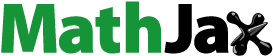
ABSTRACT
Research suggests that people prefer human over algorithmic decision-makers at work. Most of these studies, however, use hypothetical scenarios and it is unclear whether such results replicate in more realistic contexts. We conducted two between-subjects studies (N=270; N=183) in which the decision-maker (human vs. algorithmic, Study 1 and 2), explanations regarding the decision- process (yes vs. no, Study 1 and 2), and the type of selection test (requiring human vs. mechanical skills for evaluation, Study 2) were manipulated. While Study 1 was based on a hypothetical scenario, participants in pre-registered Study 2 volunteered to participate in a qualifying session for an attractively remunerated product test, thus competing for real incentives. In both studies, participants in the human condition reported higher levels of trust and acceptance. Providing explanations also positively influenced trust, acceptance, and perceived transparency in Study 1, while it did not exert any effect in Study 2. Type of the selection test affected fairness ratings, with higher ratings for tests requiring human vs. mechanical skills for evaluation. Results show that algorithmic decision-making in personnel selection can negatively impact trust and acceptance both in studies with hypothetical scenarios as well as studies with real incentives.
With the tremendous progress in artificial intelligence (AI), new applications are available to organizations that allow automating decision-making processes that were previously carried out by humans (Langer & Landers, Citation2021). As a result, humans find themselves in the decision-making power of algorithms and thus in a fundamentally different role than the well-established role of humans as users or consumers of technology (Wesche & Sonderegger, Citation2019).Footnote1
Recent reviews (Langer & Landers, Citation2021; Parent-Rocheleau & Parker, Citation2021) conclude that people mostly respond more negatively to algorithmic compared to human decision-making (in the following ADM and HDM) for decisions at work that affect them. However, as Parent-Rocheleau and Parker (Citation2021) put forth, “focusing solely on the negative consequences of algorithmic management is not a fruitful long-term approach. [… T]hese effects can be influenced and managed by the decisions of stakeholders in organizations” regarding the design and the implementation of ADM systems. Accordingly, Langer and Landers (Citation2021) identify the provision of explanations regarding ADM as one important design choice that can help to alleviate people’s scepticism and negative attitudes. Moreover, they point to another, more fundamental choice that can influence people’s scepticism and negative expectations and attitudes regarding ADM at work, namely the type of task for which ADM is implemented.
While first studies have been conducted to elucidate the effects of such design and implementation choices regarding ADM vs. HDM at work, the majority of them were based on hypothetical scenarios with no impact on participants’ real lives. For example, Langer and Landers (Citation2021) reviewed 36 empirical studies exploring ADM vs. HDM at work of which only two were not based on hypothetical scenarios. Yet, the transferability of findings from hypothetical scenario studies to real-life situations is limited (e.g., Eifler, Citation2007). Hence, it is important to study the use of ADM in organizational contexts with participants that are personally affected by the decision-making situation (Langer & Landers, Citation2021). This contribution will address this methodological challenge while exploring the effects of choices of implementation (for what kind of decisions?) and design (in what way?) of ADM systems at work on people’s responses.
Theoretical background
Type of the decision-maker
Acceptance of and compliance with orders and decisions by human decision-makers are important topics both in leadership but also in personnel selection research. In this regard, trust (Burke et al., Citation2007) as well as perceptions of fairness and justice (Dirks & Ferrin, Citation2002; Gilliland, Citation1993) have been identified as important mediators. When it comes to algorithmic decision-makers, research shows that people respond more negatively to them compared to their human counterparts: For example, people rate decisions regarding layoffs and promotions, bonus payment, or personnel selection as less fair when made by ADM compared to HDM (Acikgoz et al., Citation2020; Newman et al., Citation2020). Similarly, they perceive decisions regarding hiring or work evaluations made by ADM compared to HDM not only as less fair but also as less trustworthy and eliciting more negative emotions (Lee, Citation2018). Also, research on people’s acceptance of and compliance with orders and decisions indicates that people follow orders to a lesser extent when these come from an algorithmic compared to a human leader (Geiskkovitch et al., Citation2016). Based on these deliberations, we assume:
Hypothesis 1:
Being informed that a decision is taken by an algorithm compared to a human negatively influences a) trust in the decision-maker, b) acceptance of the decision-maker, c) acceptance of the selection decision, and d) perceived fairness of people that are subject to these decisions.
Provision of explanations
Providing explanations regarding decision processes, decision criteria, or decision results usually has positive effects on the reactions of people affected by these decisions (Truxillo et al., Citation2009). Explanations can be given at different times in a decision-making process (i.e., before, during, and after the decision) and can also convey different contents (e.g., what happens in the process, what data is used, what decision criteria apply, or the reasons for a particular decision result) (Georgiou, Citation2021).
While a positive effect of providing explanations has been found in many studies regarding human-made decisions, explainable AI (XAI, providing explanations regarding AI-systems functioning) has been identified as an important characteristic of ADM systems (Langer & Landers, Citation2021). Especially for applications in high-stakes situations, explanations are essential to understand, trust, and effectively manage AI-tools (Gunning et al., Citation2019). However, there are various groups of people interacting with AI that have different interests and informational needs: users, regulators, deployers, developers, and last but not least, affected parties (Langer et al., Citation2021).
Hence, the literature on explanations in the context of ADM and HDM is very divers, differing according to the recipients, the timing, and the content of explanations. Here, we will focus on explanations that are provided (1) to the people affected by the decision, (2) before the decision process, that (3) contain general information on the procedure. Based on the evidence regarding human-made decisions and the general deliberations regarding XAI for ADM, we assume:
Hypothesis 2:
Providing explanations regarding the decision processes and the decision criteria positively influences a) trust in the decision-maker, b) acceptance of the decision-maker, c) acceptance of the selection decision, and d) perceived fairness of people that are subject to these decisions.
However, Newman et al. (Citation2020, Study 5) describe findings that indicate a differential effect of transparency on participants’ reactions depending on the type of the decision-maker: In the HDM condition, high compared to low transparency led to lower perceptions of decontextualisation (i.e., the failure to adequately consider performance in a broader context) and higher perceptions of fairness. Conversely, in the ADM condition, high compared to low transparency made no difference in participants’ perception of decontextualisation and even led to lower perceptions of fairness. One way to interpret these findings is that due to the complexity of the system, organizations may be unable to provide a satisfactory explanation of how a specific decision is taken by ADM leading to low perceived informational fairness (Acikgoz et al., Citation2020). Another interpretation could be that participants implicitly measure ADM against higher standards regarding transparency than HDM (Zerilli et al., Citation2018) and therefore need more or different explanations regarding ADM compared to HDM to perceive a comparable level of fairness and trust.
Focussing solely on ADM, Langer and colleagues assumed that providing explanations increases perceptions of transparency, controllability, and appropriateness of such decision processes and hence positively influences people’s perceptions of and attitudes towards the decision procedure and organization. However, their findings were mixed: While they found in their first study (Langer et al., Citation2018) that providing (vs. not providing) explanations regarding the functioning of an automated selection interview software positively affects participants’ perception of knowing relevant information, transparency, and open treatment, it did not directly relate to organizational attractiveness. In their second study (Langer et al., Citation2021), they found that providing (vs. not providing) explanations regarding the functioning of the automated selection interview software did not yield assumed positive effects on neither perceived transparency, fairness, nor organizational attractiveness, but increased perceived creepiness and privacy concerns.
Despite these inconclusive findings, we assume in line with assumptions put forward in the domain of XAI that being subject to ADM compared to HDM evokes a particular need for information among participants (Zerilli et al., Citation2018) and that the absence of such information creates a “black box”-perception that will negatively influence participants’ evaluation of the decision-maker and the decision-making process.
Hypothesis 3:
The negative effects of algorithmic compared to a human decision-makers on participants’ assessments of the decision-maker and the decision-making process (as described in H1) are moderated by the degree of explanation given, such that these negative effects are stronger in the no-explanation condition than in the explanation condition.
Type of the decision-making task
A fundamental choice organizations have to take is which tasks to delegate to ADM and which tasks to leave with HDM. This is relevant, as people differ in their preferences for ADM vs. HDM depending on the field of the decision-task (e.g., prefering HDM for personnel or medical diagnoses and ADM for optimization of travel routes or text processing, Grzymek & Puntschuh, Citation2019). Even within specific fields, people’s reactions to ADM vs. HDM differs depending on the specific decision-making task. For example, regarding personnel selection, participants reacted less negatively to ADM in the screening stage compared to the interview stage (Wesche & Sonderegger, Citation2021).
In this regard, Lee (Citation2018) distinguished between tasks that (people perceive to) require human skills (e.g., subjective judgement and emotional abilities) and tasks that require mechanical skills (e.g., processing of quantitative data). She showed that participants’ ratings of fairness, trust and emotion were more positive for tasks requiring human skills (e.g., hiring decision, work evaluation) if a human was performing the task compared to an algorithm. Conversely, no differences in ratings between ADM and HDM were observed for tasks that require mechanical skills only. Similarly, Castelo et al. (Citation2019) found that people show less trust, less willingness to use, and less reliance on ADM compared to HDM, when they perceive a task to involve interpretation and intuition vs. quantifiable facts and logic. Also, Nagtegaal (Citation2021) found that participants evaluated procedural justice higher for ADM on tasks requiring mechanical skills (i.e., calculation of pension plans or commuting reimbursements) and higher for HDM on tasks requiring human skills (i.e., employee performance evaluation or hiring decisions).
Accordingly, we expect that trust in and acceptance of ADM (compared to HDM) would be lower for decision-tasks that require human skills, while no such differences would occur for decision-tasks that requiring mechanical skills.
Hypothesis 4:
Performing selection tests that require human skills for their evaluation compared to selection tests that require mechanical skills for their evaluation negatively influences a) trust in the decision-maker, b) acceptance of the decision-maker, c) acceptance of the selection decision, and d) perceived fairness of people subject to these decisions made by algorithmic compared to human decision-makers.
Study 1
Study 1 sets out to test the hypothesized effects of the type of the decision-maker (H1) and the provision of explanations (H2) as well as the interaction of both factors (H3) on a) trust in the decision-maker, b) acceptance of the decision-maker, and c) acceptance of the selection decision of people subject to these decisions. In addition, qualitative data was collected on the reasons for the respective quantitative assessments of the dependent variables.
Methods
Participants
Our sample consisted of 270 German-speaking participants from the general population, of which 64.81% identified as female and 34.81% as male, while 0.37% did not state their gender. Participants were on average 39.33 years old (SD = 14.67) with an average work experience of 16 years (SD = 13.44).
A priori sample size estimation was based on an expected population effect of ƒ = 0.21 (calculated based on meta-analytical data of a similar research question, Blacksmith et al., Citation2016). Assuming an error probability of α = .05, N = 180 participants would be necessary to achieve a power of 1–β = .80 (calculations based on G*Power software, Faul et al., Citation2007). Hence, our sample of N = 270 surpasses the estimated minimum sample size necessary to detect an effect of the estimated size.
Design and procedure
Study 1 was realized as a randomized online-experiment following a 2 × 2 between-subjects design. Participants were instructed to imagine working as a journalist at a newspaper publisher, where a decision is pending regarding participation in a training programme that would be important for their career (built closely on Vignette 2 from Ötting & Maier, Citation2018).
The factor “decision-maker” was manipulated by telling participants in the HDM condition that a “selection committee” would make the decision, while telling participants in the ADM condition that an “algorithm” would make the decision. To ensure a common understanding, we presented participants a definition of the term “algorithm” (Lee, Citation2018). By referring to a “committee of managers” in the HDM condition, we decided against using a single manager, to avoid that participants expect an individual manager taking the decision to show nepotism and rule in favour of particular applicants.
The factor “explanation” was manipulated by providing vs. not providing procedural information regarding the decision-making process. Participants in the explanation condition were informed about (1) the decision criteria, (2) the opportunity to check the personal information and correct possible mistakes, and (3) that the selection was a quality-controlled, standardized procedure complying with applicable regulations. In the no-explanation condition, no such information was provided (see ).
Table 1. Overview of the experimentally manipulated instructions in study 1.
Measures
All items were answered on five-point Likert scales (1 = strongly disagree, 5 = strongly agree, see ). Reliability checks indicated good internal consistencies (i.e., Cronbach’s alpha and for the two-item scale Spearman-Brown coefficients of .80) for all scales (see ).
Table 2. Overview of the adapted items used to measure the study variables in study 1 and study 2.
Table 3. Study 1: means, standard deviations, correlations, and reliability coefficients of the study variables.
Manipulation checks. The manipulation check regarding the type of decision-maker followed directly after participants read the scenario and was evaluated using the item: “Please indicate who took the decision about the vacant training positions.” (1) a selection committee, 2) a training provider, or 3) an algorithm (adapted from Ötting & Maier, Citation2018). If the answer was incorrect, participants were again presented with the scenario until they correctly answered the manipulation check question.
To check whether participants perceived differing levels of transparency between the conditions of providing vs. not providing explanations regarding the decision-making process, perceived transparency was assessed using a two-item scale by Langer et al. (Citation2018).
Dependent variables. Trust in the decision-maker was assessed with three items of the Trust Scale by Brockner et al. (Citation1997). Acceptance of the decision-maker and acceptance of the selection decision were assessed with one purpose-built item each. To explore the cognitive mechanisms behind participants’ quantitative ratings, they were asked to provide brief explanations in corresponding text fields.
Data analysis and preparatory analyses
Quantitative data analysis. We conducted two-factorial ANOVAs to assess the assumed main and interaction effects of the factors “decision-maker” and “explanation” on our three dependent variables.
Qualitative data analysis. The qualitative data was analysed with a mixed deductive and inductive approach to category formation (Mayring, Citation2014). After reviewing category systems of other qualitative analyses of participants’ thoughts about algorithmic selection decisions (Mirowska & Mesnet, Citation2021; Wesche & Sonderegger, Citation2021), the first coder read all participant comments and established initial topics. When coding, these topics were applied jointly to participants’ responses regarding trust, acceptance, and transparency but separately for the ADM and HDM conditions. After several iterations of coding, discussion and restructuring, a stable category system with six thematic categories and one rest category was formed. A second coder coded all comments according to this category system. Across these six categories, an inter-rater-reliability of Cohen’s kappa = 0.71 was achieved.Footnote2 Afterwards, both coders discussed discrepancies and a second coding iteration was held, resulting in a satisfactory inter-rater reliability of Cohen’s kappa = 0.80.
Manipulation checks. 18 participants (6.67%) failed the manipulation check for the decision-maker at least once, but finally all answered the respective question correctly. Providing explanations resulted in significantly higher perceptions of transparency (explanation: M = 3.51, SD = 1.02 vs. no-explanation: M = 2.40, SD = 0.98; t(268) = 9.07, p < .001, d = 1.10), thus, indicating successful manipulation.
Results
shows means, standard deviations and correlations of all relevant variables as well as reliability coefficients of all scales. shows means and standard deviations for each factor level.
Table 4. Study 1: means and standard deviations of the dependent variables by factor levels.
Analysis of quantitative data
Trust in the decision-maker. ANOVA showed higher trust ratings in the human (M = 3.01, SD = 0.70) compared to the algorithmic decision-maker (M = 2.56, SD = 0.88), F(1, 266) = 20.66, p < .001, ηp2 = .07). Giving explanations induced higher levels of trust in the decision-maker (M = 2.94, SD = 0.79) compared to the no-explanation condition (M = 2.61, SD = 0.82), F(1, 266) = 10.46, p = .001, ηp2 = .04). The interaction effect decision-maker x explanation was small and not significant (F(1, 266) = 0.55, p = .460, ηp2 = .00).
Acceptance of the decision-maker. Similarly, acceptance of the decision-maker was higher for the human (M = 3.80, SD = 0.83) compared to the algorithmic decision-maker (M = 2.80, SD = 1.15, F(1, 266) = 65.73, p < .001, ηp2 = .19) and giving explanations induced higher ratings in the explanation condition (M = 3.49, SD = 1.06) compared to the no-explanation one (M = 3.08, SD = 1.16, F(1, 266) = 8.77, p = .003, ηp2 = .03)). Again, the interaction effect of the two factors was small and not significant (F(1, 266) = 0.21, p = .650, ηp2 = .00).
Also, similar effects resulted regarding acceptance of the selection decision, with higher acceptance ratings for the human (M = 3.68, SD = 0.83) compared to the algorithmic decision-maker (M = 3.09, SD = 1.07, F(1, 266) = 25.13, p < .001, ηp2 = .08) and higher acceptance ratings in the explanation (M = 3.53, SD = 0.96) compared to the no-explanation condition (M = 3.23, SD = 1.03, F(1, 266) = 5.29, p = .022, ηp2 = .02). Again, the interaction of the two factors was small and not significant (F(1, 266) = 1.84, p = .177, ηp2 = .01).
Analysis of qualitative data
Analysis of participants’ qualitative responses resulted in six thematic categories (see ). Presented frequencies are based on the matching categorization of the first and second coder.
Table 5. Study 1: Summary of categories of text responses regarding participants’ perceptions of the decision-maker and the decision-making process.
1) Composition of the decision-maker/decision-making process (all comments n = 103; HDM condition n = 52; ADM condition n = 51). This category describes the evaluation of the quality of the decision-maker/decision-making process depending on how it has been composed (i.e., the process of creating the decision-maker/the decision-making process in terms of programming, training, or member selection) and on how the decision-maker/decision-making process works. In reference to these aspects, statements about and requests for transparency were mentioned.
Participants from the two conditions emphasized different aspects. In the ADM condition, participants were more interested in information about the composition process and the exact functioning of the decision-maker. In the HDM condition, participants were more interested in information about parameters of the selection process and who is sitting on the committee.
2) Objectivity vs. subjectivity of the decision-maker/decision-making process (all comments n = 55; HDM condition n = 20; ADM condition n = 35). This category comprises comments regarding the objectivity vs. subjectivity of the decision-maker and the decision process due to applying a consistent scheme to all candidates vs. deviating from it.
Again, participants emphasized different aspects in the two conditions. For algorithmic decision-makers, participants praised their objectivity and criticized a lack of necessary subjectivity. For human decision-makers, participants commented mostly on a lack of objectivity without mentioning positive aspects of subjectivity.
Decision-makers’ authority and legitimacy for the task (all comments n = 14; HDM condition n = 11; ADM condition n = 3). This category contains comments describing that the decision-maker has been chosen and authorized (by the organization) to make the selection decision and accordingly should bear the responsibility that comes with this role and act in the best way possible. This is also reflected in comments regarding perceived general legitimacy vs. a general lack of legitimacy of the decision-maker.
Human involvement (all comments n = 9; HDM condition n = 0; ADM condition n = 9). This category describes the general belief that humans should be involved in the selection process. Critique and discomfort about algorithms as sole decision-makers are expressed. Respective comments were only made by participants from the ADM condition.
Organizational interest (all comments n = 5; HDM condition n = 5; ADM condition n = 0). This category describes the impression that the interests of the organization are of primary concern in the selection decision and that the selection process is designed to serve the organization’s interests. Respective comments were only made by participants from the HDM condition.
General statements concerning acceptance (all comments n = 20; HDM condition n = 12; ADM condition n = 8). This last category reflects participants’ statements regarding their acceptance of the selection decision, without reference to topics of the other categories.
Discussion
Taken together, the quantitative data supports the assumed negative effect of ADM compared to HDM (H1a,b,c) and a smaller positive effect of providing (vs. not providing) explanations on trust in and acceptance of the decision-maker and acceptance of the selection decision (H2a,b,c). Contrary to our assumptions, we did not find evidence for an interaction effect between these two factors (H3).
The qualitative data helps us to understand the reasons for the negative effect of ADM vs. HDM and also people’s differential needs for information when they are subject to ADM vs. HDM. For example, participants from the ADM condition criticized decontextualisation, while participants from the HDM condition criticized that humans can or will not decide without considering personal contexts (category 2). Moreover, participants from the ADM condition mentioned that they simply do not want to be evaluated by an algorithm (category 4). Regarding participants’ informational needs, we see that participants in the ADM condition are interested to learn more about the algorithmic functioning, its parameters, and possible human regulatory authorities (category 1). In the HDM condition, participants are rather interested in who is sitting on the selection committee, whether the selection committee adheres to the communicated selection criteria and how the criteria will be weighted (category 1).
However, Study 1 has limitations. Specifically, the effect of providing explanations might have been confounded with providing opportunities for control, as our vignettes also informed participants that they have had the chance to check and correct their registered data. Moreover, scenario studies have a limited generalizability regarding real-life contexts.
Study 2
Study 2 sets out to examine whether the findings from Study 1 can be replicated with participants that are personally affected by the decision-making situation. Specifically, it tests the effects of the type of decision-maker (H1) and the provision of explanations (H2) as well as a possible interaction of these two factors (H3) on people’s evaluations of the decision-maker and the decision-making process. Moreover, Study 2 seeks to manipulate purely the provision of explanations and integrates the type of the decision-making task (H4) as an additional factor.
Methods
Participants
Our sample comprised 183 German-speaking participants from the general population, of which 39.89% identified as female, 54.50% as male, and 5.61% identified as diverse. Participants were on average 31.58 years old (SD = 8.05) with an average work experience of 9.4 years (SD = 7.83).
A priori sample size estimation was based on an expected population effect of ƒ = 0.21 (calculated based on meta-analytical data of a similar research question, Blacksmith et al., Citation2016). Assuming an error probability of α = .05, N = 180 participants would be necessary to achieve a power of 1–β = .80 (calculations based on G*Power software, Faul et al., Citation2007). Hence, our recruited sample of N = 183 meets the estimated minimum sample size necessary to detect an effect of the estimated size.
Design and procedure
Study 2 was realized as a randomized online-experiment following a 2 × 2 × 2 between-subjects design. To create a situation in which participants felt actually affected by the decision-making situation, we recruited participants for a highly attractive but bogus product test (a new online gaming engine) with an attractive remuneration of 50 EUR and convenient conditions (i.e., participation from home and personal choice of time). Participants were informed that they had to demonstrate their suitability in an online qualifying session if they wanted to take one of five available seats in this product test (see for the vignettes of all conditions) .Footnote3
Table 6. Overview of the experimentally manipulated instructions in study 2.
As experimental manipulation of the factor “decision-maker”, participants were told that the selection of participants would be made by a human vs. an algorithmic decision-maker. As in Study 1, a definition of the term “algorithm” was presented to ensure a common understanding.
Participants in the “explanation” condition received information on how the decision-maker evaluates the participants (i.e., in a standardized, quality-controlled procedure) and about the decision-making criteria, while in the no-explanation condition, no such information was provided.
The selection test, as experimental manipulation of the factor “decision-making task”, consisted either of 12 questions requiring logical reasoning from an established intelligence test (Liepmann et al., Citation2007) or questions requiring creativity taken from a creativity test used in advertisement agencies for the recruitment of creative staff (i.e., writing creative, convincing and funny short dialogues). These different tests were chosen as they require an evaluator (i.e., the human or algorithmic decision-maker) to use mechanical skills (i.e., counting correct answers in an intelligence test) or rather human skills (i.e., interpreting and evaluating participants’ answers in a creativity test).
Measures
For the sake of comparability, measurement of all variables assessed in Study 1 was kept identical (see ). As in Study 1, reliability checks indicated acceptable internal consistencies (i.e., Cronbach’s alpha and for the two-item scale Spearman-Brown coefficients of .75) for all scales (see ).
Table 7. Study 2: means, standard deviations, and correlations, and reliability coefficients of the study variables.
Dependent variables. In Study 2, four dependent variables were measured. The first three were identical to Study 1: trust in the decision-maker, acceptance of the decision-maker, and acceptance of the selection decision. Additionally, we assessed perceived fairness of the selection process with a 3-item scale from Langer et al. (Citation2018). Consistent with the other measures, the items were answered on a five-point Likert scale (1 = strongly disagree, 5 = strongly agree).
Manipulation checks. To check successful manipulation of the factor “decision-maker”, a manipulation check was inserted before participants gave their responses regarding the dependent variables. Participants were asked to indicate who would make the selection decision: (1) a neutral person, 2) an algorithm, or 3) the researcher). If the answer was incorrect, participants were again presented with the scenario until they correctly answered the manipulation check question.
As in Study 1, we assessed perceived transparency using a two-item scale by Langer et al. (Citation2018) to check whether participants perceived differing levels of transparency between the conditions of providing vs. not providing explanations regarding the decision-making process.
To check, whether participants perceived the tasks as requiring human vs. mechanical skills for their evaluation, two purpose-built items were used (“The test I took can be meaningfully evaluated by a human.” and “The test I took can be meaningfully evaluated by an algorithm.”).
Data analysis and preparatory analyses
We conducted three-factorial ANOVAs to assess the assumed effects of the factors “decision-maker”, “explanation”, and “decision-making task” on our four dependent variables. As to our knowledge, the interactions of these three factors have not yet been investigated, we calculated complete models including all main and interaction effects.
Manipulation checks. 82 participants (44.8%) failed the manipulation check for the decision-maker at least once, but answered it correctly before they could proceed.
Providing explanations regarding the selection process and selection criteria did not result in significantly higher perceptions of transparency (explanation: M = 2.70, SD = 1.19 vs. no-explanation: M = 2.90, SD = 1.16; t(181) = −1.14, p = .258, d = .17), indicating that the manipulation of the factor “explanation” was not successful.
Participants in the creativity test condition perceived their selection test to be less sensibly evaluable by an algorithm (M = 2.54, SD = 1.20) than participants in the cognitive ability test condition (M = 4.12, SD = 1.16; t(181) = −9.05, p < .001, d = 1.35). No such difference between the two selection test-conditions (creativity test: M = 4.15, SD = 1.03; cognitive ability test: M = 4.07, SD = 1.14) was found when participants were asked whether their selection test would be sensibly evaluable by a human (t(178) = −0.48, p = .627). These results indicate a successful manipulation of the factor “decision-making task”.
Results
shows means, standard deviations and correlations of all relevant variables as well as reliability coefficients of the scales. shows means and standard deviations for each factor level.
Table 8. Study 2: means and standard deviations of dependent variables by factor levels.
Trust in the decision-maker. ANOVA showed higher ratings of trust regarding the human (M = 3.46, SD = 1.09) compared to the algorithmic decision-maker (M = 2.74, SD = 1.03, F(1,175) = 22.73, p < .001, ηp2 = .12). The main effects of explanation (F(1, 175) = 0.25, p = .616, η2 = .00) and the selection test (F(1, 175) = 1.82, p = .179, ηp2 = .01) were small and not significant. While all two-way interaction effects were not significant (Fdecision-maker*explanation(1, 175) = 0.12, p = .726, ηp2 = .00; Fdecision-maker*task(1, 175) = 1.30, p = .257, ηp2 = .01; Fexplanation*task(1, 175) = 0.39, p = .533, ηp2 = .00), the interaction of all three factors “decision-maker”, “explanation” and “type of selection test” showed a significant effect (F(1, 175) = 4.49, p = .036, ηp2 = .03). However, due to the relatively small sample size for a three-way interaction and the fact that we proposed no respective hypothesis, we refrain from interpreting it.
Acceptance of the decision-maker. ANOVA showed higher acceptance ratings regarding the human (M = 4.16, SD = 1.11) compared to the algorithmic decision-maker (M = 3.45, SD = 1.20, F(1, 174) = 17.49, p < .001, ηp2 = .09). Neither the main effects of the factors explanation (F(1, 174) = 0.84, p = .362, ηp2 = .01) and selection test (F(1, 174) = 0.76, p = .383, ηp2 = .00) nor any of the interaction effects showed significant results (Fdecision-maker*explanation(1, 174) = 0.02, p = .900, ηp2 = .00; Fdecision-maker*task(1, 174) = 2.33, p = .128, ηp2 = .01; Fexplanation*task(1, 174) = 0.34, p = .560, ηp2 = .00; Fdecision-maker*explanation*task(1, 174) = 0.04, p = .840, ηp2 = .00).
Acceptance of the selection decision. ANOVA showed higher acceptance ratings of the selection decision when made by a human (M = 4.19, SD = 1.10) compared to an algorithmic decision-maker (M = 3.82, SD = 1.24, F(1, 174) = 4.37, p = .038, ηp2 = .02). Neither the main effects of the factors explanation (F(1, 174) = 0.02, p = .897, ηp2 = .00) and selection test (F(1, 174) = 1.06, p = .304, ηp2 = .01) nor any of the interaction effects showed significant results (Fdecision-maker*explanation(1, 174) = 0.16, p = .690, ηp2 = .00; Fdecision-maker*task(1, 174) = 0.35, p = .554, ηp2 = .00; Fexplanation*task(1, 174) = 0.93, p = .336, ηp2 = .01; Fdecision-maker*explanation*task(1, 174) = 0.24, p = .625, ηp2 = .00).
Fairness of the selection process. ANOVA showed lower fairness ratings regarding the selection based on the test requiring human skills for its evaluation (creativity test, M = 3.44, SD = 1.00) than based on the test requiring mechanical skills for its evaluation (cognitive ability test, M = 3.78, SD = 0.96, F(1, 175) = 5.56, p = .020, ηp2 = .03). Neither the main effects of the factors decision-maker (F(1, 175) = 1.17, p = .281, ηp2 = .01) or explanation (F(1, 175) = 0.35, p = .556, ηp2 = .00) nor any of the interaction effects (Fdecision-maker*explanation (1, 175) = 0.84, p = .362, ηp2 = .01; Fdecision-maker*task (1, 175) = 0.37, p = .544, ηp2 = .00; Fexplanation*task(1, 175) = 0.28, p = .598, ηp2 = .00; Fdecision-maker*explanation*task(1, 175) = 0.76, p = .384, ηp2 = .00) showed significant results.
Discussion
Consistent with Study 1 and previous findings from vignette studies, our results from a selection process with real incentives show that the use of ADM instead of HDM can negatively impact trust and acceptance. This was not the case for fairness of the decision-making process. Thus, our results support the main part of our central hypothesis (H1a, b, and c).
Contrary to our expectations and to the results of Study 1, the experimental manipulation of explanation did not show a significant effect on our manipulation check measure “perceived transparency” nor on any of the dependent variables (H2). Similarly, but consistent with Study 1, the expected interaction of the factor “decision-maker” and “explanation” did not receive support. Hence, the expectation that missing explanations would be perceived as particularly negative in the ADM condition and be of lesser importance in the HDM condition (H3) was not confirmed. Finally, our assumption that ADM (but not HDM) would be rated more negatively when used for decision-tasks requiring human compared to mechanic skills (H4) was also not supported. Instead, our data suggested a main effect of type of task on perceived fairness indicating that the task requiring decision-makers’ mechanical skills was generally perceived as fairer than the task requiring decision-makers’ human skills for scoring.
Overall discussion
In one experiment with hypothetical scenarios (Study 1) and one with real incentives (Study 2), we showed that HDM was rated more positively than ADM on the variables trust and acceptance. In Study 1, also providing explanations regarding the selection process resulted in more positive ratings of trust and acceptance, while it did not in Study 2. The type of decision-making task had a main effect on perceived fairness, irrespective of the type of decision-maker (human vs. algorithm). Qualitative analysis of participants’ comments from Study 1 revealed that participants were mostly concerned with the composition and creation of the decision-maker as well as subjectivity and objectivity of the decision-maker in both the ADM and the HDM conditions. These results can inform organizations’ strategic considerations regarding whether or not personnel selection decisions should be delegated to algorithmic decision making and, if so, to what extent (e.g., regarding the kind of selection tests) and in which implementation form (e.g., regarding information provision).
Limitations
The results of our experiments need to be interpreted with caution when applied to an organizational work context. This is due to the use of hypothetical scenarios in Study 1 and the specific selection situation in Study 2. As participants applied for participation in an attractively remunerated one-time activity, this selection situation might be considered similar to the work environments of gig-workers (Duggan et al., Citation2019). Hence, replications in more traditional work environments (Jarrahi et al., Citation2021) with employees who have a long-term interest in their working conditions might be of interest for future research.
Moreover, it could be argued that in Study 2 the level of desirability for participation in the product test might not have been high since 50 EUR are not a large amount of money. However, considering the statutory minimum wage per hour in Germany at the time of the study (i.e., 2019: 9,19 EUR) a remuneration of 50 EUR for a one-hour product test that was announced to be easily done from home does not fare badly. In addition, it can be assumed that people taking part in the qualifying session were interested and expected to experience fun when testing video games. However, Study 2 unfortunately provides no measure to ascertain participants’ actual motivation to take part in the product test.
Lastly, the sample in Study 2 is rather small with regard to the complex experimental design. Due to this reservation, we refrained from interpreting the significant three-way interaction on trust. Accordingly, the results of this study should be interpreted with caution and, if possible, be replicated with larger samples.
Theoretical implications
Providing explanations
Our studies give qualitative and quantitative hints to contribute to the riddle of XAI. The qualitative data informs us about what participants actually wanted to know to build trust in the decision-maker and accept the decision-maker and the decision itself. Here, we find some comparable but also different aspects that people want to know when the decision-maker is a human compared to an algorithm.
The quantitative data, especially from Study 2, is in line with previous research reporting that providing explanations to people affected by ADM does not simply or consistently contribute to their trust and acceptance (Langer et al., Citation2018, Citation2021; Newman et al., Citation2020). An interesting line of thought comes from Ananny and Crawford (Citation2016), who claim that receiving information without also being granted the power to act on that information makes transparency lose its purpose and renders it futile. While we provided participants in the “explanation” condition of both Study 1 and Study 2 with comparable information, (1) that the selection process is standardized and quality-controlled and (2) what the decision criteria are, we only informed participants in Study 1 that they have had the chance to check their registered data for possible errors. Our initial rationale for including the latter information in Study 1 was, that allowing to inspect the data that is used for the selection process would contribute to transparency. However, having conducted Study 1 and planning Study 2, we felt that allowing to inspect (and if necessary to correct) data might be perceived not only as granting transparency but also as a possibility to exert control. The difference we find regarding the effect of providing explanations to participants between Study 1 and Study 2 might thus support the assumption that information is only perceived as beneficial, when it comes with the possibility to act on that information. Our findings therefore underline the importance of information design for effective XAI.
Type of task
Study 2 set out to explore the effects of different decision-making tasks and associated with that different levels of appropriateness that people ascribe to ADM vs. HDM regarding these tasks (Castelo et al., Citation2019; Lee, Citation2018; Nagtegaal, Citation2021). However, Study 2 did not support our assumption of an interaction between the type of the decision-maker and the type of decision-making task (H4). Instead we found a main effect of the type of task on perceived fairness. An explanation for the absence of an interaction effect could be that fairness perceptions have a pervasive effect, irrespective of the decision-maker, as found in two studies by Ötting and Maier (Citation2018). Thus, the main effect might be due to participants’ perspective as a test-taker in general (e.g., that they do not like taking less predictable creativity tests) and independent of whether the task is more or less meaningfully evaluable by a human vs. an algorithmic decision-maker.
Another interpretation could be that participants perceived the creativity test as less fair in both conditions, but for different reasons: While participants criticized human decision-makers subjectivity and lack of objectivity (see the qualitative data of Study 1, category 2), they believed that algorithmic decision-makers lack the capability to meaningfully evaluate human performance in creativity tests (see the manipulation check in Study 2). Thus, we join the call of Langer and Landers (Citation2021) for further exploration of the effect of task characteristics on people’s responses to ADM.
Practical implications
Given the negative effect of ADM vs. HDM on people’s trust and acceptance regarding the decision-maker and the decision itself in both Study 1 and 2, our results underline the importance stressed in various calls (e.g., Bolander, Citation2019; Parry et al., Citation2016) that organizations should well consider which decision-making tasks they delegate to ADM and which ones should remain with HDM. In this regard, it seems to be more important what people believe that ADM systems are capable of, than their de-facto technological capability (Wesche & Sonderegger, Citation2021). Analogous to the proverb “no trust, no use” relating to users or consumers of technology (Schaefer et al., Citation2016), “no trust, no acceptance” might be relevant for people working under ADM systems.
Here, organizational communication accompanying decision-making systems comes into play and despite the inconclusive results regarding alleviating effects of explanations for scepticism regarding ADM (Langer et al., Citation2018, Citation2021; Newman et al., Citation2020, but also Study 2 of this manuscript), we advise against throwing out the baby with the bathwater. Our qualitative analysis indicates that people have specific informational needs regarding different decision-making situations and it is conceivable that answering these needs would help to increase perceived transparency, fairness and alleviate scepticism. For example, participants in the HDM condition were interested in information on the human decision-maker’s adherence to decision criteria, while participants in the ADM condition were interested in information on the algorithm’s functioning and the existence of human regulatory authorities. Others also point out that people working with the same algorithmic decision-making system have different informational needs due to their prior experience with or knowledge of such technologies (Langer et al., Citation2021). Thus, exploring thoroughly the informational needs of employees working with ADM systems and tailoring provided explanations specifically to these needs seems to be a promising route for organizations to increase trust and acceptance regarding these systems.
Conclusion
In line with previous, mostly vignette-based research, our results suggest that using ADM instead of HDM negatively impacts people’ trust and acceptance regarding decision-making processes at work, both in a study where participants read fictitious vignettes (Study 1) and in a study where participants worked for real incentives (Study 2). Effects of providing explanation to participants and the tasks that human vs. algorithmic decision-makers had to evaluate were not conclusive and need further investigations.
Taken together, our (partly inconclusive) results underscore the pressing need for an overarching theory of ADM systems in the work context that spurs systematic examinations of design and implementation features (Wesche & Sonderegger, Citation2019).Moreover, we believe that in order to achieve that, the research field needs to move beyond simple imagined one-shot interactions with ADM exploring solely basic effect (ADM vs. HDM). Examinations of the more fine-grained effects of different designs and implementations of ADM systems in studies with participants that have a real and not only imagined interests in their working situation are needed to provide for the necessary knowledge on how to design ADM technology for the good of both, organizations and employees.
Disclosure statement
No potential conflict of interest was reported by the authors.
Notes
1. All materials of both studies (instructions and items in both English and German) as well as all data and quantitative as well as qualitative analyses are documented in the corresponding project folder on the OpenScienceFramework (https://osf.io/hxwpr/). Study 2 was preregistered on OSF. Both studies obtained ethical approval (Internal Review Board University of Fribourg, IRB_520, Ethics Committee of the Department of Education and Psychology of the Free University of Berlin, Nr. 041.2019).
2. When coders assigned a qualitative response to more than one category and this resulted in an uneven number of category assignments for this response between both coders, the non-overlapping category assignments were dropped for the analysis of the inter-rater-reliability.
3. Upon completion of the study, participants were debriefed about the true purpose of the study, that the alleged qualifying session was in fact the actual study, and that the product test would not take place. Moreover, they were informed that instead of receiving 50 EUR for participation in the product test, five participants were determined by lottery that received 50 EUR.
References
- Acikgoz, Y., Davison, K. H., Compagnone, M., & Laske, M. (2020). Justice perceptions of artificial intelligence in selection. International Journal of Selection and Assessment, 28(4), 399–416. https://doi.org/10.1111/ijsa.12306
- Ananny, M., & Crawford, K. (2016). Seeing without knowing: Limitations of the transparency ideal and its application to algorithmic accountability. New Media & Society, 20(3), 973–989. https://doi.org/10.1177/1461444816676645
- Blacksmith, N., Willford, J. C., & Behrend, T. S. (2016). Technology in the employment interview: A meta-analysis and future research agenda. Personnel Assessment and Decisions, 2(1), 12–20. https://doi.org/10.25035/pad.2016.002
- Bolander, T. (2019). What do we loose when machines take the decisions? Journal of Management and Governance, 23(4), 849–867. https://doi.org/10.1007/s10997-019-09493-x
- Brockner, J., Siegel, P. A., Daly, J. P., Tyler, T., & Martin, C. (1997). When trust matters: The moderating effect of outcome favorability. Administrative Science Quarterly, 42(3), 558. https://doi.org/10.2307/2393738
- Burke, C. S., Sims, D. E., Lazzara, E. H., & Salas, E. (2007). Trust in leadership: A multi-level review and integration. The Leadership Quarterly, 18(6), 606–632. https://doi.org/10.1016/j.leaqua.2007.09.006
- Castelo, N., Bos, M. W., & Lehmann, D. R. (2019). Task-dependent algorithm aversion. Journal of Marketing Research, 56(5), 809–825. https://doi.org/10.1177/0022243719851788
- Dirks, K. T., & Ferrin, D. L. (2002). Trust in leadership: Meta-analytic findings and implications for research and practice. The Journal of Applied Psychology, 87(4), 611–628. https://doi.org/10.1037/0021-9010.87.4.611
- Duggan, J., Sherman, U., Carbery, R., & McDonnell, A. (2019). Algorithmic management and app-work in the gig economy: A research agenda for employment relations and HRM. Human Resource Management Journal, 30(1), 114–132. https://doi.org/10.1111/1748-8583.12258
- Eifler, S. (2007). Evaluating the validity of self-reported deviant behavior using vignette analyses. Quality & Quantity, 41(2), 303–318. https://doi.org/10.1007/s11135-007-9093-3
- Faul, F., Erdfelder, E., Lang, A. G., & Buchner, A. (2007). G*power 3: A flexible statistical power analysis program for the social, behavioral, and biomedical sciences. Behavior Research Methods, 39(2), 175–191. https://doi.org/10.3758/BF03193146
- Geiskkovitch, D. Y., Cormier, D., Seo, S. H., & Young, J. E. (2016). Please continue, we need more data: An exploration of obedience to robots. Journal of Human-Robot Interaction, 5(1), 82–99. https://doi.org/10.5898/JHRI.5.1.Geiskkovitch
- Georgiou, K. (2021). Can explanations improve applicant reactions towards gamified assessment methods? International Journal of Selection and Assessment, 29(2), 253–268. https://doi.org/10.1111/ijsa.12329
- Gilliland, S. W. (1993). The perceived fairness of selection systems: An organizational justice perspective. Academy of Management Review, 18(4), 694–734. https://doi.org/10.5465/amr.1993.9402210155
- Grzymek, V., & Puntschuh, M. (2019). Was Europa über Algorithmen weiß und denkt: Ergebnisse einer repräsentativen Bevölkerungsumfrage [What Europe knows and thinks about algorithms: Results of a representative survey]. Bertelsmann Stiftung. https://doi.org/10.11586/2019006
- Gunning, D., Stefik, M., Choi, J., Miller, T., Stumpf, S., & Yang, G. Z. (2019). XAI - explainable artificial intelligence. Science Robotics, 4(37), eaay7120. https://doi.org/10.1126/scirobotics.aay7120
- Jarrahi, M. H., Newlands, G., Lee, M. K., Wolf, C. T., Kinder, E., & Sutherland, W. (2021). Algorithmic management in a work context. Big Data & Society, 8(2). https://doi.org/10.1177/20539517211020332
- Langer, M., Baum, K., König, C. J., Hähne, V., Oster, D., & Speith, T. (2021). Spare me the details: How the type of information about automated interviews influences applicant reactions. International Journal of Selection and Assessment, 29(2), 154–169. https://doi.org/10.1111/ijsa.12325
- Langer, M., König, C. J., & Andromachi, F. (2018). Information as a double-edged sword: The role of computer experience and information on applicant reactions towards novel technologies for personnel selection. Computers in Human Behavior, 81, 19–30. https://doi.org/10.1016/j.chb.2017.11.036
- Langer, M., & Landers, R. N. (2021). The future of artificial intelligence at work: A review on effects of decision automation and augmentation on workers targeted by algorithms and third-party observers. Computers in Human Behavior, 123, 106878. https://doi.org/10.1016/j.chb.2021.106878
- Langer, M., Oster, D., Speith, T., Hermanns, H., Kästner, L., Schmidt, E., Sesing, A., & Baum, K. (2021). What do we want from explainable artificial intelligence (XAI)? – a stakeholder perspective on XAI and a conceptual model guiding interdisciplinary XAI research. Artificial Intelligence, 296, 103473. https://doi.org/10.1016/j.artint.2021.103473
- Lee, M. K. (2018). Understanding perception of algorithmic decisions: Fairness, trust, and emotion in response to algorithmic management. Big Data & Society, 5(1), 205395171875668. https://doi.org/10.1177/2053951718756684
- Liepmann, D., Beauducel, A., Brocke, B., & Amthauer, R. (2007). Intelligenz-struktur-test 2000 R (2nd ed.). Hogrefe.
- Mayring, P. (2014). Qualitative content analysis: Theoretical foundation, basic procedures and software solution. SSOAR. https://nbn-resolving.org/urn:nbn:de:0168-ssoar-395173
- Mirowska, A., & Mesnet, L. (2021). Preferring the devil you know: Potential applicant reactions to artificial intelligence evaluation of interviews. Human Resource Management Journal. https://doi.org/10.1111/1748-8583.12393
- Nagtegaal, R. (2021). The impact of using algorithms for managerial decisions on public employees’ procedural justice. Government Information Quarterly, 38(1), 101536. https://doi.org/10.1016/j.giq.2020.101536
- Newman, D. T., Fast, N. J., & Harmon, D. J. (2020). When eliminating bias isn’t fair: Algorithmic reductionism and procedural justice in human resource decisions. Organizational Behavior and Human Decision Processes, 160, 149–167. https://doi.org/10.1016/j.obhdp.2020.03.008
- Ötting, S. K., & Maier, G. W. (2018). The importance of procedural justice in human-machine interactions: Intelligent systems as new decision agents in organizations. Computers in Human Behavior, 89, 27–39. https://doi.org/10.1016/j.chb.2018.07.022
- Parent-Rocheleau, X., & Parker, S. K. (2021). Algorithms as work designers: How algorithmic management influences the design of jobs. Human Resource Management Review, 100838. https://doi.org/10.1016/j.hrmr.2021.100838
- Parry, K., Cohen, M., & Bhattacharya, S. (2016). Rise of the machines: A critical consideration of automated leadership decision making in organizations. Group & Organization Management, 41(5), 571–594. https://doi.org/10.1177/1059601116643442
- Schaefer, K. E., Chen, J. Y., Szalma, J. L., & Hancock, P. A. (2016). A meta-analysis of factors influencing the development of trust in automation: Implications for understanding autonomy in future systems. Human Factors, 58(3), 377–400. https://doi.org/10.1177/0018720816634228
- Truxillo, D. M., Bodner, T. E., Bertolino, M., Bauer, T. N., & Yonce, C. A. (2009). Effects of explanations on applicant reactions: A meta-analytic review. International Journal of Selection and Assessment, 17(4), 346–361. https://doi.org/10.1111/j.1468-2389.2009.00478.x
- Wesche, J. S., & Sonderegger, A. (2019). When computers take the lead: The automation of leadership. Computers in Human Behavior, 101, 197–209. https://doi.org/10.1016/j.chb.2019.07.027
- Wesche, J. S., & Sonderegger, A. (2021). Repelled at first sight? Expectations and intentions of job-seekers reading about AI selection in job advertisements. Computers in Human Behavior, 125, 106931. https://doi.org/10.1016/j.chb.2021.106931
- Zerilli, J., Knott, A., Maclaurin, J., & Gavaghan, C. (2018). Transparency in algorithmic and human decision-making: Is there a double standard? Philosophy & Technology, 32(4), 661–683. https://doi.org/10.1007/s13347-018-0330-6