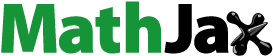
Abstract
Landscape change is intricately linked to natural resource utilization. Landscape dynamics are closely tied to land use and land cover (LULC), serving as a representation of ecosystems and human activities. In the Citarum River Basin, Indonesia, a comprehensive approach is necessary to comprehend landscape dynamics as a manifestation of human interaction with the environment. This research aims to analyze landscape dynamics and its factors that can significantly drive changes. We focused on the Cirasea Watershed, which serves as an upper region of the Citarum River Basin. Data was acquired from Landsat-series imageries from 1993 to 2023, and LULC analyses were conducted using classification and regression trees (CART), random forest (RF), and support vector machine (SVM). We analyzed seven independent variables, including slope (X1), elevation (X2), main river (X3), population (X4), central business district (X5), distance from the past settlements (X6), and accessibility (X7) using multivariate analysis. This research found that RF stands out as the optimal choice for LULC analysis over CART and SVM because it had the highest overall accuracy and overall kappa (0.91–0.92, 0.88–0.89). Notably, there was a substantial 273.43% increase in built-up areas, coupled with significant declines in plantations and horticultures. LULC changes was more pronounced in the lower areas near Bandung City. LR model highlighted X1, X3 and X6 as the significant driving forces for built-up areas expansion (r-square 0.44 with p-value < 0.01 and 95% confidence level). Without effective spatial planning, flat areas near rivers and past settlements have the greatest potential for LULC changes.
RESEARCH HIGHLIGHT
Landscape dynamics were detected best by random forest (RF), rather than classification and regression trees (CART) and support vector machine (SVM).
Landscape dynamics were dominated by increasing built-up areas with forests preservation, where plantations and horticultures were significantly reduced.
Driving factors for landscape dynamics were slope, main river, and distance from the past settlements based on the logistic regression tests.
Introduction
Landscape dynamics reflected in changes in land use have been proven to have changed environmental quality in many countries (Roy et al. Citation2022). Landscape dynamics occur massively in many countries pursuing economic growth and development targets (Rodríguez-Rodríguez et al. Citation2019; Verma and Raghubanshi Citation2019). Landscape, expressed through land use and land cover (LULC), represents the interaction between environment and human activities. (Kindu et al. Citation2015). In developing and emerging countries, landscape dynamics typically result in decresing dense vegetation cover, transforming it into human-altered lands, including agricultural, settlements, infrastructure, and industrial areas (Tariq and Mumtaz Citation2023). At present, landscape management remains highly practical, as regional government-level spatial planning policies are susceptible to modifications driven by economic interests. These changes may promote swift growth in local revenue (Sugito et al. Citation2023).
Understanding landscape dynamics requires recognizing that changes in LULC are crucial for our future (Dede et al. Citation2022a). Continuously monitoring the dynamics over an extended period is valuable not just for detecting alterations but also for understanding the spatial and temporal trends of each LULC types. This enables the development of effective measures to prevent any substantial disruption to the environmental quality caused by these changes (Sil et al. Citation2017; Mello et al. Citation2020; Daba and You Citation2022). The rapid development of computing technology has enabled us to make various estimates based on past and current data even today (Widiawaty, Ismail, et al. Citation2020). In order to achieve sustainable development objectives, a comprehensive understanding of the landscape is essential because various planning regulations related to the development process and environmental management cannot be separated from LULC (Hudalah and Woltjer Citation2007; Nugroho et al. Citation2017; Wijaya et al. Citation2017).
This occurrence underscores the necessity for conducting more comprehensive research on landscape dynamics, with a focus restricted to a watershed or river basin as an ecoregion (Dede, Sunardi, Lam, et al. 2023). Currently, many landscape dynamics studies use formal administrative units, for example, the state down to the village or neighborhood (Widiawaty, Nandi, et al. Citation2020). This situation can be understood because the development process is divided by regional interests by the local authorities (Rukmana Citation2015; Setiadi et al. Citation2023a). Dede et al. (Citation2022b) explored the combination of rural-urban interaction with administrative boundaries in a study focusing on landscape dynamics within ecoregional units. On the other hand, studies on landscape dynamics using watersheds are generally unable to understand the processes and factors that influence changes, as reported by Berget et al. (Citation2021) in Mexico, Gebreslassie (Citation2014) in Ethiopia, Prashanth et al. (Citation2023) in India, and Myers et al. (Citation2023) in the United States. Landscape dynamics, usually characterized by anthropogenic land expansion, is undoubtedly a problem because it able to reduce ecosystem services (Dede, Susiati, Widiawaty, et al. Citation2023).
Processes in landscape dynamics need to be deeply studied to understand the spatiotemporal factors as drivers, both from biogeophysical and socio-economic aspects (Dede Citation2020). In Indonesia, several studies focused on watershed landscapes merely discuss their dynamics without delving into the underlying driving forces (Setyorini et al. Citation2017; Ridwansyah et al. Citation2020; Djoharam et al. Citation2022). Many critical watersheds in Indonesia are characterized by floods, landslides, degraded land, and droughts (Susanti et al. Citation2019). The Citarum River Basin is often in national and international spotlight because this ecoregion is known as the development axis of West Java, Indonesia. Previous studies have focused on landscape changes and have not explored the driving factors in the Citarum River Basin (Sunardi et al. Citation2022; Ismail et al. Citation2024). In the other hand, a study from Widiawaty et al. (Citation2019) focused solely on urban sprawl and its typology. Understanding the driving factors that interact with LULC is essential for understanding spatial interactions.
Different from previous studies, our research aims to analyze landscape dynamics and driving factors in the Citarum River Basin. This study will produce a landscape dynamics model as a valuable input to environmental management. A unique approach was complemented by three algorithms: classification and regression trees (CART), random forest (RF), and support vector machine (SVM). These algorithms have different characteristics which require careful consideration of the Citarum River Basin. RF enhances urban vegetation mapping accuracy, surpassing traditional maximum likelihood classifiers, although sensitivity to sampling design exists (Feng et al. Citation2015; Belgiu and Drăguţ Citation2016; Georganos et al. Citation2021). In the other hand, CART classifies and resolves spectral data problems through a tree-structured approach, but bias can arise from data resampling and recursive partitioning (Loh Citation2008; Ahmadlou et al. Citation2022). Furthermore, SVM addresses image classification fragmentation and low accuracy issues (Jia and Wang Citation2011; Nandam and Patel Citation2022), yet an increase in features, especially with a small training sample, may significantly reduce classification accuracy (Pal and Foody Citation2010). The chosen algorithm, coupled with multivariate investigations, was employed to reveal driving factors using logistic regression (LR) and geographically weighted logistic regression (GWLR). This method not only enhances creative contributions to geospatial research but also holds specific significance in the Citarum River Basin management.
Methodology
This study was conducted in a sub-region of the Citarum River Basin, specifically in the Upper Citarum section, West Java Province. Upper Citarum serves as a crucial water catchment area with a strategic role in the development of West Java, particularly in Greater Bandung (Khairunnisa et al. Citation2020; Fitriana et al. Citation2023). This region is divided into smaller ecoregions, such as Cikapundung, Cirasea (Cisangkuang), Cihaur, Ciwidey, Cisangkuy, Ciminyak, and Citarik segments, with their respective watersheds (Marko et al. Citation2021). Our research focuses on the Cirasea or Cisangkuang Watershed, as shown in . Cirasea Watershed covers an area of 366.67 km2, and the main river flows from the mountainous area in the south. This area was once the focus of the Citarum Harum program (Handayani Citation2019) and is known as the textile industries center in Greater Bandung. In addition, Cirasea Watershed has developed rapidly as a development pole. Therefore, inevitable landscape changes include the expansion of residential areas and infrastructure supporting Majalaya’s industrial activities (Fathunnisa and Salami Citation2015; Sunardi et al. Citation2021; Burnama et al. Citation2022).
Data acquisition
Our study primarily examined landscape dynamics, with a focus on the classification of LULC into seven distinct types: water bodies, empty land (non-vegetated), agriculture, built-up area, forests, shrubs, grassland, plantations and horticultures (Widiawaty, Ismail, et al. Citation2020). presents more details regarding the research variables and indicators. LULC data refers to the interpretation of Landsat-series satellite imageries originating from the United States Geological Survey (USGS) for a 10-year period from 1993 to 2023. This interval was selected following the period for clear visible changes (Dede, Susiati, Widiawaty, et al. Citation2023). This research contains data originating from the dry season, which is free from cloud cover, precisely from April 1st to October 30th each year (Susiati, Dede, et al. Citation2022; Oktavia et al. Citation2023; Rachmadita et al. Citation2023;). The information underwent remote sensing processing to generate eight LULC types. Satellite imagery was interpreted using a supervised classification method (Widiawaty Citation2019). CART, RF, and SVM were selected as the algorithms for classifying remote sensing images (Lam and Noor Citation2018; Fikri et al. Citation2021; Basheer et al. Citation2022). In this context, CART pertained to 10 lead nodes, RF involved 50 trees, and SVM utilized linear kernel that considers regional characteristics.
Table 1. Variables to dataset information.
These classifications use 80 regions of interest (ROI) for each LULC type with 80% training and 20% testing. The selection of training data is based on high-resolution satellite imagery from PlanetScope and the researcher’s field experience from the previous year, ensuring purposive selection of homogeneous regions (pixels) (Patnala et al. Citation2023). In general, the training is larger than the testing to train algorithms to accurately detect LULC (Feizizadeh et al. Citation2023). All bands from three satellite images from Landsat-5 TM (1990), Landsat-7 ETM+ (2003 and 2013), and Landsat-8 OLI (2023) are used. LULC data was processed using Google Earth Engine as a cloud-based platform for processing satellite imagery and QGIS version 3.28 (Firenze) after the image selection stages (cloud cover less than 10%), cloud masking, and preprocessing to display earth surface phenomena with minimal errors (Sano et al. Citation2007; Zhu and Woodcock Citation2014; Madzen and Lam Citation2017). In addition, independent variable data, which act as driving forces, comes from various credible sources. To facilitate further analysis, geospatial data must be used (Pebesma and Bivand Citation2023). The slope comes from height difference information, which is analyzed using the slope analysis method (Böhme et al. Citation2011), whereas other data must be analyzed using buffering and density analysis (Susiati, Widiawaty, et al. Citation2022). presents details of data processing on biogeophysical and socioeconomic factors in order to provide information on driving factors. The independent variables were validated in prior landscape dynamics studies in Indonesia and served as significant geospatial driving factors (Sodikin et al. Citation2017; Widiawaty, Ismail, et al. Citation2020; Dede et al. Citation2022b).
Table 2. Data acquisition for biogeophysical and socio-economic factors.
Data validation
To ensure the quality of landscape information, validation of the interpretation results involves 86 ground-check points (field survey) adjusted to their allocation value in the Cirasea Watershed in 2023 for each LULC type (). The ground-check points were selected through a purposive sampling method, considering the distribution and accessibility of locations. Essential information includes geographic coordinates, photographs (sketches), and timestamps for data collection (Murayama Citation2012; Chaminé et al. Citation2021). In the validation phase, both overall accuracy and overall kappa were employed. Overall kappa demonstrated superior discernment as a measure for accurate classification in remote sensing data, exhibiting enhanced discrimination between classes compared to overall accuracy. (Li et al. Citation2019). Landscape information was declared valid after testing the overall accuracy and overall kappa with thresholds between 0.70 and 0.96 with a confidence level of 95% (Prasad et al. Citation2022). Determining the threshold value maintains ideal landscape data and avoids underfitting or overfitting (Montesinos-López et al. Citation2022; Widiawaty et al. Citation2022). The series of landscape data acquisition and validation is presented in .
Data analysis
Landscape dynamics were analyzed by comparing LULC information over 10 years following the gain and loss method (Widiawaty Citation2019). These landscape changes are known from the changes in each LULC area. An increase trend signifies a gain, whereas a decrease indicates a loss. Analyzing these gains and losses provides change values in area units (km2) or percentages (%) (Ismail et al. Citation2020). This descriptive landscape change pattern can be clearly depicted using a trend line. Changes in each LULC were represented in binary form, categorizing them as either changed or unchanged. These binary representations served as the dependent variable (Toma et al. Citation2023). The effect was assessed based on the coefficient of determination and the p-value at a 95% confidence level (Danapriatna et al. Citation2023). To prevent symptoms of multicollinearity, typically indicated by an r-value exceeding 0.90 with statistical significance, it is crucial for the various factors serving as independent variables to establish their relationships with one another (Widiawaty et al. Citation2022).
The influence of these factors on landscape changes in the Cirasea Watershed was determined by LR and GWLR analysis using PSPP version 1.6.2. LR models the relationship between independent and dependent variables using categorical or binary data (Tsangaratos and Ilia Citation2016). GWLR involves geographic factors that combine non-stationary and categorical parameters (Caraka and Yasin Citation2017; Nkeki and Asikhia Citation2019). In this investigation, the utilization of binary numbers derived from LULC change data is well-suited for analysis employing the regression method outlined in EquationEquation 1(1)
(1) . The GWLR model employed in this study incorporates both adaptive-Gaussian and fixed-Gaussian kernels. The model is deemed superior if the values for deviance (Dev), degrees of freedom (DoF), Dev per DoF, and Akaike information criterion (AIC) are lower compared to the LR model without geographic weighting as per EquationEquation 2
(2)
(2) (De and Acquah Citation2010; Dede et al. Citation2022a).
(1)
(1)
where Xji is the observed value of the predictor variable at location (ui, vi), βj (ui, vi) is the regression coefficient for each location (ui, vi), and p is the number of predictor variable parameters.
(2)
(2)
where ln is the natural logarithm, p the probability value, α the regression constant, and β the predictor coefficient of the independent variable.
The GWLR and LR models were then tested for feasibility using the −2 log-likelihood value, Nagelkerke test, and Hosmer-Lemeshow test provided by statistical computing software (Park Citation2013; Smith and McKenna Citation2013). The 2 log-likelihood value and the Nagelkerke test (r-square) are declared significant if the p-value 0.05, whereas the Hosmer-Lemeshow test states otherwise (Widarjono Citation2015; Barbić and Palić 2016; Ahmed Citation2017). These regression models must meet the heterogeneity aspect by referring to the Breusch-Pagan test and avoid multicollinearity using the variance inflation factor (VIF) method – it must be no more than 10.00 (Vu et al. Citation2020; Solekha and Qudratullah Citation2022). The research variables showed heterogeneity because the Breusch-Pagan value reached 1257.71 (p-value 0.00 < 0.05) and was free from multicollinearity. The VIF reached 4.30, making it suitable for further analysis.
Results
Three supervised classification algorithms yielded varying results regarding LULC in the Cirasea Watershed. RF exhibited the highest level of accuracy in detecting the landscape, as delineated in . RF demonstrated superiority over both CART and SVM, as evidenced by the utilization of ‘testing data’ in Google Earth Engine and ‘field survey data’ conducted in 2023, achieving an overall accuracy of 0.91–0.92 and an overall kappa of 0.88–0.89, respectively. SVM exhibited a lower level of accuracy in LULC detection compared to alternative methods due to its tendency to over-detect forests and misclassify rice fields as plantations and horticultures (). Conversely, SVM also demonstrated reduced precision when identifying grassland, marsh, and non-vegetated areas. Meanwhile, CART exhibited a spatial detection pattern for LULC with relatively favorable outcomes, achieving accuracy levels between those of RF and SVM. However, CART displayed a narrower tendency towards over-detection in grassland, marsh, and non-vegetated areas. CART was not the least effective in detecting LULC associated with human cultivation, particularly on agricultural lands, built-up areas, plantations, and horticulture. However, initial observations suggest that CART yields results akin to those of RF. Discrepancies in detection among grassland, marsh, and non-vegetated areas stem from the closely clustered reflectance values of these entities, rendering them difficult to distinguish without field verification (Tong et al. Citation2018; Gao et al. Citation2023).
Table 3. Accuracy results between CART, RF and SVM.
Consistency was observed in the results concerning the identification of developed regions within the Cirasea Watershed. Patterns, distributions, and extents exhibited no significant disparities, suggesting that various supervised classification algorithms adeptly detect landscapes heavily influenced by human activity. RF emerged as the most suitable algorithm for detecting landscape dynamics in this watershed, considering the minimal thresholds for overall accuracy and kappa accuracy. RF effectively discerns LULC features such as agricultural land, built-up areas, forests, as well as plantations and horticultures, which represent the predominant landscapes therein. RF demonstrated adaptability in detecting landscapes ranging from highly natural to those dominated by human activity. Discrepancies in satellite image values are effectively captured by RF, facilitating the production of precise LULC information (Belgiu and Drăguţ Citation2016; Santos et al. Citation2019).
LULC changes
The Cirasea watershed is situated in the southern part of Citarum River Basin. Three decades ago, the landscape primarily comprised agricultural land and forests associated with plantations and holticultures. This landscape configuration has persisted for the past decade (); however, by 2023, notable changes have ensued due to the continued expansion of built-up areas, resulting in a drastic alteration exceeding 13% (). The proliferation of built-up areas has led to the establishment of new villages and housing complexes. Furthermore, it is pertinent to highlight the influence of the textile industry on shaping landscape transformations in the Cirasea Watershed. Built-up areas for residential, amenity, and economic purposes exhibit spatial interconnectedness, with their locations relatively proximate to each other (Widiawaty, Ismail, et al. Citation2020). The downstream region of the Cirasea Watershed serves as the epicenter for the textile industry’s development in Greater Bandung, evidenced by the presence of multinational manufacturers situated in close proximity to highways and settlements spanning from the eastern (Majalaya) to western (Baleendah) sides (Handayani Citation2019; Ripaldi Citation2023). This phenomenon has dynamically altered the proportions of the four LULC types during the period spanning 1993 to 2023 ().
Table 4. LULC dynamics in the Cirasea Watershed.
Gain and loss analysis reveals a more than 270% increase in built-up area over the past three decades, accompanied by reductions in agricultural land, grassland, marsh, non-vegetated land, plantations, and horticulture (). Notably, forests have witnessed an increase in both area and proportion within the Citarum River Basin since 2003, attributable to landscape restoration initiatives and structured governmental efforts to mitigate critical land degradation (Pitaloka et al. Citation2020; Ayyasy et al. Citation2021). This trend contrasts with agricultural land, which experienced significant changes during 1993–2003, primarily characterized by reductions to accommodate various built-up areas. Over the past decade, the built-up area has expanded by over 100%, underscoring the heightened demand for land for residential and industrial purposes. Additionally, water bodies have expanded, facilitated by the construction of irrigation canals and small dams to cater to the requirements of industrial and agricultural activities for raw water.
Table 5. Gain and loss of LULC in 1993–2023.
Driving factors in landscape dynamics
The built-up area in the Cirasea Watershed continues to expand, nearly tripling between 1993 and 2023. Investigating this phenomenon using the LR and GWLR models to discern the spatial-temporal factors influencing its dynamics is of considerable interest. The driving forces are categorized into three biogeophysical and four socio-economic factors with distinct spatial distribution characteristics, as depicted in . As independent variables, these driving factors can construct a model with an accuracy level of up to 94.70%. This figure is significant as it yields −2 log-likelihood value lower than that of the model without log likelihood, with a difference of 222.70 (). Among the three logistic models examined, all produced significant r-square values ranging from 0.44 to 0.61 (p-value 0.01, 95%), indicating that the independent variables can elucidate landscape dynamics, particularly regarding open-land expansion in the Cirasea Watershed (). The Hosmer-Lemeshow test yielded results of 4.94 (p-value > 0.76, 95%) for both GWLR models in explicating the driving forces behind LULC dynamics (). However, these two GWLR models cannot be employed for LULC analysis due to the absence of spatial variability as a local geographical variation (Mayfield et al. Citation2018).
Table 6. Validation of the LR model.
Table 7. Hosmer-Lemeshow Test and simultaneous determination of driving forces.
Table 8. Comparison of various logistic regression models and their variances.
The positive AIC values, with magnitudes almost identical between spatial and non-spatial regression models, imply that classic LR is better suited to elucidate this phenomenon (Nakaya Citation2012; Mondal et al. Citation2015). The high r-square values (0.52 and 0.61) in the logistic regression models and the two GWLRs raise concerns regarding overfitting. In logistic regression models, low r-square values are common, typically around 0.40 if the model meets goodness of fit (Hosmer et al. Citation2013). The lack of spatial variability stems from the extensive and intensive growth of built land in the downstream part of the Cirasea Watershed, driven by numerous forces. Consequently, the LR model emerges as the most suitable model, with a driving force determination of 44% and omnibus test results of 222,697 (p-value 0.00 and dF 8.00, 95%). According to the LR model, slope (X1), the main river (X3), and distance from past settlements (X6) have emerged as significant driving forces for built-up area expansion in the Cirasea Watershed. New built-up areas tend to appear in areas with gentle slopes near surface water sources (rivers), characterized by a negative coefficient and close proximity to settlement patches and matrices from the previous period. The LR model for landscape dynamics is expressed by EquationEquation 3(3)
(3) , as derived from .
Table 9. Parameters of the LR model.
Within the LR model, only three driving forces exhibit significance, with no significant correlation observed with an r-value less than 0.10 (p-value > 0.05, 95%), as illustrated in . The correlation between slope (X1) and the main river (X3) is very weak (0.08), as is the correlation between slope (X1) and the distance from past settlements (X6) (0.03), and between the main river (X3) and the distance from past settlements (X6) (0.02). Overall, the correlation pattern between independent variables is notably low, with most values falling below 0.40, further indicating the absence of multicollinearity (Das Citation2019; Frost Citation2020; Patil and Franken Citation2021). Correlations ranging from weak to high among independent variables that were not significant for the LR model are observed. The highest correlation is between the distance from the central business district (CBD) (X5) and elevation (X2), and population (X4), reaching 0.83 and 0.58, respectively.
Table 10. Correlation between driving forces as independent variables.
Discussion
The dynamics of Land Use and Land Cover (LULC) exhibit three primary patterns: increasing, decreasing, or stagnant (), contingent upon changes in area over the past 30 years. Agricultural land has dwindled by several dozen km2, yet in the last decade, a reversal has occurred, resulting in a slight increase, though not as significant as in 1993. This trend is also noticeable in grasslands, albeit with a narrower area. Marsh and non-vegetated areas underwent a pattern characterized by an initial upward trajectory followed by a sharp decline. This shift stemmed from the repurposing of these lands for other uses, previously considered abandoned (Jalayer et al. Citation2022). Over the initial two decades, plantations and horticultures experienced growth, but in the last decade, there has been a notable loss of land, exceeding 20 km2. The dynamics of LULC in the Cirasea Watershed indicate an increasing trend in built-up areas. This escalation is commonplace, reflecting similar phenomena observed elsewhere, particularly in peri-urban regions (Dede et al. Citation2019; Admasu et al. Citation2023a, Citation2023b).
Over the past decade, built-up areas have expanded by over 20 km2, with exponential growth patterns attributed to industrialization and real estate development, particularly to accommodate workers originating from outside the region (Parveen et al. Citation2022). Ultimately, these workers chose to settle near industrial sites to mitigate commute times, as the main road from Baleendah to Majalaya often experienced traffic congestion during peak commuting hours, notwithstanding occasional flooding (Junnaedhi et al. Citation2017; Setiadi et al. Citation2023b). Conversely, the expansion of built-up areas follows a pattern not solely reliant on forests or agricultural land but can also arise from plantations and horticultures, and may even encroach upon previously marshy or non-vegetated areas (). The sustainability of these two land types is relatively assured owing to forest conservation efforts and enhanced irrigation practices. Forest protection is rigorously enforced by both governmental and community-led initiatives through community-based management (Idris et al. Citation2019). Since 2003, forests have continued to expand, surpassing the area recorded in 1993, a period marked by extensive deforestation in Indonesia and other developing countries due to the extensive utilization of tropical forest resources (Liping et al. Citation2018)
Figure 7. Landscape dynamics model in the Cirasea Watershed with its significant driving forces and potential impact.

LULC in the Cirasea Watershed will continue to be dynamic as because the population of working-age is still to grow. There will be a definite need for built-up area to accommodate economic activities and housing (Lam et al. Citation2020). The peri-urban landscape pattern encourages the emergence of built-up area with a sprawl pattern (Widiawaty et al. Citation2019), especially the Cirasea Watershed right to the south of Bandung City, which is connected via arterial and collector roads. Cirasea’s position as part of Megapolitan Bandung Raya while maintaining forests cannot be separated from the basin-like physiographic ‘Cekungan Bandung’ (Mulyadi et al. Citation2020). The expansion of built-up area in Cirasea is predominantly located in flat to undulating areas, the hilly and mountainous areas at the top of the Bandung Basin remain forested due to spatial zoning (Truax et al. Citation2015; Aisharya et al. Citation2022). Expansion of built-up areas tends to be close to the main rivers, which raises concerns about this. The Cirasea River has the potential to become a dumping ground for household and industrial waste, ultimately disrupting the aquatic ecosystem of the Cirasea Watershed (Alamanda et al. Citation2016).
In the forthcoming years or decades, future research is imperative to examine the potential landscape dynamics of the Cirasea Watershed and the Citarum River Basin. A profound understanding of various driving factors forms the essential foundation for designing a dynamic model capable of accommodating scenarios tailored to current conditions, such as socio-economic projections and government spatial planning directions (He et al. Citation2008). Furthermore, the scope needs expansion by incorporating new independent variables such as land prices, vulnerability to various disasters, and public perceptions of space (Widiawaty and Dede Citation2018). To enhance the richness of LULC types, landscape dynamics analysis requires more detailed data sources, including the utilization of unmanned aerial vehicles (UAVs) (Gomez et al. Citation2022). Exploring optimized and integrated LULC algorithms can be a crucial step in refining landscape classification methodologies (Escobar-López et al. Citation2024). Overall, this research marks the beginning of further development, and the current limitations, which have not reached a very detailed scale, present opportunities for further studies. Additionally, future research should explore the implications of landscape dynamics on environmental parameters, encompassing air, water, soil, and other species, transcending their impact solely on humans (Werner and McNamara Citation2007; Grimaldi et al. Citation2014; Melesse and Abtew Citation2016; Olson et al. Citation2017).
Conclusion
The RF algorithm emerges as the most suitable for analyzing landscape dynamics in the Cirasea watershed. Overall accuracy and kappa values are higher for RF than for CART and SVM. Over the 30-year period (1993–2023), LULC changes were predominantly characterized by the expansion of built-up areas and a reduction in plantations and horticultures. Simultaneously, the LR model identified all three independent variables as driving forces: slope (X1), main river (X3), and distance from past settlements (X6), influencing 44% of the LULC changes. These variables exhibit negative coefficient notation, signifying that built-up areas tend to manifest in locations that are flat and close to water sources. The increasing forest area in the Cirasea Watershed is encouraging despite pollution resulting from industrial activities and critical lands. However, this study is limited as it examines landscape change phenomena without developing a spatial-temporal prediction model. Efforts to control the expansion of built-up areas must secure structural and non-structural support involving active participation from all stakeholders, including government, society, academics, researchers, industry, and non-governmental organizations. The Indonesian government should broaden the Citarum Harum program to encompass landscaping beyond its previous exclusive focus on the restoration of the river water ecosystem. Local authorities must enforce stringent legal measures for housing and industrial developers, including authorization revocation, imposing high sanctions, and promoting willingness to pay for ecosystem services.
Disclosure statement
No potential conflict of interest was reported by the author(s).
References
- Admasu S, Yeshitela K, Argaw M. 2023a. Impact of land use land cover changes on ecosystem service values in the Dire and Legedadi watersheds, central highlands of Ethiopia: implication for landscape management decision making. Heliyon. 9(4):e15352. doi: 10.1016/j.heliyon.2023.e15352.
- Admasu S, Yeshitela K, Argaw M. 2023b. Impacts of land use land cover changes and climate variability on water yield in the Dire and Legedadi watersheds central Ethiopia. Water Conserv Sci Eng. 8(1):14. doi: 10.1007/s41101-023-00188-x.
- Ahmadlou M, Ebrahimian Ghajari Y, Karimi M. 2022. Enhanced classification and regression tree (CART) by genetic algorithm (GA) and grid search (GS) for flood susceptibility mapping and assessment. Geocarto International. 37(26):13638–13657. doi: 10.1080/10106049.2022.2082550.
- Ahmed LA. 2017. Using logistic regression in determining the effective variables in traffic accidents. AMS. 11:2047–2058. doi: 10.12988/ams.2017.75179.
- Aisharya IY, Gunawan B, Abdoellah OS, Gunawan W, Dewa JJPK. 2022. Role and interaction between local actors in community-based forest management in Upper Citarum Hulu. JPSL. 12(2):335–351. doi: 10.29244/jpsl.12.2.335-351.
- Alamanda DT, Amani H, Ayuningtias HG, Hidayatullah DS, Perdana TR. 2016. Conflict resolution using graph model for conflict resolution: case study Majalaya’s textile industries waste pollution. Proceedings of the 3rd International Seminar and Conference on Learning Organization. Atlantis Press; p. 152–156. doi: 10.2991/isclo-15.2016.29.
- Ayyasy MNI, Herdiansyah H, Kosandi M. 2021. Accelerating Citarum river restoration by involving peculiar multi-stakeholders approach. IOP Conf Ser Earth Environ Sci. 802(1):012026. doi: 10.1088/1755-1315/802/1/012026.
- Barbić D, Palić I, Bahovec V. 2016. Logistic regression analysis of financial literacy implications for retirement planning in Croatia. CRORR. 7(2):319–331. doi: 10.17535/crorr.2016.0022.
- Basheer S, Wang X, Farooque AA, Nawaz RA, Liu K, Adekanmbi T, Liu S. 2022. Comparison of land use land cover classifiers using different satellite imagery and machine learning techniques. Remote Sens. 14(19):4978. doi: 10.3390/rs14194978.
- Belgiu M, Drăguţ L. 2016. Random forest in remote sensing: a review of applications and future directions. ISPRS J Photogramm Remote Sens. 114:24–31. doi: 10.1016/j.isprsjprs.2016.01.011.
- Berget C, Verschoor G, García-Frapolli E, Mondragón-Vázquez E, Bongers F. 2021. Landscapes on the move: land-use change history in a Mexican agroforest frontier. Land. 10(10):1066. doi: 10.3390/land10101066.
- Böhme M, Saintot A, Henderson IHC, Henriksen H, Hermanns RL. 2011. Rock slope instabilities in Sogn and Fjordane County, Norway: a detailed structural and geomorphological analysis. SP. 351(1):97–111. doi: 10.1144/SP351.5.
- Burnama NS, Rohmat FIW, Farid M, Kuntoro AA, Kardhana H, Solehudin S. 2022. Rainfall-runoff analysis using satellite data in the Majalaya Watershed. ITB Graduate School Conf. 2(1):321–331. https://gcs.itb.ac.id/proceeding-igsc/index.php/igsc/article/view/92.
- Caraka RE, Yasin H. 2017. Geographically weighted regression (GWR): sebuah pendekatan regresi geografis. Yogyakarta: Mobius. https://www.semanticscholar.org/paper/Geographically-weighted-regression-%28GWR%29-%3A-sebuah-Caraka-Yasin/a1ce9f60b34bac830cb50e8662c10c506e992224.
- Chaminé HI, Pereira AJSC, Teodoro AC, Teixeira J. 2021. Remote sensing and GIS applications in earth and environmental systems sciences. SN Appl Sci. 3(12):870. doi: 10.1007/s42452-021-04855-3.
- Daba MH, You S. 2022. Quantitatively assessing the future land-use/land-cover changes and their driving factors in the upper stream of the Awash River based on the CA–Markov model and their implications for water resources management. Sustainability. 14(3):1538. doi: 10.3390/su14031538.
- Danapriatna N, Ismarani I, Lutfiadi R, Dede M. 2023. Effect of straw compost (Oryza sativa L.) on crop production. JTAS. 46(3):1047–1062. doi: 10.31219/osf.io/9c7px.
- Das P. 2019. Analysis of collinear data: multicollinearity. In: Das P, editor. Econometrics in theory and practice: analysis of cross section, time series and panel data with Stata 15.1. Cham: Springer; p. 137–151. doi: 10.1007/978-981-32-9019-8_5.
- De H, Acquah G. 2010. Comparison of Akaike information criterion (AIC) and Bayesian information criterion (BIC) in selection of an asymmetric price relationship. J Dev Agric Econ. 2:1–6.
- Dede M. 2020. Pemodelan spasial dinamis perubahan penggunaan lahan dan implikasinya terhadap kualitas lingkungan di peri-urban Cirebon [master thesis]. Universitas Padjadjaran. https://kandaga.unpad.ac.id:3000/koleksi/repository/item/250120190001.
- Dede M, Asdak C, Setiawan I. 2022a. Spatial dynamics model of land use and land cover changes: a comparison of CA, ANN, and ANN-CA. Regist j Ilm Teknol Sist Inf. 8(1):38–49. doi: 10.26594/register.v8i1.2339.
- Dede M, Asdak C, Setiawan I. 2022b. Spatial-ecological approach in Cirebon’s peri-urban regionalization. IOP Conf Ser Earth Environ Sci. 1089(1):012080. doi: 10.1088/1755-1315/1089/1/012080.
- Dede M, Pramulatsih GP, Widiawaty MA, Ramadhan YRR, Ati A. 2019. Dinamika suhu permukaan dan kerapatan vegetasi di Kota Cirebon. JMKG. 6(1):23–31. doi: 10.36754/jmkg.v6i1.111.
- Dede M, Sunardi S, Lam KC, Withaningsih S. 2023. Relationship between landscape and river ecosystem services. Global J Environ Sci Manage. 9:637–652. doi: 10.22035/gjesm.2023.03.18.
- Dede M, Susiati H, Widiawaty MA, Kuok-Choy L, Aiyub K, Asnawi NH. 2023. Multivariate analysis and modeling of shoreline changes using geospatial data. Geocarto International. 38(1):2159070. doi: 10.1080/10106049.2022.2159070.
- Djoharam V, Widiatmaka W, Marimin M, Panuju DR, Tarigan SD. 2022. Vegetation dynamics of Sangkub watershed in North Sulawesi Province indicated by NDVI of Landsat data. J Degrade Min Land Manage. 10(1):3991. doi: 10.15243/jdmlm.2022.101.3991.
- Escobar-López A, Castillo-Santiago MÁ, Mas JF, Hernández-Stefanoni JL, López-Martínez JO. 2024. Identification of coffee agroforestry systems using remote sensing data: a review of methods and sensor data. Geocarto International. 39(1):2297555. doi: 10.1080/10106049.2023.2297555.
- Fathunnisa F, Salami IRS. 2015. Content of heavy metals in the water and water hyacinth (Eichhornia crassipes) in water bodies receiving wastewater from textile industry (Case study: cikacembang River, Majalaya Districts, Bandung Regency). The Third Joint Seminar of Japan and Indonesia Environmental Sustainability and Disaster Prevention (3rd ESDP-2015); p. 207–217.
- Feizizadeh B, Omarzadeh D, Kazemi Garajeh M, Lakes T, Blaschke T. 2023. Machine learning data-driven approaches for land use/cover mapping and trend analysis using Google Earth Engine. J Environ Plann Manage. 66(3):665–697. doi: 10.1080/09640568.2021.2001317.
- Feng Q, Liu J, Gong J. 2015. UAV remote sensing for urban vegetation mapping using random forest and texture analysis. Remote Sens. 7(1):1074–1094. doi: 10.3390/rs70101074.
- Fikri AS, Setiawan F, Violando WA, Muttaqin AD, Rahmawan F. 2021. Analisis perubahan penutupan lahan menggunakan Google Earth Engine dengan algoritma CART (studi kasus: Wilayah pesisir Kabupaten Lamongan. Provinsi Jawa Timur). Prosiding Forum Ilmiah Tahunan (FIT)-Ikatan Surveyor Indonesia (ISI).
- Fitriana F, Yudianto D, Sanjaya S, Roy AFV, Seo YC. 2023. The assessment of Citarum river water quality in Majalaya District, Bandung Regency. RS. 17(1):37–46. doi: 10.21776/ub.rekayasasipil.2023.017.01.6.
- Frost J. 2020. Regression analysis: an intuitive guide for using and interpreting linear models. Pennsylvania: Statistics By Jim Publishing.
- Gao X, Hao F, Pi W, Zhu X, Zhang T, Bi Y, Zhang Y. 2023. Identification and classification of degradation-indicator grass species in a desertified steppe based on HSI-UAV. Spectroscopy. 38:14–20. doi: 10.56530/spectroscopy.dr5881c1.
- Gebreslassie H. 2014. Land use-land cover dynamics of Huluka watershed, Central Rift Valley, Ethiopia. International Soil and Water Conservation Research. 2(4):25–33. doi: 10.1016/S2095-6339(15)30055-1.
- Georganos S, Grippa T, Niang Gadiaga A, Linard C, Lennert M, Vanhuysse S, Mboga N, Wolff E, Kalogirou S. 2021. Geographical random forests: a spatial extension of the random forest algorithm to address spatial heterogeneity in remote sensing and population modelling. Geocarto International. 36(2):121–136. doi: 10.1080/10106049.2019.1595177.
- Gomez C, Setiawan MA, Listyaningrum N, Wibowo SB, Hadmoko DS, Suryanto W, Darmawan H, Bradak B, Daikai R, Sunardi S, et al. 2022. LiDAR and UAV SfM-MVS of Merapi volcanic dome and crater rim change from 2012 to 2014. Remote Sens. 14(20):5193. doi: 10.3390/rs14205193.
- Grimaldi M, Oszwald J, Dolédec S, Hurtado M, del P, de Souza Miranda I, Arnauld de Sartre X, Assis WS, de Castañeda E, Desjardins T, et al. 2014. Ecosystem services of regulation and support in Amazonian pioneer fronts: searching for landscape drivers. Landscape Ecol. 29(2):311–328. doi: 10.1007/s10980-013-9981-y.
- Handayani S. 2019. Majalaya as the center for textile industry in spatial historical perspectives. IOP Conf Ser Earth Environ Sci. 243:012167. doi: 10.1088/1755-1315/243/1/012167.
- He C, Okada N, Zhang Q, Shi P, Li J. 2008. Modelling dynamic urban expansion processes incorporating a potential model with cellular automata. Landscape Urban Plann. 86(1):79–91. doi: 10.1016/j.landurbplan.2007.12.010.
- Hosmer DW, Lemeshow S, Sturdivant RX. 2013. Applied logistic regression. New Jersey: John Wiley & Sons.
- Hudalah D, Woltjer J. 2007. Spatial planning system in transitional Indonesia. Int Plann Stud. 12(3):291–303. doi: 10.1080/13563470701640176.
- Idris AMS, Permadi ASC, Kamil AI, Wananda BR, Taufani AR. 2019. Citarum Harum Project: a restoration model of river basin. Indonesian J Dev Plann. 3(3):310–324. doi: 10.36574/jpp.v3i3.85.
- Ismail A, Dede M, Widiawaty M. 2020. Urbanisation and HIV in Bandung City (health geography perspectives). BPK. 48(2):139–146. doi: 10.22435/bpk.v48i2.2921.
- Ismail A, Greve K, Widiawaty MA, Dede M, Nandi N. 2024. Effect of vegetation cover changes on the runoff coefficient characteristics in the Cisangkuy Watershed, Indonesia. Anuário do Instituto de Geociências. 47:1–11.
- Jalayer S, Sharifi A, Abbasi-Moghadam D, Tariq A, Qin S. 2022. Modeling and predicting land use land cover spatiotemporal changes: a case study in Chalus Watershed, Iran. IEEE J Sel Top Appl Earth Observ Remote Sens. 15:5496–5513. doi: 10.1109/JSTARS.2022.3189528.
- Jia X, Wang J. 2011. Research of remote sensing classification about land survey based on SVM. 2011 2nd International Conference on Artificial Intelligence, Management Science and Electronic Commerce (AIMSEC), 3230–3233. doi: 10.1109/AIMSEC.2011.6011377.
- Junnaedhi IDGA, Riawan E, Suwarman R, Hadi TW, Lubis A, Trilaksono NJ, Rahayu R, Kombara P, Waskito R, Oktora HE, et al. 2017. Majalaya flood early warning system: a community based approach. IOP Conf Ser Earth Environ Sci. 71(1):012013. doi: 10.1088/1755-1315/71/1/012013.
- Khairunnisa F, Tambunan MP, Marko K. 2020. Estimation of soil erosion by USLE model using GIS technique (A case study of upper Citarum Watershed). IOP Conf Ser Earth Environ Sci. 561(1):012038. doi: 10.1088/1755-1315/561/1/012038.
- Kindu M, Schneider T, Teketay D, Knoke T. 2015. Drivers of land use/land cover changes in Munessa-Shashemene landscape of the south-central highlands of Ethiopia. Environ Monit Assess. 187(7):452. doi: 10.1007/s10661-015-4671-7.
- Lam KC, Jaafar M, Halime LAR, Asnawi NH, Rose RAC. 2020. Analysis of land use and land cover change of Sidon City, Lebanon. Geografia-Malaysian J Soc Space.16(3):108–120. doi: 10.17576/geo-2020-1603-09.
- Lam KC, Noor N. 2018. Kajian perubahan guna tanah menerusi aplikasi penderiaan jauh. Geografia-Malaysian J Soc Space. 14(2):108–124. doi: 10.17576/geo-2018-1402-09.
- Li W, Liu H, Wang Y, Li Z, Jia Y, Gui G. 2019. Deep learning-based classification methods for remote sensing images in urban built-up areas. IEEE Access. 7:36274–36284. doi: 10.1109/ACCESS.2019.2903127.
- Liping C, Yujun S, Saeed S. 2018. Monitoring and predicting land use and land cover changes using remote sensing and GIS techniques—a case study of a hilly area, Jiangle, China. PLoS One. 13(7):e0200493. doi: 10.1371/journal.pone.0200493.
- Loh W-Y. 2008. Classification and regression tree methods. In: Encyclopedia of statistics in quality and reliability. Chichester: John Wiley & Sons, Ltd. doi: 10.1002/9780470061572.eqr492.
- Madzen AA, Lam KC. 2017. Respons fenologi tumbuhan terhadap taburan hujan di johor menggunakan data indeks tumbuhan satelit MODIS-Aqua. JSM. 46(3):421–428. doi: 10.17576/jsm-2017-4603-09.
- Marko K, Sutjiningsih D, Kusratmoko E. 2021. Watershed health changes based on vegetated land cover in the Upper Citarum Watershed, West Java, Province, Indonesia. IOP Conf Ser Earth Environ Sci. 940(1):012045. doi: 10.1088/1755-1315/940/1/012045.
- Mayfield H, Lowry J, Watson C, Kama M, Nilles E, Lau C. 2018. Use of geographically weighted logistic regression to quantify spatial variation in the environmental and sociodemographic drivers of leptospirosis in Fiji: a modelling study. Lancet Planetary Health. 2(5):e223–e232. doi: 10.1016/S2542-5196(18)30066-4.
- Melesse AM, Abtew W, editors. 2016. Landscape dynamics, soils and hydrological processes in varied climates. Cham: Springer International Publishing. doi: 10.1007/978-3-319-18787-7.
- Mello Kd, Taniwaki RH, Paula FR, de Valente RA, Randhir TO, Macedo DR, Leal CG, Rodrigues CB, Hughes RM. 2020. Multiscale land use impacts on water quality: assessment, planning, and future perspectives in Brazil. J Environ Manage. 270:110879. doi: 10.1016/j.jenvman.2020.110879.
- Mondal B, Das DN, Dolui G. 2015. Modeling spatial variation of explanatory factors of urban expansion of Kolkata: a geographically weighted regression approach. Model Earth Syst Environ. 1(4):29. doi: 10.1007/s40808-015-0026-1.
- Montesinos-López OA, Montesinos-López A, Crossa J. 2022. Multivariate statistical machine learning methods for genomic prediction. Cham: Springer International Publishing. doi: 10.1007/978-3-030-89010-0.
- Mulyadi A, Dede M, Widiawaty MA. 2020. Spatial interaction of groundwater and surface topographic using geographically weighted regression in built-up area. IOP Conf Ser Earth Environ Sci. 477(1):012023. doi: 10.1088/1755-1315/477/1/012023.
- Murayama Y, editor. 2012. Progress in geospatial analysis. Tokyo: Springer Japan. doi: 10.1007/978-4-431-54000-7.
- Myers D, Jones D, Oviedo-Vargas D, Schmit J, Ficklin D, Zhang X. 2023. Seasonal variation in landcover estimates reveals sensitivities and opportunities for environmental model. EGUsphere. 2023:1–22. doi: 10.21203/rs.3.rs-2289167/v3.
- Nakaya T. 2012. GWR 4.09 user manual: windows application for geographically weighted regression modelling. Maynooth: GWR4 Development Team. https://www.semanticscholar.org/paper/GWR-4-.-09-User-Manual-GWR-4-Windows-Application-Nakaya/80959614149d242970797725838a614f0300c76d.
- Nandam V, Patel PL. 2022. A novel hybrid approach using SVM and spectral indices for enhanced land use land cover mapping of coastal urban plains. Geocarto Int. 37(16):4714–4736. doi: 10.1080/10106049.2021.1899300.
- Nkeki FN, Asikhia MO. 2019. Geographically weighted logistic regression approach to explore the spatial variability in travel behaviour and built environment interactions: accounting simultaneously for demographic and socioeconomic characteristics. Appl Geogr. 108:47–63. doi: 10.1016/j.apgeog.2019.05.008.
- Nugroho HYSH, Veen A, van der Skidmore A, Hussin YA. 2017. Theoretical framework for spatial planning and forest management in Indonesia: securing the basic rights for adat people. InaJForRes. 4(1):69–83. doi: 10.20886/ijfr.2017.4.1.69-83.
- Oktavia D, Pratiwi SD, Kamaludin NN, Dede M, Widiawaty MA. 2023. Dynamics of land use and land cover in the Belitung Island, Indonesia. Sumedang: Universitas Padjadjaran.
- Olson MG, Knapp BO, Kabrick JM. 2017. Dynamics of a temperate deciduous forest under landscape-scale management: implications for adaptability to climate change. For Ecol Manage. 387:73–85. doi: 10.1016/j.foreco.2016.07.033.
- Pal M, Foody GM. 2010. Feature selection for classification of hyperspectral data by SVM. IEEE Trans Geosci Remote Sens. 48(5):2297–2307. doi: 10.1109/TGRS.2009.2039484.
- Park HA. 2013. An introduction to logistic regression: from basic concepts to interpretation with particular attention to nursing domain. J Korean Acad Nurs. 43(2):154–164. doi: 10.4040/jkan.2013.43.2.154.
- Parveen N, Siddiqui L, Siddiqui MA, Sarif MN, Islam MS, Khan S, Khanam N, Mohibul S, Shariq M. 2022. Monitoring built-up area expansion led by industrial transformation in Delhi using geospatial techniques. Environ Sci Pollut Res Int. 30(49):106936–106950. doi: 10.1007/s11356-022-23221-3.
- Patil VH, Franken FH. 2021. Visualization of statistically significant correlation coefficients from a correlation matrix: a call for a change in practice. J Market Anal. 9(4):286–297. doi: 10.1057/s41270-021-00120-z.
- Patnala A, Stadtler S, Schultz MG, Gall J. 2023. Multi-modal self-supervised learning for boosting crop classification using Sentinel2 and Planetscope. IGARSS 2023 - 2023 IEEE International Geoscience and Remote Sensing Symposium, 2223–2226. doi: 10.1109/IGARSS52108.2023.10282665.
- Pebesma E, Bivand R. 2023. Spatial data science: with applications in R. Boca Raton: CRC Press. doi: 10.1201/9780429459016.
- Pitaloka EF, Karuniasa M, Moersidik SS. 2020. Time series of forest land cover change in the Upper Citarum Watershed, West Java Province, Indonesia. E3S Web Conf. 211:04001. doi: 10.1051/e3sconf/202021104001.
- Prasad P, Loveson VJ, Chandra P, Kotha M. 2022. Evaluation and comparison of the earth observing sensors in land cover/land use studies using machine learning algorithms. Ecol Inf. 68:101522. doi: 10.1016/j.ecoinf.2021.101522.
- Prashanth M, Kumar A, Dhar S, Verma O, Rai SK, Kouser B. 2023. Land use/land cover change and its implication on soil erosion in an ecologically sensitive Himachal Himalayan watershed, Northern India. Front for Glob Change. 6:1124677. doi: 10.3389/ffgc.2023.1124677.
- Rachmadita R, Widiana A, Rahmat A, Sunardi S, Dede M. 2023. Utilizing satellite imagery for seasonal trophic analysis in the freshwater reservoir. J Multidiscip Appl Nat Sci. 4(1):63–75. doi: 10.47352/jmans.2774-3047.188.
- Ridwansyah I, Yulianti M, Apip, Onodera S, Shimizu Y, Wibowo H, Fakhrudin M. (2020). The impact of land use and climate change on surface runoff and groundwater in Cimanuk watershed, Indonesia. Limnology. 21(3), 487–498. doi: 10.1007/s10201-020-00629-9.
- Ripaldi D. 2023. Eksploitasi air tanah berlebih berbuah krisis air di Bandung. Tirto. https://tirto.id/gDk8.
- Rodríguez-Rodríguez D, Martínez-Vega J, Echavarría P. 2019. A twenty year GIS-based assessment of environmental sustainability of land use changes in and around protected areas of a fast developing country: Spain. Int J Appl Earth Obs Geoinf. 74:169–179. doi: 10.1016/j.jag.2018.08.006.
- Roy PS, Ramachandran RM, Paul O, Thakur PK, Ravan S, Behera MD, Sarangi C, Kanawade VP. 2022. Anthropogenic land use and land cover changes—a review on its environmental consequences and climate change. J Indian Soc Remote Sens. 50(8):1615–1640. doi: 10.1007/s12524-022-01569-w.
- Rukmana D. 2015. The change and transformation of Indonesian spatial planning after Suharto’s new order regime: the case of the Jakarta metropolitan area. Inte Plann Stud. 20(4):350–370. doi: 10.1080/13563475.2015.1008723.
- Sano EE, Ferreira LG, Asner GP, Steinke ET. 2007. Spatial and temporal probabilities of obtaining cloud-free Landsat images over the Brazilian tropical savanna. Int J Remote Sens. 28(12):2739–2752. doi: 10.1080/01431160600981517.
- Santos F, Graw V, Bonilla S. 2019. A geographically weighted random forest approach for evaluate forest change drivers in the Northern Ecuadorian Amazon. PLoS One. 14(12):e0226224. doi: 10.1371/journal.pone.0226224.
- Setiadi S, Sumaryana A, Bekti H, Sukarno D. 2023a. The flood management policy in Bandung city: challenges and potential strategies. Cogent Soc Sci. 9(2):2282434. doi: 10.1080/23311886.2023.2282434.
- Setiadi S, Sumaryana A, Bekti H, Sukarno D. 2023b. Public policy communication for flood management. J Soc Media. 7(2):300–312. doi: 10.26740/jsm.v7n2.p300-312.
- Setyorini A, Khare D, Pingale SM. 2017. Simulating the impact of land use/land cover change and climate variability on watershed hydrology in the Upper Brantas basin, Indonesia. Appl Geomat. 9(3):191–204. doi: 10.1007/s12518-017-0193-z.
- Sil Â, Fonseca F, Gonçalves J, Honrado J, Marta-Pedroso C, Alonso J, Ramos M, Azevedo JC. 2017. Analysing carbon sequestration and storage dynamics in a changing mountain landscape in Portugal: insights for management and planning. Int J Biodiver Sci Ecosyst Serv Manage. 13(2):82–104. doi: 10.1080/21513732.2017.1297331.
- Smith TJ, McKenna CM. 2013. A comparison of logistic regression pseudo R2 indices. General Linear Model J. 39(2):17–26.
- Sodikin S, Sitorus S, Prasetyo L, Kusmana C. 2017. Spatial analysis of mangrove deforestation and mangrove rehabilitation directive in Indramayu Regency, West Java, Indonesia. AACL Bioflux. 10:1654–1662.
- Solekha NA, Qudratullah MF. 2022. Pemodelan geographically weighted logistic regression dengan fungsi adaptive gaussian kernel terhadap kemiskinan di Provinsi NTT. Jambura J Math. 4(1):17–32. doi: 10.34312/jjom.v4i1.11452.
- Sugito NT, Gumilar I, Hernandi A, Handayani AP, Dede M. 2023. Utilizing Semi-variograms and geostatistical approach for land value model in urban region. IJE. 36(12):2222–2231. doi: 10.5829/IJE.2023.36.12C.12.
- Sunardi S, Ariyani M, Withaningsih S, Darma AP, Wikarta K, Parikesit P, Kamarudin MKA, Abdoellah OS. 2021. Peri-urbanization and sustainability of a groundwater resource. Environ Dev Sustain. 23(6):8394–8404. doi: 10.1007/s10668-020-00972-y.
- Sunardi S, Nursamsi I, Dede M, Paramitha A, Arief MCW, Ariyani M, Santoso P. 2022. Assessing the influence of land-use changes on water quality using remote sensing and GIS: a study in Cirata Reservoir, Indonesia. SciTechnolIndonesia. 7(1):106–114. doi: 10.26554/sti.2022.7.1.106-114.
- Susanti Y, Syafrudin S, Helmi M. 2019. Soil erosion modelling at watershed level in Indonesia: a review. E3S Web Conf. 125:01008. doi: 10.1051/e3sconf/201912501008.
- Susiati H, Dede M, Widiawaty MA, Ismail A, Udiyani PM. 2022. Site suitability-based spatial-weighted multicriteria analysis for nuclear power plants in Indonesia. Heliyon. 8(3):e09088. doi: 10.1016/j.heliyon.2022.e09088.
- Susiati H, Widiawaty MA, Dede M, Akbar AA, Udiyani PM. 2022. Modeling of shoreline changes in West Kalimantan using remote sensing and historical maps. Int J Conserv Sci. 13(3):1043–1056. 10.5281/zenodo.7109747.
- Tariq A, Mumtaz F. 2023. A series of spatio-temporal analyses and predicting modeling of land use and land cover changes using an integrated Markov chain and cellular automata models. Environ Sci Pollut Res Int. 30(16):47470–47484. doi: 10.1007/s11356-023-25722-1.
- Toma MB, Belete MD, Ulsido MD. 2023. Historical and future dynamics of land use land cover and its drivers in Ajora‐Woybo watershed, Omo‐Gibe basin, Ethiopia. Nat Resour Model. 36(1):e12353. doi: 10.1111/nrm.12353.
- Tong X, Luo X, Liu S, Xie H, Chao W, Liu S, Liu S, Makhinov AN, Makhinova AF, Jiang Y. 2018. An approach for flood monitoring by the combined use of Landsat 8 optical imagery and COSMO-SkyMed radar imagery. ISPRS J Photogramm Remote Sens. 136:144–153. doi: 10.1016/j.isprsjprs.2017.11.006.
- Truax B, Gagnon D, Lambert F, Fortier J. 2015. Multiple-use zoning model for private forest owners in agricultural landscapes: a case study. Forests. 6(12):3614–3664. doi: 10.3390/f6103614.
- Tsangaratos P, Ilia I. 2016. Comparison of a logistic regression and Naïve Bayes classifier in landslide susceptibility assessments: the influence of models complexity and training dataset size. CATENA. 145:164–179. doi: 10.1016/j.catena.2016.06.004.
- Verma P, Raghubanshi AS. 2019. Rural development and land use land cover change in a rapidly developing agrarian South Asian landscape. Remote Sens Appl: Soc Environ. 14:138–147. doi: 10.1016/j.rsase.2019.03.002.
- Vu K, Hartley K, Kankanhalli A. 2020. Predictors of cloud computing adoption: a cross-country study. Telem Inform. 52:101426. doi: 10.1016/j.tele.2020.101426.
- Werner BT, McNamara DE. 2007. Dynamics of coupled human-landscape systems. Geomorphology. 91(3-4):393–407. doi: 10.1016/j.geomorph.2007.04.020.
- Widarjono A. 2015. Analisis multivariat terapan: dengan program SPSS, AMOS, dan SMARTPLS. Yogyakarta: STIM-YKPN. https://www.semanticscholar.org/paper/Analisis-multivariat-terapan-%3A-dengan-program-SPSS%2C-Widarjono/816f3d522cfce528a2b2892db98d3e4bfc702c3c.
- Widiawaty MA. 2019. Mari mengenal sains informasi geografis. Bandung: Aria Mandiri Group. doi: 10.5281/zenodo.3263711.
- Widiawaty MA, Dede M. 2018. Pemodelan spasial bahaya dan kerentanan bencana banjir di wilayah timur Kabupaten Cirebon. Jurnal Dialog Penanggulangan Bencana. 9(2):142–153.
- Widiawaty MA, Dede M, Ismail A. 2019. Analisis tipologi urban sprawl di Kota Bandung menggunakan sistem informasi geografis. SNG. 3:547. doi: 10.24895/SNG.2018.3-0.1007.
- Widiawaty MA, Ismail A, Dede M, Nurhanifah N. 2020. Modeling land use and land cover dynamic using geographic information system and Markov-CA. Geos Ind. 5(2):210. doi: 10.19184/geosi.v5i2.17596.
- Widiawaty MA, Lam KC, Dede M, Asnawi NH. 2022. Spatial differentiation and determinants of COVID-19 in Indonesia. BMC Public Health. 22(1):1030. doi: 10.1186/s12889-022-13316-4.
- Widiawaty MA, Nandi N, Murtianto H. 2020. Physical and social factors of shoreline change in Gebang, Cirebon Regency 1915-2019. JAGI. 4(1):327–334. doi: 10.30871/jagi.v4i1.2020.
- Wijaya N, Bisri MBF, Aritenang AF, Mariany A. 2017. Spatial planning, disaster risk reduction, and climate change adaptation integration in Indonesia: progress, challenges, and approach. In: Djalante R, Garschagen M, Thomalla F, Shaw R, editors. Disaster risk reduction in Indonesia: progress, challenges, and issues. Cham: Springer International Publishing; p. 235–252. doi: 10.1007/978-3-319-54466-3_9.
- Zhu Z, Woodcock CE. 2014. Continuous change detection and classification of land cover using all available Landsat data. Remote Sens Environ. 144:152–171. doi: 10.1016/j.rse.2014.01.011.