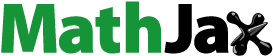
ABSTRACT
Background and Aims
To evaluate the association of Chinese visceral adiposity index (CVAI) and its dynamic trends with risk of renal damage, and to compare its prediction performance with that of other obesity indices.
Methods and Results
A community-based population with 23 905 participants from Shantou city was included in the cross-sectional analysis. A total of 9,778 individuals from two separated cohort were included in the longitudinal portion. Five patterns of CVAI change were predefined (low-stable, decreasing, moderate, increasing, and persistent-high). Logistic and Cox regressions were used to evaluate the association between CVAI and renal damage. We explored potential mechanisms using the mediating effect method, and the prediction performance was determined by receiver operating characteristic curve analysis. Results from both cross-sectional and longitudinal data revealed a positive and linear association between CVAI and risk of renal damage. Pooled analysis of the two cohorts showed that per unit increase in Z score of CVAI induced 18% increased risk of renal damage (P = .008). Longitudinal trends of CVAI were also associated with renal damage, and the moderate, increasing, and persistent-high patterns showing a higher risk. Blood pressure and glucose had a mediating effect on renal damage induced by CVAI. Among several obesity indices, CVAI was the optimal for predicting renal damage.
Conclusion
A higher level of immediate CVAI and longitudinal increasing and persistent-high patterns of CVAI were independently associated with increased risk of renal damage. Monitoring immediate level and long-term trend of CVAI may contribute to the prevention of renal damage.
Introduction
Chronic kidney disease (CKD) is a global health threat as it has a strong association with increased risk of adverse events, including cardiovascular disease, end-stage renal disease and premature mortality (Citation1,Citation2). CKD affects about 13.4% of adults worldwide, and it serves as the 11th cause of death (Citation3,Citation4). The prevalence of CKD has exceeded 10% in China, where 120 million people are affected (Citation5). Along with an ongoing epidemic of metabolic disorders, such as hypertension, diabetes and obesity, CKD has been rapidly increasing (Citation6). Both general and abdominal obesity are associated with the occurrence and progression of renal damage, and a greater impact of abdominal obesity has been observed (Citation7,Citation8). Recently, visceral adiposity has become the focus of research because it has been shown to be more closely related to target organ injury (Citation9,Citation10).
Simple indicators for measuring visceral fat accumulation are advised in clinical practice and epidemiological surveys because of the limitations of standard tools, such as magnetic resonance imaging and computed tomography. The Chinese visceral adiposity index (CVAI) was recently established for evaluation of visceral fat and metabolic health status among the Chinese population (Citation11). It has been demonstrated to outperform other adiposity indicators in predicting hypertension, diabetes and atherosclerotic cardiovascular disease (Citation12–14). Several studies have shown a cross-sectional association between visceral obesity and CKD (Citation15–17), whereas the association of CVAI with renal damage have been rarely reported either in cross-sectional or longitudinal studies. In addition, a growing number of studies have focused on the impact of the longitudinal trends of weight and waist circumference (WC) on disease and death, given the fact that obesity status could change over time (Citation18–20). However, studies evaluated the association between changes in visceral fat and kidney damage is limited.
Therefore, this study was conducted to assess the association between CVAI and renal damage in the general population, aiming to provide evidence, from cross-sectional to longitudinal analyses, and also the impacts of CVAI change. In addition, we evaluated the discriminating/predicting performance of CVAI for kidney disease, and explored the potential mechanisms of visceral fat accumulation inducing renal damage.
Materials and methods
Study design and population
Individuals for this study were participants who received a health examination in two districts (Chaonan and Haojiang) of Shantou, China from 2012 to 2021. During this period 27 301 individuals participated in the investigation. The study profile is shown in . Briefly, in the cross-sectional analysis, all participants were included except for those younger than 18 years, and those who have any missing data or history of malignant tumor. Those who participated in at least two investigations were included in the longitudinal data of this study. For the purpose of evaluating the longitudinal association, those with renal damage at baseline were excluded. This study was performed in accordance with the Declaration of Helsinki and approved by the ethics committee of the First Affiliated Hospital of Shantou University Medical College, and written informed consent was obtained.
For the validation and generalization of the results, data from another cohort were used to confirm the longitudinal association between CVAI and renal damage. The China Health and Retirement Longitudinal Study (CHARLS) is an ongoing nationally representative cohort study. The details of CHARLS have been described elsewhere (Citation21), and data are available at its website (http://charls.pku.edu.cn/). In short, a total of 17 708 participants from 28 provinces of China participated in a survey at baseline (2011–2012, wave 1). Physical measurements were performed every two years, and blood samples were collected once every two follow-up periods. Those who participated in both wave 1 and wave 3 (2015–2016) surveys and had complete blood data were selected for the longitudinal data of the present study. The selection process is shown in . Finally, 5,733 eligible individuals were included.
Data collection
Data were collected face-to-face by trained investigators using a predesigned questionnaire that included age, sex, height, weight, waist circumference (WC), smoking status (yes or no), alcohol consumption (yes or no), blood pressure (BP), fasting plasma glucose (FPG), total cholesterol (TC), triglycerides (TG), low-density lipoprotein cholesterol (LDL-C), high-density lipoprotein cholesterol (HDL-C), aspartate amino transferase (AST), alanine amino transferase (ALT), serum creatinine, and date of investigation. Hypertension was defined as a systolic BP (SBP) ≥140 mmHg and/or diastolic BP (DBP) ≥90 mmHg, or the current use of antihypertensive medications. Diabetes was defined as FPG ≥7.0 mmol/L, or a history of diabetes diagnosed by a physician. Cardio-cerebrovascular disease was indicated if the participants had a self-reported history of coronary heart disease, heart failure, or stroke. BP was measured in a seated position after resting for at least 5 min by investigators using electric devices, and an average of three readings was used for analysis. Reagents for measuring the above biomarkers were sourced from Abbott Laboratories and blood samples were processed using Alinity c chemistry analyzer (Abbott Laboratories, Illinois, USA).
Primary exposure and outcome
The primary exposure was CVAI, which was calculated as follows (Citation11):
Several forms of exposure were defined using baseline CVAI. First, a standardized CVAI value was analyzed as continuous variable. Second, the study population was divided into four groups according to CVAI quartile, with quartile 1 (Q1) and Q4 serving as the lowest and highest groups, respectively. Third, dichotomous groups were defined using the optimal cutoff value derived from receiver operating characteristic (ROC) curve analysis.
Five change patterns were identified based on the longitudinal changes of CVAI from baseline to follow-up visit (ΔCVAI). Low-stable was defined as baseline CVAI ≤ 68 (the upper limit value of the lowest quartile of CVAI) and changes of ΔCVAI less than 25%. Decreasing pattern was defined as decrease of ΔCVAI more than 25%, and increasing pattern was defined as an increase of ΔCVAI more than 25%. Moderate pattern was defined as baseline CVAI ranging from 68 to 122 and changes of ΔCVAI less than 25%. Persistent-high was defined if baseline CVAI ≥ 122 (the lower limit value of the highest quartile of CVAI) and changes of ΔCVAI less than 25%.
The study outcome was renal damage which was defined as an estimated glomerular filtration rate (eGFR) <60 ml/min/1.73 m2. eGFR was calculated using the simplified modification of diet in renal disease (MDRD) equation based on data from Chinese adults (Citation22). In addition, in the CHARLS cohort, patient self-reported CKD was also considered an outcome event.
Statistical analysis
Baseline characteristics were summarized and compared between renal damage and non-renal damage groups in the cross-sectional data, and across CVAI quartile groups in the longitudinal data. Data are presented as mean ± standard deviation (SD), median (interquartile range), or number (percentage). The Student’s t test or Mann–Whitney U test were used for comparison of continuous variables between groups, while the Chi-square test was used for categorical variables. Linear trends were investigated across CVAI quartile groups.
Three adjustment models were constructed and introduced into the subsequent analyses to evaluate the independent association between CVAI and renal damage. Potential confounding factors were selected based on previous literature reports, clinical knowledge and collinearity testing. Model 1 was adjusted for age, sex, BMI, smoking status, and alcohol consumption. Model 2 further considered SBP, FPG, TC, LDL-C, ALT, and AST. Model 3 considered the adjusted variables in model 2 plus health status, including hypertension, diabetes, and cardio-cerebrovascular diseases. Linear regression was used to determine the correlation of CVAI with renal function (measured by eGFR), with the results reported as changes (B coefficients) in eGFR induced by per unit increase in Z score of CVAI. The linear mixed model was applied to verify the correlation between baseline CVAI and changes of renal function in the longitudinal data, and the slope of eGFR from baseline to follow-up was reported. Logistic regression and Cox proportional hazards models were constructed to evaluate the cross-sectional and longitudinal association between CVAI and renal damage. Estimated coefficients for risk of renal damage were calculated for CVAI quartiles (reference to the first quartile), high CVAI group (reference to the low group), and per unit increase in Z score of CVAI. We also incorporated restricted cubic splines into the multivariable models to explore the shape of the association. Data of the CHARLS cohort were used to confirm the longitudinal association of CVAI and its change patterns with the risk of renal damage. Results in the longitudinal data from the two cohorts were pooled using fixed effect meta-analysis.
To evaluate heterogeneity of the associations, stratification analyses with interaction terms were performed according to age (<60 or ≥60 years), sex (male or female), BMI (<24 or ≥24 kg/m2), hypertension (yes or no), diabetes (yes or no), smoking status (yes or no), and alcohol consumption (yes or no). Receiver operating characteristic (ROC) curve analysis was applied to examine the performance of CVAI for discriminating/predicting renal damage. The area under ROC curve (AUC) of the CVAI was directly compared with other traditional and novel indices using the Delong test, including the visceral adiposity index (VAI), BMI, WC, waist-to-height ratio (WHtR), lipid accumulation product index (LAP), and body round index (BRI). Given the impact of fat accumulation on blood pressure and glucose levels, we attempted to explore whether these factors played a mediating effect, between CVAI and renal damage, by using the change in values from baseline to follow-up visit (including ΔSBP, ΔDBP, and ΔFPG). Statistical analyses were performed using R version 4.0.3 and SPSS 23.0 for Windows. A two-sided P < .05 was considered to indicate statistical significance.
LAP (Citation23) and BRI (Citation24) were calculated as follow:
Results
Characteristics of the study population in the cross-sectional analysis
A total of 23 905 participants were included for the cross-sectional analysis, had a mean age of 54.8 ± 15.2 years, and 69.6% were women. Details of the baseline characteristics are shown in . Renal damage was identified in 2,335 participants (9.8%). The proportion of hypertension and diabetes was 45.4% and 15.8%, respectively. Those with renal damage tended to have higher BMI, WC, BP, FPG, and blood lipid levels; and they were more likely to have hypertension, diabetes, and cardio-cerebrovascular diseases ().
Table 1. Baseline characteristics of study population in the cross-sectional analysis.
Cross-sectional association of CVAI with renal function and renal damage
Linear regression showed that CVAI was negatively correlated with renal function. After adjusting for demographic factors, biochemical indicators and health status, per unit increase in Z score of CVAI was correlated with a nearly 4 unit decrease of eGFR (Table S1). The prevalence of renal damage was 2.9%, 7.3%, 11.1%, and 17.7% across the respective quartiles of CVAI (P for trend < .001). The multivariable logistic model adjusted for age, sex, smoking, alcohol consumption, BMI, SBP, FPG, TC, LDL-C, ALT, AST and disease status revealed an independent and significant association between CVAI and renal damage (Table S2). The odds of renal damage for those in quartiles 2, 3, and 4 of CVAI were 1.56, 2.06, and 2.98 times higher as compared with those in the first quartile of CVAI (all P < .001). Every 10 units and per unit increase in Z score of CVAI was associated with 16% and 104% increased odds of renal damage (P < .001), respectively. Consistent results were yield in subgroup analyses (Figure S1), with stronger associations observed for female, non-obese individuals, and nonsmokers (P for interaction < 0.05).
Baseline characteristics of the study population in the longitudinal analysis
A total of 9,778 individuals (4,045 from the Shantou community and 5,733 from the CHARLS cohort) were included in the longitudinal analysis. Baseline characteristics across quartiles of CVAI are presented in Table S3. Compared to those in the lowest CVAI quartile group, participants in the other quartile groups were older and had higher levels of BMI, WC, BP (SBP, DBP), FPG, and serum lipids (TG, TC, LDL-C). In contrast, HDL-C levels decreased with increasing CVAI quartiles. Increases in CVAI quartile came with increased percentages of hypertension, diabetes, and cardio-cerebrovascular diseases (P < .05). In the Shantou cohort, the incidence of renal damage was 13.8/1000 person-years during the total follow-up of 15 218 person years (median: 3.2 years; interquartile range: 1.7–5.7 years). The follow-up time among quartile groups was comparable (P = .215), whereas the incidence of renal damage was significantly increased with an increase in CVAI quartile (Table S3). The Kaplan-Meier curve showed a similar trend in cumulative incidence (Figure S2). In the CHARLS cohort, 259 participants (4.5%) developed renal damage by the wave 3 survey, and the incidence rate across CVAI quartile was 2.8, 4.7, 5.1 and 5.4% (P for trend = .001).
Longitudinal association of CVAI with renal function and renal damage
The multivariable linear regression model revealed that per unit increase in Z score of baseline CVAI induced a 3.34 unit decrease of follow-up eGFR (Table S4). The linear mixed model confirmed the results, with a slope of −1.76 obtained from the fully adjusted model (Table S4). Multivariable models of both separate cohorts yielded similar results that baseline CVAI was significantly associated with risk of renal damage (). Pooled analysis showed that individuals in CVAI quartiles 2 to 4 had 72% to 83% increased risk of developing renal damage, after adjustment for demographics, and biochemical and medical factors. Per unit increase in Z score in CVAI corresponded to an 18% increased risk of renal damage (P = .008). A linear association was observed between the two (). Consistent trends were found in subgroup analysis, including age (<60 or ≥60 years), BMI (<24 or ≥ 24), sex, hypertension, diabetes, smoking and alcohol consumption status (Figure S3).
Figure 2. Restricted cubic spline analyses for the association between CVAI and renal damage in the Shantou population (A and B) and CHARLS population (C and D). Results for the Shantou population were adjusted for age, sex, smoking, alcohol consumption, BMI, SBP, FPG, TC, LDL-C, ALT, AST, hypertension, diabetes, and cardio-cerebrovascular disease. Results for the CHARLS population were adjusted for age, sex, smoking, alcohol consumption, educational status, SBP, FPG, TC, LDL-C, hypertension, diabetes, cardiovascular disease, and stroke.

Table 2. Longitudinal association between CVAI and incident renal damage.
CVAI change patterns and renal damage
The details of sample distribution among the five patterns of CVAI change were shown in Table S5. Compared with the low-stable pattern, the moderate, increasing, and persistent-high patterns had significantly higher incidence of renal damage in the two populations. As shown in , pooled analysis adjusted for all confounding factors revealed that there was 107%, 80%, and 93% increased risk of developing renal damage for the moderate, increasing, and persistent-high patterns (all P < .05), respectively.
Table 3. Association between CVAI change patterns and incident renal damage.
Prediction performance and mediating effects
Results of ROC analysis of both cross-sectional (Table S6) and longitudinal (Table S7) studies suggested that CVAI had the highest AUC, which was significantly higher than VAI, BMI, WC, WHtR, BRI, and LAP, indicating an optimal performance for discriminating/predicting renal damage. For exploring the potential mechanisms between CVAI and renal damage, we further evaluated whether BP and glucose had mediating effects. As expected, there were significant mediating effects of SBP (5.4%), DBP (4.6%), and FPG (6.2%) changes from baseline to follow-up point (all P < .05, Figure S4).
Discussion
In the present study, we observed a linear association between CVAI and risk of renal damage in the general population, with similar results obtained in both cross-sectional and longitudinal analysis, and also in two separate populations. Individuals with higher CVAI level had increased risk of developing renal damage, whether elderly or young and middle-aged, male or female, overweight/obese or normal, hypertensive or non-hypertensive, or diabetic or non-diabetic. Furthermore, longitudinal trends of CVAI were evaluated, and the moderate, increasing, and persistent-high patterns carried higher risk of developing renal damage. The association between CVAI and renal damage was partly mediated by BP and glucose. Among several commonly used indicators of measuring adiposity, CVAI was the most discriminating/predicting for renal damage. These findings, from cross-sectional to longitudinal changes, advance our understanding of the complex association of visceral fat and its changes with kidney disease, highlighting the importance of maintaining a low CVAI.
Although it has been demonstrated that visceral fat accumulation is associated with organ injury and metabolic disease, such as hypertension and diabetes (Citation13,Citation25,Citation26), most previous studies only used a cross-sectional design, and the association between CVAI and renal damage has been rarely reported. Recently, two studies evaluated the association in specific populations with high risk of kidney disease. Wan et al. reported in a cross-sectional study with only diabetic patients and concluded that CVAI was positively associated with prevalence of diabetic kidney disease in both men and women (Citation27). Similar results were observed in a longitudinal study, which was conducted in patients with coexistent hypertension and abnormal glucose metabolism (Citation28). Our study confirms and extends the association between visceral fat accumulation and renal damage into the general population, with homogeneity being observed in subgroup analyses. Moreover, significant association was found after adjustment for BMI, indicating that the adverse impacts of visceral fat may be independent of general obesity. This was supported by the subgroup analysis conducted in those with BMI <24 kg/m2, which showed significant results in both cross-sectional and longitudinal data. It is interesting that the shape of the association was quite similar to that in a previous study (Citation28), with both showing a potential saturation effect between CVAI and the risk of renal damage.
Obesity is a dynamic condition (Citation29), and changing status has different effects on kidney disease. Studies have reported that large increases in weight and WC are associated with higher risk of CKD in patients with diabetes (Citation30), while short-term weight loss could decrease CKD risk in patients with nonalcoholic fatty liver disease (Citation31). It is known that the degree of fat accumulation can be controlled or even reduced by increasing exercise and maintaining a healthy diet (Citation32). To our knowledge, the present study is the first to evaluate the impact of longitudinal trends of visceral fat accumulation (measured by the CVAI) on the risk of renal damage. Among the five change patterns, the low-stable pattern carries the lowest risk for developing renal damage, and the moderate, increasing, and persistent-high patterns had higher risk of renal damage. Notably, results from the both cohorts showed that the decreasing pattern had a comparable low risk of renal damage as the low-stable group, although more than 50% of the individuals in the decreasing group were in the third-to-fourth quartiles of CVAI at baseline. These results suggest that potential benefits for the kidney may be obtained from lowering the CVAI for individuals with increased levels.
Both cross-sectional and longitudinal analyses revealed that CVAI is the optimal indicator for discriminating/predicting renal damage as compared with traditional (BMI, WC and WHtR) and other novel indices (LAP, BRI and VAI). Similar results were reported in patients with hypertension and abnormal glucose metabolism (Citation28). These results were supported by the insight that visceral adiposity has a greater impact on organ damage than general or abdominal obesity (Citation33). It also reconfirms that CVAI is more suitable for the Chinese population than VAI, not only for predicting diabetes, but also for CKD.
The links between adiposity and kidney disease are bidirectional, multi-layered and complex, and overlapping pathophysiological pathways and shared risk factors may serve as parts of the explanation. Although it remains to be further explored, several potential mechanisms may be involved in the process of renal damage induced by visceral fat. In this study, we found that BP and glucose have a mediating effect between the CVAI and renal damage. Increased BP and glucose and insulin resistance induce cytokine imbalances in the kidney, including decreased endothelial nitric oxide and increased production of endothelin-1 and growth factors, which eventually trigger a series of intra-renal reaction, resulting in impaired endothelial function, increased oxidative stress, and pro-inflammatory and pro-fibrotic changes (Citation7,Citation34). Notably, slight mediating effects were observed in BP and glucose, suggesting that other pathways are involved. An elevation of CVAI is indicative of either a gain in total body fat or body fat redistribution, resulting in increased availability and ectopic tissue accumulation of free fatty acids (Citation35). Renal lipotoxicity causes damage to glomerular basement membranes and podocytes, leading to increased membrane permeability and loss of lipoprotein lipase activators and albumin (Citation36,Citation37), and eventually the manifestation of renal damage. In addition, chronic systematic inflammation, activation of the local adipose tissue rennin-angiotensin-aldosterone-system, and comorbidities (such as hypertension, diabetes, and sleep-disordered breathing) may be involved in the complex process (Citation38–41).
Several limitations warrant discussion. First, although the definition of renal damage is the same as in most previous studies, it is not identical to the standard definition of CKD. Repeated measurements of eGFR and urine albumin are necessary in future studies. Second, residual and unmeasured confounding could not be completely ruled out, although a wide range of confounders has been considered. In addition, Mendelian randomization and interventional studies are required to verify a causal relationship. Third, sample size in the longitudinal analysis of the Shantou population was significantly smaller than that of the cross-sectional analysis. However, analysis of the CHARLS data confirmed the longitudinal results, and pooled analysis could improve the statistical power. Fourth, longitudinal trends of the CVAI were identified based on only two measurements. Multiple measurements could help to understand its trajectory more accurately.
In conclusion, a higher level of baseline CVAI and longitudinal increasing and persistent-high pattern of the CVAI were independently associated with increased risk of renal damage. Among several commonly used indicators of measuring obesity, CVAI was the optimal for discriminating/predicting renal damage. Monitoring the CVAI and maintaining a low level may contribute to the prevention of renal damage.
Author contributions
Conceptualization, MYL, YQC and XRT; Methodology, MYL and YQC; Software, MYL and SWW; Validation, XLD; Formal analysis, MYL; Investigation, MYL, SWW and XLD; Data Curation, YQC and XRT; Writing-Original Draft Preparation, MYL; Writing-Review & Editing, MYL, SWW, XLD, YQC and XRT; Visualization, MYL; Funding Acquisition, YQC and XRT.
Supplemental Material
Download MS Word (246.7 KB)Disclosure statement
No potential conflict of interest was reported by the author(s).
Supplementary material
Supplemental data for this article can be accessed online at https://doi.org/10.1080/10641963.2023.2271187
Data availability statement
Data of the China Health and Retirement Longitudinal Study are available at its website (http://charls.pku.edu.cn/). Original data of the Shantou cohort are available from the corresponding authors upon reasonable request.
Additional information
Funding
References
- Herzog CA, Asinger RW, Berger AK, Charytan DM, Díez J, Hart RG, Eckardt K-U, Kasiske BL, McCullough PA, Passman RS, et al. Cardiovascular disease in chronic kidney disease. A clinical update from kidney disease: improving Global outcomes (KDIGO). Kidney Int. 2011;80(6):572–8. doi:10.1038/ki.2011.223.
- Gansevoort RT, Correa-Rotter R, Hemmelgarn BR, Jafar TH, Heerspink HJL, Mann JF, Matsushita K, Wen CP. Chronic kidney disease and cardiovascular risk: epidemiology, mechanisms, and prevention. Lancet. 2013;382(9889):339–52. doi:10.1016/S0140-6736(13)60595-4.
- Hill NR, Fatoba ST, Oke JL, Hirst JA, O’Callaghan CA, Lasserson DS, Hobbs FDR. Global prevalence of chronic kidney disease – a systematic review and meta-analysis. PloS One. 2016;11(7):e0158765. doi:10.1371/journal.pone.0158765.
- Vos T, Abajobir AA, Abate KH, Abbafati C, Abbas KM, Abd-Allah F, Abdulkader RS, Abdulle AM, Abebo TA, Abera SF, et al. Global, regional, and national incidence, prevalence, and years lived with disability for 328 diseases and injuries for 195 countries, 1990–2016: a systematic analysis for the Global burden of disease study 2016. Lancet. 2017;390(10100):1211–59. doi:10.1016/S0140-6736(17)32154-2.
- Zhang L, Wang F, Wang L, Wang W, Liu B, Liu J, Chen M, He Q, Liao Y, Yu X, et al. Prevalence of chronic kidney disease in China: a cross-sectional surve. Lancet. 2012;379(9818):815–22. doi:10.1016/S0140-6736(12)60033-6.
- Lv JC, Zhang LX. Prevalence and disease burden of chronic kidney disease. Adv Exp Med Biol. 2019;1165:3–15.
- Lakkis JI, Weir MR. Obesity and kidney disease. Prog Cardiovasc Dis. 2018;61(2):157–67. doi:10.1016/j.pcad.2018.07.005.
- Noori N, Hosseinpanah F, Nasiri AA, Azizi F. Comparison of overall obesity and abdominal adiposity in predicting chronic kidney disease incidence among adults. J Ren Nutr. 2009;19(3):228–37. doi:10.1053/j.jrn.2008.11.005.
- Koenen M, Hill MA, Cohen P, Sowers JR. Obesity, adipose tissue and vascular dysfunction. Circ Res. 2021;128(7):951–68. doi:10.1161/CIRCRESAHA.121.318093.
- González N, Moreno-Villegas Z, González-Bris A, Egido J, Lorenzo Ó. Regulation of visceral and epicardial adipose tissue for preventing cardiovascular injuries associated to obesity and diabetes. Cardiovasc Diabetol. 2017;16(1):44. doi:10.1186/s12933-017-0528-4.
- Xia MF, Chen Y, Lin HD, Ma H, Li X-M, Aleteng Q, Li Q, Wang D, Hu Y, Pan B-S, et al. A indicator of visceral adipose dysfunction to evaluate metabolic health in adult Chinese. Sci Rep. 2016;6(1):38214. doi:10.1038/srep38214.
- Bi H, Zhang Y, Qin P, Wang C, Peng X, Chen H, Zhao D, Xu S, Wang L, Zhao P, et al. Association of Chinese visceral adiposity index and its dynamic change with risk of carotid plaque in a large cohort in China. J Am Heart Assoc. 2022;11(1):e022633. doi:10.1161/JAHA.121.022633.
- Li B, Wang J, Zhou X, Liu Y, Wang W, Gao Z, Tang X, Yan L, Wan Q, Luo Z, et al. Chinese visceral adiposity index is more closely associated with hypertension and prehypertension than traditional adiposity indices in Chinese population: results from the REACTION study. Front Endocrinol (Lausanne). 2022;13:921997.
- Huang YC, Huang JC, Lin CI, Chien H-H, Lin Y-Y, Wang C-L, Liang F-W, Dai C-Y, Chuang H-Y. Comparison of innovative and traditional cardiometabolic indices in estimating atherosclerotic cardiovascular disease risk in adults. Diagnostics (Basel). 2021;11(4):603. doi:10.3390/diagnostics11040603.
- Seong JM, Lee JH, Gi MY, Son YH, Moon AE, Park CE, Sung HH, Yoon H. Gender difference in the association of chronic kidney disease with visceral adiposity index and lipid accumulation product index in Korean adults: Korean National Health and Nutrition Examination Survey. Int Urol Nephrol. 2021;53(7):1417–25. doi:10.1007/s11255-020-02735-0.
- Xiao H, Xiong C, Shao X, Gao P, Chen H, Ning J, Chen Y, Zou Z, Hong G, Li X, et al. Visceral adiposity index and chronic kidney disease in a non-diabetic population: a cross-sectional study. Diabetes Metab Syndr Obes. 2020Vol. 13pp. 257–65 p.
- Chen YC, Lai SH, Tsai YW, Chang S-S. Visceral adiposity index as a predictor of chronic kidney disease in a relatively healthy population in Taiwan. J Ren Nutr. 2018;28(2):91–100. doi:10.1053/j.jrn.2017.07.006.
- Yuan Y, Liu K, Zheng M, Chen S, Wang H, Jiang Q, Xiao Y, Zhou L, Liu X, Yu Y, et al. Analysis of changes in weight, waist circumference, or both, and all-cause mortality in Chinese adults. JAMA Netw Open. 2022;5(8):e2225876. doi:10.1001/jamanetworkopen.2022.25876.
- Huang YY, Jiang CQ, Xu L, Zhang WS, Zhu F, Jin YL, Thomas GN, Cheng KK, Lam TH. Adiposity change and mortality in middle-aged to older Chinese: an 8-year follow-up of the Guangzhou biobank cohort study. BMJ Open. 2020;10(12):e039239. doi:10.1136/bmjopen-2020-039239.
- Chen C, Ye Y, Zhang Y, Pan X-F, Pan A. Weight change across adulthood in relation to all cause and cause specific mortality: prospective cohort study. Bmj. 2019;367:l5584. doi:10.1136/bmj.l5584.
- Zhao Y, Hu Y, Smith JP, Strauss J, Yang G. Cohort profile: the China health and Retirement longitudinal study (CHARLS). Int J Epidemiol. 2014;43(1):61–68. doi:10.1093/ije/dys203.
- Ma YC, Zuo L, Chen JH, Luo Q, Yu X-Q, Li Y, Xu J-S, Huang S-M, Wang L-N, Huang W, et al. Modified glomerular filtration rate estimating equation for Chinese patients with chronic kidney disease. J Am Soc Nephrol. 2006;17(10):2937–44. doi:10.1681/ASN.2006040368.
- Kahn HS. The lipid accumulation product is better than BMI for identifying diabetes: a population-based comparison. Diab Care. 2006;29(1):151–53. doi:10.2337/diacare.29.01.06.dc05-1805.
- Chang Y, Guo X, Li T, Li S, Guo J, Sun Y. A body shape index and body Roundness index: two New body indices to identify left ventricular hypertrophy among rural populations in Northeast China. Heart Lung Circ. 2016;25(4):358–64. doi:10.1016/j.hlc.2015.08.009.
- Xia MF, Lin HD, Chen LY, Wu L, Ma H, Li Q, Aleteng Q, Chen Y, Sun Y-X, Hu Y, et al. Association of visceral adiposity and its longitudinal increase with the risk of diabetes in Chinese adults: a prospective cohort study. Diabetes Metab Res Rev. 2018;34(7):e3048. doi:10.1002/dmrr.3048.
- Wei J, Liu X, Xue H, Wang Y, Shi Z. Comparisons of visceral adiposity index, body shape index, body Mass index and waist circumference and their associations with diabetes mellitus in adults. Nutrients. 2019;11(7):1580. doi:10.3390/nu11071580.
- Wan H, Wang Y, Xiang Q, Fang S, Chen Y, Chen C, Zhang W, Zhang H, Xia F, Wang N, et al. Associations between abdominal obesity indices and diabetic complications: Chinese visceral adiposity index and neck circumference. Cardiovasc Diabetol. 2020;19(1):118. doi:10.1186/s12933-020-01095-4.
- Lin M, Li N, Heizhati M, Gan L, Zhu Q, Yao L, Li M, Yang W. Chinese visceral adiposity index is associated with incident renal damage in patients with hypertension and abnormal glucose metabolism: a longitudinal study. Front Endocrinol (Lausanne). 2022;13:910329. doi:10.3389/fendo.2022.910329.
- Vlassopoulos A, Combet E, Lean ME. Changing distributions of body size and adiposity with age. Int J Obes (Lond). 2014;38(6):857–64. doi:10.1038/ijo.2013.216.
- Chung HF, Al Mamun A, Huang MC, Long KZ, Huang Y-F, Shin S-J, Hwang S-J, Hsu C-C. Obesity, weight change, and chronic kidney disease in patients with type 2 diabetes mellitus: a longitudinal study in Taiwan. J Diabetes. 2017;9(11):983–93. doi:10.1111/1753-0407.12514.
- Hu S, Li X, Sun Y, Wu S, Lan Y, Chen S, Wang Y, Liao W, Wang X, Zhang D, et al. Short-term weight loss decreased the risk of chronic kidney disease in men with incident nonalcoholic fatty liver disease. Obesity (Silver Spring). 2022;30(7):1495–506. doi:10.1002/oby.23459.
- Baria F, Kamimura MA, Aoike DT, Ammirati A, Leister Rocha M, de Mello MT, Cuppari L. Randomized controlled trial to evaluate the impact of aerobic exercise on visceral fat in overweight chronic kidney disease patients. Nephrol Dial Transplant. 2014;29(4):857–64. doi:10.1093/ndt/gft529.
- Okamura T, Hashimoto Y, Hamaguchi M, Obora A, Kojima T, Fukui M. Ectopic fat obesity presents the greatest risk for incident type 2 diabetes: a population-based longitudinal study. Int J Obes (Lond). 2019;43(1):139–48. doi:10.1038/s41366-018-0076-3.
- Sarafidis PA, Ruilope LM. Insulin resistance, hyperinsulinemia, and renal injury: mechanisms and implications. Am J Nephrol. 2006;26(3):232–44. doi:10.1159/000093632.
- Izquierdo-Lahuerta A, Martínez-García C, Medina-Gómez G. Lipotoxicity as a trigger factor of renal disease. J Nephrol. 2016;29(5):603–10. doi:10.1007/s40620-016-0278-5.
- Joles JA, Kunter U, Janssen U, KRIZ W, RABELINK TJ, KOOMANS HA, FLOEGE J. Early mechanisms of renal injury in hypercholesterolemic or hypertriglyceridemic rats. J Am Soc Nephrol. 2000;11(4):669–83. doi:10.1681/ASN.V114669.
- Ruan XZ, Varghese Z, Moorhead JF. An update on the lipid nephrotoxicity hypothesis. Nat Rev Nephrol. 2009;5(12):713–21. doi:10.1038/nrneph.2009.184.
- Kawai T, Autieri MV, Scalia R. Adipose tissue inflammation and metabolic dysfunction in obesity. Am J Physiol Cell Physiol. 2021;320(3):C375–c391. doi:10.1152/ajpcell.00379.2020.
- Sakaguchi Y, Shoji T, Kawabata H, Niihata K, Suzuki A, Kaneko T, Okada N, Isaka Y, Rakugi H, Tsubakihara Y, et al. High prevalence of obstructive sleep apnea and its association with renal function among nondialysis chronic kidney disease patients in Japan: a cross-sectional study. Clin J Am Soc Nephrol. 2011;6(5):995–1000. doi:10.2215/CJN.08670910.
- Dinh Cat AN, Friederich-Persson M, White A, Touyz RM. Adipocytes, aldosterone and obesity-related hypertension. J Mol Endocrinol. 2016;57(1):F7–f21. doi:10.1530/JME-16-0025.
- Goossens GH, Blaak EE, van Baak MA. Possible involvement of the adipose tissue renin-angiotensin system in the pathophysiology of obesity and obesity-related disorders. Obes Rev. 2003;4(1):43–55. doi:10.1046/j.1467-789X.2003.00091.x.