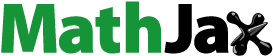
Abstract
Landuse alteration is a critical factor that exerts influence over hydrological mechanisms and the oversight of water resources across the globe. Northern Ghana is characterized by erratic rainfall, leading to the intense construction of small dams and dugouts. This study investigated historical landuse/cover (LULC) changes and physical factors associated with surface runoff generation within eight (8) sub-catchments namely; Guno, Gbahali, Sambu, Duago, Denugo, Kwisini, Sandu, and Nyeko, with areas of 29.5, 110, 38.5, 106.04, 67.81, 2.59, 10.56 and 120.65 km2, respectively, located in four (4) regions of northern Ghana. For LULC change detection, historical satellite imagery and geospatial data were used with the aid of remote sensing and applying supervised maximum likelihood classification to Landsat images of 1995, 2005, 2015, and 2023. Runoff factors and depths were identified based on LULC classes, hydrologic soil groups, and precipitation conditions prevailing in the areas, based on the curve number (CN) method. With regards to LULC, the results from the overall analysis gave an insight into the rampant conversion, where grassland/mix vegetation witnessed significant declines, with decreases ranging from 150 to 395.25% in different catchments. Agricultural and arable lands emerged as the dominant landuse category, indicating a remarkable expansion of 11.61–51.17 km2, from 1995 to 2023 respectively, followed by built-up areas. While grassland decreased in all catchments over the same period, in some places, open tree (savannah woodland) increased, which confirmed the implementation of the afforestation policy. The CN values offered essential insights into the hydrological characteristics of each catchment. In addition to the impact of LULC and watershed characteristics on runoff, the observed variations in runoff patterns can be attributed to interannual variability in total annual precipitation which is critical driver of runoff. The findings hold significant importance for developing effective strategies and integrated watershed management policies to contribute to better water resource management and inform sustainable landuse planning in the face of changing environmental conditions.
1. Introduction
Climate change stands as the primary obstacle to agriculture, the security of food supply for billions of people, and rural livelihoods around the world (Hussain et al. Citation2022). Due to the growth of society, the hydrological cycle and water resource management have been significantly impacted by a wide range of human activities, exacerbated by climate change. According to the study by Gbohoui et al. (Citation2021), on understanding the hydrological behaviour of watersheds in the West Africa Sahel (WAS), the findings underscore the impact of climate and environmental shifts on surface runoff within the Sahelian context. Climate and LULC have a great influence on hydrological processes (Yonaba et al. Citation2023), and awareness of their occurrence and respective impacts is of great importance concerning land use planning (Obahoundje and Diedhiou Citation2022). However, assessing variability and attribution of changes in surface runoff was conducted by Yonaba et al. (Citation2021) in the Sahelian watershed, where isolated contributions of climate variability and LULC changes on surface runoff showed that LULC conditions play a dominant role in runoff increase over climate. The phenomenon of land use/land cover (LULC) change is one of the significant indicators of such impacts (Umukiza et al. Citation2021). Alterations in LULC are a significant topic of global discourse due to being recognized as a primary contributor to worldwide environmental transformations (Toure et al. Citation2020). LULC changes have been identified as the main driving force of global change (Nyatuame et al. Citation2023) and have essential impacts on hydrological processes, the economy, and the ecology of watersheds (Marie Mireille et al. Citation2019). LULC change influences the watershed hydrology (Manzoor et al. Citation2022) and, eventually, the sustainability of water availability. Significant changes between physical and hydraulic soil properties under agricultural areas and natural vegetation cover were observed, supporting the hypothesis that anthropogenic activities such as agricultural activity and construction among others may influence the water balance (Umukiza et al. Citation2021). Therefore, the dynamics of LULC change and its impacts on hydrological processes have become crucial research areas in the face of rapid societal changes leading to urbanization and agricultural expansion.
While comprehensive quantitative generalizations about the effects of forest cover loss are limited due to insufficient data, many studies provided, clearly indicate that LULC changes do influence hydrological fluxes, particularly discharge and surface runoff, even though the outcomes vary considerably (Guo et al. Citation2011; Apollonio et al. Citation2016; Shaina Beegam and Prince Arulraj Citation2018; Dionizio and Costa Citation2019; Hu et al. Citation2020). Beyond their economic value, forests serve as vital carbon sinks, influencing climate change rates, aiding in soil formation, and regulating water resources. The study conducted by Barasa and Perera (Citation2018) in the Sosiani River basin in Kenya, examined the correlation between LULC changes and river peak discharge. Their findings revealed a direct association between an increase in farmlands and urban areas and a corresponding rise in peak discharge, while a reduction in forest areas was also linked to the same increase in peak discharge. This is in agreement with the study by Yonaba et al. (Citation2021) which concluded that the conversion of natural vegetation into cultivated areas led to a significant increase in the runoff potential under a dynamic LULC input in picturing the Sahelian paradox.
LULC changes have a profound impact on the hydrological characteristics within a catchment, influencing the generation of runoff and the occurrence of soil erosion resulting from runoff events (Sertel et al. Citation2018; Marie Mireille et al. Citation2019; Mounirou et al. Citation2022). The conversion of forests and grasslands into croplands and built-up areas has the potential to alter the hydrological regime of the catchments in the region (Umukiza et al. Citation2022). Furthermore, land use changes can affect runoff dynamics by modifying surface infiltration, evapotranspiration rates, and the overall hydrological connectivity of the landscape (Praskievicz and Chang Citation2011; Grimaldi et al. Citation2012). These alterations, in return, impact the timing, magnitude, and frequency of runoff events, influencing water availability and quality in both upstream and downstream areas.
From the perspective of effective and sustainable management of water resources in water shortage areas like arid and semi-arid, it is very crucial to comprehensively monitor the characteristics of LULC dynamics and their influence on runoff generation. In recent years, the integration of advanced technologies, particularly deep learning and artificial intelligence (AI) models in remote sensing (RS) has played a pivotal role (Zhu et al. Citation2024). Utilizing RS is crucial for obtaining extensive and high-quality Earth observation (EO) data efficiently. However, the conventional expert system-centric approach faces challenges in meeting the demand of the RS big data era. Therefore, advanced AI, such as deep learning, have demonstrated success in various RS and geoscience applications (Du et al. Citation2023; Hong et al. Citation2023; Zhu et al. Citation2024). With a specific focus on state-of-the-art (SOTA) deep learning and AI models applied in remote sensing (Du et al. Citation2023), it is crucial for extracting meaningful insights from vast and complex datasets, facilitating accurate assessments of LULC changes, and understanding their implications on hydrological processes.
The northern regions of Ghana are characterized by a semi-arid climate (Antwi-Agyei et al. Citation2015; Yomo et al. Citation2020), making this part vulnerable to fluctuations in precipitation and water availability. Characterizing the LULC dynamics and physical factors influencing surface runoff is essential for accurately developing and managing the construction of the small dams in the area. Furthermore, in the context where environmental conditions and landuse patterns are rapidly involved, understanding the relationship between landuse change and runoff generation is of paramount importance for sustainable water resource management and effective land use planning (Bahremand Citation2006; Bhatti et al. Citation2019; Yang et al. Citation2019).
As land se change has been recognized as a significant driver of hydrological processes, affecting local water availability and quality, landuse change has been occurring at an accelerated pace in many regions worldwide (Ohana-Levi et al. Citation2018), with northern Ghana being no exception. According to Wróbel and Boczoń (Citation2020), the soil conservation service curve number (SCS–CN) technique was employed to assess the potential capabilities of the individual sub-catchments, and this approach has stood as a widely utilized means for estimating effective rainfall runoff over the years. When landuse changes occur, such as urbanization, deforestation, or agricultural expansion, they can directly alter the land surface characteristics that influence the curve number (CN) (Mishra and Singh Citation2006; Gajbhiye et al. Citation2014; Ohana-Levi et al. Citation2018; Umukiza et al. Citation2021) and consequently, hydrological processes. On the other hand, beyond the general term of land use, it is also surface hydraulic properties which turn affect runoff generation (Mounirou et al. Citation2021; Yang et al. Citation2021). Moreover, diffuse erosion remains dominant since surface runoff is laminar and sediment transport capacity is limited (Mounirou et al. Citation2022). Therefore, it is crucial to understand the dynamics of land use change and its impact on rainfall-runoff generation to support effective water resource management and ecosystem conservation. For instance, CN which is a critical factor in estimating runoff and predicting the effects of precipitation on watersheds is widely used in hydrology to assess the potential for runoff (USDA-NRCS Citation2010; Ara and Zakwan Citation2018). Assessing the patterns of land use change over time and identifying the key physical factors: Topographic parameters, hydrologic soil group and Curve Number (CN) values are essential (Mustafa et al. Citation2012). The relationship between LULC and hydrograph main characteristics was investigated considering the CN approach (Zeng et al. Citation2017; Ohana-Levi et al. Citation2018).
However, the utilization of SCS-CN methods comes with acknowledged limitations (Kang and Yoo Citation2020) that are crucial to consider in the long-term estimation of runoff. One significant drawback is the method’s inability to adequately capture temporal variability, especially in cases of significant LULC changes within a watershed, or during intense and prolonged drought conditions. This limitation can impact the accuracy of runoff predictions. The underestimation if runoff due to convers due CN composition sis most severe for wide CN ranges,
On the positive side, the CN method offers flexibility by allowing the use of available data, such as past or future historical LULC and soil data, which can be readily accessible. This characteristic simplifies the data requirements compared to continuous hydrological models like the Soil Water Assessment Tool (SWAT), Hydrologic Engineering Center’s Hydrologic Modeling System (HEC-HMS), and Variable Infiltration Capacity (VIC) models. These continuous models typically demand observed data for calibration and validation, which are often missed in small catchments.
Given that this study was conducted in ungauged catchments where detailed hydrological data for calibration and validation were not available (Gajbhiye et al. Citation2014; Kowalik and Walega Citation2015; Piscopia et al. Citation2015), the SCS-CN method emerged as a preferable and pragmatic choice since it has been the foundation of the hydrology algorithms in most simulation models developed by United states department of Agriculture (USDA) for hydrology and water resource (Garen and Moore Citation2005; Wróbel and Boczoń Citation2020). The decision was primarily driven by the accessibility of the data and the specific characteristics of the study areas.
Despite the growing recognition of the impact of hydrological factors on runoff generation, limited research has been conducted in the specific context of northern Ghana where small dams and reservoirs were intensively constructed to harvest surface runoff. Therefore, this study aims to address this knowledge gap by characterizing the dynamics of land use change and investigating physical drivers that involve hydrological processes in the study of individual catchments within the region. By gaining a comprehensive understanding of the interplay between land use change and runoff dynamics of the study areas in northern Ghana, this study aims to contribute to informed decision-making processes and foster the sustainable utilization of water resources in the region. The findings may also have broader implications for similar regions facing a similar problem.
2. Materials and methods
2.1. General overview of the sandy area
The research was conducted in four (4) northern regions of Ghana ( and ).
Table 1. Description of the study dam catchments.
Ghana is geographically positioned between latitudes 4°30′ N and 11° N and longitudes 1° E and 3° 30′ W, and shares eastern, western, and northern borders with Togo, Côte d’Ivoire, and Burkina Faso, respectively. The northern region is one of the driest regions in the country due to its proximity to the Sahara desert and the Sahel region (Ampim et al. Citation2021). The principal feature of rainfall in Ghana is its seasonal character and its variability from year to year. The rainfall pattern is uni-model, covering the period from June to September with mean annual rainfall ranging between 800 and 110 mm, followed by long dry spell from November to March and dry and dusty harmattan winds (Yomo et al. Citation2020; Bekoe et al. Citation2021). The climate is tropical, characterized by high temperatures with an average annual temperature ranging from 25 to 35 °C (Barnes Citation2009). Vegetation is predominantly savannah woodlands dominated by drought-resistant trees and grasses (Bekoe et al. Citation2021), with scattered trees and shrubs (Ampim et al. Citation2021; Manzoor et al. Citation2022). The region is also known for its agricultural activities with farming being a primary occupation and main economic activity. The soil is predominantly formed ingenious rocks quite shallow (Bekoe et al. Citation2021).
Physiographically, the northern regions are marked by the presence of significant watersheds, including the Black Volta, White Volta, and Oti River, collectively contributing to the extensive Volta River system. The terrain is a mix of savannah and semi- arid landscapes, featuring flat to gently rolling topography. Despite the agricultural focus, the semi-arid conditions and sporadic, intense rainfall pose challenges for soil erosion.
2.2. Data used for LULC classification
Surface reflectance images (level 2) Landsat 5 and 8 at a resolution of 30 m were used for LULC classification. Four (4) Landsat images from 1995, 2005, 2025, and 2023 were obtained from State Geological Survey (USGS) official website (https://earthexplorer.usgs.gov/). The downloaded Landsat images had already some standard preprocessing (e.g. geometric, radiometric correction) done by USGS which can be considered for the type of analysis performed (Guzha et al. Citation2018; Ohana-Levi et al. Citation2018). An interval of approximately 10 years was selected as it struck a balance between effectively monitoring land change dynamics and keeping the data and analysis workload manageable. To avoid confusion that may arise in distinguishing some vegetation-based land use classes (e.g. crops vs grassland), the downloaded images were taken during the peak dry season period in the region (January–March). The post-harvest period was found to be optimal for single-date LULC mapping in terms of LULC dynamics in the Sahelian landscape under semi-arid climate as found in a study conducted in northern Burkina Faso by Yonaba et al. (Citation2021) ).
Table 2. Details of acquired satellite images.
2.3. LULC data preparation and classification
As the respective catchment areas are small parts of the entire north of Ghana, after processing the mosaic, layer stack, the subset was done, and the study area maps were imported in Erdas Imagine software 2015 for LULC classification. Classification is supervised type of machine learning (Jawale Citation2019). For the process of supervised classification, the most employed algorithm is the Maximum Likelihood (ML) algorithm (Anua and Wong Citation2022). Supervised classification has demonstrated superior accuracy compared to the unsupervised classification technique (Mohd Hasmadi et al. Citation2009). In this paper, supervised classification was adopted and performed using an ML algorithm. The decision to employ the supervised classification method was influenced by factors such as the relatively modest dimensions of the study area and the authors’ comprehensive understanding of the catchments. Moreover, ML has proven successful in applications such as crop mapping and more broadly in LULC mapping, including specific applications in the Sahelian context (Gardelle et al. Citation2010; Yonaba et al. Citation2021). The ML algorithm ensures efficient classification of satellite imagery and allows for accurate delineation of land use classes within the study area. This parametric classifier relies on the assumption that the statistical distributions for each class and across each spectral band follow a normal distribution. The calculation process involves estimating the parameters associated with these normal distributions and making decisions based on the resultant statistical model ().
Table 3. Land use landcover (LULC) types in the dam catchments.
2.4. Accuracy assessment
Accuracy assessment is useful to assess the quality of the data collected in the field and classified images (Owar Othow et al. Citation2017). Confirmation of results obtained from LULC classification was a pivotal stride, marked by accuracy assessment. Prior to the utilization of a map, it is imperative to subject it to an evaluation process, gauging its precision and ensuring that it aligns adequately with the requisites of its intended application (Marie Mireille et al. Citation2019). In an investigation led by the Food and Agriculture Organization of the United Nations (FAO Citation2016) concerning map accuracy assessment and area estimation, a trio of critical metrics emerged. These metrics encompass the overall accuracy, denoting the proportion of correctly categorized areas reflecting the likelihood that an arbitrarily chosen point on the map is accurately categorized (EquationEquation (1)(1)
(1) ). The user’s accuracy, a second measure, signifies the proportion of an area classified as class i that also corresponds to class i in the reference dataset (EquationEquation 2
(2)
(2) ). This metric extends the probability that a designated region of class i on the map corresponds to the same class in reality. Finally, the producer’s accuracy, the third metric, signifies the proportion of area characterized as reference class j, which also aligns with class j on the map (Equation identified as the same class on the map (FAO Citation2016).
(1)
(1)
(2)
(2)
(3)
(3)
Where: A is the overall accuracy; Ui is the user’s accuracy; pj is the producer’s accuracy; pii is the number of classes i classified on the map and also on the ground; pjj is the number of class j on the ground and also classified as the same class on the map; pi is the classified total of class i on the map and pj is the total number of class j on the ground.
Accuracy assessment was very important to determine how accurate the referenced data agreed with classified images of the remotely sensed data (Owar Othow et al. Citation2017; Guzha et al. Citation2018). Therefore, the accuracy performance was important (Mohamed et al. Citation2020) to detect if the LULC classification was useful the accuracy measures (Overall accuracy, User accuracy, Producer accuracy) were determined by using an error matrix.
2.5. Digital elevation model (DEM) and hydrologic soil group (HSG)
The DEM for each of the respective catchments was gathered from Google Explorer 2023 of the United States Geological Survey’s Earth Explorer (USGS) site (http://earthexplorer.usgs.gov/) (accessed on 22 February 2023) with 30 meters resolution. The DEM was processed and clipped for each catchment for delineation of the whole watersheds and subbasins in ArcMap 10.7.
The hydrologic soil groups (HSG) data, as they are fundamental components for estimation of rainfall-runoff based on the United States Department of Agriculture(USDA) curve number(CN) method, were gathered from the NASA website global hydrologic soil group (https://daac.ornl.gov/SOILS/guides/Global_Hydrologic_Soil_Group.html).
The catchment areas were identified by delineating the boundaries of watersheds (drainage areas). The data Characteristics are global with spatial coverage. These data are modelled to represent contemporary soil runoff potential (Ross et al. Citation2018). The data information was one data file of global HSGs at 250 m resolution in GeoTIFF format. Ghana Hydrologic soil map was therefore extracted by mask with the help of Arc GIS as done in Hengl et al. (Citation2017). Thereafter, the hydrological soil group of the research zone was produced from the Ghana soil map with the help of GIS and the hydrological soil group classification look-up table (Mishra and Singh Citation2006).
After subtracting each catchment area in terms of hydrologic soil group, the proportions in percentage () indicate the distribution rate of each catchment area. Group C was found to be representative of each catchment.
Table 4. Hydrological soil groups in the catchments.
2.6. Rainfall data
Rainfall data for each of the eight (8) catchments under study was collected from the Modern-Era Retrospective Analysis for Research and Applications, Version 2 (MERRA-2) (Gelaro et al. Citation2017) product of NASA POWER. It incorporates observational data from various sources to create a comprehensive and accurate representation of the Earth’s climate over a specific period. The reanalysis was performed to determine maximum yearly data from 1990 to 2021. Since the catchments of our study are ungauged and no meteorological weather is available, we used MERRA-2 data acquired using coordinates of each catchment to perform precipitation and temperature analysis. MERRA-2 was reanalysed in the study conducted by Rodrigues and Braga (Citation2021), to estimate daily weather variables in hot summer Mediterranean climate, the study found good agreement between MERRA-2 data and observed data for all parameters except wind speed. Also, comparison between daily values from MERRA-2 and meteorological stations using several statistical tools are in acceptable agreement (Marzouk Citation2021). The study conducted by Ngurah et al. (Citation2022), on the island of Bali using observed rainfall from several locations and MERRA-2 rainfall data found high correlation values. In places rainfall data are not available as it is a case of our catchments; rainfall from MERRA-2 can be utilised as a credible source. MERRA-2 is reliable source for global meteorological data (precipitation maximum and minimum temperature, humidity and solar radiation) when compared with observed data (Bandira et al. Citation2022).
2.7. Determination of surface runoff
The effects of LULC changes on surface runoff are well known when considering the conversion of forests or grassland to cropland or built up (Hundecha and Bárdossy Citation2004; Descroix et al. Citation2009; Mustafa et al. Citation2012). Although, the impact of LULC on watershedhydrology are known the spatial distribution of LULC characteristics can affect the runoff (Pechlivanidis et al. Citation2011; Kalantari et al. Citation2014). In this study direct runoff was determined using Curve Number (CN) methods. CN is dimensionless parameter indicating the runoff response characteristics of the drainage basin (Stewart et al. Citation2012). As the intention of the process was to be applicable in ungauged watersheds without available data, the model parameter (CN) was linked to soil and vegetation characteristics and can be approximated using looked-up tables (Garen and Moore Citation2005; Sitterson et al. Citation2018). The CN values range theoretically between 0 and 100 (Stewart et al. Citation2012; Umukiza et al. Citation2021). However, CN ranges typically between 30 and 100 according to different land use and soil conditions, where lower CN values indicate better water infiltration and higher CN values signify reduced infiltration and higher runoff potential. The CN parameter is related to land use treatment, hydrological condition, hydrologic soil group and antecedent soil moisture condition (AMC) at the moment of precipitation in the drainage basin. The determination of CN in this context involved the integration of spatial LULC data, soil type information, and an assumption of AMC (Antecedent Moisture Condition) categorized as II (indicating moderate wetness of the soil prior to precipitation). The detailed process encompassed the initial assignment of CN values to distinct LULC types based on authoritative lookup tables. Average CN values have been determined on each catchment-wide scale for combined land areas and types. The average CN value and runoff depth for the entire catchment were respectively computed using the following EquationEquations (4)(4)
(4) ; (5) and (6) (Sitterson et al. Citation2018; Ara and Zakwan Citation2018; Wróbel and Boczoń Citation2020).
(4)
(4)
Where CNi and Ai are the CN value (−) and area value (km2), respectively, of the generic LULC, CN (−) is the weighted Curve Number considering the specific areas as weights and A (km2) is the total area of the investigated case study.
(5)
(5)
(6)
(6)
Where: Q is the runoff depth (mm), S is the potential maximum abstraction (mm), CN is the Curve Number, P is the rainfall depth (mm).
3. Results
3.1. Analysis of landuse landcover (LULC) dynamics in the dam catchments
The accuracy of the LULC classification for categorized images from 1995, 2005, 2015, and 2023 was systematically evaluated for each class. The assessment results for all classified LULC maps revealed an overall classification accuracy exceeding 80%. Producer accuracy (PA) and User accuracy (UA) were calculated and presented in .
Table 5. Accuracy assessment of classified images.
The producer accuracy (PA) and user accuracy (UA) data for LULC change across different sub-catchments and years (1995, 2005, 2015, and 2023) provide valuable insights into the performance of the classification system. In general, for the category of built up, there was a consistent increase in both PA and UA over the years across all sub-catchments. The Grasslands category exhibits variations, but overall, the accuracy is relatively high (+80%). Agricultural Lands consistently show high accuracy, with both PA and UA maintaining an upward trend. Waterbody accuracy fluctuates but remains generally high. Remarkably, some sub-catchments achieve perfect accuracy (100%) for certain classes, such as built-up areas in Sambu and built-up areas in Duago. The detailed data underscores the robustness of the land use and land cover classification system, emphasizing its effectiveness in accurately capturing changes over the years and across diverse sub-catchments (Yonaba et al. Citation2021).
The analysis of LULC dynamics from various catchments over the year 1995, 2005, 2015 and 2023 highlighted diverse trends in LULC across different catchments as presented in .
The analysis of distribution and composition of different LULC types highlighted each catchment into different LULC classes, and corresponding areas in square kilometers (km2) and are given for each year as presented in over changes in the period of 1995–2023.
Table 6. Landuse and landcover classes and dynamics in various catchments.
Based on the data in the aforementioned table, the trends and implications of each class are noticeable for each catchment. In the overall analysis, agricultural lands have experienced consistent increase. For instance, in Guno Catchment, agricultural activities expanded from 9 km2 in 1995 to 25.64 km2 in 2023 suggesting an intensification of cultivation. Moreover, agricultural lands have experienced a noticeable increase from 11.61 km2 in 1995 to 51.17 km2 in 2023, signifying remarkable agricultural expansion in the Duago catchment.
However, the built-up land remains relatively less changing over the years except in Ghahali catchment while built-up areas have increased substantially, nearly quadrupling from 21 km2 in 1995 to 80 km2 in 2023, indicative of urbanization. In the same catchment, grasslands have undergone considerable decline, reducing from 79.8 km2 in 1995 to 19 km2 in 2023. Water bodies had a minimal presence despite that the catchments have small dams, this was encountered because the images were analyzed for peak dry season, and many small dams dried out after a certain period of rainfall shortage (Andoh et al. Citation2018). Agricultural expansion, grassland reduction, urbanization and shifts in land management practices are prominent drivers of the observed changes.
The analysis was performed as presented in to further understand the changes from interval stages from 1995 to 2005, 2005 to 2015 and 2015 to 2023 of various activities in the study areas.
The overall LULC analysis of each catchment from the period of 1995–2023 is summarized in presented as follows:
Table 7. Summary of landuse cover dynamics (1995–2023) in percentage.
The studied sub-catchments: Guno, Gbahali, Sambu, Duago, Denugo, Kwisini, Sandu and Nyeko experienced landuse and landccover changes over the studied period (1995–2023). Across all catchments, the predominant LULC was agricultural/arable lands with a significant increase of 385.56% and a lower rate of 50.0% for Denugu and Guno while grasslands decreased by 166.4% and 308.5% for the same catchments respectively. Indeed in 1995, the covered areas were predominantly grassland (Open savanna, pasture lands), over time, intensification of agricultural activities converted grasslands into farmlands.
The observed LULC changes in the eight catchments of the study area from 1995 to 2023 showed a significant rise in agricultural lands while grasslands were consistently diminishing. This can be attributed to the change in lifestyle to meet the growth of food demand and introduction of agricultural machinery. However, the increase of 95.8% in open trees in the Sandu catchment, could eventually be attributed to the awareness and changes in mentality to plant trees to reverse the effects of climate changes. Moreover, a remarkable exception in an increase of built-up Gbahali is noticeable. This can be justified by the settlement of the population due to its proximity to the Tamale metropolis where several businesses and opportunities can attract the population to run businesses in the area and tackle construction.
3.2. Digital elevation model of the study catchments
The study analyzed different catchments where Digital Elevation Maps (DEMs) obtained from the Shuttle Radar Topography Mission (SRTM) satellite data, ensuring a spatial resolution of 30 m were developed for each of the respective catchments represented by a specific location. As they play a crucial role in the context of water management, essential information about the topography and elevation of the study areas are presented in and .
The range of elevations within each catchment area is quite substantial, varying from one area to another. This variation has implications for the distribution of rainfall runoff.
In terms of elevation, the highest area was the Gbahali catchment the elevation ranged from 268 to 370 meters.
The average elevation found that the range of mean from the lowest to the highest was 135 meters and 319 meters for the Sandu and Duago catchments, respectively ().
Table 8. Summary of the topographic maps.
The maximum and minimum elevations for each catchment areas were recorded, providing insights into topographical diversity of the areas under study. Understanding these variations in elevation and their impacts on rainfall and runoff is crucial for various purposes, including water resources management and land use planning. This suggests that different catchment areas might experience varying levels of overall hydrological behavior. The maximum and minimum elevations for each catchment area were recorded Understanding these variations in elevation and their impacts on rainfall and runoff is crucial for various purposes, including water resources management and land use planning. This suggests that different catchment areas might experience varying levels of overall hydrological behavior.
3.3. Curve number (CN) characterization and runoff from the study areas
A significant purpose of CN investigation involved examining the effects of LULC on the factors influencing rainfall-runoff patterns. Therefore, we have collected and analyzed crucial data pertaining to values across various catchments from the year 1995 to 2023. It is worth noting that runoff in the given watershed is dependent on LULC (Kowalik and Walega Citation2015) so a similar approach is followed here.
The variation rate (in percentage) of CN was further analyzed in comparison to the 1995 LULC as presented in .
Table 9. Variation of CN values from the investigated areas with respect to 1995 conditions.
The difference in CN values (%) between 1995 and 2023 indicates how much the potential runoff generation has changed over time. By comparing the CN values of 1995 and 2923, we discerned the alternations in each catchment’s ability to manage rainfall runoff over this period. For instance, Guno and Denugo catchments, were characterized by significant increases in CN values of 6.6 and 8.1% with respect to the 1995 condition respectively. The increase of Similar trends is observed in other catchments as well. In addition, rainfall is the fundamental driving force in rainfall-runoff generation. The distribution of runoff follows the same trend of precipitation as the correlation trend is remarkable (). With the annual maximum rainfall of 1550.73 mm and minimum of 926.15 mm, the highest maximum, minimum and average annual runoff depth, is ranging from 1477.04, 854.07 and 1197, respectively for Denugo catchment, which represents 95.24% of the total annual rainfall. Based on the annual maximum rainfall of the respective catchments the potential runoff depth is more than 94% of the annual rainfall ().
The historical rainfall data helped to analyze how different factors interplay and influence runoff generation in the study areas.
Quantification of the potential amount of runoff provides basic information to plan rainfall runoff harvesting and infrastructure storage capacity.
The increase in CN values suggests that potential runoff generation has increased in the catchments. This could be attributed to various factors such as land use, urbanization due to the growth of population, intensification of mechanization agriculture leading to soil compaction and alterations in natural vegetation. Analyzing these CN values across the different catchments allowed us to confirm how LULC changes have impacted their hydrological behavior. The observed percentage differences in CN values between 1995 and 2023 provide a quantifiable measure of alternations in each catchment’s runoff generation potential. These findings contribute to our understanding of how LULC changes over time influence the hydrological characteristics of various catchments in northern regions of Ghana, which can have significant implications on water resource management and sustainable development in the region. For instance, the upward trend indicates a rise in the catchment’s capacity to influence the increase in runoff generation (Habete and Ferreira Citation2016). The simplicity of the CN method and its relatively low data input requirements circumvent the issues associated with parameterizing and executing more intricate models. This approach has been incorporated into numerous models focused on hydrology, erosion, and water quality, effectively sidestepping complexities (Ross et al. Citation2018). Furthermore, the Study by Ara and Zakwan (Citation2018) determined CN based on LULC concluded that accurate CN as numeric catchment characteristics which are the basis of catchment runoff determination define the runoff potential.
4. Discussion
The study highlights the complexities of assessing the dynamics of LULC change and rainfall runoff generation patterns in different catchments using the CN method. The obtained results are in agreement with recent literature findings which revealed that LULC pattern has drastically changed in which the built-up area increases year after year as long as human beings continue to exist, urbanization continues (Dionizio and Costa Citation2019; Guzha et al. Citation2018; Shaina Beegam and Prince Arulraj Citation2018). The pronounced surge in agricultural/arable lands was attributed to the influence of socio-economic factors, population growth and adoption of mechanization agriculture driving substantial expansion across most catchments. Conversely, the significant decline in grasslands emphasizes the need for conservation and regulation (Yonaba et al. Citation2021). The LULC changes like urbanization and intensification of agricultural activities have a direct effect on hydraulic properties and the environment which in turn affects the variation of hydrology.
According to Nair et al. (Citation2015), the growth of urbanization as the process of land use alteration results in significant changes in the physical properties of the land surface. As a result, there is an increase in the area of impervious surface resulting in enhanced surface runoff (Mwangi et al. Citation2017; Othow et al. Citation2017). This was supported in the study conducted by Vojtek and Vojteková (Citation2019); in the Radisa basin, Western Slovakia to estimate surface runoff, which analyzed the change in surface runoff characteristics based on the assessment of land use change between 1949 and 2017 concluded that the risk of surface runoff in the research area decreased over the studied periods due to afforestation. The intensification of agricultural activities in the catchment can also influence the increase in runoff due to soil compaction from machinery passage. The impacts of land use change on hydrology in the study conducted by Marie Mireille et al. (Citation2019) in the Kakia and Esamburmbur sub-watersheds of Narok County, Kenya, found that land use has a major impact on soil hydrological properties of the watershed.
The digital elevation model (DEM) of the study areas, provided valuable insights into the topography and slopes of the land, enabling the potential placement of check dams. It is therefore important to analyze the information derived from the DEMs to optimize the design and implementation of the dam construction system, hence for efficient and sustainable utilization of water resources in considered study areas.
Furthermore, despite that the area can receive an equal amount of precipitation, the spatial patterns appear to be controlled significantly by land cover and slope. Area with steeper gradients has more runoff, whereas topographical depressions have more recharge (Hassan et al. Citation2021). For instance, the Study conducted by Baiyinbaoligao et al. (2011) adopted the distributed Kinematic Wave hydrological model to calculate the two rainfall-runoff events in 2005 and 2006 and used Esri’s ArcView 8.3 and its spatial analyst extension module to determine the flow direction, flow accumulation, and stream network as features, with a 50 m DEM spatial data issued by Japan Geographical Survey Institute in 1996 to produce the digital basin of the Kuronagi River. They also utilized the Arc Hydro Tools to divide the catchment into 27 sub-catchments and then to calculate the slope, area, average slope, river’s length, and slope for each sub-catchment.
The results reported in show that the CN for the investigated areas is increasing by 13.88%; 3.88 and 9.85 for the Guno, Gbahali, and Sambu catchments respectively. This confirms how LULC dynamics impacted the direct runoff within the 1995–2023 period. The CN values offer essential insights into the hydrological characteristics of each catchment (Gajbhiye et al. Citation2014; Wróbel and Boczoń Citation2020). These values provide an indicator of the land’s ability to absorb and generate runoff, thereby influencing the overall water flow patterns within the region. Moreover, upstream changes pose a direct influence on rainfall-runoff parameters, especially as CN increases the runoff accordingly. For instance, according to Bussi et al. (Citation2016), watershed hydrology is affected by vegetation types, soil properties, geology, terrain, climate, land use practices, and spatial patterns of interaction, among others. The observed variations in runoff patterns can be attributed to changes in LULC, climatic conditions and watershed characteristics. The observed interannual variability can be attributed to variations in annual precipitation which is a critical driver of runoff. The high percentage of rainfall becomes runoff (+94%) indicates poor infiltration, hence high potential runoff, consequently, a need for better stormwater management practices to reverse water shortage during the dry season.
Therefore, we can state that in terms of water resources management, land management plays a key role in influencing runoff. This is in agreement with the study by Vojtek and Vojteková (Citation2019), which stated that in small basins of Golestan province, Iran subjected to great deforestation and an increase in urbanization, a 5% increase in CN strongly enhances the surface runoff.
5. Conclusion
In this study, eight (8) dam catchments namely; Guno, Gbahali, Sambu, Duago, Denugo, Kwisini, Sandu, and Nyeko catchments assessed in terms of LULC dynamics and its associated impacts on surface runoff. The results showed that the most prominent trends include substantial increases in agricultural lands with percentages ranging from 50.0 to 385.56%. Notably built-up areas have expended notably in Gbahali demonstrating the impact of urbanization, increase of population and business activities due to its proximity to Tamale metropolis Conversely, grasslands have experienced reductions in all catchments, with percentages ranging from −106.93 to −395.25% indicating ecological shifts and LULC changes justified by population growth and modernization of agriculture.
However, we could not quantitatively identify the implication of future LULC change on hydrology, also due to the lack of available gauge streamflow. Therefore, the employment of a continuous runoff simulation model with observed data for calibration and validation is still a case for further study. The findings underscore the importance of adopting sustainable land-use practices that promote both economic growth and hydrological integrity. In a large number of regions, hydrological model calibration is challenging due to a lack of streamflow observations and other ground data, this method can be used to estimate the direct runoff from a catchment.
Furthermore, we recommend gauging each dam for accurate calibration and validation based on observed data from the continuous hydrological model. By understanding these interactions, policymakers and stakeholders can make informed decisions to promote sustainable land use practices and effective water resource management in the region, ultimately enhancing the resilience of the local ecosystems and communities.
Disclosure statement
No potential conflict of interest was reported by the author(s).
Additional information
Funding
References
- Ampim PAY, Ogbe M, Obeng E, Akley EK, Maccarthy DS. 2021. Land cover changes in Ghana over the past 24 years. Sustainability. 13(9):4951. https://doi.org/10.3390/su13094951.
- Andoh C, Gupta S, Khare D. 2018. Status of rainwater harvesting (RWH) in Ghana. Curr World Environ. 13(1):172–179. https://doi.org/10.12944/CWE.13.1.17.
- Antwi-Agyei P, Dougill AJ, Stringer LC. 2015. Barriers to climate change adaptation: evidence from northeast Ghana in the context of a systematic literature review. Clim Develop. 7(4):297–309. https://doi.org/10.1080/17565529.2014.951013.
- Anua SN, Wong WVC. 2022. Utilizing Landsat 8 OLI for land cover classification in plantations area. IOP Conf Ser: earth Environ Sci. 1053(1):012027. https://doi.org/10.1088/1755-1315/1053/1/012027.
- Apollonio C, Balacco G, Novelli A, Tarantino E, Piccinni AF. 2016. Land use change impact on flooding areas: the case study of Cervaro Basin (Italy). Sustainability. 8(10):996. https://doi.org/10.3390/su8100996.
- Ara Z, Zakwan M. 2018. Estimating runoff using SCS curve number method. Int J Emerg Technol Adv Eng. 8(5):195–200.
- Bahremand A. 2006. Simulating the effects of reforestation on floods using spatially distributed hydrologic modeling and GIS. p. 186. Brussel: Vrije Universiteit Brussel.
- Baiyinbaoligao, Ding W, XiangYang L. 2011. Application of Arc GIS in the calculation of basin rainfall runoff. Proccedia Environ Sci. 10:1980–1984. doi: 10.1016/j.proenv.2011.09.310.
- Bandira PNA, Tan ML, Teh SY, Samat N, Shaharudin SM, Mahamud MA, Tangang F, Juneng L, Chung JX, Samsudin MS. 2022. Optimal solar farm site selection in the George Town conurbation using GIS-based multi-criteria decision making (MCDM) and NASA power data. Atmosphere. 13(12):2105. https://doi.org/10.3390/atmos13122105.
- Barnes DA. 2009. Assessment of rainwater harvesting in northern Ghana. p. 101. (Doctoral dissertation). Massachusetts Institute of Technology.
- Barasa BN, Perera EDP. 2018. Analysis of land use change impacts on flash flood occurrences in the Sosiani River basin Kenya. Int J River Basin Manag. 16(2):179–188. https://doi.org/10.1080/15715124.2017.1411922.
- Beegam N S, Arulraj G P. 2018. A review article on impact of urbanization on hydrological parameters. Int J Civ Eng Technol. 9(199–208):199–208.
- Bekoe J, Balana BB, Nimoh F. 2021. Social cost-benefit analysis of investment in rehabilitation of multipurpose small reservoirs in northern Ghana using an ecosystem services-based approach. Ecosyst Serv. 50(June):101329. https://doi.org/10.1016/j.ecoser.2021.101329.
- Bhatti NB, Siyal AA, Qureshi AL, Bhatti IA. 2019. Land covers change assessment after small dam’s construction based on the satellite data. Civ Eng J. 5(4):810–818. https://doi.org/10.28991/cej-2019-03091290.
- Bussi G, Dadson SJ, Prudhomme C, Whitehead PG. 2016. Modelling the future impacts of climate and land-use change on suspended sediment transport in the River Thames (UK). J Hydrol. 542:357–372. doi: 10.1016/j.jhydrol.2016.09.010.
- Descroix L, Mahé G, Lebel T, Favreau G, Galle S, Gautier E, Olivry JC, Albergel J, Amogu O, Cappelaere B, et al. 2009. Spatio-temporal variability of hydrological regimes around the boundaries between Sahelian and Sudanian areas of West Africa: a synthesis. J Hydrol. 375(1–2):90–102. https://doi.org/10.1016/j.jhydrol.2008.12.012.
- Dionizio EA, Costa MH. 2019. Influence of land use and land cover on hydraulic and physical soil properties at the Cerrado agricultural frontier. Agriculture. 9(1):1–14. https://doi.org/10.3390/agriculture9010024.
- Du S, Leng Y, Liang X, Li J, Liu W, Du Q. 2023. Degradation aware unfolding network for spectral super-resolution. IEEE Geosci Remote Sens Lett. 21:1–1. https://doi.org/10.1109/lgrs.2023.3346929.
- FAO. 2016. Map accuracy assessment and area estimation: a practical guide. National Forest Monitoring Assessment Working Paper. Rome: Food and Agriculture Organization of the United Nations. p. 69. https://scholar.google.com/citations?view_op=view_citation&hl=en&user=KQFl_6AAAAAJ&citation_for_view=KQFl_6AAAAAJ:roLk4NBRz8UC
- Gajbhiye S, Mishra SK, Pandey A. 2014. Relationship between SCS-CN AND SEDIMENT YIELD. Appl Water Sci. 4(4):363–370. https://doi.org/10.1007/s13201-013-0152-8.
- Gardelle J, Hiernaux P, Kergoat L, Grippa M. 2010. Less rain, more water in ponds: a remote sensing study of the dynamics of surface waters from 1950 to present in pastoral Sahel (Gourma region, Mali). Hydrol Earth Syst Sci. 14(2):309–324. https://doi.org/10.5194/hess-14-309-2010.
- Garen DC, Moore DS. 2005. Curve number hydrology in water quality modeling: uses, abuses, and future directions. J Am Water Resources Assoc. 41(2):377–388. https://doi.org/10.1111/j.1752-1688.2005.tb03742.x.
- Gbohoui YP, Paturel JE, Tazen F, Mounirou LA, Yonaba R, Karambiri H, Yacouba H. 2021. Impacts of climate and environmental changes on water resources: a multi-scale study based on Nakanbé nested watersheds in West African Sahel. J Hydrol Reg Stud. 35:100828. https://doi.org/10.1016/j.ejrh.2021.100828.
- Gelaro R, McCarty W, Suárez MJ, Todling R, Molod A, Takacs L, Randles CA, Darmenov A, Bosilovich MG, Reichle R, et al. 2017. The modern-era retrospective analysis for research and applications, version 2 (MERRA-2). J Climate. 30(14):5419–5454. https://doi.org/10.1175/JCLI-D-16-0758.1.
- Grimaldi S, Petroselli A, Tauro F, Porfiri M. 2012. Temps de concentration: un paradoxe dans l’hydrologie moderne. Hydrol Sci J. 57(2):217–228. https://doi.org/10.1080/02626667.2011.644244.
- Guo H, Li X, Huang Q, Zhang D. 2011. Effect of Lulc change on surface runoff inurbanization area Zhongchang SUN. In Proceedings of the ASPRS 2011 Annual Conference, Milwaukee, Wisconsin, May (Vol. 1, No. 5).
- Guzha AC, Rufino MC, Okoth S, Jacobs S, Nóbrega RLB. 2018. Impacts of land use and land cover change on surface runoff, discharge and low flows: evidence from East Africa. J Hydrol Reg Stud. 15:49–67. https://doi.org/10.1016/j.ejrh.2017.11.005.
- Habete D, Ferreira CM. 2016. Impact of forecasted land use change on design peak discharge at watershed and catchment scales: simple equation to predict changes. J Hydrol Eng. 21(7):04016019. https://doi.org/10.1061/(asce)he.1943-5584.0001384.
- Hassan NA, Gathenya JM, Raude JM. 2021. Estimating groundwater recharge rates and identifying groundwater recharge zones in Kakia and Esamburmbur Sub-catchmnet Narok, Kenya. J Sustain Res Eng. 7(1):31–45.
- Hengl T, De Jesus JM, Heuvelink GBM, Gonzalez MR, Kilibarda M, Blagotić A, Shangguan W, Wright MN, Geng X, Bauer-Marschallinger B, et al. 2017. SoilGrids250m: global gridded soil information based on machine learning. PLOS One. 12(2):e0169748. (https://doi.org/10.1371/journal.pone.0169748.
- Hong D, Zhang B, Li X, Li Y, Li C, Yao J, Yokoya N, Li H, Ghamisi P, Jia X, et al. 2023. SpectralGPT: spectral foundation model. arXiv preprint arXiv:2311.07113. p. 1–15.
- Hu S, Fan Y, Zhang T. 2020. Assessing the effect of land use change on surface runoff in a rapidly urbanized City: a case study of the central area of Beijing. Land. 9(1):17. https://doi.org/10.3390/land9010017.
- Hundecha Y, Bárdossy A. 2004. Modeling of the effect of land use changes on the runoff generation of a river basin through parameter regionalization of a watershed model. J Hydrol. 292(1-4):281–295. https://doi.org/10.1016/j.jhydrol.2004.01.002.
- Hussain S, Mubeen M, Karuppannan S. 2022. Land use and land cover (LULC) change analysis using TM, ETM + and OLI Landsat images in district of Okara, Punjab, Pakistan. Phys Chem Earth Parts A/B/C. 126(June 2021):1–10.
- Jawale A. 2019. Comparison of image classification techniques: binary and multiclass using convolutional neural network and support vector machines. INFOCOMP J Comput Sci. 18(2):28–35.
- Kalantari Z, Lyon SW, Folkeson L, French HK, Stolte J, Jansson PE, Sassner M. 2014. Quantifying the hydrological impact of simulated changes in land use on peak discharge in a small catchment. Sci Total Environ. 466–467:741–754. https://doi.org/10.1016/j.scitotenv.2013.07.047.
- Kang M, Yoo C. 2020. Application of the SCS–CN method to the Hancheon basin on the volcanic Jeju Island, Korea. Water. 12(12):3350. doi: 10.3390/w12123350.
- Kowalik T, Walega A. 2015. Estimation of CN parameter for small agricultural watersheds using asymptotic functions. Water. 7(12):939–955. https://doi.org/10.3390/w7030939.
- Manzoor SA, Griffiths GH, Robinson E, Shoyama K, Lukac M. 2022. Linking pattern to process: intensity analysis of land-change dynamics in Ghana as correlated to past socioeconomic and policy contexts. Land. 11(7):1070. https://doi.org/10.3390/land11071070.
- Marie Mireille N, M. Mwangi H, K. Mwangi J, Mwangi Gathenya J. 2019. Analysis of land use change and its impact on the hydrology of Kakia and Esamburmbur sub-watersheds of Narok County, Kenya. Hydrology. 6(4):86. https://doi.org/10.3390/hydrology6040086.
- Marzouk OA. 2021. Assessment of global warming in Al Buraimi, sultanate of Oman based on statistical analysis of NASA POWER data over 39 years, and testing the reliability of NASA POWER against meteorological measurements. Heliyon. 7(3):e06625. https://doi.org/10.1016/j.heliyon.2021.e06625.
- Mishra SK, Singh VP. 2006. A relook at NEH-4 curve number data and antecedent moisture condition criteria. Hydrol Processes. 20(13):2755–2768. https://doi.org/10.1002/hyp.6066.
- Mohamed MA, Anders J, Schneider C. 2020. Monitoring of changes in land use/land cover in Syria from 2010 to 2018 using multitemporal Landsat imagery and GIS. Land. 9(7):226. https://doi.org/10.3390/land9070226.
- Mohd Hasmadi I, Pakhriazad HZ, Shahrin MF. 2009. Evaluating supervised and unsupervised techniques for land cover mapping using remote sensing data. Malaysia J Soc Space. 5(1):1–10.
- Mounirou LA, Yonaba R, Koïta M, Paturel JE, Mahé G, Yacouba H, Karambiri H. 2021. Hydrologic similarity: dimensionless runoff indices across scales in a semi-arid catchment. J Arid Environ. 193:104590. https://doi.org/10.1016/j.jaridenv.2021.104590.
- Mounirou LA, Yonaba R, Tazen F, Ayele GT, Yaseen ZM, Karambiri H, Yacouba H. 2022. Soil erosion across scales: assessing its sources of variation in Sahelian landscapes under semi-arid climate. Land. 11(12):2302. https://doi.org/10.3390/land11122302.
- Mustafa YM, Amin MSM, Lee TS, Shariff ARM. 2012. Evaluation of land development impact on a tropical watershed hydrology using remote sensing and GIS. J Spat Hydrol. 5(2):16–30.
- Mwangi HM, Lariu P, Julich S, Patil SD, McDonald MA, Feger KH. 2017. Characterizing the intensity and dynamics of land-use change in the Mara River Basin, East Africa. Forests. 9(1):8. https://doi.org/10.3390/f9010008.
- Nair AM, Vijayan A, George B. 2015. Impact of temporal variation on land use on surface runoff-a case study of Cochin city. Int J Adv Earth Sci Eng. 4(1):265–274.
- Ngurah G, Dharmayasa P, Simatupang CA, Sinaga DM. 2022. NASA Power’s: an alternative rainfall data resources for hydrology research and planning activities in Bali Island, Indonesia. J Infrastruct Plan Eng. 1(1):1–7.
- Nyatuame M, Agodzo S, Amekudzi LK, Mensah-Brako B. 2023. Assessment of past and future land use/cover change over Tordzie watershed in Ghana. Front Environ Sci. 11:1–14. https://doi.org/10.3389/fenvs.2023.1139264.
- Obahoundje S, Diedhiou A. 2022. Potential impacts of climate, land use and land cover changes on hydropower generation in West Africa: a review. Environ Res Lett. 17(4):043005. https://doi.org/10.1088/1748-9326/ac5b3b.
- Ohana-Levi N, Givati A, Alfasi N, Peeters A, Karnieli A. 2018. Predicting the effects of urbanization on runoff after frequent rainfall events. J Land Use Sci. 13(1–2):81–101. https://doi.org/10.1080/1747423X.2017.1385653.
- Othow OO, Sl G, Gemeda DO. 2017. Analyzing the rate of land use and land cover change and determining the causes of forest cover change in Gog district, Gambella regional state. Ethiopia J Remote Sens. GIS. 6(4):218.
- Owar Othow O, Legesse Gebre S, Obsi Gemeda D. 2017. Analyzing the rate of land use and land cover change and determining the causes of forest cover change in Gog District, Gambella Regional State, Ethiopia. J Remote Sens GIS. 06(04):218. https://doi.org/10.4172/2469-4134.1000219.
- Pechlivanidis IG, Jackson BM, Mcintyre NR, Wheater HS. 2011. Catchment scale hydrological modelling: a review of model types, calibration approaches and uncertainty analysis methods in the context of recent developments in technology and applications. Global Nest J. 13(3):193–214. https://doi.org/10.30955/gnj.000778.
- Piscopia R, Petroselli A, Grimaldi S. 2015. A software package for predicting design-flood hydrographs in small and ungauged basins. J Agricult Engineer. 46(2):74–84. https://doi.org/10.4081/jae.2015.432.
- Praskievicz S, Chang H. 2011. Impacts of climate change and urban development on water resources in the Tualatin River Basin, Oregon. Ann Assoc Am Geograph. 101(2):249–271. https://doi.org/10.1080/00045608.2010.544934.
- Rodrigues GC, Braga RP. 2021. Evaluation of NASA power reanalysis products to estimate daily weather variables in a hot summer Mediterranean climate. Agronomy. 11(6):1207. https://doi.org/10.3390/agronomy11061207.
- Ross CW, Prihodko L, Anchang J, Kumar S, Ji W, Hanan NP. 2018. HYSOGs250m, global gridded hydrologic soil groups for curve-number-based runoff modeling. Sci Data. 5(1):180091. https://doi.org/10.1038/sdata.2018.91.
- Sertel E, Topaloğlu RH, Şallı B, Algan IY, Aksu GA. 2018. Comparison of landscape metrics for three different level land cover/land use maps. IJGI. 7(10):408. https://doi.org/10.3390/ijgi7100408.
- Sitterson J, Knightes C, Parmar R, Wolfe K, Avant B, Muche M. 2018. An overview of rainfall-runoff model types. Types An Overview of Rainfall-Runoff Model. September. p. 0–29.
- Stewart D, Canfield E, Hawkins R. 2012. Curve number determination methods and uncertainty in hydrologic soil groups from semiarid watershed data. J Hydrol Eng. 17(11):1180–1187. https://doi.org/10.1061/(asce)he.1943-5584.0000452.
- Toure SI, Stow DA, Clarke K, Weeks J. 2020. Patterns of land cover and land use change within the two major metropolitan areas of Ghana. Geocarto Int. 35(2):209–223. https://doi.org/10.1080/10106049.2018.1516244.
- Umukiza E, Raude JM, Petroselli A, Wandera SM, Gathenya JM, Apollonio C. 2022. Drainage systems design in urbanized areas under land use changes scenarios. Acta Hydrologica Slovaca. 23(2):29–37. https://doi.org/10.31577/ahs-2022-0023.02.0017.
- Umukiza E, Raude JM, Wandera SM, Petroselli A, Gathenya JM. 2021. Impacts of land use and land cover changes on peak discharge and flow volume in Kakia and Esamburmbur sub-catchments of Narok Town, Kenya. Hydrology. 8(2):82. https://doi.org/10.3390/hydrology8020082.
- USDA-NRCS. 2010. National engineering handbook chapter 15, time of concentration. p. 1–15.
- Vojtek M, Vojteková J. 2019. Land use change and its impact on surface runoff from small basins: a case of Radiša basin. Folia Geographica. 61(2):104–125.
- Wróbel M, Boczoń A. 2020. Determining the potential retention of a forest catchment based on the CN parameter. Model Earth Syst Environ. 7(3):2145–2148. https://doi.org/10.1007/s40808-020-00938-z.
- Yang W, Jin F, Si Y, Li Z. 2021. Runoff change controlled by combined effects of multiple environmental factors in a headwater catchment with cold and arid climate in northwest China. Sci Total Environ. 756:143995. https://doi.org/10.1016/j.scitotenv.2020.143995.
- Yang W, Long D, Bai P. 2019. Impacts of future land cover and climate changes on runoff in the mostly afforested river basin in North China. J Hydrol. 570:201–219. https://doi.org/10.1016/j.jhydrol.2018.12.055.
- Yomo M, Villamor GB, Aziadekey M, Olorunfemi F, Mourad KA. 2020. Climate change adaptation in semi-arid ecosystems: a case study from Ghana. Clim Risk Manage. 27:100206. https://doi.org/10.1016/j.crm.2019.100206.
- Yonaba R, Biaou AC, Koïta M, Tazen F, Mounirou LA, Zouré CO, Queloz P, Karambiri H, Yacouba H. 2021. A dynamic land use/land cover input helps in picturing the Sahelian paradox: assessing variability and attribution of changes in surface runoff in a Sahelian watershed. Sci Total Environ. 757:143792. https://doi.org/10.1016/j.scitotenv.2020.143792.
- Yonaba R, Koïta M, Mounirou LA, Tazen F, Queloz P, Biaou AC, Niang D, Zouré C, Karambiri H, Yacouba H. 2021. Spatial and transient modelling of land use/land cover (LULC) dynamics in a Sahelian landscape under semi-arid climate in northern Burkina Faso. Land Use Pol. 103:105305. https://doi.org/10.1016/j.landusepol.2021.105305.
- Yonaba R, Mounirou LA, Tazen F, Koïta M, Biaou AC, Zouré CO, Queloz P, Karambiri H, Yacouba H. 2023. Future climate or land use? Attribution of changes in surface runoff in a typical Sahelian landscape. Comptes Rendus. Géoscience. 355(S1):1–28.
- Zeng Z, Tang G, Hong Y, Zeng C, Yang Y. 2017. Development of an NRCS curve number global dataset using the latest geospatial remote sensing data for worldwide hydrologic applications. Remote Sens Lett. 8(6):528–536. https://doi.org/10.1080/2150704X.2017.1297544.
- Zhu H, Li Q, Miao K, Wang J, Hou B, Jiao L. 2024. LargeRSDet: a large mini-batch object detector for remote sensing images. IEEE Geosci Remote Sensing Lett. 21:1–5. https://doi.org/10.1109/LGRS.2023.3345946.