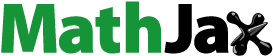
ABSTRACT
The success of graduates and their employability hinge on a crucial alignment between the skills and competencies sought by employers and those possessed by job seekers. This study delves into the extent to which the competencies cultivated in the curricula of selected Slovenian universities align with the ever-evolving requirements of the Slovenian labour market. Employing a combination of LLaMA-2-7B-chat, a generative language model, and the multilingual Sentence Transformer, a contextual language model, we identify skill gaps and pinpoint the most in-demand competencies that align with those generated by the faculty. This data-driven approach offers a roadmap for confirming the relevance and enhancing the university curricula, thus fostering a stronger connection between education and employment as well as improving the labour market efficiency.
1. Introduction
In the contemporary landscape of higher education and labour market dynamics, the imperative to continuously align graduate skills structure with the evolving demands of the labour market has become increasingly relevant (J. Cleary et al., Citation2014; J. L. Cleary et al., Citation2017). The rapid pace of technological advancements, coupled with shifts in global economic paradigms, necessitates a keen understanding of the competencies that students acquire during their academic pursuits and how these align with the skills sought by employers (Frank et al., Citation2019; Małkowska et al., Citation2021).
This research endeavours to bridge the gap between academic curricula and industry needs, employing advanced language models to extract and analyse competencies. As current and future business leaders play a pivotal role in stimulating, planning and leading the transition processes, the characteristics of their skills will play a pivotal role in the success of such a transition. Consequently, the paper focuses on this subsegment of the labour market.
Due to the availability and nature of the data, we focus on Slovenian labour market and business degrees in Slovenia. Slovenia, a prominent educational institution, recognised for its triple international accreditation (EQUIS (EFMD Global, Citation2024), AACSB (AACSB International, Citation2024), AMBA (AMBA, Citation2024)) and acknowledged by FT rankings, stands as the largest tertiary-level establishment in the country. With an extensive track record of granting business and economics degrees, this institution, akin to its numerous global educational counterparts, holds a crucial role in shaping the skillsets of aspiring professionals.
The following are the research goals of the paper:
Develop a methodological tool, which can be more widely used to support policy-making in the field of education and labour market, but allowing a more efficient bridging of the competence gap using timely identification of the main discrepancies,
Study the content of curricula and job advertisements to distil the main skills and competences, either provided or desired in the labour market in the field of business professionals,
Determine the gap in the desired skills and competences between the supply and demand side;
Study the gap between the typical esco occupational skills and competences and those either provided or desired by the supply or demand.
Methodologically, we leverage the power of a generative language model to extract competencies from both the undergraduate and graduate business and economics courses curricula of one of the largest tertiary educational institutions in Slovenia, and job adverts (openings) in Slovenia. The emergence of open-source language models, such as LLaMA (Touvron, Lavril, et al., Citation2023), has provided unprecedented capabilities in natural language processing. Furthermore, contextual language model (Devlin et al., Citation2018) is utilised to generate embeddings in a vector space, which allows identification of the most analogous ESCO (Commission & Directorate-General for Employment, S. A., and Inclusion, Citation2017) competencies, and thus establishing a robust framework for analysing the alignment between academic outputs and industry requirements.
The paper makes several contributions which we show that when used lead to results that may serve as a support in the practical policy decision-making. First, we use state-of-the-art language models and apply them to labour market analysis, thus leveraging the open-source language models to identify competency gaps and align academic offerings with industry demands on the Slovenian market. This methodological advancement paves the way for more effective and data-driven policy decisions in Slovenia. We used both demand (market job postings) and supply (academia curricula) datasets that have not been previously used for Slovenia to exhaustedly observe the current labour market for business and economy.
Second, the research provides valuable insights for strategic decision-making in both academia and industry in Slovenia. By pinpointing the most in-demand skills and identifying areas of misalignment between academic programmes and labour market requirements, the study is a guidance for tailoring educational offerings and enhancing workforce preparedness.
The following sections delve into the existing literature, describe the data and methodology employed, present the key findings, and conclude with a discussion of the implications of the research and potential avenues for future exploration.
2. Related work
The literature has extensively examined the relationships between educational attainment, over- and under-education, and field of education in relation to job search and employment quality (Bauer, Citation2002; Hartog, Citation2000). However, studies have often relied on the broad category of field of education as an indicator of job fit, which may be an insufficient measure of the alignment between a job candidate’s skills and competencies and the specific requirements of an occupation. Employability and career development crucially depend on the match between employers’ need and candidates’ skills. Besides improving career perspectives, the match also increases individuals’ productivity, as well as motivation, and positively impacts their wages while contributing also to firm and national competitiveness and performance (Mocanu et al., Citation2014). While the idea is not novel, methodologically, previous analyses relied primarily on the qualitative data or registry-based datasets, which either lack detail or are depending on specific, usually quite aggregated data, as for example the number of years, field of education or specific segment of educational programme. Robst (Citation2007), for example, highlighted the need to investigate the match in more detail by focusing on the relationship between college majors and occupations. Prior research, such as (Mardis et al., Citation2018) and (Alanazi & Benlaria, Citation2023), also highlighted the challenges and opportunities associated with aligning academic programmes with the dynamic demands of the labour market. The approach varied from qualitative analysis to survey-based approach (Anastasiu et al., Citation2017; Terblanche, Citation2011). More novel approaches already investigate the employers’ needs using data mining approaches, including textual analysis (Maer Matei & Aldea, Citation2019). Studies also explored how training approaches impact skills development and structure (Lee et al., Citation2019) employed a survey-based approach in medical training.
Utilising advanced language models for competence analysis has become increasingly prevalent in recent research. Wyskwarski (Citation2021) used relatively rudimentary text mining to show how job advertisements can help identify the most desired project manager competencies. He also stressed the relevance of such analysis for understanding labour market needs. Debortoli et al. (Citation2014) used latent semantic analysis to develop competency taxonomy for big data and business intelligence, guiding individuals, organisations, and academic institutions in assessing and advancing their skills. Schedlbauer et al. (Citation2021) used text-mining of job advertisements to categorise expected skills for a standardised medical informatics as a base for identifying learning goals.
Recently, the fast development of statistical techniques, relying on artificial intelligence and also large language models, opened a number of new research opportunities. (Zhang et al. (Citation2022) develop a skills extraction algorithm, which identifies and evaluates educational competencies, revealing that students have diverse skills, but these do not necessarily always align with industry needs. F¨oll & Thiesse (Citation2021) employed text-mining to study the content of the curricula in informatics in Germany. Other authors, such as (Bommarito et al., Citation2018), demonstrated the effectiveness of language models in extracting nuanced information from academic datasets. This methodology closely aligns with our approach, where we employ a language model to extract competencies from study plans.
The exploration of decision support systems in academic planning, as explained by (Tao et al., Citation2022), closely aligns with the objectives of our research. This work underscores the potential of data-driven insights to inform strategic decision-making within educational institutions. This resonates with our approach, where we aim to leverage empirical data to provide insights into adapting academic offerings and curricula to align more effectively with the evolving needs of the industry.
The effective utilisation of job postings for labour market analysis has been demonstrated (Mytna Kurekova et al., Citation2014; Wyskwarski, Citation2021). This research parallels our approach, wherein we scrape and analyse job postings from Slovenian job portals to gain real-time insights into the competencies actively sought by employers, i.e. exploring the skills-level characteristics of the demand side of the market. In addition, the skills supply is based on the analysis of the curricula of the business and economic programmes at a selected tertiary-level business and economics school in Slovenia. By grounding our analysis in the actual demands articulated by employers in the labour market and identifying the mismatch and the skills gap, we aim to utilise the novel research methods to provide information for data-based decision-making and curricula change, thereby enhancing the relevance and responsiveness of academic programmes to industry needs.
Our research extends the existing discourse by specifically addressing the alignment between competencies in the field of business education, specifically generated by the School of Economics and Business in Slovenia. The demand side represents the competencies sought by employers in the Slovenian labour market. While the findings are immediately relevant for Slovenia and its business education, the fact that this is a triple accredited school and Slovenian labour market is relatively typical in terms of structure of demand (industry) to many other small emerging open economies, findings also carry broader relevance. In addition, the integration of language models and the comparative analysis with the esco framework contribute to the ongoing research on decision support in academic planning and the dynamic relationship between academia and industry.
3. Data collection and methodological framework for competency gap identification
3.1. Data
To establish the empirical foundation for our study, we employ a dual-pronged data acquisition strategy that encompasses a leading Slovenian job portal (mojedelo.com) and the course curricula of School of Economics and Business, University of Ljubljana. All of the data used for the subsequent analysis is publicly available.
Table 1. Dataset description.
Description of the datasets is provided in . A total of 514 syllabi were acquired, covering both undergraduate and graduate courses spanning a diverse array of study programmes from the field of business and economics. The aim is to assemble a representative dataset that captures the multifaceted competencies embedded in academic curricula, ensuring a thorough understanding across a spectrum of courses and academic levels.
Concurrently, we curated job postings from a leading Slovenian job portal in the period of March 2023–December 2023, focusing specifically on those with detailed descriptions exceeding 250 characters. Our strategy involved extracting competencies explicitly mentioned in job advertisements across various sectors, industries, and experience levels. To refine our dataset, we collected 9337 job postings related to specific isco occupations associated with the educational fields of economics and business. isco is a global standard for classifying occupations based on job tasks, while esco is a European framework that categorises occupations, skills, competences, and qualifications to align workforce and educational needs within the EU. esco ontology assigns so-called isco level 6 codes for each esco occupation. As a result, there is a bijective mapping between isco and esco. Since isco level 4 codes are currently more prevalent in the job postings, we use isco codes only to gather the target job postings set. The description of the isco codes is provided in .
Table 2. Description of the ISCO codes associated with the educational fields of economics and business.
This ensures that our dataset reflects the dynamic and nuanced requirements of the labour market, providing real-time insights into industry demands related to the field of Economics and Business.
3.2. Methodology
Upon data collection, we initiate a fully automated preprocessing phase using a custom Python pipeline to refine the obtained datasets for subsequent analyses. This phase includes extracting competence descriptions from job adverts and academic curricula using Python scripts that parse text data and identify relevant sections through regular expressions or keyword-based filtering.
First, we normalise the descriptions of the esco competencies using Unicode standard normalisation (NFKD), followed by the removal of non-ASCII characters to standardise textual data. Next, we filter out empty entries and eliminate duplicates. The competencies are then extracted from the academic curricula and job adverts, transformed to lowercase and stripped of newline characters. Finally, these competencies are mapped to the esco framework to categorise them according to established esco labels and descriptions, aligning the extracted data with recognised standards and enhancing the validity of our analyses.
These pristine datasets lay the groundwork for applying language models and conducting further research. The workflow of the methodology given in summarises the essential idea of the paper. The methodology employs LLaMA (Touvron, Martin, et al., Citation2023) to explore the gap in the demands of the labour market (approximated by the data from job adverts) and the skills and competences highlighted by the curricula of the analysed courses.Footnote1 The text analysis highlights the gaps (). In continuing, the approach and the data are described in more detail.
Competence extraction from the acquired datasets is facilitated by the advanced LLaMA language model, specifically, the LLaMA-2-7B-Chat model from the LLaMA-2 family (Touvron, Martin, et al., Citation2023). This model, which consists of 7 billion parameters, is fine-tuned to function as an assistant, providing enhanced language understanding capabilities. For our analysis, we use the specific prompt: ‘Which ESCO competences can be extracted from this text? List ESCO competencies with a very short description for each competency’. This prompt directs the model to identify and list relevant esco competencies from the text data contained in the job adverts and academic curricula. The model consists of 7 billion parameters and is fine-tuned as an assistant, providing enhanced language understanding capabilities. In our iterative application of LLaMA to both academic study plans and job postings, we employ the following hyperparameters:
seed value of 44
temperature setting of 0.8
context prompt size of 1024 tokens.
The temperature setting of the model determines the level of randomness in the generated text (values ranging from 0 to 1), with higher values leading to more diverse outputs. A temperature of 0.8 balances novelty and coherence, while the context prompt size of 1024 tokens influences the amount of information provided to the model for generating responses, impacting both comprehensiveness and specificity. The consistent use of the model across both sources ensures a unified and thorough approach to competence extraction, fostering a holistic understanding of competencies generated by academic institutions and those sought by employers.
After extracting competencies from both academic curricula and job postings, we standardise them using Sentence-BERT (SBERT) (Reimers & Gurevych, Citation2019), a modification of the pre-trained BERT network that uses siamese and triplet network structures to derive semantically meaningful sentence embeddings. This model efficiently maps sentences to a 512-dimensional vector space, enabling us to compare the semantic similarity of competencies at scale. To integrate and align these competencies with the esco framework, the SBERT embeddings of extracted competencies are compared against embeddings of all competencies listed in the esco database. We compute the cosine similarity between each extracted competency’s embedding and the embeddings of esco competencies. This similarity measure helps us to identify which esco competencies are most semantically similar to the ones identified in our datasets. We then rank these esco competencies based on their cosine similarity scores and select the top 10 for each extracted competency. This not only ensures a consistent alignment between our data-driven competencies from academic and job-related sources with the standardised esco competencies but also enhances the granularity of our analysis by focusing on the most relevant esco competencies. This methodology allows for a detailed and nuanced comparison across different sources and categories, aiding in a comprehensive competency gap analysis which is crucial for tailoring educational programmes to meet job market demands more effectively.
The cosine similarity is given by the following expression:
where A and B represent the vectors corresponding to the competencies being compared. The dot product of A and B is divided by the product of their Euclidean norms, resulting in a cosine similarity score. This score quantifies the directional similarity between the two vectors, with values ranging from −1 (perfect dissimilarity) to 1 (perfect similarity). In our case, this metric plays a crucial role in determining the 10 most analogous esco competencies for each competency identified within academic sources or job adverts. The vectors, or embeddings, are representations generated by the SBERT model, providing a nuanced measure of similarity that aids in the alignment of competencies in the analysis.
4. Results and discussion
The goal of the analysis was to describe the gap between the supply of skills and competences (academia) and the demand (employers). It should be noted that esco ‘Skill and competences’ pillar distinguishes four subsets: skills, traversal skills and competences, knowledges and languages. The analysis in this section distinguishes ‘knowledges’, since this subset represents more generalised concepts that are most commonly provided in the syllabi.
summarises the most common competencies that are both desired by the employers and provided in the curricula. This highlights a good overlap between the supply and demand sides. These competencies encompass primarily the following: management skills, analytical/thinking skills and competences, communication, collaboration and creativity skills, problem solving skills, project management skills and similar. These are also skills that are quite generally required for work in business or economics, therefore, the results are not surprising.
Figure 2. Most common competencies desired by the employers: provided and not provided by the academic curricula. Competences marked with * represent esco knowledges.

Around 30% of the job advertisements require at least one skill/competency that is among the 20 most common competencies not provided by the academic curricula (). Some of the listed skills/competencies that curricula do not encompass are shown in . It should be noted that the majority of the esco skills extracted from the syllabi belong to the group knowledges, whereas the missing esco skills in the job adverts are very specific. Typical examples for industry-specific skills are coordinate assembling room in footwear manufacturing, etc., and there are too broad such as simplify communication.
Since curricula naturally focus on knowledge segment of the esco skills, it is expected that such a mismatch exists. The fact that job adverts list among skills/competencies also such task-level activities could also demonstrate the lack of understanding on the side of employers the distinction between skill and esco knowledge. In addition, the mismatch could also indicate that not all skills and competencies are mentioned in the curricula or that the granularity difference is significant.
Additionally, depicts that 0.7% of job adverts for job openings directly linked to business/economics professionals do not mention even one of the competencies, which are mentioned by educational institutions’ curricula. These adverts either misspecified the profile they need or do not know, what the specific skills of economics/business professionals are. Considering the increasing role of technology in labour market matching, such examples indicate the problems of misspecification of professional’s profile for future match.
Regional dispersion of the mismatches () highlights that overall, the problem of mismatch is more specific in some regions, either those, industrially strong, where consequently there is also large demand, and in regions which are more characterised by daily migration of workers to other regions, leaving the local labour market with more (specific) job vacancies. Detailed analysis of the daily job migration patterns can be found in Tomić (Citation2022). A practical implication of this result points to the potential for local educational institutions and employment services to develop life-long learning programmes that could bridge such gaps, where possible and find consequently available candidates in the local labour markets.
Figure 4. Distribution of job postings among the Slovenian regions. The dots represent the job postings and the colours are with respect to .

Labour market efficiency, in particular job matching, as well as active labour market policies and educational policies (curricular changes) could be made significantly more efficient if the employers and educational institutions used the same terminology, implying that they would rely on the well-developed esco competence framework. esco identifies and categorises skills, competences, qualifications and described each of the isco occupations with relevant skills and competences. In view of increasing fluidity and interdisciplinary between occupations, esco approach could be extremely valuable in promoting job matching.
Implications, limitations and future research guidelines The analysis applies advanced large language models to develop a tool to support the efficiency of the labour market matching process. The results highlight that there is in fact a strong match between the skills and competences provided and those desired by the employers. Importantly, the results also show that the identified mismatch is partially based on the ‘human’ factor due to the lack of understanding of what educational competencies and what specific job tasks or firm-level business processes are. To avoid this mismatch, both educational institutions as well as employers could rely on 2-level approach to job descriptions. The first could be a list of competencies based on esco classifications, but not necessarily just of 1 occupation due to increasingly interdisciplinary and fluid jobs. The second level could be ‘job specific tasks’, which would highlight those tasks that are very specific just for the selected job offering and are not widely typical for a specific profile. Another implication is methodological. Given that the specifics of how both agents in the labour market describe their skills and competencies will take time to change, gap analysis could be widened to include also very general descriptions of specific programmes. These typically mention industries, where individuals work (e.g. finance) or list also some generally known skills.
The analysis provides an important insight into how advanced LlaMA tools (open source, adapted to specific needs of an analytical problem) can be used to support more efficient decision-making. The processing pipeline could also be extended to other fields of education to facilitate the development of a search tool, which could identify suitable candidates, needed in more interdisciplinary fields.
The findings reveal a significant alignment between the skills and competencies provided by educational institutions and those sought after by employers. Notably, the identified skill gaps are partially attributable to a ‘human factor’ arising from a lack of comprehension regarding educational competencies and specific job tasks or firm-level business processes.
The proposed methodology can be employed as a feedback loop for decision-making regarding appropriate syllabus updates. By regularly analysing the alignment between educational curricula and employer requirements, educational institutions can identify areas for improvement and make timely adjustments to their academic programmes. This dynamic approach ensures that students acquire the skills and competencies most relevant to the evolving demands of the labour market.
5. Conclusion
Our analysis has brought to light discernible disparities between the competencies emphasised in academic study plans and those explicitly sought by employers in job postings. These findings underscore potential gaps in the preparation of graduates for the ever-evolving landscape of the labour market. The identification of these disparities opens avenues for targeted interventions and adjustments in academic curricula to better align with industry needs.
The application of language models, notably the LLaMA model for competence extraction and the SBERT model for alignment with ESCO competencies has proven to be a robust and effective approach in handling the intricacies of linguistic data. These models have facilitated the extraction and comparison of competencies, providing insights into the competence landscape.
While disparities were evident, our study also revealed instances of alignment and overlaps between academic competencies and job market requirements. These alignments offer valuable insights into areas where academic offerings meet or anticipate industry needs. This nuanced understanding of aligned competencies presents opportunities for academic institutions to bolster their relevance and responsiveness to the expectations of the labour market. The integration of the ESCO framework into our analysis has enhanced the comparability of competencies, providing a standardised reference point. The ESCO framework has proven to be instrumental in contextualising academic competencies in a standardised and widely recognised framework.
The research serves as a valuable contribution to the ongoing efforts on decision support in academic planning and the continual adaptation of educational offerings to meet the evolving needs of the workforce. The identified disparities, alignments, and the robustness of language models collectively contribute to a more nuanced understanding of the complex interplay between academic competencies and industry demands.
Acknowledgments
The authors acknowledge the research core funding No. P2-0001 and No. P2-0383 and research projects J5-4575 and V5-2267 financially supported by the Slovenian Research and Innovation Agency.
Disclosure statement
No potential conflict of interest was reported by the author(s).
Additional information
Funding
Notes
1. Both the ads as well as curricula typically higlight the most important skills/competences, not necessarily all.
References
- AACSB International. (2024). AACSB international - Advancing quality, global business education.
- Alanazi, A.S., & Benlaria, H. (2023). Bridging higher education outcomes and labour market needs: A study of jouf university graduates in the context of vision 2030. Social Sciences, 12(6), 360. https://doi.org/10.3390/socsci12060360
- AMBA. (2024). Association of MBAs (AMBA).
- Anastasiu, L., Anastasiu, A., Dumitran, M., Crizboi, C., Holmaghi, A., & Roman, M. (2017). How to align the university curricula with the market demands by developing employability skills in the civil engineering sector. Education Sciences, 7(3), 74. https://doi.org/10.3390/educsci7030074
- Bauer, T.K. (2002). Educational mismatch and wages: A panel analysis. Economics of Education Review, 21(3), 221–229. https://doi.org/10.1016/S0272-7757(01)00004-8
- Bommarito, M.J., Katz, D.M., & Detterman, E. (2018). Lexnlp: Natural language processing and information extraction for legal and regulatory texts. SSRN Electronic Journal. https://doi.org/10.2139/ssrn.3192101
- Cleary, J.L., Kerrigan, M.R., & Van Noy, M. (2017). Towards a new understanding of labor market alignment. Higher Education: Handbook of Theory and Research: Published Under the Sponsorship of the Association for Institutional Research (AIR) and the Association for the Study of Higher Education (ASHE), 32(1), 577–629. https://doi.org/10.1007/978-3-319-48983-4_12
- Cleary, J., & Van Noy, M. (2014). A framework for higher education labor market alignment: Lessons and future directions in the development of jobs–driven strategies. working paper. John J. Heldrich Center for Workforce Development.
- Commission, E., & Directorate-General for Employment, S. A. and Inclusion. (2017). ESCO handbook : European skills, competences, qualifications and occupations. Publications Office.
- Debortoli, S., Mu¨ller, O., & Brocke, J. (2014). Vergleich von kompetenzanforderungen an business-intelligence- und big-data-spezialisten: Eine text-mining-studie auf basis von stellenausschreibungen. WIRTSCHAFTSINFORMATIK, 56(5), 315–328. https://doi.org/10.1007/s11576-014-0432-4
- Devlin, J., Chang, M.-W., Lee, K., & Toutanova, K. (2018). Bert: Pre-training of deep bidirectional transformers for language understanding. arXiv preprint arXiv:1810.04805.
- EFMD Global. (2024). EQUIS - EFMD global.
- F¨oll, P., & Thiesse, F. (2021). Exploring information systems curricula: A text mining approach. Business & Information Systems Engineering, 63(6), 711–732. https://doi.org/10.1007/s12599-021-00702-2
- Frank, M.R., Autor, D., Bessen, J.E., Brynjolfsson, E., Cebrian, M., Deming, D.J., Feldman, M., Groh, M., Lobo, J., Moro, E., Wang, D., Youn, H., & Rahwan, I. (2019). Toward understanding the impact of artificial intelligence on labor. Proceedings of the National Academy of Sciences, 116(14), 6531–6539. https://doi.org/10.1073/pnas.1900949116
- Hartog, J. (2000). Over-education and earnings: Where are we, where should we go? Economics of Education Review, 19(2), 131–147. https://doi.org/10.1016/S0272-7757(99)00050-3
- Lee, H., Shimotakahara, R., Fukada, A., Shinbashi, S., & Ogata, S. (2019). Impact of differences in clinical training methods on generic skills development of nursing students: A text mining analysis study. Heliyon, 5(3), e01285. https://doi.org/10.1016/j.heliyon.2019.e01285
- Maer Matei, M.M., & Aldea, A.B. (2019). Employers’ requirements for data scientists - an analysis of job posts. Logos Universality Mentality Education Novelty: Economics & Amp; Administrative Sciences, 4(1), 21–32. https://doi.org/10.18662/lumeneas/10
- Małkowska, A., Urbaniec, M., & Kosal-A, M. (2021). The impact of digital transformation on European countries: Insights from a comparative analysis. Equilibrium Quarterly Journal of Economics and Economic Policy, 16(2), 325–355. https://doi.org/10.24136/eq.2021.012
- Mardis, M.A., Ma, J., Jones, F.R., Ambavarapu, C.R., Kelleher, H.M., Spears, L.I., & McClure, C.R. (2018). Assessing alignment between information technology educational opportunities, professional requirements, and industry demands. Education and Information Technologies, 23(4), 1547–1584. https://doi.org/10.1007/s10639-017-9678-y
- Mocanu, C., Zamfir, A.M., & Pirciog, S. (2014). Matching curricula with labour market needs for higher education: State of art, obstacles and facilitating factors. Procedia - Social & Behavioral Sciences, 149, 602–606. https://doi.org/10.1016/j.sbspro.2014.08.234
- Mytna Kurekova, L., Beblavy, M., & Thum, A.-E. (2014). Using internet data to analyse the labour market: A methodological enquiry. SSRN Electronic Journal. https://doi.org/10.2139/ssrn.2514748
- Reimers, N., & Gurevych, I. (2019). Sentence-Bert: Sentence embeddings using siamese Bert-networks. In Proceedings of the 2019 Conference on Empirical Methods in Natural Language Processing, Asia World Expo, Hong Kong. Association for Computational Linguistics.
- Robst, J. (2007). Education and job match: The relatedness of college major and work. Economics of Education Review, 26(4), 397–407. https://doi.org/10.1016/j.econedurev.2006.08.003
- Schedlbauer, J., Raptis, G., & Ludwig, B. (2021). Medical informatics labor market analysis using web crawling, web scraping, and text mining. International Journal of Medical Informatics, 150, 104453. https://doi.org/10.1016/j.ijmedinf.2021.104453
- Tao, M., Jiang, J., Wang, X., Zhou, J., Xie, J., & Kayvanfar, V. (2022). A decision support framework for curriculum planning in undergraduate supply chain management program: An integrated approach. Mathematical Problems in Engineering, 2022, 1–17. https://doi.org/10.1155/2022/3494431
- Terblanche, C. (2011). Meeting employers’ and students’ expectations through the use of employer demand ontology in curriculum development. In 2011 5th IEEE International Conference on E-Learning in Industrial Electronics (ICELIE), Melbourne, Australia. IEEE.
- Tomić, M. (2022). An Empirical Analysis of Daily Migration in Slovenia: Master’s thesis [ PhD thesis]. Univerza v Ljubljani, Ekonomska fakulteta.
- Touvron, H., Lavril, T., Izacard, G., Martinet, X., Lachaux, M.-A., Lacroix, T., Rozière, B., Goyal, N., Hambro, E., Azhar, F., & Rodriguez A. (2023). Llama: Open and efficient foundation language models. arXiv preprint arXiv:2302.13971.
- Touvron, H., Martin, L., Stone, K., Albert, P., Almahairi, A., Babaei, Y., Bashlykov, N., Batra, S., Bhargava, P., Bhosale, S., & Bikel D. (2023). Llama 2: Open foundation and fine-tuned chat models. https://arxiv.org/abs/2307.09288
- Wyskwarski, M. (2021). Requirements for project managers—what do job advertisements say? Sustainability, 13(23), 12999. https://doi.org/10.3390/su132312999
- Zhang, M., Jensen, K.N., Sonniks, S.D., & Plank, B. (2022). Skillspan: Hard and soft skill extraction from English job postings. arXiv preprint arXiv:2204.12811.