito breeding cycle. API value in this area is low to high, high wetness, low to high slope and area covered by water bodies. As stated by Ahmad et al. (Citation2017), the very high rating malaria hotspot area is highly dominated by tribal population, covered with dense forest, high wetness, high rainfall with a lot of perennial streams with a gentle slope and moderate to low elevation provide a most conducive environment for the growth of malaria parasite, in addition to the above factor high amount of API value is used to identify the highly vulnerable area (Kassaw et al. Citation2019).
Low vulnerable area of this study was found that areas of low priority are more likely to be in the Northeast and Southeast part of the study area with a low population, moderate to high slope, low Pf cases and high proximity to the river. Previously worked research states low vulnerable areas with low populations in general and low to sparse populations. It is covered with open forest to dense forest, low wetness, low rainfall, high slope and high elevation, which provides less favourable conditions for the malaria parasite to grow (Ahmad et al. Citation2017; Kassaw et al. Citation2019), so this study fairly agreeable with previously worked researches that worked on the area of malaria vulnerability hotspots.
The findings of this study are used as input for malaria prevention and control activities, the identification of the malaria hotspots in woreda used to allocate preventive materials like an insecticide-treated bed net (ITN), medicines and other materials to the right population in the study area. It is also used as input for policymakers and NGOs.
Several exciting aspects may be explored further by applying geospatial techniques with other technologies to predict the outbreak of malaria and malaria diffusion. It may be used as input in the country to achieve the malaria elimination program scheduled for 2025. In addition, it is important to consider population migration and deforestation as factors because these two are taking more place, especially population migration in our country and worldwide due to the COVID-19 pandemic.
4. Conclusions
This study discussed modelling malaria vulnerability hotspots in a Kindo Koysha Woreda area with the help of different data types, technologies and interpretations. Malaria is the most silent killer disease around the world, even though it is a treatable disease. Applying new technologies rather than conventional methods is important to minimize the spread and elimination. Reliable maps of malaria hotspot areas are critical for estimating the scope of the problem and, consequently, the resources required to combat malaria. Thematic products serve as a benchmark for assessing control progress and indicate which geographic areas should be prioritized.
The final result was obtained by applying the multi-criteria evaluation method. Weight was computed using pair-wise comparison in the AHP module based on the influence of factors that consider the importance of each factor to the facilitation of mosquito breeding and the interrelationships between the factors. A weighted overlay was used to map malaria vulnerability zones in the study area. Thematic products were used to show the extent of the individual factors in the study area.
The results of this study show that very high and highly vulnerable areas make up around 51% (224.14 km2), medium coverage 26% (116.560 km2) and lowly vulnerable areas also make up 23% (103.600 km2) of the study area. Mashenga, Mundena and the Fajana Mata Kebeles These very endangered hotspots from the Kindo Koysha Woreda Kebeles and also Borkeshie, Dada Kare, Moliticho, Sere Finchawa and Chereche are highly vulnerable. With low elevation, the western part of the study area near the Omo River is very prone to malaria incidence. Finally, this study confirmed that the geospatial technology based on a multi-criteria evaluation to model malaria vulnerability is more capable and efficient to apply.
5. Recommendations
The findings of this particular study have shown that RS, GIS and multi-criteria evaluation models are useful tools for studies of malaria vulnerability hotspot identification. The following are also recommended as potential research recommendations based on the results of the study:
One of the results noticed that the area near the Omo River is highly vulnerable to malaria in the study area. The Kindo Koysha Woreda health office and stakeholders should prioritize the three kebeles in prevention and control practice: Mashenga, Mundena and Fajana Mata.
The preventive action of the Woreda health and data handling system in malaria cases is conventional. Therefore, the Woreda health office should design a digital system rather than an analog.
Future researchers should have to consider population migration and deforestation as factors.
Authors’ contributions
Conceptualization, A.D.B. and H.A.W.; methodology, S.K.; software, A.D.B.; validation, H.A., S.K., P.K. and S.H.; investigation, H.G.A.; resources, A.D.B.; data curation, S.K.; writing – original draft preparation, H.A., S.K., S.H., H.A., H.G.A., H.T.H., A.T. and M.J.A.; writing – review and editing. P.K., H.A.W., H.G.A. and H.T.H.; visualization, S.K.; supervision, S.K.; funding acquisition, H.G.A. All authors have read and agreed to the published version of the manuscript.
Acknowledgement
The authors extend their appreciation to the Deanship of Scientific Research at King Khalid University for funding this work through Large Groups Project under grant number RGP 2/349/44.
Disclosure statement
The authors have no conflicts of interest to declare.
Data availability statement
The data that support the findings of this study are available on request from the corresponding author.
Additional information
Funding
References
- Ahmad F, Goparaju L, Qayum A. 2017. Studying malaria epidemic for vulnerability zones: multi-criteria approach of geospatial tools. GEP. 05(05):30–53. doi: 10.4236/gep.2017.55003.
- Ahmed A. 2014. GIS and remote sensing for malaria risk mapping, Ethiopia. Int Arch Photogramm Remote Sens Spatial Inf Sci. XL-8(8):155–161. doi: 10.5194/isprsarchives-XL-8-155-2014.
- Buke T, Gidago G. 2016. The effect of Np fertlizer rates on the yield and yield components of Taro (Colocasia esculenta L. Schott.) in Boloso-Sore Woreda Wolaita Zone, Snnpr, Ethiopia. Int J Agric Innov Res. 5(3):329–341.
- Dana DD. 2018. Spatial modelling of Malaria risk in Bayesian setting: a case study of Wolaita and Dawuro Zones in SNNPR, Ethiopia. J Nat Sci Res. 8(15):68–74.
- Eastman JR. 1999. Multi-criteria evaluation and GIS. Edinburgh, Scotland: University of Edinburg, G.B.; p. 493–502. https://www.geos.ed.ac.uk/∼gisteac/gis_book_abridged/files/ch35.pdf.
- Eniyew S. 2018. Modelling of malaria hotspot sites using geospatial technology in the north-western highlands of Ethiopia. Int J Mos Res. 5(5):59–70.
- Kassaw M, Zewdie A, Ameneshewa W. 2019. Identifying malaria epidemic prone area hotspot map by using geospatial technologies and spatial multi criteria evaluation techniques: the case of Majang Zone, Gambella Region, Ethiopia. IOSR J Hum Soc Sci. 24(11):65–79. doi: 10.9790/0837-2411056579.
- Kebede Y, Edmealem W, Alemayehu B. 2017. Malaria hazard and risk mapping using GIS based spatial multicriteria evaluation technique (SMCET) in Tekeze basin development corridor, Amhara Region, Ethiopia. J Environ Earth Sci. 7(5):76–87.
- Kumar D, Singh AK, Taloor AK, Singh DS. 2021. Recessional pattern of Thelu and Swetvarn glaciers between 1968 and 2019, Bhagirathi basin, Garhwal Himalaya, India. J Quat Inter. 575–576:227–235. doi: 10.1016/j.quaint.2020.05.017.
- Manish K. 2017. [Malaria risk zonation and validation using remote sensing and GIS in Haora district and Kolikata Municipal Corporation, West Bengal] [Masters’ thesis]. Howrah, West Bengal: Indian Institute of Engineering Science and Technology, Shibpur; p. 9–64.
- Mestewat S. 2014. Geographic information system and remote sensing based Malaria risk mapping using environmental factors: a case of Arba Minch Zuria Woreda, Southern Nations nationalities and peoples’ regional stat [Unpublished masters’ thesis]. Ethiopia: Addis Ababa Uiversity; p. 21–30.,
- Muluken B. 2019. [GIS and remote sensing-based malaria risk assessment and mapping in Raya Kobo Woreda, North Wollo, Ethiopia] [Unpublished masters’ thesis]. Ethiopia: Addis Ababa Uiversity.
- Naqvi SAA, Sajjad M, Tariq A, Sajjad M, Waseem LA, Karuppannan S, Rehman A, Hassan M, Al-Ahmadi S, Hatamleh WA. 2023. Societal knowledge, attitude, and practices towards dengue and associated factors in epidemic-hit areas: geoinformation assisted empirical evidence. Heliyon. 10(1):e23151. doi: 10.1016/j.heliyon.2023.e23151.
- Okogun GRA. 2005. Life-table analysis of Anopheles malaria vectors: generational mortality as tool in mosquito vector abundance and control studies. J Vector-Borne Dis. 42(2):45–53.
- Pam DD, Nigeria Omalu ICJ, Akintola AA, Dan AY, Kalesanwo AO, Babagana M, Muhammad SA, Ocha IM, Adeniyi KA. 2017. The role of GIS and remote sensing in the control of malaria. Online J of Heal and All Sci. 16(3):1–4.
- Qayum A, Arya R, Kumar P, Lynn AM. 2015. Socio-economic, epidemiological and geographic features based on GIS-integrated mapping to identify malarial hotspots. Malar J. 14(1):192. doi: 10.1186/s12936-015-0685-4.
- Saaty TL. 2008. Decision-making with the analytic hierarchy process. Int J Serv Sci. 1(1):83–98.
- WHO. 2019. Guidelines for malaria vector control. Guidelines for Malaria Vector Control. https://apps.who.int/iris/bitstream/handle/10665/310862/9789241550499-eng.pdf?ua=1%0A http://www.ncbi.nlm.nih.gov/pubmed/30844152.
- WHO. 2020. Malaria. https://www.who.int/news-room/fact-sheets/detail/malaria.
- WorHO. 2020. Kindo Koyisha WorHO disease registration - Kindo Koyisha WorHO. p. 1–4.
- WZHD. 2019. Wolayta zone health department report.
- Yadav K, Nath MJ, Talukdar PK, Saikia PK, Baruah I, Singh L. 2012. Malaria risk areas of Udalguri district of Assam, India: A GIS-based study. Int J Geogr Inf Sci. 26:123–131. doi: 10.1080/13658816.2011.576678.