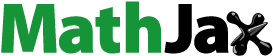
Abstract
To systematically and thoroughly analyze the sensitivity and reliability of the MSBAS regularization for the estimation of surface deformation over a mine in combination with an application example, this study processed 101 Sentinel-1A/B SAR images, constructed and solved the 2D deformation models using SVD and Tikhonov regularization methods with different orders and parameters, and estimated the vertical and east-west surface deformation time series in a mine of China. Then, this study collected the leveling-monitoring vertical surface deformation data on three leveling points, and compared and analyzed the sensitivity and reliability of the MSBAS regularization methods for estimating vertical surface deformation. The results indicate that different regularization orders and parameters can lead to thousands of times differences in condition numbers and significant differences in ill-posed degree of the deformation models. The zero-order Tikhonov regularized deformation model with regularization parameter of 0.1 has the minimum condition number and the equation is not ill-posed. The first-order Tikhonov regularized deformation model with regularization parameter of 0.001 has the maximum condition number and the equation is seriously ill-posed. As a result, the estimates of 2D surface deformation models with different parameters and orders are also different in terms of numerical values and accuracy. Compared with the leveling-monitoring data, the first- and second-order MSBAS regularization methods with parameter 0.1 have the minimum fluctuation and the maximum correlation coefficients between the estimated values and the leveling-monitoring values, and are also closest to the leveling-monitoring results with the highest accuracy.
1. Introduction
Mineral resources are a major strategic resource for the national socio-economic development. However, the continuous mining of underground coal resources can easily cause the rocks above the goaf to be deformed by gravity, which will lead to the destruction of buildings, residential houses, farmland and transportation facilities in mining areas, affecting the production and life of human beings and even threatening the safety of people’s lives and property. Therefore, it is necessary to take effective technical means to monitor the surface deformation in the mining areas (Grzovic and Ghulam Citation2015; Ding et al. Citation2019; Wang et al. Citation2020).
With the development of space-to-earth observation technology, DInSAR (Differential Interferometric Synthetic Aperture Radar) technology (Gabriel et al. Citation1989; Chen et al. Citation2019) and SBAS InSAR (Small Baseline Subset Interferometric Synthetic Aperture Radar) technology (Berardino et al. Citation2002) have gradually become the important technical means for monitoring surface deformation in mines (Jiang Citation2020; Liu et al. Citation2022). However, SBAS InSAR based on the single-track SAR images can only obtain the 1D (One-dimensional) deformation information on the LOS (line-of-sight) of SAR images in the study area. The emergence of the MSBAS InSAR (Multi-dimensional Small Baseline Subset InSAR) technology breaks the limitation, so that the 2D deformation in the vertical and east-west directions of the study area can be obtained, which improves the observation dimension (Samsonov and D'Oreye Citation2012; Du and Zhao Citation2020). In recent years, many researchers have applied MSBAS InSAR for surface deformation monitoring, such as for monitoring 2D (two-dimensional) surface deformation due to coal mining activities in the Greater Luxembourg region and southern Saskatchewan (Samsonov et al. Citation2013; Samsonov et al. Citation2014a), 2D surface deformation due to volcanic eruptions in Italy (Samsonov et al. Citation2014b), 2D deformation monitoring of landslides in the upper Jinsha River (Xiong et al. Citation2021), 2D urban deformation monitoring in Mexico and Shanghai (Samsonov and D'Oreye Citation2017; Dong et al. Citation2018), and 2D deformation monitoring of subsurface fluid injection in the Karamay oil field in Xinjiang (Yang et al. Citation2019).
Researchers generally believe that the deformation model of MSBAS InSAR suffers from an ill-posed problem, and small errors in differential interferometric phase inevitably lead to serious deviations between the estimated and the true values (Liu Citation2018; Xiong et al. Citation2021; Kong Citation2023). The regularized least squares method with Tikhonov regularization (Tikhonov and Arsenin Citation1977) or the SVD (Singular Value Decomposition) method (Ren and Feng Citation2020) are commonly used to estimate the vertical and east-west surface deformation information of the study area (Samsonov et al. Citation2013; Samsonov et al. Citation2014a; Derauw et al. Citation2020. Yu et al. Citation2023). In 2017, Samsonov and D'Oreye proposed three Tikhonov regularization models for MSBAS InSAR, namely zero-, first-, and second-order regularization models. However, in specific experimental applications, only the first-order regularization model with a parameter of 0.03 was used for estimation (Samsonov and D'Oreye Citation2017). The sensitivity and reliability of the MSBAS regularization methods with different orders and parameters for estimation of surface deformation over a mine have not been studied in detail.
Based on these, this study processed a large amount of ascending and descending Sentinel-1A/B SAR images covering a mine, constructed and solved the Tikhonov regularized 2D deformation models of MSBAS InSAR with three different orders and three different parameters, and analyzed the sensitivity and reliability of the MSBAS regularization methods for estimating vertical and east-west surface deformation time series over the mine by comparing with leveling-monitoring results. The specific work was as follows: (1) The basic principle of MSBAS InSAR, the construction and the causes of ill-posed problem of its 2D deformation model were briefly described and analyzed in theory, and the main process of data processing was put forward. (2) 51 ascending Sentinel-1A SAR images and 50 descending Sentinel-1B SAR images were jointly processed using MSBAS InSAR, and 263 unwrapped ascending and descending differential interferograms were obtained. (3) The zero-, first- and second-order Tikhonov regularized 2D deformation models of MSBAS InSAR were constructed with three different regularization parameters (0.1, 0.01 and 0.001), then the ranks, singular values (or eigenvalues) and condition numbers of the coefficient matrices of their observation equations and normal equations were calculated, and the ill-posed problem of the 2D deformation model of MSBAS InSAR were studied and analyzed in practical application. (4) Based on SVD method and Tikhonov regularization methods with different orders and parameters, the 2D deformation models of MSBAS InSAR were solved and the vertical and east-west surface deformation time series of the study area were estimated, compared and analyzed. The temporal curves of the vertical surface deformation obtained by SVD and 9 Tikhonov regularization methods were plotted and compared with the leveling-monitoring data by calculating their correlation coefficients and RMSE (Root Mean Square Error).
The novelty of this study mainly lied in systematically and thoroughly analyzing the ill-posed problem, the sensitivity and reliability of MSBAS regularization in combination with the practical application in a mine area. This paper was organized as follows: Section 2 focused on the background information and SAR images of the study area. Section 3 illustrated the composition of the 2D deformation model of MSBAS InSAR, the ill-posed problem and the main process of data processing. Section 4 gave the experimental results and analysis. Section 5 discussed the research content of this study. Section 6 drew some conclusions.
2. Study area and data
2.1. Study area
The study area is a mine area that is located in the southern part of the North China Plain and the northern bank of the Yellow River. Its administrative division is under the jurisdiction of Jinan City, Shandong Province. The average east-west strike length of the entire mine area is 9.9 km, the average north-south tilt width is 5 km, and the total area is approximately 49 km2. The main mineral in the mine area is coal, and the surface deformation continues to occur during the mining process. As it is densely populated and has multiple villages, the monitoring of surface deformation in the region will provide robust data support for disaster prevention and safe production of coal mines (Pan et al. Citation2020).
2.2. Data
This study collected 101 C-band Sentinel-1A/B SAR images in the IW (Interferometric wide swath) and VV (Vertical linear transmission and vertical linear reception) mode. The 51 ascending SAR images are from October 6, 2018 to August 20, 2020, and the 50 descending SAR images are from October 12, 2018 to August 14, 2020. In addition, this study used 30-m ground resolution Shuttle Radar Topography Mission (SRTM) Digital Elevation Model (DEM) provided by National Aeronautics and Space Administration (NASA) to simulate and remove the topographic phase contribution. shows the location of study area and the coverage of ascending and descending SAR images.
According to the time span of ascending and descending SAR images, this study collected the leveling-monitoring vertical surface deformation on three leveling points Q43, Q49 and Q97 by ourselves, and compared, analyzed, and verified the sensitivity and reliability of the vertical surface deformation estimated by the MSBAS regularization methods with different orders and parameters. the leveling-monitoring results included 7 periods of cumulative subsidence values from October 12, 2018 to June 27, 2019, which were measured using the American Trimble DiNi03 electronic level and leveling rod according to the fourth-order leveling surveying with the allowable closing error of mm (
is the length of leveling line in km). The locations of the three leveling points are shown in . Q43 is located at the edge of the subsidence basin, Q49 is located between the center and the edge of the subsidence basin, and Q97 is located at the center of the subsidence basin. shows the monitoring data of three characteristic leveling points.
Table 1. Leveling-monitoring dates and results of three characteristic leveling points.
3. Methodology
3.1. MSBAS InSAR
The MSBAS InSAR (Samsonov and D'Oreye Citation2012) is a multi-dimensional deformation time series analysis technique. It is mainly used to calculate vertical and east-west deformation time series from spatiotemporal overlapping ascending and descending SAR images.
Assuming that the MSBAS InSAR differential interferogram sequence contains three ascending interferometric combinations and three descending interferometric combinations, the corresponding SAR image acquisition times are and
respectively, as shown in .
The observation equation established in time order is as follows (Samsonov and D'Oreye Citation2017):
(1)
(1)
Where
is the azimuth angle,
is the central incident angle.
is the acquisition time interval of the adjacent SAR images. For example, in this experiment,
the azimuth angles of the first, second and third ascending SAR images are
and
and the central incidence angles are
and
the azimuth angles of the first, second and third descending SAR images are
and
and the central incidence angles are
and
and
are the vertical and east–west average deformation phase change velocities, respectively, between adjacent SAR image acquisition times.
is the interferometric phase difference after unwrapping. Assuming that there are m ascending and descending differential interferograms and n adjacent SAR image acquisition intervals, the observation equation is as follows:
(2)
(2)
Assuming that there are m ascending and descending differential interferograms and n adjacent SAR image acquisition intervals, V = (
) is the 2n-dimensional parameter vector to be solved, Φ is the m-dimensional known observation vector composed by ascending and descending unwrapped differential interferometric phases, and A is the known coefficient matrix with m rows and 2n columns.
V can be obtained by solving the observation EquationEquation (2)(2)
(2) , and then the vertical and east-west deformation phase time series of the surface can be obtained by integrating V in temporal domain.
Analyzing the coefficient matrix of the observation EquationEquations (1)(1)
(1) and Equation(2)
(2)
(2) , it is easy to find the following relationships between columns 1 and 2, columns 3 and 4, columns 5 and 6, ……, and columns 2n − 1 and 2n:
(3)
(3)
Therefore, there is a linear correlation between the two, rank(A) = (n + 1) < 2n, rank() = (n + 1) < 2n. The coefficient matrix A in the observation Equationequation (2)
(2)
(2) is a column rank-deficient matrix, the coefficient matrix
of its normal equation is a rank-deficient matrix whose Cayley–Hamilton inverse matrix does not exist, and the unique optimal estimation of V in the observation EquationEquation (2)
(2)
(2) belongs to an ill-posed problem in physics and mathematics. The SVD method and Tikhonov regularization method are two commonly used methods to solve the ill-posed problem (Zhai Citation2020).
3.2. Tikhonov regularization methods
In 1963, Tikhonov, a former Soviet scientist, first proposed a regularization method for solving the ill-posed problem. The Tikhonov regularization method transforms the original ill-posed problem into a well-posed problem by adding the condition of minimum weighted squares sum of all or part of parameters (Tikhonov and Arsenin Citation1977; Chu et al. Citation2011; Mehrabi et al. Citation2019). According to the Tikhonov regularization method, the of solving the observation EquationEquation (2)
(2)
(2) can be transformed into the following form:
(4)
(4)
where λ is the regularization parameter, L is the difference operator, and
is the regularization matrix. When L is equal to the unit matrix
that is
it is the zero-order Tikhonov regularization method; When
it is the first-order Tikhonov regularization method; When
it is the second-order Tikhonov regularization method.
The observation EquationEquation (2)(2)
(2) after Tikhonov regularization is:
(5)
(5)
According to the optimal estimation criterion (4), it is obtained that:
(6)
(6)
3.3. Data processing
In this study, GAMMA software was used to preprocess the 51 ascending SAR images and 50 descending SAR images in , which is a full-featured software platform for interferometric radar data processing developed by the Swiss GAMMA company. Specifically, the SAR images were first de-skewed and cropped according to the geographical scope of the study area. Then, according to the principle of highest coherence of differential interferograms, the SAR image on February 3, 2019 was determined to be the master image of the ascending SBAS InSAR data processing, the SAR image on August 8, 2019 was determined to be the master image of the descending SBAS InSAR data processing, and the other 50 ascending SAR images and 49 descending SAR images were used as the slave images to make co-registration with their respective master image (Hooper Citation2006). Next, differential interference, various kinds of error phase filter removal and phase unwrapping were performed on the co-registrated SAR images, and the 132 ascending and 131 descending differential interferograms of MSBAS InSAR were selected based on the interference coherence and phase unwrapping quality. Finally, the obtained ascending and descending unwrapping differential interferograms were resampled to the same reference geographic coordinate system to complete co-registrating them (Han et al. Citation2022).
The preprocessing and differential interferometric processing of MSBAS InSAR are similar to those of single-track SBAS InSAR. It requires multiple SLC (Single Look Complex) SAR images and external DEM to carry out the basic data processing steps such as co-registration, flat-earth phase removal, differential interference, filtering enhancement, high coherence pixel selection, phase unwrapping, and geocoding. Among them, co-registration is mainly due to the deviation of the orbits and look angles of SLC SAR imaging, and there is a certain displacement and distortion between the SAR images. Flat-earth phase removal and differential interference are used to remove the influence of flat-earth and topography phase on the estimated 2D deformation results. Filtering enhancement is used to remove various kinds of error phase. The meaning and reason of executing other steps can be obtained by referring to relevant literature (Liu et al. Citation2019).
After preprocessing and differential interferometric processing, the coefficient matrix A in the observation EquationEquation (2)(2)
(2) was calculated and the deformation model was established based on the time series of differential interferometric processing, imaging parameters (incidence and azimuth angle) of SAR images and the ascending and descending unwrapping differential interferograms, and the ill-posed problem of the 2D deformation model of MSBAS InSAR were studied and analyzed based on A and
Then the 2D deformation models were established and solved using the SVD and zero-, first- and second-order Tikhonov regularization methods with three different regularization parameters to inverse the annual average deformation rate, the cumulative deformation in the vertical and east-west directions from October 12, 2018 to August 14, 2020. The 2D surface deformation information inversed in the previous step was compared with the results of leveling monitoring for the accuracy analysis and verification with calculating their correlation coefficients and RMSE. shows the data processing flow.
In fact, there are many error sources in MSBAS InSAR, mainly including SAR systems, electromagnetic wave coherence, radar system propagation paths, SAR images, and data processing. The error of SAR systems mainly refers to the thermal noise in the radar systems, the error of electromagnetic wave coherence mainly refers to the error caused by spatiotemporal baseline incoherence, the error of radar system propagation paths mainly refers to atmospheric delay error and orbital error, the error of SAR images mainly refers to the layover, shadowing, and foreshortening of SAR images, and the error of data processing mainly refer to co-registration error, unwrapping error, residual DEM error, and so on (Liu et al. Citation2019).
4. Results
4.1. 2D Deformation model and its ill-posed problem
Based on the data processing flow shown in , the ascending and descending SAR images were preprocessed and differential interfered, and 132 ascending and 131 descending temporal differential interferograms were obtained. According to the observation EquationEquation (1)(1)
(1) of MSBAS InSAR, the coefficient matrice A in the observation EquationEquation (2)
(2)
(2) ,
rank and singular values of A and eigenvalues of
were calculated, respectively. shows the composition of the ascending and descending temporal differential interferograms. shows the basic characteristics of A and
shows the rows, columns, ranks and condition numbers of zero-, first- and second-order Tikhonov regularization coefficient matrix with three different regularization parameters.
Figure 4. Composition of the ascending and descending differential interferograms for this experiment.

Table 2. Basic characteristics of A and
Table 3. Basic characteristics of zero-, first- and second-order tikhonov regularization coefficient matrix with three different regularization parameters.
In , the “Row and column” refers to the number of rows and columns of “Rank” refers to the rank of
or
"Condition number" refers to the condition number of
and its calculation formula is
where k is the condition number of the matrix,
and
is the maximum and minimum eigenvalue of the matrix, respectively. k can be used to represent the ill-posed degree of
the larger k is, the more serious the ill-posed degree of the equation is, and the more unstable the solution is. In general, when k is greater than zero and less than 100, the matrix is not ill-posed, when k ranges from 100 to 1000, the matrix is moderately ill-posed, when k is greater than 1000, the matrix is seriously ill-posed (Liu et al. Citation2012).
The following observations were made from and and .
When retained to five decimal places, the non-zero elements of each column in the matrix A have equal values and are very close to zero, mainly because
(
) is the same or a multiple relationship, and the azimuth and central incidence angles of the ascending and descending SAR images are roughly the same. In addition, the elements in
are also very close to zero.
The coefficient matrix A and ATA are both in serious rank deficit. Tikhonov regularization can transform the rank-defect coefficient matrix ATA into a full-rank matrix
and solve this problem of rank deficit. However, different regularization orders and parameters will lead to different condition numbers and the ill-posed degree of the deformation model. In comparison, the zero-order Tikhonov regularized deformation model with regularization parameter of 0.1 has the minimum condition number and the equation is not ill-posed. The first-order Tikhonov regularized deformation model with regularization parameter of 0.001 has the maximum condition number, and the equation is seriously ill-posed.
4.2. Results of SVD and Tikhonov regularization
The deformation models (2) and (5) were solved using the SVD method and Tikhonov regularization methods with different orders and parameters, respectively, and the vertical and east-west surface deformation time series in the mine area were estimated. and show the annual average vertical and east-west deformation rates from October 12, 2018 to August 14, 2020. shows the vertical cumulative surface deformation at five imaging moments. shows the maximum values of vertical and east-west deformation rates and vertical final cumulative surface deformation obtained by the two methods. and show the spatial variation curves of the vertical and east-west deformation annual average rate obtained by the two methods on section lines 1 and 2 ((a)) from October 12, 2018 to August 14, 2020. shows the spatial variation curves of vertical final cumulative surface deformation on section lines 1 and 2 on August 14, 2020.
Figure 5. Annual average vertical deformation rate estimated by the SVD and Tikhonov regularization methods with different orders and parameters from October 12, 2018 to August 14, 2020.

Figure 6. Annual average east-west deformation rate estimated by the SVD and Tikhonov regularization methods with different orders and parameters from October 12, 2018 to August 14, 2020.

Figure 7. Vertical cumulative deformation at five imaging moments in the mine area estimated by the SVD and Tikhonov regularization methods with different orders and parameters.

Figure 8. Spatial variation curves of the annual average vertical deformation rate on section lines 1 and 2 estimated by the two methods from October 12, 2018 to August 14, 2020.

Figure 9. Spatial variation curves of the annual average east-west deformation rate on section lines 1 and 2 estimated by the two methods from October 12, 2018 to August 14, 2020.

Figure 10. Spatial variation curves of final vertical cumulative deformation on section lines 1 and 2 estimated by the two methods on August 14, 2020.

Table 4. Maximum values of surface deformation estimated by the SVD and Tikhonov regularization methods with different orders and parameters from October 12, 2018 to August 14, 2020.
Analysis of and revealed that:
As shown in and , the vertical and east-west surface deformation in the mine area estimated by the SVD and Tikhonov regularization methods continued to become stronger with time. The location and spatio-temporal distribution of the vertical and east-west surface deformation were consistent with each other, only slightly different in the values of deformation(Except for the zero-order Tikhonov regularization method with parameter 0.1). The 2D surface deformation estimated by the zero-order Tikhonov regularization method with parameter 0.1 was significantly smaller than that of other methods, and the results were the most different from those of other methods.
As shown in , the spatial variation trend of vertical land subsidence in the mine area estimated by the SVD and Tikhonov regularization methods were consistent with each other, only slightly different in the values of subsidence in the peaks and tails of the curves (Except for the zero-order Tikhonov regularization method with parameter 0.1). The spatial variation trend of subsidence estimated by the zero-order Tikhonov regularization method with parameter 0.1 was consistent with that of other methods, but the numerical values were much smaller.
As shown in , and , the zero-order Tikhonov regularized deformation model with regularization parameter of 0.1 has the minimum condition number and the equation is not ill-posed, but the 2D surface deformation estimated by it was significantly different from that of other methods and the numerical values were much smaller. The zero-, first and second-order Tikhonov regularized deformation model with regularization parameter of 0.001 has the maximum condition number and the equation is seriously ill-posed. but the 2D surface deformation estimated by it was consistent with that of other methods (Except for the zero-order Tikhonov regularization method with parameter 0.1).
4.3. Sensitivity analysis and reliability verification
In this study, the sensitivity and reliability of the vertical surface deformation estimated by the SVD and Tikhonov regularization methods were compared and verified with the leveling-monitoring results on three leveling points using correlation coefficient and RMSE as the evaluation indicators. The three leveling points were Q43, Q49 and Q97 ( and ), and their specific longitude and latitude coordinates were (117.028°, 36.819°), (117.029°, 36.820°) and (117.030°, 36.821), respectively. It should be noted that the collected leveling data only included vertical surface deformation values, so only the sensitivity and reliability of the vertical surface deformation estimated by MSBAS regularization were verified.
The correlation coefficient was calculated by the following equation:
(7)
(7)
where X is the vector of vertical surface deformation results inversed by the SVD and Tikhonov regularization methods, Y is the vector of leveling-monitoring results,
is the covariance between X and Y,
is the variance of X, and
is the variance of Y (Mukaka Citation2012).
The RMSE was calculated by the following equation:
(8)
(8)
where
is the vertical surface deformation result at the ith imaging moment inversed by the SVD and Tikhonov regularization methods, and
is the result of linear interpolation of the leveling- monitoring vertical surface deformation results at the ith imaging moment.
Based on the leveling-monitoring data on Q43, Q49 and Q97, this study compared and verified the sensitivity and reliability of the vertical land subsidence by the SVD and Tikhonov regularization methods. It should be noted that the time span of the collected leveling-monitoring data was from October 12, 2018 to June 27, 2019, so only the estimated results of the SVD and Tikhonov regularization methods during the corresponding time span were selected for the sensitivity and reliability verification. In addition, the monitoring dates of the two were not completely consistent (Leveling had 7 monitoring dates and ascending and descending SAR images had 42 monitoring dates from October 12, 2018 to June 27, 2019), the leveling-monitoring results were interpolated into the monitoring dates of ascending and descending SAR images by piecewise linear interpolation and solved the above problem.
show the comparison of vertical surface deformation time series on Q43, Q49 and Q97 from October 12, 2018 to June 27, 2019. statistics the correlation coefficient between InSAR and leveling data and RMSE of the vertical surface deformation on Q43, Q49 and Q97, respectively.
Figure 11. Vertical surface deformation time series of leveling, SVD, tikhonov regularization with different orders and parameters on Q43.

Figure 12. Vertical surface deformation time series of leveling, SVD, tikhonov regularization with different orders and parameters on Q49.

Figure 13. Vertical surface deformation time series of leveling, SVD, tikhonov regularization with different orders and parameters on Q97.

Table 5. Correlation coefficient and RMSE of the vertical surface deformation on Q43.
Table 6. Correlation coefficient and RMSE of the vertical surface deformation on Q49.
Table 7. Correlation coefficient and RMSE of the vertical surface deformation on Q97.
Analysis of and revealed that:
On the whole, with the increase of the deformation magnitude on Q43, Q49 and Q97, the RMSE of the vertical surface deformation results in the mine area estimated by the SVD and Tikhonov regularization methods gradually increased, the difference between them was growing and the reliability was reduced.
On Q43, Q49 and Q97, the time series of vertical surface deformation estimated by the zero-order Tikhonov regularization method with parameter 0.1 differed most significantly from the leveling results, and the estimated deformation values were generally smaller. The RMSE reached 26.886, 65.139 and 79.288 mm respectively, with the worst reliability. In contrast, the first- and second-order Tikhonov regularization methods with parameter 0.1 didn’t encounter this problem, and the solved vertical surface deformation curves had the minimum fluctuation and the maximum correlation coefficients, which indicated that the trends were most consistent with the leveling-monitoring trends.
Relatively speaking, during the period from October 12, 2018 to June 27, 2019, the deformation degree on Q43, Q49 and Q97 was not too serious, the time series of vertical surface deformation of the SVD and Tikhonov regularization methods (Except for the zero-order Tikhonov regularization method with parameter 0.1) were very consistent with each other, and also close to the leveling-monitoring results.
5. Discussion
Compared with the previous literature mentioned in the introduction, which only believed that the deformation model of MSBAS InSAR suffered from the ill-posed problem and should be solved by the Tikhonov regularization method. This study, based on ascending and descending Sentinel-1A/B SAR images acquired between October 2018 and August 2020, computed a 2D surface deformation model using MSBAS, systematically studied and analyzed the cause and specificity of the ill-posed problem and the sensitivity and reliability of the MSBAS regularization methods for solving 2D surface deformation time series in the mine area.
After comparative analysis and verification, our experimental results showed that the composition of the coefficient matrix A of the 2D deformation model determined that its columns were linearly correlated to each other. There were many identical elements with values close to zero. The non-zero singular values were between 0.0189 and 0.1720; At the same time, the elements in the coefficient matrix of its normal equation were very small, its non-zero eigenvalues were between 0.0004 and 0.0296, and its rank was far less than the number of parameters to be solved. Although the estimated 2D surface deformation location, distribution, annual average deformation rate and other deformation information in the mine area were more reliable and accurate compared with the leveling-monitoring results. The impact of the above factors on the monitoring results of the two-dimensional surface deformation of MSBAS InSAR needed to be further verified by more leveling-monitoring data, theoretical and applied research. In addition, we only used the zero-, first- and second-order Tikhonov regularization methods with three parameters to solve the MSBAS InSAR deformation model. Among them, the zero-order Tikhonov regularization method with parameter 0.1 had the smallest condition number and the equation was not ill-posed, but the solution results were the worst; When the parameter was 0.001, the condition number was very large and the ill-posed degree of the equation was serious, but it did not have a significant impact on the estimated deformation results. All of these needed further discussion and research.
6. Conclusion
In this study, the ill-posed problem, sensitivity, and reliability of MSBAS regularization for estimating surface deformation in mining areas were analyzed, and compared and verified with SVD and leveling-monitoring data. The following conclusions were drawn:
In the coefficient matrix of the MSBAS InSAR 2D deformation model, There are many identical elements with values close to zero and its non-zero singular values are small. Similarly, all of the elements in the coefficient matrix of the normal equation and its non-zero eigenvalues are very small, which rank is far less than the number of parameters to be solved. The ill-posed problem of the MSBAS InSAR deformation model is mainly a serious rank defect problem caused by linearly correlated columns in the coefficient matrix.
The 2D surface deformation in the mine area solved by the Tikhonov regularization methods (Except for the zero-order Tikhonov regularization method with parameter 0.1) are very similar, and the results show that the vertical and east-west surface deformation in the mine area are gradually increasing with time, and the deformation location, spatio-temporal distribution, annual average maximum deformation rate and so on are very consistent with each other, only slightly different in the deformation values. The 2D surface deformation values estimated by the zero-order Tikhonov regularization method with parameter 0.1 are significantly smaller than those of other methods, and the results are the most different from those of other methods.
The vertical surface deformation time series estimated by the Tikhonov regularization methods (Except for the zero-order Tikhonov regularization method with parameter 0.1) are very consistent with each other, and also close to the leveling-monitoring results. However, with the increase of the deformation magnitude, the reliability of the vertical surface deformation results in the mine area estimated by the Tikhonov regularization methods gradually decrease. In contrast, the vertical surface deformation results estimated by the first- and second-order Tikhonov regularization methods with parameter 0.1 are most consistent with the leveling-monitoring results. The vertical surface deformation time series estimated by the zero-order Tikhonov regularization method with parameter 0.1 differ most significantly from the leveling results, and the estimated deformation values are obviously smaller.
Different regularization orders and parameters will lead to significantly different condition number and ill-posed degree of the deformation model, but the differences between the estimated 2D surface deformation values is not significant (Except for the zero-order Tikhonov regularization method with parameter 0.1). In comparison, the zero-order regularization is more sensitive to parameter settings, when the parameter is 0.1, the estimated 2D surface deformation of MSBAS regularization is significantly different from the estimated values of the other two parameters, and the reliability of the solution is poor. The difference between 2D surface deformations estimated by the first- and second-order MSBAS regularization with three different parameters is small, and the first- and second-order MSBAS regularizations are not very sensitive to parameter settings in this study.
This study will help to better understand the composition of the MSBAS InSAR deformation model, solve its ill-posed problem, and ultimately improve the accuracy and application capability of InSAR technique for monitoring the mining surface deformation.
Acknowledgments
The authors wish to thank the European Space Agency (ESA) for supplying the free Sentinel-1A/B data and NASA for providing the SRTM3 DEM data.
Disclosure statement
No potential conflict of interest was reported by the authors.
Data availability statement
The remote sensing and DEM datasets supporting the results of this study are publicly available through ASF Data Search (https://search.asf.alaska.edu) as well as Earth Explorer (https://earthexplorer.usgs.gov). Reference data are available from the corresponding author upon reasonable request.
Additional information
Funding
References
- Berardino P, Fornaro G, Lanari R, Sansosti E. 2002. A new algorithm for surface deformation monitoring based on small baseline differential SAR interferograms. IEEE Trans Geosci Remote Sensing. 40(11):2375–2383. doi:10.1109/TGRS.2002.803792.
- Chu D, Lin L, Tan R, Wei Y. 2011. Condition numbers and perturbation analysis for the Tikhonov regularization of discrete ill-posed problems. Numer Linear Algebra Appl. 18(1):87–103. doi:10.1002/nla.702.
- Chen Y, Tao Q, Liu T, Liu G, Wang K, Ding L. 2019. Analysis of land subsidence monitoring capability of double-track DInSAR mining area. Safety in Coal Mines. 50(12):212–218. doi:10.13347/j.cnki.mkaq.2019.12.047.
- Dong S, Samsonov S, Yin H, Huang H. 2018. Two-dimensional ground deformation monitoring in shanghai based on SBAS and MSBAS InSAR methods. J Earth Sci. 29(4):960–968. doi:10.1007/s12583-017-0955-x.
- Ding L, Tao Q, Gao T, LX, Sun C. 2019. Application of SBAS InSAR in monitoring mining land subsidence. China Science Paper. 14(3):320–325.
- Du J, YL, Zhao C.2020. Multidimensional deformation monitoring using InSAR technology in Yuxian mining area. Coal Geol Explor. 48(1):172–177.
- Derauw D, Nicolas D, Jaspard M, Caselli A, Samsonov S. 2020. Ongoing automated ground deformation monitoring of Domuyo - Laguna del Maule area (Argentina) using sentinel-1 MSBAS time series: methodology description and first observations for the period 2015–2020. J South Am Earth Sci. 104:102850. doi:10.1016/j.jsames.2020.102850.
- Gabriel A, Goldstein R, Zebker H. 1989. Mapping small elevation changes over large areas differential radar interferometry. J Geophys Res. 94(B7):9183–9191. doi:10.1029/JB094iB07p09183.
- Grzovic M, Ghulam A. 2015. Evaluation of land subsidence from underground coal mining using Time SAR (SBAS and PSI) in Springfield, Illinois, USA. Nat Hazards. 79(3):1739–1751. doi:10.1007/s11069-015-1927-z.
- Hooper A. 2006. Persistent scatter radar interferometry for crustal deformation studies and modeling of volcanic deformation [dissertation]. California: Stanford University.
- Han Y, Tao Q, Liu G, Hou A, Guo Z, Wang T. 2022. Accuracy verification and correction of ascending and descending SBAS- and MSBAS-InSAR in monitoring mining surface subsidence. Geocarto Int. 37(27):16370–16397. doi:10.1080/10106049.2022.2108909.
- Jiang Z. 2020. Research on the solution method of the ill-posed problem for the short baseline set InSAR deformation model. J Surv Map. 49(03):399.
- Kong X. 2023. Research on the Method of Extracting Highly Accurate Three-Dimensional Deformation by Multi-Orbit InSAR [dissertation]. Inner: Inner Mongolia University of Technology.
- Liu G, Zhao C, Zhang S, Wang Z, Tao Q. 2012. Theories and methods of modern measurement adjustment. Xuzhou: China University of Mining and Technology Press.
- Liu Y. 2018. [Research on the monitoring and inversion of different-scale complex surface deformation with multi-temporal InSAR ]. [[master’s thesis]. ]. Chang’an: University Xi’an.
- Liu G, Chen Q, LX, Cai G. 2019. InSAR principles and applications. Beijing: Science Press.
- Liu X, Tao Q, Niu C, Li B, Zhang Y, Ren Y. 2022. Comparative analysis and verification of land subsidence monitoring ability between DInSAR and SBAS InSAR mining area. PG. 37:1825–1833.
- Mukaka M. 2012. A guide to appropriate use of Correlation coefficient in medical research. Malawi Med J. 24(3):69–71.
- Mehrabi H, Voosoghi B, Motagh M, Hanssen RF. 2019. Three-dimensional displacement fields from insar through Tikhonov regularization and least-squares variance component estimation. J Surv Eng. 145(4):04019011–04019011. 04019011.15. doi:10.1061/(ASCE)SU.1943-5428.0000289.
- Pan G, Tao Q, Chen Y, Wang K. 2020. Settlement monitoring and analysis of Jiyang mining area in Shandong Province based on SBAS InSAR. Chin J Geol Hazard Control. 31:8.
- Ren H, Feng X. 2020. Calculating vertical deformation using a single InSAR pair based on singular value decomposition in mining areas. Int J Appl Earth Obs Geoinf. 92:102115. doi:10.1016/j.jag.2020.102115.
- Samsonov S, D'Oreye N. 2012. Multidimensional time‐series analysis of ground deformation from multiple InSAR data sets applied to Virunga Volcanic Province. Geophys J Int. 191(3):1095–1108.
- Samsonov S, D'Oreye N, Smets B. 2013. Ground deformation associated with post-mining activity at the French-German border revealed by novel InSAR time series method. Int J Appl Earth Obs. 23:142–154. doi:10.1016/j.jag.2012.12.008.
- Samsonov SV, González PJ, Tiampo KF, D'Oreye N. 2014a. Modeling of fast ground subsidence observed in southern Saskatchewan (Canada) during 2008-2011. Nat Hazards Earth Syst Sci. 14(2):247–257. doi:10.5194/nhess-14-247-2014.
- Samsonov SV, Tiampo KF, Camacho AG, Fernández J, González PJ. 2014b. Spatiotemporal analysis and interpretation of 1993-2013 ground deformation at Campi Flegrei, Italy, observed by advanced DInSAR. GRL. 41(17):6101–6108. doi:10.1002/2014GL060595.
- Samsonov SV, D'Oreye N. 2017. Multidimensional small baseline subset (MSBAS) for Two-dimensional deformation analysis: case study Mexico City. Can J Remote Sens. 43(4):318–329. doi:10.1080/07038992.2017.1344926.
- Tikhonov A, Arsenin V. 1977. Solutions of ill-posed problems. SIAM. 21(2):266–267.
- Wang X, Wei K, Chen J, Wang X, Xue H, Li K. 2020. Status and development trend of surface subsidence monitoring and prediction technology in coal seam mining. Sci Technol Eng. 20(24):9966–9706.
- Xiong G, Yang C, Zhu S, DJ, Zhang Q. 2021. Deformation analysis of Sala landslide in the upper reaches of Jinsha River based on MSBAS technology. Chin J Geol Disaster Prevent Control. 32(5):1–9.
- Yang C, Zhang D, Zhao C, Han B, Sun R, Du J, Chen L. 2019. Ground deformation revealed by sentinel-1 MSBAS-InSAR time-series over Karamay oilfield, China. RS. 11(17):2027. doi:10.3390/rs11172027.
- Yu B, Hu Y, Liu G. 2023. Time-series InSAR inversion of two-dimensional surface deformation time series in Tangshan City. Surv Map Sci. 48(01):82–94.
- Zhai M. 2020. Solution method of ill conditioned model and its application in InSAR deformation inversion of short baseline set [dissertation]. Shandong: Shandong University of Science and Technology.