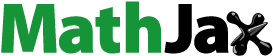
Abstract
This study seeks to identify the key wavelengths and spectral transformation techniques that may be used to accurately estimate soil nitrogen, phosphorus, and potassium (NPK) concentration in the Hutton soils. For this purpose, the reflectance spectra of 74 soil samples were measured in the laboratory, and the chemical properties of the soil were analysed. Pearson correlation (r), stepwise multiple linear regression (SMLR), and Partial Least Square Regression were used to model the relationships between the selected variables under study. The study findings revealed that moderate to weak correlations (r = −0.2 − 0.6) exist between wavelengths (400 − 2500 nm) and soil NPK content. Important wavelengths for accurately predicting Soil NPK content were found within visible (400 nm − 729nm), near-infrared (805 nm − 1200nm) and mid-infrared region (1375 nm to 2462 nm). The PLSR model coupled with different spectral transformations was found to be the optimal model for estimating soil NPK (RPD > 2). The introduction of the first and second derivate spectral transformation approach markedly improved all the models investigated in this study. The results retrieved from the study provide a high-accuracy prediction of soil nutrient contents, which is critical for soil fertility management and sustainable food production.
1. Introduction
Soil is one of the most significant natural resources on Earth, it offers essential nutrients, minerals, and water for plant growth (Banerjee and van der Heijden Citation2023). Nutrients including Nitrogen, Potassium and Phosphorus (NPK) are critical for enhancing soil fertility and fostering plant development (Patel and Ghosh Citation2019). Techniques like laboratory-based chemical analysis and soil testing procedures assisted in determining soil fertility status (Patel and Ghosh Citation2019). The nutrient uptake that occurs during plant growth and fertilization has a direct impact on the NPK nutrient contents in the soil (Qi et al. Citation2017). Fertilizers are very expensive, and if they are not utilised properly, they may harm the environment. Nutrient-deficient soil results in stunted growth and poor yields, whereas nutrient-rich soil may result in toxicity with detrimental effects on yields (Masrie et al. Citation2017). To promote better soil fertility management and efficient crop productivity, it is therefore critical to assess soil nutrient status. This may be done by rapidly and precisely retrieving the available information on the NPK nutrients (Jin et al. Citation2020).
Laboratory (chemical analysis) based estimations of soil properties are limited in that they are point-based and do not apply to monitor the spatial and temporal dynamics of chemical component contents over large areas (Zhai et al. Citation2013). Most laboratories in developing countries use the Kjeldahl method to determine N (Chagas et al. Citation2021), Olsen’s and Bray’s method (Lumbanraja et al. Citation2017) to quantify P and Gravimetric or Volumetric sodium tetraphenyl boron method (Correndo et al. Citation2021) to determine K in the soil. Although these techniques are accurate and reliable, they produce noxious fumes and hazardous chemical laboratory waste (Sharifi et al. Citation2009). There have been also health and safety concerns associated with the Kjeldahl method, especially in the handling of concentrated acid, heavy metals such as Selenium (Se), precipitation of salt or cracking of sample digestion, and uneven digestion (Sharifi et al. Citation2009). Therefore, remote sensing technologies provide rapid, safe, spatial explicit assessment of soil nutrient content and offer the opportunity for monitoring these elements at a local, regional, and global scale at a lower cost (Peng et al. Citation2019). This is why most researchers are interested in using remote sensing techniques. There are few hyperspectral remote sensing platforms available for the estimation of soil NPK, which include, spaceborne, airborne and ground-based systems. Airborne and spaceborne hyperspectral images are now believed to be useful data sources for estimating the chemical contents of soil over large areas. Hively et al. (Citation2011) used an airborne hyperspectral imaging spectrometer to map soil properties at grain farms in Chesapeake Bay, USA. While Xu et al. (Citation2022) demonstrated the use of ZY1-02D spaceborne hyperspectral imager for estimation and mapping of soil N content in the black soil area of China. Although airborne and spaceborne hyperspectral platforms are becoming popular, their availability (e.g. Hymap, Hyperion and AVIRIS are not readily available in Africa), cost and quality limit their extensive use in estimating soil properties in many developing countries (Yu et al. Citation2020).
As an alternative data source, Near-Infrared (NIR) and Visible (Vis) spectroscopy is the most widely recognised soil detecting technique (adopted for both field and laboratory estimations) that is used to assess soil properties and is also regarded as complementary to chemical laboratory examination techniques (Pantazi et al. Citation2016). This is mainly because the technique is fast and non-destructive, and it has high spectral resolution with minimal atmospheric interferences when compared to space and airborne hyperspectral platforms. The data from Laboratory-Based Spectroscopy has been utilized for various soil studies such as soil gradation (Zribi et al. Citation2012; Ewing et al. Citation2020), soil salinity mapping (Nguyen et al. Citation2020), and soil quality mapping (Selige et al. Citation2006; Vohland et al. Citation2022). For example, Patel and Ghosh (Citation2019) used hyperspectral remote sensing data to successfully assess soil fertility status in Uttarakhand, India. Although many studies have been done to estimate NPK content in the soil using the hyperspectral data, in most cases, the soil conditions are location-specific (due to, for instance, climate, management, crops etc), consequently, results obtained at one location cannot be extrapolated to other areas. Moreover, the most effective combination of spectral retrieval models (e.g. Linear, or non-linear) and spectral transformations for the assessments of soil NPK content has not been identified, especially in Hutton soils of Limpopo Province, South Africa.
Most of the studies have focused on traditional chemical analysis methods for determining NPK in the Hutton soils e.g. (Moshia et al. Citation2008; Phefadu and Kutu Citation2016; Munjonji et al. Citation2017). Consequently, this study seeks to test the applicability of Laboratory-Based Spectroscopy to estimate NPK content in the Hutton soils. This will help to improve soil health and maximise agricultural productivity while reducing the cost of soil analysis and preventing the over and under the application of nutrients for plant growth. Therefore, the objectives of this study were to (i) determine the spectral key wavelengths that can be used to estimate soil NPK and (ii) model soil NPK based on spectral data transformations at Syferkuil Farmlands, South Africa.
2. Materials and methods
2.1. Study area description
The study was conducted at the Syferkuil Experimental Farm (SEF) of the University of Limpopo (). Syferkuil farm is found within Capricorn District Municipality, Limpopo Province, South Africa of coordinates (23°51′0″S. 29°42′0″E). The study area is situated about 9 km North-West of the University of Limpopo main campus. The main objective of the SEF is to provide effective student training and research tools for staff and students. The farm has a semi-arid climate, with average minimum and maximum temperatures ranging from 4 to 20°C in winter and 17 to 27°C in summer. The area receives annual average rainfall ranging from 400 to 600 mm (Munjonji et al. Citation2020). On this farm, the soils are formed in situ on sandstone, basalt, biotic processes, and classified as Hutton soils (Phefadu and Kutu Citation2016). It is about 1 650 ha in size, with 25 ha of cropland that is not irrigated and 80 ha of irrigated cropland. Approximately, 77 ha of irrigated land is provided with a consistent irrigation system and rotating and cultivating winter or summer crops use 40 ha simultaneously. Maize, soybeans, sugar beans and sorghum are the summer crops found on the farm (Phefadu and Kutu Citation2016).
2.2. Data and pre-processing
2.2.1. Soil sampling and laboratory analysis
A field survey was undertaken between January and March 2019 at Syferkuil Experimental Farm. A random sampling method was adopted for this study, to ensure that all parts of the study area have an equal chance of being sampled with no operator bias (Chen et al. Citation2009). Seventy-four soil samples (n = 74) were randomly collected at 0 – 20 cm depth using Auger. The sampling locations were recorded using a handheld GPS GPSmap 60CSx, Garmin, Ltd., Kansas, TX, USA) at sub-meter accuracy. The spatial distribution of the soil sampling points was superimposed on a high-resolution georeferenced Google Earth imagery using ArcMap 10.8.
In the laboratory, soil samples were air-dried and sieved to pass through a 2 mm sieve. The sieved soil samples were weighed, scanned (spectral measurements), packaged and sent to an accredited laboratory for soil nutrients (NPK) analysis. P and K were extracted using Ambic-2 solution and determined using atomic absorption while total N was analyzed by the Automated Dumas dry combustion method using a LECO CNS 2000 (LECO Corporation, Michigan).
2.3. Measurements and pre-processing of soil samples
Laboratory soil reflectance measurements were carried out in a dark room at the Risk and Vulnerability Science Center, University of Limpopo, South Africa on 8 June 2019. The seventy-four (74) samples were scanned using a contact probe of a portable spectroradiometer (FieldSpec® 4 Optical Sensor, Analytical Spectral Devices (ASD) Inc., Longmont, CO, USA). ASD measures wavelengths from 350 to 2500 nm and with 3 – 10 nm spectral resolution. The spectral sampling interval was 1.4 nm in the 350 − 1000 nm range and 1.1 nm in the 1001–2500 nm range. Each soil sample was measured four times, and the average of the four measurements was taken to remain with one measurement for every single point. A Spectralon (Labsphere Inc., Sutton, NH, USA) reference panel (white reference) was used to optimize the ASD instrument prior to taking reflectance measurements for each soil sample. The averaging of the dataset was carried out using RS3 View Spec pro 6.0 software produced by ASD. The intensity of the incoming light, the overlapping peaks and the variation of the baseline are known to affect the spectrum, in this case, there was a need for pre-processing of the data to correct these interferences (Bangelesa et al. Citation2020). To solve the problem of low radiation that was appearing in the spectrum edge, it was necessary to remove the noisy ends. Consequently, wavelengths ranging from 350 – 400 nm were removed, since it had the noise caused by the instability of the equipment. Other wavelengths ranging from 1350 to 1460, 1790 to 1960 and 2350 − 2500 nm were also removed because they are water vapour absorption bands, and they were reported to have effects on the estimation models (Smith et al. Citation2003). The laboratory spectra were also smoothed using the Savitzky-Golay filter with a window size of 15 spectral bands and a polynomial degree of two (Savitzky and Golay Citation1964), and, subsequently, resampled to a resolution of 5 nm using a cubic spline interpolation (Tavakoli et al. Citation2023), in the intervals between 400 and 930 nm and 1070 and 2150 nm. Subsequently, a total of 1900 spectral bands between 400 nm and 2400 nm were used for the analyses. The spectral reflectance of soil can be difficult to analyse using raw data (RD), consequently, the first derivative (FD) and second derivative spectra were computed in RS3 ViewSpec pro 6.0 software to improve predictive accuracy (Peng et al. Citation2019). Thus, shows the untransformed spectral reflectance of the raw data (RD), (B) shows untransformed raw spectral data with noisy ends removed, and (C) shows the first derivative (FD). Whereases, shows the second derivation data spectral curve of the soil samples under study.
2.4. Data analysis
Firstly, the normality of soil NPK values within the datasets was checked using the Kolmogorov–Smirnov goodness-of-fit test to guide the selection of the most suitable statistical test (Massey Citation1951). Thereafter, Kruskal–Walli’s test was utilized to compare the training, testing, and whole datasets because of the lack of normality in the dataset (McKnight and Najab Citation2010).
2.4.1. Selection of key wavelengths for estimating soil NPK content
To select the important wavelengths for estimating soil NPK content, Pearson correlation was used to examine the relationship between total nitrogen, potassium, and phosphorus with soil spectral reflectance data. The Pearson correlation is the most common method to use for selecting key predictive variables based on the strength of a linear relationship between two or multiple variables (Nettleton Citation2014). This method assigns a value between −1 and 1, where 0 is no correlation, 1 is a total positive correlation, and −1 is a total negative correlation (see ). In this study, spectral wavelengths with strong correlations were deemed as important for estimating NPK. Pearson correlation was assumed as follows:
(1)
(1)
Table 1. A table showing correlation coefficient interpretation.
Table 2. A table of descriptive statistics of NPK nutrient content of the soil in sykerfuil farm.
Where denotes the correlation coefficient between x and y. Then
the values of x within a sample and
is the value of y within a sample. Whereas
the average of the values of X within a sample and
is the average of the values within a sample (Cohen et al. Citation2009).
In addition, Principal component analysis (PCA) biplot analysis, were adopted to explore the relationships between soil reflectance spectra and NPK concentrations as demonstrated by (Cheng et al., Citation2019). Three major factors were used in PCA biplot analysis for each soil attribute and the soil spectra. For visualization, the connections between NPK and soil reflectance spectra were combined into the 3 × 3 array of plots. Gower et al. (Citation2016) have provided a description of PCA biplot analysis in depth.
2.4.2. Modelling soil NPK content
The soil NPK (predicted variables) was estimated from the spectral data (predictor variables) using the Stepwise Multiple Linear regression model (SMLR). SMLR is a technique that iteratively evaluates each independent variable’s statistical significance in a linear regression model (Kokaly and Clark Citation1999). It may also be used to filter and eliminate redundant variables when estimating soil nutrients (Song et al. Citation2019). This model was used in this study since it can exclude variables with a low rate of concentration while retaining those with a high rate of contribution. The subsequent formula for SMLR is as follows:
(2)
(2)
Where is the soil nutrient (N, P, K),
are variable vectors corresponding to spectral data or indices,
and
are the coefficients that characterise the model and the 𝜀 is the additive bias (Corona et al., Citation1998). To further explore important wavelengths derived for laboratory-based spectroscopy for the estimation of soil NPK, Partial least squares regression (PLSR) models were adopted. The variable importance in projection (VIP) (Farrés et al. Citation2015) and PLSR coefficients (b-coefficients) (Salehi-Varnousfaderani et al. Citation2022) were utilized to determine the important wavelengths used in the PLSR models. According to Farrés et al. (Citation2015) the VIP threshold should be set as 1, however in this study it was set at 2 to reduce the spectral redundancy and Salehi-Varnousfaderani et al. (Citation2022) approach was adopted were they determined the b-coefficient thresholds based on their standard deviations. Therefore, when the b-coefficient was larger than the standard deviation and the VIP score was greater than 2, spectral wavelengths were deemed significant.
2.4.3. Accuracy assessment
The optimal SMLR and PLSR model for estimating soil NPK was determined using the coefficient of determination (r2) and root mean square error (RMSE) of the observed and estimated values. Ratio performance to deviation (RPD) was calculated to produce results for the prediction quality models. RPD was considered as the ratio of standard deviation to RMSE. To calculate the Coefficient of determination, RMSE and RDP, the following formulas were used.
(3)
(3)
Whereby, m is assumed to be the number of testing samples within the approval set, yi is the genuine value of sample i, f () is the anticipated value of sample i, and y is the normal value of y (Qi et al. Citation2017)
(4)
(4)
Where ym are NPK values measured directly from laboratory measurement, yp are predicted values inferred from spectral information using the SMLR and PLSR model, and N refers to the number of samples. Ratio Performance to Deviation (RPD) was calculated as follows.
(5)
(5)
Where SD is the standard deviation of the observed NPK values and RMSE is the root mean square error. According to Viscarra Rossel and Lark (Citation2009), the interpretation of RPD can be categorised as follows:
RPD > 2.5 = excellent prediction
RPD between 2.0 & 2.5 = very good prediction
RPD between 1.8 & 2.0 = good prediction
RPD between 1.4 & 1.8 = fair prediction
RPD between 1.0 & 1.4 = poor prediction
RPD <1.0 = very poor prediction
3. Results
3.1. Descriptive analysis
displays the statistical data for NPK nutrient concentration ranging from 0.00 to 0.25%, 0.64 to 29.09 mg kg−1 and 67.22 to 867.32 mg kg−1, respectively. N had an approximately symmetric skewness distribution, with a mean value of 0.10% and a standard deviation of 0.06. P and K values demonstrated a positive highly skewed distribution with a kurtosis that is larger than 0, mean value of 6.12 mg kg−1 and 206.991 mg kg−1 and standard deviation of 6.17 and 125.1812 mg kg−1, respectively. As a result, the NPK distribution would be regarded as offset to the right since the skewness is greater than 0.
3.2. Determination of key wavelengths to estimate soil NPK
3.2.1. Correlation analysis
Pearson correlation was used to determine the relationship between soil NPK, and spectral reflectance as shown in . A negligible positive correlation (0.07 <r > 0.25) was observed across all soil spectral reflectance’s for N (). The visible (i.e. blue) (400- 500 nm) yielded the lowest correlation (0.07 <r > 0.12) when related to Soil N. Whereas, the green and red spectra (500 − 750 nm) achieved the greatest correlation (r) for N, ranging from 0.1 to 0.25. Concurrent with the latter, the near-infrared (750 nm − 1.4 nm) and short-wavelength infrared (1.4 − 2349 nm) infrared spectra also yielded low positive correlations ranging from 0.24 − 0.21. Moreover, P as demonstrated in managed to achieve correlations ranging from −0.33 to 0.61, thus denoting low to moderate positive and negative correlation across all spectral regions. The visible spectra showed a moderate positive correlation (0.0<r > 0.61) when compared to all other spectral regions for soil P content. Interestingly, the red-edge region portrayed negligible negative correlation (−0.33 <r> −0.32) with P. Whereas, near- and short-wavelength infrared regions demonstrated weak negative correlations (-0.28 <r> −0.18 and −0.21 <r> −0.19), respectively.
In addition, , shows weak negative correlations that exist between K and soil spectral data ranging from −0.25 to −0.05. Specifically, the wavelengths in the visible spectrum (750 nm − 1.4 nm) demonstrated weak negative correlations ranging from −0.25 to −0.05. Weak negative correlations (-0.18 <r> −0.16, −0.21 <r> −0.17) were also observed between K and wavelengths found within the near and short-wavelength infrared region (750 nm − 1.4 and 1.4 nm − 2349 nm), respectively. The overall findings indicated that there are significant (p < 0.05) relationships that exist between spectral wavelengths derived using Laboratory-Based Spectroscopy and in-situ soil NPK measurements.
3.2.2. Models to estimate NPK based on spectral wavelengths
Soil NPK content was predicted using original spectral data (raw data) and transformed data (first and second derivatives) with the aid of SMLR, PSLR and PCA biplot analysis.
Key wavelengths were distinctively selected using SMLR. As shown in , for raw data (RD), the identified key wavelengths for estimating N were 729 nm, then for P they ranged from 404 nm to 1 011 nm and no wavelength was identified for K due to the model saturation. In addition, key wavelengths identified to be effective for the prediction of NPK ranged from 400 nm to 2 488 nm, 2 462 nm and 417 nm to 2011, for the first derivative (FD). Whereas, for the second derivative (SD), 352 nm and 1003 nm were identified as key wavelengths for the estimation of N, then for P, 400 nm and 999 nm were identified and for K, they ranged from 356 to 382 nm. All these wavelengths fall within the visible and near-infrared regions of the electromagnetic spectrum.
Table 3. Table showing SMLR model performance for the prediction of NPK soil content based on raw spectral data (RD), first derivative (FD) and second derivative spectra (SD).
The pre-processing transformation techniques provided various prediction accuracies for the NPK regarding the values of r2, RMSE and RPD. further shows the predictive accuracy performance of the NPK soil nutrients produced using original spectral data (Raw Data), First Derivative and Second Derivative. The results showed that for the raw data, the fitting accuracy of SMLR for soil N yielded the lowest coefficient of determination (r2) of 0.06 and root means square error (RMSE) of 8.30. Whereas soil P yielded a good coefficient of determination (r2) of 0.85 and RMSE of 0.12 and for soil K no model was produced due to model saturation. Moreover, the first derivative spectral transformation managed to yield the largest coefficient of determination for soil P (r2 = 0.99, RMSE = 8.67), followed by soil N (r2 = 0.91, RMSE = 0.12) and soil K (r2 = 0.60; RMSE = 241.46). The second derivative performed poorly for all nutrients (see ). For instance, SD models managed to only explain less than 20% of the data variations for N (r2 = 0.16; RMSE = 0.12), P (r2 = 0.17, RMSE = 8.67) and K (r2= 0.19; RMSE = 241.46).
Normally, a model that performs well will have a low RMSE and a high r2 and RPD. Considering N in , RPD increased from 0.01 (raw data) to 2.40 (FD & SD). For P, it has decreased from 2.6 (RD) to 0.71 (FD and SD). Whereas K obtained an RPD of 1.84 for the first derivative and 0.52 for the second derivative. Compared to N and K the results showed that P performed well only when using the original spectral data rather than transformed data, whereas N accuracy was improved by data transformation. Phosphorus showed an RPD that is greater than 2 presenting an excellent prediction. The results showed that phosphorus performed better with the first derivative than any other models, with RPD that is greater than 2 showing an excellent prediction.
The results of the PLSR models for the estimation of NPK based on different spectral wavelengths and transformations are shown in . The results portray the influence of spectral transformations on the model performance. For instance, the poorest estimation result was observed using FD method to estimate soil N yielding the lowest R2 of 0.67, RMSE of 0.005 and RDP of 1.59. In addition, the overall performance FD was very low when compared to other techniques for all the soil properties under study, with R2 for P and K ranging from 0.73 to 0.88, RPD and AIC ranging from 1.62 to 5.88 and −246 to −374.17, respectively. SD method was observed shown to be the best spectral transformation technique to accurately estimate the soil NPK by achieving the highest R2 ranging from 0.95 to 0.98, with RPD and AIC ranging from 3.52 to 7.63 and −318.64 to −348.76, respectively. Whereas PLSR RD method preformed overall intermediately for estimating soil NPK. For instance, excellent predictions were obtained for K (R2 = 0.92, RMSE = 0.004, RPD = 4.65 and AIC =378.45), P (R2 = 0.82, RMSE = 0.004, RPD = 2.03 and AIC =−253.25) and fair prediction for N (R2 = 0.72, RMSE = 0.008, RPD = 1.11 and AIC = −242.97).
Table 4. PLSR model performance for the prediction of NPK soil content based on raw spectral data (RD), first derivative (FD) and second derivative spectra (SD).
shows important wavelength plots for predicting soil N (a-c), P (d-f) and K (g–i) based on various spectral transformations Variable Importance Projection (VIP) and the b-coefficient derived from PLSR model. Important wavelengths for estimating N were found to be in the visible (400 – 800 nm) and Short-wave infrared (2300 − 2349n) region surpassing the threshold of 2 for raw spectra (RD) (). Near-infrared region (900 -1200nm) proved to be useful for estimating P () and K () based on raw spectra (RD). Other interesting results were observed for N (), P (), and K (), showing the highest estimation power for the visible wavelength region and shortwave infrared region with an importance value greater than the threshold of 2. The results inf further demonstrated the superiority of the second derivative (SD) spectral transformation for estimating soil NPK, with a clear distinction of important wavelengths by eliminating redundant information. For instance, important wavelengths for estimating soil N () and K () were found to be in the visible regions (400-406nm), near infrared region (1011 − 1016 nm) and shortwave infrared region (1788 nm − 1968 nm). On the other hand, important wavelengths for estimating P () were found only in the near-infrared (400 -496nm), and shortwave infrared (1200 − 2259 nm) region of the electromagnetic spectrum.
Figure 4. Important wavelength plots for predicting soil N (a- c), P (d-f) and K (g – i) based on various spectral transformations, PLSR model, Variable Importance projection (VIP) and the b-coefficients.

illustrates the principal component loadings and scores and in case of normal distribution of the scores, to determine the relationships between the soil NPK and spectral reflectance. Firstly, the Kolmogorov-Smirnov tests and histograms for all the three scores show varying distribution, with p-values ranging from 0.05 to 0.07 (), thus biplot diagrams are proper for classification of Soil NPK to their spectral characteristic properties. Normal distribution was only observed on PC3, whereas PC1 and PC2 showed positive skewness. The black points in the figure represent the soil samples (N = 74). Certain points demonstrated superior performance in comparison to the remaining investigated samples, since they were the most distinguishable or located furthest from the biplot origin. On the other hand, some points were nearer the biplot origin, indicating that the qualities we evaluated have less variability in these samples. Since PC1 held the majority of the original spectral information, it was chosen to represent and examine the spectral information and its connections to soil parameters. The degree of correlation between the two variables is shown by the cosine of the angle formed by the soil property and PC1. For instance, from , it is evident that there was a strong and significant correlation between N and K concentrations. Moreover, the first two principal components (PC1 and PC2) were then chosen despite their poor performance since they were able to only explain 34% of the variation in the spectral data (). PC3 demonstrated the lowest performance by managing to only explain 31% of the variation in the spectral data. In , cumulative Proportion for the first three components ranged from 34 to 100 interestingly.
Figure 5. Biplot of the principal components (PC1 -PC3) (red) and scores (black) for the relationship between soil NPK and soil reflectance spectra.

Table 5. Explained and the accumulated explained variances of the first three principal components of soil reflectance spectra.
4. Discussion
This study investigated the most effective wavelengths, spectral transformations and selected statistical models for the estimation of soil nitrogen, phosphorus, and potassium content in Hutton soils. Determination of spectral key wavelengths is crucial for several reasons, including ensuring targeted measurements, enhancing the accuracy and precision of the estimations and maximising efficiency. Non-destructive analyses are particularly beneficial in agricultural contexts, where timely decisions are essential for crop management. As described in Section 3.2.1 the results showed that very weak to strong correlations (−0.20 < r > 0.61) were observed between NPK soil nutrients and spectral bands. More specifically, the wavelength found in the visible (400 nm − 750nm) and near-infrared spectrum (800 nm − 1300 nm) proved to be more sensitive to NPK soil nutrients. These nutrients often have distinct absorption bands within the visible and near-infrared spectrum due to the electronic transitions of their constituent atoms or ions (Soriano-Disla et al. Citation2014). For instance, in this study, a weak positive correlation (0.07<r > 0.25) was observed across all soil spectral reflectance’s for N. The weak correlations could be attributed to the very low reflectance of soil N in both visible and near-infrared (VNIR) spectral regions. Therefore, the presence or concentration of nitrogen in the soil in this study could not lead to a significant change in the reflectance properties of soil within the VNIR range (Vibhute et al. Citation2020). Soil phosphorus (P) demonstrated moderate to positive and negative correlations (r) ranging from −0.33 to 0.61 when related to all spectral regions. These results show a complex relationship that exists between soil P and hyperspectral variables. For instance, soil P content may affect the reflectance properties of the soil in some spectral regions, while in others, its influence might be less pronounced (Tavares et al. Citation2023). The unique absorption and scattering characteristics of P in these regions may have led to varying correlation strengths observed in this study. However, only the visible spectra performed better when compared to another spectrum, yielding a moderate positive correlation (0.0 <r > 0.61). This occurred because compounds related to phosphorus, such as iron phosphate minerals in the soils, can have absorption features in the visible spectrum. These absorption features can create patterns in the reflectance spectrum that correlate with the concentration of such compounds, hence influencing the correlation (Zahir et al. Citation2023). The results of the study also showed weak negative correlations between potassium (K) and soil spectral data (−0.25 <r> −0.05). Potassium absorption features appeared to be in the Short-wave infrared (SWIR) region between 1400 nm and 1700 nm. These absorption features are related to potassium-bearing minerals. Spectral bands within this range, such as 1600 nm (known as the "K-band"), are sensitive to potassium content. Some of these selected wavelengths are in general agreement with the previous studies (Guo et al. Citation2021; Peng et al. Citation2021; Kim et al. Citation2023).
Moreover, different spectral data transformations, SMLR, PLSR and PCA biplots analysis were explored for their utilisation in estimating NPK in Hutton soils. Results showed that raw spectra within the visible region such as 729 nm, 404 nm to 1011 nm can be used to estimate N and P in Hutton soils using SMLR model. However, raw spectra also proved to be not useful for predicting soil K based on SMLR, since the model saturated and produced no results (). When we delve into the first derivative transformation, wavelengths found in the visible to short wave infrared region (400 nm to 2 488 nm, 2 462 nm and 377 nm to 2011) were identified as key for estimating Soil NPK. Similarly, specific spectral wavelengths such as 400, 422, 490, 453, 454, 999, and 1003 nm were identified as important for the determination of Soil NPK based on second derivative transformation. These results concur with the findings of previous studies such as Farrar et al. (Citation2023). Comparing our results with similar studies such as Lin et al. (Citation2020) SMLR performed poorly in estimating Soil NPK using original (RD) (r2 = 0.06 − 0.17), first derivative (r2 = 0.60 − 0.99)), second derivative (r2 = 0.17 − 0.19). Poor performance of the SMLR could be attributed to model overfitting, especially when there are many predictor variables (i.e. 2500 spectral wavelengths) relative to the sample size (n = 74). Including too many variables can lead to a model that fits the training data very well but performs poorly on new, unseen data (Babyak Citation2004). SMLR is also prone to Multicollinearity issue (Johnston et al. Citation2018). Consequently, in order to overcome the shortcomings of the SMLR, the PLSR model was also employed to estimate soil NPK based on different transformations of spectral data. The collinearity issue can be effectively resolved with the help of PLSR linear modelling technique. PLSR model in this study yielded excellent predictions for K (r2 = 0.88 − 0.95), P (r2 = 0.73 − 0.98) and fair prediction for N (r2 = 0.72 − 0.98) when subjected to different spectral transformations (). These results demonstrate that Laboratory-Based Spectroscopy coupled with PLSR model is a promising method to estimate the concentration of Soil NPK in Hutton soils. The results concur with similar results from previous studies such as Shi et al. (Citation2013) and Song et al. (Citation2018). Although, PLSR performed relatively well, PLSR model is linear and there are still nonlinear correlations between reflectance spectra and soil characteristics. Therefore, the PLSR model may have certain shortcomings when it comes to handling nonlinear interactions. In order to enhance the effectiveness of estimating models for soil NPK, future research may concentrate on examining nonlinear estimation approaches (such as artificial neural networks, or ANN) or the combination of linear and nonlinear techniques (such as PLSR-ANN). In attempts to model the relationship between Soil NPK and Spectral data, PCA biplots () demonstrated the lowest performance explaining the maximum of 34% and minimum of 31% of the variation in the spectral data (). Insufficient variability in the data and small sample size could be attributed to poor performance of the PCA biplots. The analysis needs enough data points to accurately estimate the covariance or correlation matrix. The PCA biplots results of this study were found to be very poor when compared to similar studies such as Cheng et al. (2019).
The study also further explored the predictive accuracy of the SMLR, PLSR and data transformations for estimating soil NPK by comparing the RPD values. The results showed that SMLR achieved the lowest RPD values for NPK ranging from 0.01 to 2.61. The highest RPD was observed for second derivative transformation for predicting N (RPD = 2.40) and K (RPD = 2.61). Other spectral transformations yielded low RPD of less than 2 portraying poor prediction capability. These results are similar to the study by Shi et al. (Citation2013) which discovered a very poor accuracy for N with RPD < 1.0 in all models. The reason behind this is directed by the fact that the estimation accuracy of the models is usually lower when developed using a dataset with a small range value. Furthermore, Fystro (Citation2002) reported that in most circumstances, each spectrum sample could be predicted to have distinct data in a small heterogeneous sample population, and more samples could be acquired for calibration to produce excellent estimation accuracy. PLSR models on the other hand demonstrated overall excellent predictions for N (RPD = 1.11 − 7.63), P (RPD = 1.62 − 6.66), and K (RPD = 3.52 − 5.88) when subjected to different spectral transformations. PSLR was deemed to be the optimal model for estimating soil NPK using laboratory-based spectroscopy in this study. These results concur with the studies by Malmir et al. (Citation2019) and El-Sayed et al. (Citation2023) who used partial least square regression, least square-support vector regression model (LS-SVR) and machine learning algorithms to estimate soil NPK with varying model performances. Different predictive performances may be because of differences in quality, type of data, and the area of study. In future studies, models that were calibrated in our study require validation in other areas and should further be compared with other statistical methods such as linear and non-linear models. In addition, this current study only explored two data transformation techniques (FD and SD), therefore future studies the combination of different spectral transformations such as Fractional Order Derivative (FOD) and Fractional Order Savitzky-Golay (FOSG) should be investigated to improve the estimation accuracies of Soil NPK in Hutton soils.
5. Conclusions
This study focused on the performance of laboratory-based spectroscopy in estimating the NPK nutrient content of the Hutton soils using distinctive data transformation approaches, SMLR, PLSR, and PCA biplots analysis. The selection of key wavelengths was assessed based on RD, FD, and SD. The results revealed that (i) among the three predicted soil nutrients, Phosphorus had the best fitting accuracy based on original spectral data (raw data) with (R2 = 0.85, RMSE = 0.21 mg kg-1 and RPD> 2) when explored using SMLR. Whereas PCA biplots performed very poor in determining the relationship between the soil reflectance and soil NPK, explaining less than 34% of the variation in the spectral data across all principal components. PLSR improved the estimation accuracy (i.e. RPD ranging from 1.1 to 7) of all soil properties significantly, surpassing the SMLR and PCA biplots analysis.
Furthermore, the results have showed that (ii) transformation methods such as FD and SD improve the raw spectral data because, with K, SMLR model saturation was observed leading to no model being produced when using raw data but with transformed spectral data, models were generated. Improvement in the accuracy of the N SMLR prediction model was observed due to data transformation for example R2 increasing from 0.06 to 0.91. Similar improvements were also observed when transformed soil reflectance data was subjected to PLSR model. Overall, the second derivative transformation approach proved to be useful for predicting Soil NPK using both SMLR and PLSR in Hutton soils. Lastly (iii) the key wavelength to predict NPK contents was found within visible light and near-infrared for both raw and transformed data ranging from 400 nm to 2462 nm as indicated by correlation, Variable Importance Projection (VIP) and the b-coefficients (400–2400 nm), using the PLSR models.
Therefore, in this study, we can conclude that PLSR model demonstrated superior performance for estimating soil NPK. Yet, this study offers numerous perspectives to conduct future research. It is also important to note that findings presented in this study are specific to the conditions under which the research was conducted, primarily focusing on the properties of the soil type used. It is important to recognize that variations in soil composition, environmental factors, and experimental procedures can significantly influence the outcomes of analyses such as Stepwise multiple linear regression (SMLR), Principal Component Analysis (PCA) and Partial Least Squares Regression (PLSR). Therefore, the results and interpretations provided here are constrained to the characteristics of the soil type investigated in this study. For a broader applicability or generalization of these findings, further research encompassing diverse soil types and environmental contexts is warranted. We recommend future researchers estimate soil nutrient contents using the analysis of soil spectroscopy in Hutton soils to use different statistical methods such as, non-linear models, machine learning, and deep learning models, as well as explore more other multivariate analysis, chemometrics and spectral transformations such as fractional order derivative and Fractional Order Savitzky-Golay to improve the estimations.
Disclosure statement
No potential conflict of interest was reported by the author(s).
Data availability statement
The data that support the findings of this study are available from the corresponding author, [FM], upon reasonable request.
Additional information
Funding
References
- Babyak MA. 2004. What you see may not be what you get: a brief, nontechnical introduction to overfitting in regression-type models. Psychosom Med. 66(3):411–421. doi: 10.1097/01.psy.0000127692.23278.a9.
- Banerjee S, van der Heijden MG. 2023. Soil microbiomes and one health. Nat Rev Microbiol. 21(1):6–20. doi: 10.1038/s41579-022-00779-w.
- Bangelesa F, Adam E, Knight J, Dhau I, Ramudzuli M, Mokotjomela TM. 2020. Predicting soil organic carbon content using hyperspectral remote sensing in a degraded mountain landscape in lesotho. Appl Environ Soil Sci. 2020:1–11. doi: 10.1155/2020/2158573.
- Chagas JO, Gomes JM, Rodrigues JHV, de Matos Cunha IC, De Melo NFS, Da Silva GA, Lobo FA. 2021. Nitrogen, phosphorous, and potassium polymeric microparticles: application and validation of analytical methods for determination of a promising fertilizer. Eclética Química. 46(2):36–47.
- Cheng H, Shen R, Chen Y, Wan Q, Shi T, Wang J, Wan Y, Hong Y, Li X. 2019. Estimating heavy metal concentrations in suburban soils with reflectance spectroscopy. Geoderma. 336:59–67. doi: 10.1016/j.geoderma.2018.08.010.
- Chen J, Gu S, Shen M, Tang Y, Matsushita B. 2009. Estimating aboveground biomass of grassland having a high canopy cover: an exploratory analysis of in situ hyperspectral data. Int J Remote Sens. 30(24):6497–6517. doi: 10.1080/01431160902882496.
- Cohen I, Huang Y, Chen J, Benesty J. 2009. Pearson correlation coefficient. Noise Reduction in Speech Process. 2:1–4. doi: 10.1007/978-3-642-00296-0_5.
- Corona MEP, Aldana BRVD, Criado BG, Ciudad AG. 1998. Variations in Nutritional Quality and Biomass Production of Semiarid Grasslands. Journal of Range Management. 51(5):570.doi: 10.2307/4003378.
- Correndo AA, Rubio G, García FO, Ciampitti IA. 2021. Subsoil-potassium depletion accounts for the nutrient budget in high-potassium agricultural soils. Sci Rep. 11(1):11597. doi: 10.1038/s41598-021-90297-1.
- El-Sayed MA, Abd-Elazem AH, Moursy AR, Mohamed ES, Kucher DE, Fadl ME. 2023. Integration Vis-NIR spectroscopy and artificial intelligence to predict some soil parameters in arid region: a case study of Wadi Elkobaneyya, South Egypt. Agronomy. 13(3):935. doi: 10.3390/agronomy13030935.
- Ewing J, Oommen T, Jayakumar P, Alger R. 2020. Utilizing hyperspectral remote sensing for soil gradation. Remote Sens. 12(20):3312. doi: 10.3390/rs12203312.
- Farrar MB, Wallace HM, Tahmasbian I, Yule CM, Dunn PK, Bai SH. 2023. Rapid assessment of soil carbon and nutrients following application of organic amendments. Catena. 223:106928. doi: 10.1016/j.catena.2023.106928.
- Farrés M, Platikanov S, Tsakovski S, Tauler R. 2015. Comparison of the variable importance in projection (VIP) and of the selectivity ratio (SR) methods for variable selection and interpretation. J Chemom. 29(10):528–536. doi: 10.1002/cem.2736.
- Fystro G. 2002. The prediction of C and N content and their potential mineralisation in heterogeneous soil samples using Vis–NIR spectroscopy and comparative methods. Plant and Soil. 246(2):139–149. doi: 10.1023/A:1020612319014.
- Gower JC, Le Roux NJ, Gardner−Lubbe S. 2016. Biplots: qualititative data. WIREs Computational Stats. 8(2):82–111. doi: 10.1002/wics.1377.
- Guo P, Li T, Gao H, Chen X, Cui Y, Huang Y. 2021. Evaluating calibration and spectral variable selection methods for predicting three soil nutrients using vis-nir spectroscopy. Remote Sens. 13(19):4000. doi: 10.3390/rs1319:4000.
- Hively WD, McCarty GW, Reeves JB, Lang MW, Oesterling RA, Delwiche SR. 2011. Use of airborne hyperspectral imagery to map soil properties in tilled agricultural fields. Appl Environ Soil Sci. 2011:1–13. doi: 10.1155/2011/358193.
- Jin X, Li S, Zhang W, Zhu J, Sun J. 2020. Prediction of soil-available potassium content with visible near-infrared ray spectroscopy of different pretreatment transformations by the boosting algorithms. Applied Sciences (Switzerland). 10(4):1520. doi: 10.3390/app10041520.
- Johnston R, Jones K, Manley D. 2018. Confounding and collinearity in regression analysis: a cautionary tale and an alternative procedure, illustrated by studies of British voting behaviour. Qual Quant. 52(4):1957–1976. doi: 10.1007/s11135-017-0584-6.
- Kim MJ, Lee JE, Back I, Lim KJ, Mo C. 2023. Estimation of total nitrogen content in topsoil based on machine and deep learning using hyperspectral imaging. Agriculture. 13(10):1975. doi: 10.3390/agriculture13101975.
- Kokaly RF, Clark RN. 1999. Spectroscopic determination of leaf biochemistry using band-depth analysis of absorption features and stepwise multiple linear regression. Remote Sens Environ. 67(3):267–287. doi: 10.1016/S0034-4257(98)00084-4.
- Lin L, Gao Z, Liu X. 2020. Estimation of soil total nitrogen using the synthetic color learning machine (SCLM) method and hyperspectral data. Geoderma. 380:114664. doi: 10.1016/j.geoderma.2020.114664.
- Lumbanraja J, Mulyani S, Utomo M, Sarno S. 2017. Phosphorus extraction from soil constituents using Bray P-1, Mehlich-1 and Olsen Solutions. J Trop Soils. 22(2):67–76. doi: 10.5400/jts.2017.v22i2.67-76.
- Malmir M, Tahmasbian I, Xu Z, Farrar MB, Bai SH. 2019. Prediction of soil macro-and micro-elements in sieved and ground air-dried soils using laboratory-based hyperspectral imaging technique. Geoderma. 340:70–80. doi: 10.1016/j.geoderma.2018.12.049.
- Masrie M, Rosman MSA, Sam R, Janin Z. 2017. Detection of nitrogen, phosphorus, and potassium (NPK) nutrients of soil using optical transducer. In 2017 IEEE 4th International Conference on Smart Instrumentation, Measurement and Application (ICSIMA). IEEE. doi: 10.1109/ICSIMA.2017.8312001.
- Massey Jr FJ. 1951. The Kolmogorov-Smirnov test for goodness of fit. J Am Stat Assoc. 46(253):68–78. doi: 10.1080/01621459.1951.10500769.
- McKnight PE, Najab J. 2010. Mann−Whitney U Test. The Corsini Encyclopedia of Psychol. 1:1–1. doi: 10.1002/9780470479216.corpsy0491.
- Moshia ME, Mashatola MB, Shaker P, Fouché PS, Boshomane MAW. 2008. Land suitability assessment and precision farming prospects for irrigated maize-soybean intercropping in syferkuil experimental farm using geospatial information technology. J Agric Soc Res. 8(2):1–12. doi: 10.4314/jasr.v8i2.43351.
- Munjonji L, Ayisi KK, Vandewalle B, Dhau I, Boeckx P, Haesaert G. 2017. Yield performance, carbon assimilation and spectral response of triticale to water stress. Ex Agric. 53(1):100–117. doi: 10.1017/S0014479716000107.
- Munjonji L, Ayisi KK, Mudongo EI, Mafeo TP, Behn K, Mokoka MV, Linstädter A. 2020. Disentangling drought and grazing effects on soil carbon stocks and CO2 fluxes in a semi-arid African savanna. Front Environ Sci. 8:1–14. doi: 10.3389/fenvs.2020.590665.
- Nettleton D. 2014. Pearson correlation-an overview. Science Direct Topics. Available at: https://www.sciencedirect.com/topics/computer-science/pearson-correlation (Accessed 19 Septermber 2023).
- Nguyen KA, Liou YA, Tran HP, Hoang PP, Nguyen TH. 2020. Soil salinity assessment by using near-infrared channel and Vegetation Soil Salinity Index derived from Landsat 8 OLI data: a case study in the Tra Vinh Province, Mekong Delta, Vietnam. Prog Earth Planet Sci. 7(1):1–16. doi: 10.1186/s40645-019-0311-0.
- Pantazi XE, Moshou D, Bravo C. 2016. Active learning system for weed species recognition based on hyperspectral sensing. Biosyst Eng. 146:193–202. doi: 10.1016/j.biosystemseng.2016.01.014.
- Patel AK, Ghosh JK. 2019. Soil fertility status assessment using hyperspectral remote sensing. SPIE-Intl Soc Optical Eng. 14. doi: 10.1117/12.2533115.
- Peng Y, Wang L, Zhao L, Liu Z, Lin C, Hu Y, Liu L. 2021. Estimation of soil nutrient content using hyperspectral data. Agriculture. 11(11):1129. doi: 10.3390/agriculture11111129.
- Peng Y, Zhao L, Hu Y, Wang G, Wang L, Liu Z. 2019. Prediction of soil nutrient contents using visible and near-infrared reflectance spectroscopy. IJGI. 8(10):437. doi: 10.3390/ijgi8100437.
- Phefadu KC, Kutu FR. 2016. Evaluation of spatial variability of soil physico-chemical characteristics on rhodic ferralsol at the syferkuil experimental farm of University of Limpopo, South Africa. JAS. 8(10):92. doi: 10.5539/jas.v8n10p92.
- Qi H, Paz-Kagan T, Karnieli A, Li S. 2017. Linear multi-task learning for predicting soil properties using field spectroscopy. Remote Sens. 9(11):1099. doi: 10.3390/rs9111099.
- Salehi-Varnousfaderani B, Honarbakhsh A, Tahmoures M, Akbari M. 2022. Soil erodibility prediction by Vis-NIR spectra and environmental covariates coupled with GIS, regression and PLSR in a watershed scale, Iran. Geoderma Regional. 28:e00470. doi: 10.1016/j.geodrs.2021.e00470.
- Savitzky A, Golay MJ. 1964. Smoothing and differentiation of data by simplified least squares procedures. Anal Chem. 36(8):1627–1639. doi: 10.1021/ac60214a047.
- Selige T, Böhner J, Schmidhalter U. 2006. High-resolution topsoil mapping using hyperspectral image and field data in multivariate regression modelling procedures. Geoderma. 136(1-2):235–244. doi: 10.1016/j.geoderma.2006.03.050.
- Sharifi M, Zebarth BJ, Burton DL, Grant CA, Hajabbasi MA, Abbassi−Kalo G. 2009. Sodium hydroxide direct distillation: A method for estimating total nitrogen in soil. Commun Soil Sci Plant Anal. 40(15–16):2505–2520. doi: 10.1080/00103620903111376.
- Shi T, Cui L, Wang J, Fei T, Chen Y, Wu G. 2013. Comparison of multivariate methods for estimating soil total nitrogen with visible/near-infrared spectroscopy. Plant Soil. 366(1–2):363–375. doi: 10.1007/s11104-012-1436-8.
- Smith ML, Martin ME, Plourde L, Ollinger SV. 2003. Analysis of hyperspectral data for estimation of temperate forest canopy nitrogen concentration: comparison between an airborne (AVIRIS) and a spaceborne (Hyperion) sensor. IEEE Trans Geosci Remote Sensing. 41(6):1332–1337. doi: 10.1109/TGRS.2003.813128.
- Song X, Gao Y, Liu Z, Zhang M, Wan Y, Yu X, Liu W, Li L. 2019. Development of a predictive tool for rapid assessment of soil total nitrogen in wheat-corn double cropping system with hyperspectral data. Environ Pollutants and Bioavail. 31(1):272–281. doi: 10.1080/26395940.2019.1679041.
- Song YQ, Zhao X, Su HY, Li B, Hu YM, Cui X. 2018. Predicting spatial variations in soil nutrients with hyperspectral remote sensing at regional scale. Sensors (Basel). 18(9):3086. doi: 10.3390/s18093086.
- Soriano-Disla JM, Janik LJ, Viscarra Rossel RA, Macdonald LM, McLaughlin MJ. 2014. The performance of visible, near-, and mid-infrared reflectance spectroscopy for prediction of soil physical, chemical, and biological properties. Appl Spectrosc Rev. 49(2):139–186. doi: 10.1080/05704928.2013.811081.
- Tavakoli H, Correa J, Sabetizade M, Vogel S. 2023. Predicting key soil properties from Vis-NIR spectra by applying dual-wavelength indices transformations and stacking machine learning approaches. Soil Tillage Res. 229:105684. doi: 10.1016/j.still.2023.105684.
- Tavares TR, de Almeida E, Junior CRP, Guerrero A, Fiorio PR, de Carvalho HWP. 2023. Analysis of total soil nutrient content with X-ray Fluorescence Spectroscopy (XRF): Assessing different predictive modeling strategies and auxiliary variables. AgriEngineering. 5(2):680–697. doi: 10.3390/agriengineering5020043.
- Vibhute AD, Kale KV, Gaikwad SV, Dhumal RK. 2020. Estimation of soil nitrogen in agricultural regions by VNIR reflectance spectroscopy. SN Appl Sci. 2(9):1–8. doi: 10.1007/s42452-020-03322-9.
- Viscarra Rossel RA, Lark RM. 2009. Improved analysis and modelling of soil diffuse reflectance spectra using wavelets. European J Soil Science. 60(3):453–464. doi: 10.1111/j.1365-2389.2009.01121.x.
- Vohland M, Ludwig B, Seidel M, Hutengs C. 2022. Quantification of soil organic carbon at regional scale: benefits of fusing vis-NIR and MIR diffuse reflectance data are greater for in situ than for laboratory-based modelling approaches. Geoderma. 405:115426. doi: 10.1016/j.geoderma.2021.115426.
- Xu Z, Chen S, Zhu B, Chen L, Ye Y, Lu P. 2022. Evaluating the capability of satellite hyperspectral imager, the ZY1–02D, for topsoil nitrogen content estimation and mapping of farmlands in black soil area, China. Remote Sens. 14(4):1008. doi: 10.3390/rs14041008.
- Yu H, Kong B, Wang Q, Liu X, Liu X. 2020. Hyperspectral remote sensing applications in soil: a review. In: Hyperspectral remote sensing. Amsterdam, Netherlands: Elsevier; p. 269–291. doi: 10.1016/b978-0-08-102894-0.00011-5.
- Zahir S, Jamlos MF, Omar AF, Jamlos MA, Mamat R, Muncan J, Tsenkova R. 2023. Review–Plant nutritional status analysis employing the visible and near-infrared spectroscopy spectral sensor. Spectrochim Acta A Mol Biomol Spectrosc. 304:123273. doi: 10.1016/j.saa.2023.123273.
- Zhai Y, Cui L, Zhou X, Gao Y, Fei T, Gao W. 2013. Estimation of nitrogen, phosphorus, and potassium contents in the leaves of different plants using laboratory-based visible and near-infrared reflectance spectroscopy: comparison of partial least-square regression and support vector machine regression methods. Int J Remote Sens. 34(7):2502–2518. doi: 10.1080/01431161.2012.746484.
- Zribi M, Kotti F, Lili-Chabaane Z, Baghdadi N, Issa NB, Amri R. 2012. Analysis of soil texture using TERRASAR X-band SAR. In 2012 IEEE International Geoscience and Remote Sensing Symposium. p. 7027–7030.