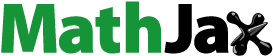
ABSTRACT
The adjusted headcount ratio from the multidimensional poverty measures has been used in cross-sectional research on the labour market to study the cumulative aspects of multiple job deprivations. This article takes advantage of the decomposition property of this method to develop a dynamic model for examining and understanding the transitions between precarious employment dimensions and index changes from a longitudinal perspective using panel data from Chile. Evidence produced by this method shows multidimensional precarious employment traps in women, while men suffer greater deprivations in the social protection dimension despite economic growth and the overall decrease in the multidimensional deprivation index. Both results would not be evident if only a cross-sectional analysis were used.
I. Introduction
In recent years, international agencies have recommended that economic policies should not only focus on creating more jobs but also on creating better jobs (e.g. OECD Citation2014). To further this debate, it is necessary to distinguish between a secure or ‘good job’ and a precarious or ‘bad job’, but we are far from reaching a global consensus on how jobs should be classified as one or the other (Piasna, Burchell, and Sehnbruch Citation2019). There is agreement in academic and political debates that poor quality employment is a multidimensional concept that comprises an accumulation of deprivations in multiple dimensions (Muñoz de Bustillo et al. Citation2011). Dedicated methodological approaches are therefore needed to address their measurement. Recent studies have proposed multidimensional measures of precarious employment (García-Pérez, Prieto-Alaiz, and Simón Citation2017) and job quality (Sehnbruch et al. Citation2020) based on the advances developed by the multidimensional poverty literature, in particular, the indices proposed by Alkire and Foster (Citation2011).
Until now, these multidimensional measures of employment quality have been applied mainly to repeated cross-sectional data to study differences and changes in the quality of employment as well as the contribution of their dimensions. However, this static approach is blind to i) the dynamics of jobs with cumulative deprivations relative to other states of the labour market such as unemployment or inactivity; and ii) the dynamics of the contributing dimensions of the index and their interdependencies that are ultimately the drivers behind index changes. In the latter, identifying the driving dimensions is not trivial (Alkire et al. Citation2015). Analysing any multidimensional index over time needs to simultaneously identify the dynamics of each dimension within the composite index’s transitions.
This article develops a method for measuring multidimensional precarious employment from a longitudinal perspective. It uses the decomposability property of the Alkire-Foster methodology to analyse dynamics subgroups of workers and their dimensional contributions using 2004–2020 panel data from Chile’s Social Protection Survey (EPS).
II. Methodology and data
Measurement of multidimensional precarious employment
This approach adapts the dual-cut-off counting approach proposed by Alkire and Foster (Citation2011) to produce an aggregate decomposable index of multidimensional precarious employment (PE) (García-Pérez, Prieto-Alaiz, and Simón Citation2017). Footnote1 First, the methodology applies a vector of deprivation cut-offs (denoted ) to identify those workers who are deprived in a given dimension. It then defines a counting vector
as the weighted sum of labour deficiencies experienced by the worker
, where
is a variable that takes the value 1 if the worker achievement
is less than
in dimension
and 0 if not.
is the weight assigned to each dimension and D is the total number of deprivations analysed.
In a second step, it sets a multidimensional threshold (denoted ) to classify individuals working in multidimensional PE. After identification, a simple form of aggregation is the calculation of the incidence of PE. It is defined as
, where
is the number of workers in multidimensionally precarious jobs and N is the total of the population. The intensity or the percentage of deprivations of the average PE, is defined as
/
, where
is the censored counting vector because the deprivations of secure jobs for the individual
are replaced with zero values (if
then
).
The PE adjusted ratio is calculated as the weighted sum of deprived dimensions among those who hold precarious jobs in relation to the total number of possible deprived dimensions for all workers:
.Footnote2 The
can be decomposed into dimensional partial indices
called censored headcount ratios that depict the percentage of the population who are working in multidimensional precarious jobs and are deprived in the employment dimension
at the same time. Formally,
.
Multidimensional precarious employment dynamics
In our approach, we focus on six distinct labour market dynamics between and
: i) individuals who are not-working and take a job; ii) individuals who are working and exit a job; iii) individuals who are ongoing in precarious employment; iv) individuals who move from precarious employment to secure employment; v) individuals who move from secure employment to a precarious job; vi) individuals who are ongoing in a secure job. We refer to these mutually exclusive and exhaustive groups as dynamic subgroups of workers.
The first two cases are simple to analyse because are the for each dynamic subgroup:
=
and
=
. The other four transitions must consider the interactions between the dimensions and their relationship with the PE index dynamics; therefore, they require further elaboration.
One important property that the Alkire/Foster family of measures satisfies is decomposability. Thus, PE changes over time can be calculated and decomposed into different aggregate measures following the recent literature on multidimensional poverty dynamics (Apablaza and Yalonetzky Citation2013; Alkire et al., Citation2017; Suppa Citation2018). Following these decompositions, the change in the adjusted headcount ratio of PE can be decomposed by population subgroups:
The first component of EquationEquation 1(1)
(1) is due to the change in the intensity of those who remain in PE in both periods – the ongoing PE – weighted by the size of this dynamic subgroup. The second component reveals the change in the intensity of those who enter precarious jobs also weighted by the size of this dynamic subgroup and the third component reflects the population-weighted change in the intensity of those who exit precarious jobs.
The challenge to identify changes in dimensional partial indices of PE is that they can result from workers in precarious jobs exiting deprivation or from workers leaving precarious jobs due to improvements in other PE dimensions. Changes in dimensional partial indices of PE can also be decomposed into dynamic subgroups as follows:
Each component is related to a pair of transitions (see ). Formally,
Figure 1. Transitions that change the censored headcount ratio.

=
,
=
and
=
.
Data description
The empirical analysis is based on six waves of the Chilean Social Protection Survey (Encuesta de Protección Social, EPS) gathered between 2004 and 2020. During each round, the EPS registers information on an individual’s employment situation and working conditions. EPS data are therefore useful for measuring and analysing the multidimensional precariousness of employment. Subsamples with individuals interviewed in both wave and wave
were selected to analyse these data from a longitudinal perspective (e.g. the two-wave panel 2006/07–2009/10 has 9,670 individuals in both waves).
The dimensions of precariousness must capture the economic job insecurities that affect people’s well-being (Kalleberg, Citation2018). The measure of precarious employment includes objective dimensions of employment-related economic insecurity such as income insecurity, job insecurity, working time insecurity, and social protection insecurity (e.g. Rodgers, Citation1989). We adapts the dimensions and cut-offs of the PE proposed by García-Pérez, Prieto-Alaiz, and Simón (Citation2017) to use with the EPS data ().
Table 1. Precarious employment insecurity dimensions, indicators and cut-offs.
III. Results
The four dimensions selected for precarious employment measurement are assigned an equal weight, and the precarious employment cut-off is set at k = 2.Footnote3 shows the evolution of the PE index by gender. Precarious employment is higher among women than men during the period studied, showing an upward trend in the gender gap since 2012/13. illustrates that the MPE for men decreased steadily from 0.184 in 2009/10 to 0.125 in 2019/20, while for women, the MPE increased from 0.250 in 2009/10 to 0.290 in 2012/07, although then dropped to 0.232 in 2019/20. That year the MPE difference between men and women was 0.106 (40% greater than 2004/05).
Figure 2. Evolution of the multidimensional PE Index (Mpe), 2004/05 – 2019/20.

Below, two dynamic analyzes of the multidimensional PE index illustrate the advantages of the longitudinal perspective. The first compares the MPE of those who remain employed between two waves with those who leave/enter non-working status by gender. reveals a precarious employment trap: both women and men move from unemployment/inactivity to precarious jobs and vice versa, but the jobs obtained by women are significantly more precarious than men. For example, the MPE of women workers who remained employed was 0.259, and for those who entered or exited unemployment was 0.345. In the case of men, the former’ MPE was 0.165, and the latter was 0.251.
Table 2. Multidimensional PE index by workers dynamics subgroups.
Regarding the dimensions’ contributions to the MPE, shows that income insecurity is the dimension that contributes the most to the MPE for working women who enter/leave unemployment but for men who remain in employment, it is the one that contributes the least to it.
Figure 3. Contribution of each dimension to MPE in three workers dynamics subgroups by gender.

The second analysis focuses on MPE dynamics for individuals employed in two consecutive waves by gender. By way of illustration, it focuses on two economic periods. The first corresponds to measurements between 2006/07 and 2009/10 (economic crisis). The second to the period between 2012/13 and 2015/16 (economic growth). shows that despite having changes in the MPE of − 6.9 values in the entry of precarious employment for male workers during the financial crisis, the MPE does not improve. In the economic growth period, women improved the MPE because those who moved to more secure jobs changed the MPE to − 11.2 values while those who entered precarious jobs were less, showing an improvement in the MPE index of 5.7 values.
Figure 4. Mpe index dynamics by gender during different economic periods.

Dynamic analysis of the censored headcount ratios or partial indices allows us to understand the contributions of the dimensions to the changes in the MPE index. shows how income insecurity increase explains why the MPE did not vary despite improving economic insecurities in the other dimensions during the financial crisis. For the period of economic expansion, both improving income and insecure jobs dimensions drive a significant reduction in the MPE index among women. In the case of men, an increase in insecurity of the social protection dimension is observed despite the decrease in the MPE index.
IV. Conclusion
Monitoring and policy evaluation of the development of labour markets implies understanding how multidimensional job deprivations change over time and what drives these changes. The adjusted headcount ratio of the Alkire/Foster class of measures has been shown to be relevant for studying the cumulative dimensions of job deprivation. From a longitudinal perspective, the property of this measure of breaking down subgroups and dimensions allows for dynamic analysis of (1) overall transitional trends; and (2) multidimensional index changes resulting from transitions within the dimensions.
Acknowledgements
We thank Mauricio Apablaza and Gastón Yalonetzky for their comments. We would also like to acknowledge the feedback offered by the participants of the WEIPO seminar at the University of Alcalá and the HDCA Conference in Antwerp. We are grateful for the support of the British Academy Grant number (GP1\100170).
Disclosure statement
No potential conflict of interest was reported by the author(s).
Additional information
Funding
Notes
1 The same approach could be applied to other multidimensional deprivations in job measures, such as quality of employment (e.g. Sehnbruch et al. Citation2020).
2 The is known as the Adjusted Headcount Ratio (
) in the family of poverty measures developed by Alkire and Foster (Citation2011).
3 Sensitivity analyses for other K cut-offs and other weight distributions can be requested from the authors.
References
- Alkire, S., and J. Foster. 2011. “Counting and Multidimensional Poverty Measurement.” Journal of Public Economics 95 (7–8): 476–487. doi:10.1016/j.jpubeco.2010.11.006.
- Alkire, S., J. Foster, S. Seth, M. E. Santos, J. M. Roche, and P. Ballon. 2015. “Distribution and Dynamics.” In Multidimensional Poverty Measurement and Analysis, 256–294. Oxford: Oxford University Press.
- Alkire, S., J. M. Roche, and A. Vaz. 2017. “Changes Over Time in Multidimensional Poverty: Methodology and Results for 34 Countries.” World Development 94: 232–249.
- Apablaza, M., and G. Yalonetzky. 2013. “Measuring the Dynamics of Multiple Deprivations Among Children: The Cases of Andhra Pradesh, Ethiopia, Peru and Vietnam.” Young Lives Working Paper 101. University of Oxford.
- García-Pérez, C., M. Prieto-Alaiz, and H. Simón. 2017. “A New Multidimensional Approach to Measuring Precarious Employment.” Social Indicators Research 134 (2): 437–454. doi:10.1007/s11205-016-1447-6.
- Kalleberg, A. L. 2018. Precarious Lives: Job Insecurity and Well-Being in Rich Democracies. Cambridge, UK: Polity Press.
- Muñoz de Bustillo, R., E. Fernández-Macías, F. Esteve, and J. -I. Antón. 2011. “E Pluribus Unum? A Critical Survey of Job Quality Indicators.” Socio-Economic Review 9 (3): 447–475. doi:10.1093/ser/mwr005.
- OECD. 2014. “Non-Regular Employment, Job Security and the Labour Market Divide.” In OECD Employment Outlook 2014, 141–209. Paris: OECD Publishing.
- Piasna, A., B. Burchell, and K. Sehnbruch. 2019. “Job Quality in European Employment Policy: One Step Forward, Two Steps Back?” Transfer: European Review of Labour and Research 25: 165–180. doi:10.1177/1024258919832213.
- Rodgers, G. 1989. “Precarious work in Europe: The state of the debate.” In Precarious Jobs in Labour Market Regulation: The Growth of Atypical Employment in Western Europe, edited by G. Rodgers and J. Rodgers, 1–16. Geneva: International Institute for Labour Studies, International Labour Organization.
- Sehnbruch, K., P. González, M. Apablaza, R. Méndez, V. Arriagada, J. L. Leroy, P. Menon, D. K. Olney, S. Roy, and M. Ruel. 2020. “The Quality of Employment (QoE) in Nine Latin American Countries: A Multidimensional Perspective.” World Development 127: 20. doi:10.1016/j.worlddev.2019.104822.
- Suppa, N. 2018. “Transitions in Poverty and Its Deprivations: An Analysis of Multidimensional Poverty.” Social Choice and Welfare 51 (2): 235–258. doi:10.1007/s00355-018-1114-8.