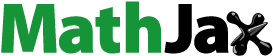
ABSTRACT
In this study, we analyse how beliefs concerning environmental action influence choices in a stated preference survey on coastal water quality and marine habitat areas. Survey respondents were presented with a series of choice tasks featuring improvements in water clarity, reef health, seagrass area and a series of costs in the form of reallocated state budget against a baseline of no new improvements and no change in the budget. The reallocated budget scenario was used as it is likely to be realistic to respondents. Estimates of willingness to reallocate were obtained using a hybrid choice model which included the choice data, attitudinal data regarding support for environmental action and sociodemographic characteristics. Our results suggest that beliefs supporting environmental action influence the willingness to reallocate for attributes that change coastal water quality. A decision support tool for policy analysts was developed to illustrate how public support, as a probability of supporting reallocation, is shaped by beliefs and changes with sociodemographic characteristics.
Introduction
Coastal ecosystems are coming under increasing pressure from human development. Pollution generated by human activity may emanate from diffuse sources in urban areas (e.g. stormwater runoff) or point-sources (e.g. sewage treatment plants, desalination plants) with impacts on water quality, water clarity and seagrass health. In peri-urban areas or rural coastal catchments, the pressures may come from agriculture, aquaculture, or energy generation (Halpern et al. Citation2007). These pressures impact the health of coastal reef systems (Bennett et al. Citation2015) and when compounded with rising water temperatures, shifts in ocean currents, movement of invasive species (Layton et al. Citation2020), there can be spatially extensive impacts on coastal ecosystems (Kenchington and Day Citation2011). These coastal ecosystems are placed further at risk of infrequent, extreme weather events with further loss of coastal seagrass and beach erosion (Gravois et al. Citation2016).
Managing coastal waters and complex coastal ecosystems may require a shifting emphasis in urban water management and urban development. Wu, Keremane, and McKay (Citation2016) point out the importance of considering social and institutional factors in urban development as part of integrated environmental management. Assessments of coastal ecosystems can highlight how broader approaches to coastal management can emphasise the ecological resilience of the system, as well as reduce institutional segmentation (Elliott Citation2014; Griffiths, Connolly, and Brown Citation2020). This fuller evaluation of options may further benefit from the incorporation of individual attitudes, beliefs and values in addition to the consideration of the financial costs and benefits of alternate restoration options.
The aim of this study is to explore whether beliefs related to environmental action influence choices expressed in a stated preference survey. As well, we demonstrate how accounting for these beliefs in the econometric modelling can be used in the calculation of the probability of individuals supporting a package of environmental improvements. Our interest in individual attitudes and related beliefs stems from the role that psychological factors may have in explaining choices made when evaluating alternative goods or policies (Kim, Rasouli, and Timmermans Citation2014). Previous studies have linked respondent’s motivations in stated preference studies to responses with their stated environmental attitudes (Kotchen and Reiling Citation2000; Martín-López, Montes, and Benayas Citation2007). A study by Faccioli et al. (Citation2020) highlights the importance of environmental attitudes as a determinant of the willingness to pay for policies that facilitate peatland restoration. In this study we investigate the willingness to reallocate public funds, the role of beliefs and attitudes and the acceptability of policies that impact coastal water quality and habitat improvements. We present a hybrid choice model which integrates a discrete choice model on how a state government budget in Australia might be re-allocated with a latent variable model of beliefs regarding environmental action.
Hybrid choice models are complex due to the simultaneous estimation of the various components and might not be immediately intuitive. To overcome this issue, we have developed a decision support tool that utilises the model estimates as part of a practical aid to marine policy planners. The approach could be adapted and used in other applications. This tool, in Excel format, allows the user to vary the level of restoration (i.e. adopted policies/improvements), check acceptability as well as compute the budget that the population would support reallocating to improve coastal environs from other public goods.
In the next sections, we briefly outline the background literature on: stated preference surveys; how budgets are prepared by state governments; and hybrid choice models, with a focus on incorporating environmental attitudes. The section on methods describes the design of the online survey employed to collect the empirical data as well as a conceptual description of the hybrid choice model (details of the econometric model are included in the supplementary data). The results of the hybrid choice model are then presented. Our decision support tool is then presented as part of the discussion to illustrate how the model results can be interpreted for policy analysis.
Background
Across a number of fields, stated preference surveys have encompassed a range of techniques and have been used to investigate willingness to pay for new or improved characteristics or attributes of private or public goods. Early applications in transportation include Davidson (Citation1973) and Louviere and Woodworth (Citation1983) in product marketing. Early environmental applications in Australia largely employed contingent valuation type approaches, such as iterative bidding (Majid, Sinden, and Randall Citation1983), direct questioning or opened-ended valuation (Bennett Citation1984), or voluntary contributions to a trust fund (Lockwood, Loomis, and DeLacy Citation1993; Sinden Citation1988) to construct a willingness to pay measure. Later Australian contingent valuation studies considered a range of research questions in an Australian context such as the potential payment vehicle bias (Morrison, Blamey, and Bennett Citation2000), yea-saying (Blamey, Bennett, and Morrison Citation1999) and the role of trust and responsibility (Blamey Citation1998).
Discrete choice experiments in environmental economics emerged in the 1990s onwards with applications such as Adamowicz et al. (Citation1998) and Hanley, Mourato, and Wright (Citation2001). In these applications, respondents are typically asked to choose amongst a series of improvements in environmental quality with varying costs, often comparing against a baseline. Australian environmental applications have considered a wide range of ecosystem types and public policy applications including tropical rainforest preservation (Rolfe, Bennett, and Louviere Citation2000); major rivers (Hatton MacDonald et al. Citation2011; Morrison and Bennett Citation2004); urban water restrictions (Cooper, Burton, and Crase Citation2011), beach management (Graham et al. Citation2020; Windle and Rolfe Citation2014) and marine ecosystems (Davis et al. Citation2019). The breadth of work provides an indication of the flexibility of the stated preference approach for constructing estimates of the benefits of environmental restoration.
In these applications, researchers are seeking to provide estimates of willingness to pay (e.g. implicit prices, or in some cases welfare estimates) for different environmental quality improvements and many seek to explore specific methodological questions while adhering to the current guidance (e.g. Johnston et al. Citation2017; Mariel, Meyerhoff, and Hess Citation2015). One issue is the potential realism of the survey instruments. To be meaningful to respondents, the respondent should view the payment vehicle as realistic. To this end, the scenario presented in the survey should account for the local context and the way public goods are actually decided upon and paid for within the jurisdiction. Rogers et al. (Citation2020) explored how experts and a public sample consider budget reallocation versus a personal-cost mechanism, and identified differences in mean preferences across groups. This latter study suggests that a respondent’s preferences for a program are linked to whether the payment vehicle aligns with how policies are actually funded by local jurisdictions.
Paying for public goods in the Australian context
The ability to make laws on taxation sits with the Commonwealth government according to the Australian constitution s.51(ii), setting out exclusive powers (customs and excise), concurrent powers (income tax) and residual powers (Commonwealth of Australia Citation1900). Periodically state and local governments do impose various taxes. The bulk of taxes have been largely imposed and collected by the Commonwealth government since World War II (Parliament of Australia Citation2022). As a result, there is a mismatch between the jurisdiction that delivers a public good or service and the level of government which collects the tax revenue such that a vertical fiscal imbalance emerges (Parliament of Australia Citation2022). For example, from 2014–2015 to 2019–2020, the Commonwealth collected approximately 80 per cent of the taxation revenue; state governments collected 15–17 per cent of tax revenue with the remainder being local government or shared jurisdiction (Australian Bureau of Statistics Citation2021). State governments are often responsible for delivering on-the-ground programs e.g. natural resource management programs. These programs are funded by state tax revenue as well as transfers from the Commonwealth government which range from 30 to 70 per cent (Productivity Commission Citation2017, 8).
As the potential for budget allocation is central to this article, it is useful to review how budgets are typically prepared. Budgets, at the state and Commonwealth level in Australia, generally begin with a strategic planning process at the cabinet level to set out the priorities and fiscal principles. Initially central agencies, such as Treasury and Finance, provide estimates of expenditures and revenues based on ‘no-policy change’ in the overall budget envelope. Government departments prepare their budget submissions based on the current delivery of services as well as any new proposals. A central committee, usually of cabinet ministers in the state or Commonwealth government, reviews all budget submissions and then decides on the priorities to arrive at the next budget which can involve reallocation between government departments.
Budget re-allocation literature
Previous studies have found that respondents will sometimes object to scenarios with new taxes and prefer that current government revenues instead be used to fund new projects. Compensating budget reallocation (CBR) as a payment vehicle has been used to reallocate existing government budgets to environmental goods and protection of natural resources (or other public goods) which are not currently provided. The concept of budget reallocation as a payment vehicle was first introduced in a contingent valuation setting (Bergstrom, Boyle, and Yabe Citation2004; Kontoleon, Yabe, and Darby Citation2005) and in discrete choice experiments (e.g. see Huang, Andersson, and Zhang Citation2017; Kafle, Swallow, and Smith Citation2015; Morrison and Hatton MacDonald Citation2011; Nunes and Travisi Citation2009; Remoundou et al. Citation2014; Rogers et al. Citation2020). The method does not directly alter a household’s income or commodity prices, as the cost of the environmental (stated) management action is funded through the reallocation of an existing budget (Nunes and Travisi Citation2009). This is important for lower-income households who may be potentially income constrained and as such, estimates based upon additional payments may underrepresent their true preference for improvements in environmental quality (Morrison and Hatton MacDonald Citation2011; Swallow and McGonagle Citation2006). Further, for lower-income households, the environment (and other public goods) are likely to make up a larger proportion of their overall utility, given lower levels of consumption of market goods (Krutilla Citation1967).
Hybrid choice models and pro-environmental attitudes
Discrete choice models represent a widely used technique to elicit how individuals value environmental goods and which aspects shape these values (Ojea and Louriero Citation2007). In addition to instrumental factors (e.g. the number of different species living in a particular environment), individual preferences towards environmental goods/policies/scenarios are also affected by psychological factors, such as attitudes, perceptions and beliefs (Borriello and Rose Citation2021; Hoyos, Mariel, and Hess Citation2015). Hybrid choice models allow the analyst to include latent measures of psychological constructs into the modelling approach, providing greater insights into the determinants of preference heterogeneity. Often these constructs are described as latent as they are not directly observable requiring indirect methods to measure, for example, responses to a set of Likert scale questions. These models have been used in several applications dealing with environmental valuation including water quality (Hess and Beharry-Borg Citation2012), wildlife preservation (Bartczak et al. Citation2015), landscape externalities (Mariel, Meyerhoff, and Hess Citation2015), climate change (Lundhede et al. Citation2015), and ecosystem services (Faccioli et al. Citation2020; Franceschinis et al. Citation2020).
Methods
Sampling and survey design
The survey was designed as a split-sample online survey with two treatments. The survey programming was undertaken by the panel provider Online Research Unit.Footnote1 A stratified random sample was drawn from the online panel based on the age/gender profile of the state of South Australia. Invitations to the panel were sent out in waves in January–February 2013 with up to two follow-up invitations sent to non-respondents. We had a target sample size of 500 respondents per treatment.
In this article, we explore the budget reallocation treatment where respondents saw a multi-part survey including six choice tasks featuring cost as a state budget reallocation. Following the survey introduction and informed consent screens, information on different quality water sources available for outdoor water use was presented to the respondents to aid in thinking about the types of urban water management options available. The relationship between urban water management and coastal ecosystem service outcomes was explained using concise, plain language. Next, the choice tasks with the budget reallocation payment vehicle were presented followed by a set of attitudinal and sociodemographic questions.
Beaches around the Adelaide metropolitan area are generally safe but coastal water quality is affected by stormwater discharge immediately after large rainfall events (South Australia Health Citation2021). However, there are a number of environmental management actions that can be used to improve coastal water quality, including the diversion, treatment and use of recycled wastewater and recycled stormwater. It was explained to respondents that different combinations of projects could help improve water clarity, seagrass habitat area, and the health of reefs. It was highlighted that water clarity would be different across the 40 km coastline due to spatial patterns of rainfall and stormwater drains. It was noted that continuing to dispose of stormwater and wastewater using current practices would result in more seagrass loss, deterioration of reef systems and more days of reduced water clarity.
The definitions of each of the choice attributes were as follows: the number of days each year where the water is murky, the percentage of seagrass remaining, and the number of reef areas in good condition. Each of these attributes was developed through a review of related literature and through consultation with experts in marine biology and stormwater monitoring.
Study respondents were presented with the cost of the program being covered through a reallocation of the State government budget. Moving $1 million in a year was described in terms of the following:
Public law and order will result in: seven fewer police officers, or
Health will result in: two fewer hospital beds available, or
Education will result in: eight fewer teachers, or
Transportation will result in: two km of streets not being resurfaced or
Housing and Community in: 83 fewer public housing units subsidies in a year.
The survey text then described how it would be difficult to take money from only one area and therefore an equal amount would be taken from all these areas. presents the information on the payment vehicle provided in the survey. While a reallocation of a public budget would reduce the provision of public goods associated with key budget categories (health, education, transportation etc.), there is always the possibility that some respondents would perceive there to be limited or no consequences to themselves. This would be a form of yea-saying which is a risk in any stated preference study (Blamey, Bennett, and Morrison Citation1999). To combat this, we use a cheap talk script (Morrison and Brown Citation2009) to remind respondents of the importance of truthful responses.
Respondents were then presented with a choice task and asked to select their preferred option amongst three options (options A – current situation, B and C) in six different choice tasks, as shown in . Respondents were then asked a series of debriefing questions on whether they understood the information in the survey. Attitudinal questions eliciting beliefs about environmental action (Ryan and Spash Citation2012) and on water conservation (Leonard et al. Citation2014) were presented to the respondents. These questions were presented as statements, for which respondents were asked to state the level of agreement/disagreement using a five- or seven-point Likert scale (1 indicating strongly disagree, and 5/7 indicating strongly agree).Footnote2 Finally, a series of questions on sociodemographic characteristics of the respondent were asked.
Experimental design
The attributes and levels were initially laid out in choice tasks according to a utility-balanced efficient design for the pilot testing. A small pilot based on 56 respondents was used to generate parameter priors for the reallocation treatment. The final design was created in NGENE (version 1.1.2) minimising the D-error (Scarpa and Rose Citation2008). The final Bayesian efficient design for the budget reallocation treatment had 60 choice tasks, split into 10 blocks. The final design had a D-error of 0.000702.
Econometric overview
A Hybrid Choice Model (HCM) was used to investigate the impact that beliefs towards the environment might have on preferences for improving coastal waters. The HCM framework (e.g. Ben-Akiva et al. Citation2002; McFadden Citation1986) combines a structural equation model, which defines how the sociodemographic characteristics of a respondent predict the probability of providing specific responses to sets of Likert scale responses, with a classic discrete choice model, through which the analyst derives a measure of preferences from the sample. The HCM model was estimated using Python Biogeme (Bierlaire Citation2016) with code adapted from Rose and Zhang (Citation2017).
The intuition behind the integration of these two components is summarised in . Our justification for taking this modelling approach was based on the hypothesis that respondent’s attitudes and beliefs regarding environmental action are correlated with their preference for environmental changes as offered in each choice task.Footnote3 Using the terminology employed by McFadden (Citation1986) we are treating the belief as a generalised attitude, which can be linked to attitude inventories and preferences. By accounting for beliefs associated with environmental action, issues such as omitted variable bias as well as measurement error can be reduced (e.g. see Vij and Walker [Citation2016] on hybrid models).
To provide some intuition about the various components of the HCM, we begin with the choice model estimated as a mixed logit model with an error component with full correlation. The mixed logit specification accounts for heterogeneity in preferences and the error component allows for a flexible substitution pattern between the current situation – option A and the other two options. Further, the choice model is estimated directly in Willingness to Reallocate (WTR) space which allows the estimated coefficients, specifically the ratio of each attribute and cost, to be interpreted as millions of dollars in state budget reallocated to these restoration efforts. The latent variables are then included as an interaction term in the hybrid choice model. The detailed econometric specifications can be found in the supplementary data The Cholesky matrix is available from the authors on request.
There are three parameters that are central to the aims of this study. First, the mean parameter of each non-cost attribute is the estimated WTR for the attribute when the latent variable is zero (i.e. an individual holds weak beliefs in environmental action). The estimated coefficient on the interaction term between the respondent’s environmental beliefs and the choice task attributes provides a measure of the influence of beliefs on WTR. Finally, the standard deviation parameters indicate whether there is heterogeneity in the WTR attribute.
The other component of the hybrid model is the measurement equations that model the latent variable as a predictor for the various attitudinal questions. The latent variable is a function of several sociodemographic characteristics including age (continuous), gender (dummy variable = 1 for female), low income (dummy variable = 1 if household income less than $400 a week), education (several dummy variables = 1 based on stated highest education level), and whether a respondent actively interacts with coastal waters (dummy variable = 1 if respondent either dives, surfs, fishes, or swims in coastal waters). It is postulated that different sociodemographic characteristics explain differences in response patterns to each attitudinal question, in effect modelling differences in the strength in beliefs towards environmental action. At the same time, these characteristics are also postulated to explain differences in WTR for each of the policy attributes modelled.
Following the estimation of the HCM, a decision support tool is developed to demonstrate how the model can be used for policy analysis. The tool utilises the parameter estimates as well as known population characteristics to determine the probability that a specific policy will be accepted. Specifically, alternative attribute levels can be inputted to determine the probability that the population supports reallocation. Data raking was applied to create weightings based on known population characteristics (Battaglia, Hoaglin, and Frankel Citation2009).
Results
In total 506 responses were collected, representing a response rate of 18.9 per cent. Based on the sample characteristics reported in there was an oversampling of older respondents, female respondents, respondents with more educational qualifications as well as higher income relative to census data for the greater Adelaide area.Footnote4
Table 1. Sample characteristics.
The factor analysis led to one factor being identified, with the correlation between the component and the individual statements shown in . The factor identified, labelled ENV, represents attitudes regarding the benefits of environmental action. This factor explains 63.90 per cent of the variation across the nine statements. An internal reliability test was performed with the reported Cronbach alpha (0.926) suggesting a high level of reliability. Based on these results, all nine statements were included in the hybrid choice model as measurement indicators of the latent variable. The individual factor loadings are different from those reported by Ryan and Spash (Citation2012). However, the statement items together do match the factor they report as ‘beliefs supportive of environmental action’.
Table 2. Factor analysis for ENV latent variable.
The next set of results relates to the structural equations for the latent variable identified in the factor analysis. reports the parameter estimates that measure the relationship between different sociodemographic characteristics and the latent variable. The coefficients associated with low income and having a diploma educational qualification are not statistically significant. The coefficient on having an undergraduate degree is statistically significant at 10 per cent, whilst the remaining ones are statistically significant at 1 per cent. These coefficients suggest a number of positive relationships with the latent variable e.g. an older female respondent with a postgraduate education who interacts with the water will be most likely to have a strong positive attitude towards taking environmental action. Conversely, a young male with a high school level of education who does not interact with the ocean is less likely to have a positive attitude towards environmental action.
Table 3. Coefficients for structural and measurement equations.
These results are similar to those reported in previous studies looking at the correlation between various pro-environmental domains and sociodemographic characteristics. Previous studies suggest that women tend to be more likely to agree with pro-environmental statements as measured through scales (Liu et al. Citation2019; McMillan et al. Citation1997). There is also some evidence in past studies that environmental knowledge is linked to pro-environmental attitudes (Liobikienė and Poškus Citation2019).
The estimated coefficients for the structural equation are used to simulate the latent variable for each respondent. This makes it possible to identify groups of respondents, as defined by their sociodemographic profile, which is more likely to have a positive WTR for each of the attributes. shows the parameters estimated through the Mixed Logit (ML) and Hybrid Mixed Logit (HML) models. All the parameters in the ML are significant except the standard deviation of the seagrass attribute. For the HML the mean attributes for water clarity, seagrass, and reefs are statistically insignificant, however, the interaction parameters and standard deviations are significant. The mean parameter for each choice attribute is interpreted as the estimated WTR when the latent variable is equal to zero.Footnote5 Compared to the ML, the standard deviation parameters in the HML are all relatively smaller for every attribute excluding cost and seagrass. This result suggests that some of the unobserved heterogeneity at the individual level has been accounted for in the interaction terms in the model. It is important to note that, although the mean parameter estimates for the water clarity and reef attributes are negative, the WTR is not, as the interactions with the latent attitude ENV should be included in the calculation. As a matter of example, the average WTR for reefs is given by:
where dr is a normally distributed random draw included to account for the random parameter structure. The results of these calculations are reported at the bottom of , as well as the per person amount so as to be comparable with prior studies.
Table 4. Hybrid choice model results.
Discussion
The results of the choice model suggest that there are differences in WTR based on the different features of coastal waters. This result is not surprising given the different ecosystem services that these features may be providing to people. This variation in WTP estimates is in line with previous studies focusing on the value of water quality particularly when distinguishing between the use and non-use values. Wielgus et al. (Citation2003) estimated that divers in the United States were WTP $1.20 USD ($2.84 AUD)Footnote6 per dive to increase water visibility. Thomas and Stratis (Citation2002) and Lipton (Citation2004) identified that boat users were WTP on average $423.94 USD ($1107.16 AUD) and $54.68 USD ($122.99 AUD) per annum in additional taxes to improve local water quality, the difference between these figures varying by the degree of water use for each group of respondents. Other studies have focused on the valuation of the ecosystem services that lead to improved water quality. For example, Atkins and Burdon (Citation2006) found that Danish households were willing to pay €12.02 ($28.12 AUD) per year for 10 years to fund programs which lead to reduced nutrient inputs, increasing over time the water quality of the Randers Fjord.
Seagrass was presented to respondents as a fish nursery and habitat for a wide array of marine life. Previous valuations of seagrass nurseries have estimated the fish commercial value of seagrass areas, with McArthur and Boland (Citation2006) estimating the economic contribution of seagrass in South Australia being worth $114 million ($169 million in 2022) AUD per year. Unsworth et al. (Citation2010) calculate the mean value of unmanaged seagrass meadows in Indonesia as equal to $77.9 USD ($169.43 AUD) per hectare.
A decision support tool has been created to demonstrate how the model results translate into potential societal support of different restoration levels. A screen capture of the tool is presented in . The tool [https://www.marktocock.com/dst-how-environmental-beliefs-influence-the-acceptance-of-real] has been designed to demonstrate how the results of this study can be used to assess support for different policies which lead to environmental improvements. The outputs for the model, specifically the WTR values, can be used as inputs for other models exploring the trade-offs within larger allocation models, as suggested by Mallawaarachchi et al. (Citation2001), or for determining the degree of policy acceptability as demonstrated below.
Using the model results and sociodemographic characteristics of the sample, the WTR of the sample is simulated based on the values of the policy attributes that can be set by the user. From these individual values, an unweighted and weighted average WTR is calculated and summed over a five-year period (the discount rate can also be changed). In addition to the total WTR, the proportion of the population who would vote for and against the policy is calculated and reported. shows an example of one policy evaluated. Based on the inputted policy attributes and a discount rate of 4.00 per cent, the WTR over five years is equal to $42.80 million, with an acceptance rate of 44.73 per cent.
Table 5. Decision support tool example analysis.
Our example in part highlights how sociodemographic information can be used to provide richer insights into the acceptability of different policies. Our approach seeks a similar goal to that of Pakalniete et al. (Citation2017). Their modelling approach involved identifying independent latent factors based on different combinations of sociodemographic characteristics. The latent factors were labelled as different members of society, such as students and pensioners. They found that the WTP for various policy interventions, for example, improvements in water quality, varied widely between groups with some having strictly positive WTP at the 95 per cent level of significance. While we have a similar finding, we highlight that sociodemographic characteristics, but more importantly latent beliefs about the importance of environmental action, are important determinants of our results.
Conclusion
Managing coastal ecosystems requires developing robust policy responses to mitigate the effects of continual urban development as well as a changing climate. Discrete choice models are frequently utilised to estimate the value of alternative policies, however, there is often significant heterogeneity with the estimated values that goes unexplained. The aim of this study was to explore whether beliefs towards environmental action can explain differences in the willingness to reallocate public funds towards coastal ecosystem management. Our results suggest that policymakers and planners could benefit from accounting for individual beliefs when evaluating alternative policies. To demonstrate this extension, we have developed a decision-support tool to show how the results can be used to provide information on the probability that proposed policies will be supported. We believe that consolidating the results into a decision support tool could help eliminate one of the barriers preventing such models from being incorporated into policy analysis.
In every study, there are limitations which may provide avenues for future research. In this study, we used a particular set of psychometric scales to measure beliefs and attitudes to environmental action. For other case study sites and environmental management problems, there will be opportunities to utilise different psychometric scales and expand the set of hybrid choice model applications. Similarly, other instrumental factors and latent attitudes that influence respondents’ use and non-use values could be included in the modelling. Further, the model results do not reflect updated preferences resulting from the experience of living through more extreme weather events. Also, it should be acknowledged that the costs to implement changes in the attribute levels, for example increasing the number of reefs, may now be understated. Consequently, the payment vehicle amounts may need to be updated to reflect these higher costs.
While a budget reallocation mechanism is realistic, future research could explore different payment vehicles as well as budget reallocation mechanisms that account for respondents’ beliefs regarding whether they feel they are impacted by alternative payment vehicles. In this study, respondents were told the reallocated amounts would be taken equally from the various budget categories. Future studies may wish to explore whether a respondent’s sensitivity to the payment vehicle is conditional on whether the funds are taken from specific categories, for example reallocating funds from the health budget versus the education budget.
Supplemental Material
Download PDF (362.2 KB)Decision Support Tool Final.xlsm
Download (85.6 KB)Disclosure statement
No potential conflict of interest was reported by the author(s).
Notes
2 We used the psychometric scales from Leonard et al. (Citation2014) and a subset of the Ryan and Spash (Citation2012) scale that measures beliefs supportive of environmental action.
3 A respondent’s beliefs regarding environmental action are long-held or established beliefs. In contrast, a respondent’s perceptions of the choice task attributes might be shaped by recent experiences, e.g. the perceived benefit of increased water clarity (for more on the issue regarding attitudes/beliefs versus perceptions see Borriello and Rose (Citation2021)).
4 Almost three-quarters of the sample indicated that they had some interaction with coastal waters which would not seem unusual when a large portion (approximately 77% of the state population lives in the greater Adelaide area), in close proximity to Gulf St Vincent. However, we have no reference population data with which to compare.
5 As the latent variable approaches zero, there is a diminishing positive attitude towards environmental action, resulting in a WTR that is statistically insignificant from zero. This means that, for example, for the attribute Water Clarity there will be around 68% of respondents who have a (main effect) WTR within 0 (mean parameter) ±0.488 (standard deviation parameter). Ignoring the role of the standard deviation reduces the quality of the model that attempts at shedding light on the heterogeneity of the WTR within the sample.
6 All original dollar values are converted to 2022 amounts measured in $AUD. A CPI calculator inflated each value based on the year the data was collected for each study. Next, the appropriate average 2022 foreign exchange rate was applied.
References
- Adamowicz, W., P. Boxall, M. Williams, and J. Louviere. 1998. “Stated Preference Approaches for Measuring Passive Use Values: Choice Experiments and Contingent Valuation.” American Journal of Agricultural Economics 80 (1): 64–75. https://doi.org/10.2307/3180269.
- Atkins, J. P., and D. Burdon. 2006. “An Initial Economic Evaluation of Water Quality Improvements in the Randers Fjord, Denmark.” Marine Pollution Bulletin 53 (1-4): 195–204. https://doi.org/10.1016/j.marpolbul.2005.09.024.
- Australian Bureau of Statistics. 2011. “2011 Greater Adelaide, Census All Persons QuickStats.” Dataset. Accessed December 12, 2022. https://quickstats.censusdata.abs.gov.au/census_services/getproduct/census/2011/quickstat/4GADE#:~:text=In%20the%202011%20Census%2C%20there%20were%201%2C225%2C235%20people,%28Greater%20Capital%20City%20Statistical%20Areas%29%20was%2039%20years.
- Australian Bureau of Statistics. 2021. “Taxation Revenue, Australia, Statistics About Taxation Revenue Collected by the Various Levels of Government in Australia, 2019–20 Financial Year.” Dataset. Accessed September 26, 2022. https://www.abs.gov.au/statistics/economy/government/taxation-revenue-australia/latest-release accessed 29/10/2021.
- Bartczak, A., P. Mariel, S. Chilton, and J. Meyerhoff. 2015. “The Impact of Latent Risk Preferences on Valuing the Preservation of Threatened Lynx Populations in Poland.” Australian Journal of Agricultural and Resource Economics 60 (2): 284–306. https://doi.org/10.1111/1467-8489.12123.
- Battaglia, M. P., D. C. Hoaglin, and M. R. Frankel. 2009. “Practical Considerations in Raking Survey Data.” Survey Practice 2 (5): 1–10. https://doi.org/10.29115/SP-2009-0019.
- Ben-Akiva, M., D. McFadden, K. Train, J. Walker, C. Bhat, M. Bierlaire, D. Bolduc, et al. 2002. “Hybrid Choice Models: Progress and Challenges.” Marketing Letters 13 (3): 163–175. https://doi.org/10.1023/A:1020254301302.
- Bennett, J. W. 1984. “Using Direct Questioning to Value the Existence Benefits of Preserved National Areas.” Australian Journal of Agricultural Economics 28 (2-3): 136–152. https://doi.org/10.1111/j.1467-8489.1984.tb00644.x.
- Bennett, S., S. Thomas Wernberg, D. Connell, A. J. Hobday, C. R. Johnson, and E. S. Poloczanska. 2015. “The ‘Great Southern Reef’: Social, Ecological and Economic Value of Australia’s Neglected Kelp Forests.” Marine and Freshwater Research 67: 47–56. https://doi.org/10.1071/MF15232.
- Bergstrom, J. C., K. J. Boyle, and M. Yabe. 2004. “Trading Taxes vs. Paying Taxes to Value and Finance Public Environmental Goods.” Environmental & Resource Economics 28 (4): 533–549. https://doi.org/10.1023/B:EARE.0000036779.58923.02.
- Bierlaire, M. 2016. “Python Biogeme: A Short Introduction.” Report TRANSP-OR 160706, Series on Biogeme. Transport and Mobility Laboratory, School of Architecture, Civil and Environmental Engineering. Ecole Polytechnique Fédérale de Lausanne, Switzerland. https://transp-or.epfl.ch/documents/technicalReports/Bier16a.pdf.
- Blamey, R. K. 1998. “Trust, Responsibility and the Interpretation of Contingent Valuation Results.” Australian Economic Papers 37 (3): 273–291. https://doi.org/10.1111/1467-8454.00020.
- Blamey, R. K., J. W. Bennett, and M. D. Morrison. 1999. “Yea-saying in Contingent Valuation Surveys.” Land Economics 75 (1): 126–141. https://doi.org/10.2307/3146997.
- Borriello, A., and J. M. Rose. 2021. “Global Versus Localised Attitudinal Responses in Discrete Choice.” Transportation 48 (1): 131–165. https://doi.org/10.1007/s11116-019-10045-3.
- Commonwealth of Australia. 1900. “Commonwealth of Australia Constitution Act 1900.” https://www.aph.gov.au/constitution.
- Cooper, B., M. Burton, and L. Crase. 2011. “Urban Water Restrictions: Attitudes and Avoidance.” Water Resources Research 47 (12): 1–14. https://doi.org/10.1029/2010WR010226.
- Davidson, J. D. 1973. “Forecasting Traffic on STOL.” Journal of the Operational Research Society 24 (4): 561–569. https://doi.org/10.1057/jors.1973.105.
- Davis, K. J., M. Burton, A. Rogers, A. Spencer-Cotton, and R. Pandit. 2019. “Eliciting Public Values for Management of Complex Marine Systems: An Integrated Choice Experiment.” Marine Resource Economics 34 (1): 1–21. https://doi.org/10.1086/701303.
- Elliott, M. 2014. “Integrated Marine Science and Management: Wading Through the Morass.” Marine Pollution Bulletin 86 (1-2): 1–4. https://doi.org/10.1016/j.marpolbul.2014.07.026.
- Faccioli, M., M. Czajkowski, K. Glenk, and J. Martin-Ortega. 2020. “Environmental Attitudes and Place Identity as Determinants of Preferences for Ecosystem Services.” Ecological Economics 174: 106600. https://doi.org/10.1016/j.ecolecon.2020.106600.
- Franceschinis, C., U. Liebe, M. Thiene, J. Meyerhoff, D. Field, and A. McBratney. 2020. “The Effect of Social and Personal Norms on Stated Preferences for Multiple Soil Functions: Evidence from Australia and Italy.” Australian Journal of Agricultural and Resource Economics 66 (2): 335–362. https://doi.org/10.1111/1467-8489.12466.
- Graham, V., C. S. Fleming, F. Agimass, and J. C. R. Smart. 2020. “Preferences for the Future of the Southport Spit: Evidence from a Choice Experiment.” Australasian Journal of Environmental Management 27 (4): 396–414. https://doi.org/10.1080/14486563.2020.1843193.
- Gravois, U., D. Callaghan, T. Baldock, K. Smith, and B. Martin. 2016. “Review of Beach Profile and Shoreline Models Applicable to the Statistical Modelling of Beach Erosion and the Impacts of Storm Clustering.” Bushfire and Natural Hazards CRC Report. https://espace.library.uq.edu.au/view/UQ:499750.
- Griffiths, L. L., R. M. Connolly, and C. J. Brown. 2020. “Critical Gaps in Seagrass Protection Reveal the Need to Address Multiple Pressures and Cumulative Impacts.” Ocean & Coastal Management 183: 104946. https://doi.org/10.1016/j.ocecoaman.2019.104946.
- Halpern, B. S., K. A. Selkoe, F. Micheli, and C. V. Kappel. 2007. “Evaluating and Ranking the Vulnerability of Global Marine Ecosystems to Anthropogenic Threats.” Conservation Biology 21 (5): 1301–1315. https://doi.org/10.1111/j.1523-1739.2007.00752.x.
- Hanley, N., S. Mourato, and R. E. Wright. 2001. “Choice Modelling Approaches: A Superior Alternative for Environmental Valuatioin?” Journal of Economic Surveys 15 (3): 435–462. https://doi.org/10.1111/1467-6419.00145.
- Hatton MacDonald, D., M. D. Morrison, J. M. Rose, and K. J. Boyle. 2011. “Valuing a Multistate River: The Case of the River Murray.” Australian Journal of Agricultural and Resource Economics 55 (3): 374–392. https://doi.org/10.1111/j.1467-8489.2011.00551.x.
- Hess, S., and N. Beharry-Borg. 2012. “Accounting for Latent Attitudes in Willingness-to-Pay Studies: The Case of Coastal Water Quality Improvements in Tobago.” Environmental and Resource Economics 52 (1): 109–131. https://doi.org/10.1007/s10640-011-9522-6.
- Hoyos, D., P. Mariel, and S. Hess. 2015. “Incorporating Environmental Attitudes in Discrete Choice Models: An Exploration of the Utility of the Awareness of Consequences Scale.” Science of the Total Environment 505: 1100–1111. https://doi.org/10.1016/j.scitotenv.2014.10.066.
- Huang, D., H. Andersson, and S. Zhang. 2017. “Willingness to Pay to Reduce Health Risks Related to Air Quality: Evidence from a Choice Experiment Survey in Beijing.” Journal of Environmental Planning and Management 61 (12): 2207–2229. https://doi.org/10.1080/09640568.2017.1389701.
- Johnston, R. J., K. J. Boyle, W. Adamowicz, J. Bennett, R. Brouwer, T. A. Cameron, W. M. Hanemann, et al. 2017. “Contemporary Guidance for Stated Preference Studies.” Journal of the Association of Environmental and Resource Economists 4 (2): 319–405. https://doi.org/10.1086/691697.
- Kafle, A., S. K. Swallow, and E. C. Smith. 2015. “Does Public Funding Affect Preferred Tradeoffs and Crowd-In or Crowd-Out Willingness to Pay? A Watershed Management Case.” Environmental and Resource Economics 60 (3): 471–495. https://doi.org/10.1007/s10640-014-9782-z.
- Kenchington, R. A., and J. C. Day. 2011. “Zoning, a Fundamental Cornerstone of Effective Marine Spatial Planning: Lessons Learnt from the Great Barrier Reef, Australia.” Journal of Coastal Conservation 15 (2): 271–278. https://doi.org/10.1007/s11852-011-0147-2.
- Kim, J., S. Rasouli, and H. Timmermans. 2014. “Expanding Scope of Hybrid Choice Models Allowing for Mixture of Social Influences and Latent Attitudes: Application to Intended Purchase of Electric Cars.” Transportation Research Part A: Policy and Practice 69: 71–85. https://doi.org/10.1016/j.tra.2014.08.016.
- Kontoleon, A., M. Yabe, and L. Darby. 2005. “Alternative Payment Vehicles in Contingent Valuation: The Case of Genetically Modified Foods.” 14th Annual Meeting of the European Association of Environmental and Resource Economists, Bremen, Germany, June 25. https://mpra.ub.uni-muenchen.de/1827/1/MPRA_paper_1827.pdf.
- Kotchen, M. J., and S. D. Reiling. 2000. “Environmental Attitudes, Motivations, and Contingent Valuation of Nonuse Values: A Case Study Involving Endangered Species.” Ecological Economics 32 (1): 93–107. https://doi.org/10.1016/S0921-8009(99)00069-5.
- Krutilla, J. V. 1967. “Conservation Reconsidered.” American Economic Review 57 (4): 777–786. https://www.jstor.org/stable/1815368.
- Layton, C., M. A. Coleman, E. M. Marzinelli, P. D. Steinberg, S. E. Swearer, A. Vergés, T. Wernberg, and C. R. Johnson. 2020. “Kelp Forest Restoration in Australia.” Frontiers in Marine Science 7 Article 74: 1–12. https://doi.org/10.3389/fmars.2020.00074.
- Leonard, R., A. Walton, B. Koth, M. Green, A. Spinks, B. Myers, S. Malkin, et al. 2014. “Community Acceptance of Water Sensitive Urban Design: Six Case Studies.” Goyder Institute for Water Research Technical Report Series No. 14/3. https://publications.csiro.au/rpr/download?pid=csiro:EP14349&dsid=DS2.
- Liobikienė, G., and M. S. Poškus. 2019. “The Importance of Environmental Knowledge for Private and Public Sphere Pro-Environmental Behavior: Modifying the Value-Belief-Norm Theory.” Sustainability 11 (12): 3324. https://doi.org/10.3390/su11123324.
- Lipton, D. 2004. “The Value of Improved Water Quality to Chesapeake Bay Boaters.” Marine Resource Economics 19 (2): 265–270. https://doi.org/10.1086/mre.19.2.42629432.
- Liu, T., L. Geng, L. Ye, and K. Zhou. 2019. “‘Mother Nature’ Enhances Connectedness to Nature and pro-Environmental Behavior.” Journal of Environmental Psychology 61: 37–45. https://doi.org/10.1016/j.jenvp.2018.12.003.
- Lockwood, M., J. B. Loomis, and T. DeLacy. 1993. “A Contingent Valuation Survey and Benefit-Cost Analysis of Forest Preservation in East Gippsland, Australia.” Journal of Environmental Management 38 (3): 233–243. https://doi.org/10.1006/jema.1993.1042.
- Louviere, J. J., and G. Woodworth. 1983. “Design and Analysis of Simulated Consumer Choice or Allocation Experiments: An Approach Based on Aggregate Data.” Journal of Marketing Research 20 (4): 350–367. https://doi.org/10.1177/002224378302000403.
- Lundhede, T., J. B. Jacobsen, N. Hanley, N. Strange, and B. J. Thorsen. 2015. “Incorporating Outcome Uncertainty and Prior Outcome Beliefs in Stated Preferences.” Land Economics 91 (2): 296–316. https://doi.org/10.3368/le.91.2.296.
- Majid, I. T., J. A. Sinden, and A. Randall. 1983. “Benefit Evaluation of Increments to Existing Systems of Public Facilities.” Land Economics 59 (4): 377–392. https://doi.org/10.2307/3145653.
- Mallawaarachchi, T., R. K. Blamey, M. D. Morrison, A. K. L. Johnson, and J. W. Bennett. 2001. “Community Values for Environmental Protection in a Cane Farming Catchment in Northern Australia: A Choice Modelling Study.” Journal of Environmental Management 62 (3): 301–316. https://doi.org/10.1006/jema.2001.0446.
- Mariel, P., J. Meyerhoff, and S. Hess. 2015. “Heterogeneous Preferences Toward Landscape Externalities of Wind Turbines – Combining Choices and Attitudes in a Hybrid Model.” Renewable and Sustainable Energy Reviews 41: 647–657. https://doi.org/10.1016/j.rser.2014.08.074.
- Martín-López, B., C. Montes, and J. Benayas. 2007. “The Non-Economic Motives Behind the Willingness to Pay for Biodiversity Conservation.” Biological Conservation 139 (1-2): 67–82. https://doi.org/10.1016/j.biocon.2007.06.005.
- McArthur, L. C., and J. W. Boland. 2006. “The Economic Contribution of Seagrass to Secondary Production in South Australia.” Ecological Modelling 196 (1-2): 163–172. https://doi.org/10.1016/j.ecolmodel.2006.02.030.
- McFadden, D. 1986. “The Choice Theory Approach to Market Research.” Marketing Science 5 (4): 275–297. https://doi.org/10.1287/mksc.5.4.275.
- McMillan, M., T. J. Hoban, W. B. Clifford, and M. R. Brant. 1997. “Social and Demographic Influences on Environmental Attitudes.” Journal of Rural Social Sciences 13 (1): 91–107. https://egrove.olemiss.edu/jrss/vol13/iss1/5.
- Morrison, M. D., and J. W. Bennett. 2004. “Valuing New South Wales Rivers for Use in Benefit Transfer.” The Australian Journal of Agricultural and Resource Economics 48 (4): 591–611. https://doi.org/10.1111/j.1467-8489.2004.00263.x.
- Morrison, M. D., R. K. Blamey, and J. W. Bennett. 2000. “Minimising Payment Vehicle Bias in Contingent Valuation Studies.” Environmental and Resource Economics 16 (4): 407–422. https://doi.org/10.1023/A:1008368611972.
- Morrison, M. D., and T. C. Brown. 2009. “Testing the Effectiveness of Certainty Scales, Cheap Talk, and Dissonance-Minimization in Reducing Hypothetical Bias in Contingent Valuation Studies.” Environmental and Resource Economics 44 (3): 307–326. https://doi.org/10.1007/s10640-009-9287-3.
- Morrison, M. D., and D. Hatton MacDonald. 2011. “A Comparison of Compensating Surplus and Budget Reallocation with Opportunity Costs Specified.” Applied Economics 43 (30): 4677–4688. https://doi.org/10.1080/00036846.2010.493143.
- Nunes, P. A. L. D., and C. M. Travisi. 2009. “Comparing Tax and Tax Reallocation Payments in Financing Rail Noise Abatement Programmes: Results from a Stated Choice Valuation Study in Italy.” Environmental and Resource Economics 43 (4): 503–517. https://doi.org/10.1007/s10640-008-9243-7.
- Ojea, E., and M. Louriero. 2007. “Altruistic, Egoistic and Biospheric Values in Willingness to Pay (WTP) for Wildlife.” Ecological Economics 63 (4): 807–814. https://doi.org/10.1016/j.ecolecon.2007.02.003.
- Pakalniete, K., J. Aigars, M. Czajkowski, S. Strake, E. Zawojska, and N. Hanley. 2017. “Understanding the Distribution of Economic Benefits from Improving Coastal and Marine Ecosystems.” Science of the Total Environment 584-585: 29–40. https://doi.org/10.1016/j.scitotenv.2017.01.097.
- Parliament of Australia. 2022. “Chapter 4 Vertical Fiscal Imbalance.” https://www.aph.gov.au/Parliamentary_Business/Committees/Senate/Former_Committees/reffed/reffed/report/c04.
- Productivity Commission. 2017. “Commonwealth-State Relations, Shifting the Dial: 5 Year Productivity Review.” Supporting Paper No. 14, Canberra.
- Remoundou, K., F. Adaman, P. Koundouri, and P. A. L. D. Nunes. 2014. “Is the Value of Environmental Goods Sensitive to the Public Funding Scheme? Evidence from a Marine Restoration Programme in the Black Sea.” Empirical Economics 47 (4): 1173–1192. https://doi.org/10.1007/s00181-014-0806-x.
- Rogers, A. A., M. P. Burton, J. A. Cleland, J. C. Rolfe, J. J. Meeuwig, and D. J. Pannell. 2020. “Expert Judgements and Community Values: Preference Heterogeneity for Protecting River Ecology in Western Australia.” Australian Journal of Agricultural and Resource Economics 64 (2): 266–293. https://doi.org/10.1111/1467-8489.12365.
- Rolfe, J., J. Bennett, and J. J. Louviere. 2000. “Choice Modelling and Its Potential Application to Tropical Rainforest Preservation.” Ecological Economics 35 (2): 289–302. https://doi.org/10.1016/S0921-8009(00)00201-9.
- Rose, J. M., and J. Zhang. 2017. “An Introductory Guide to Estimating Discrete Choice Models Using Python Biogeme.”. BIDA Working Paper 17-01. Business Intelligence and Data Analytics Research Centre, University of Technology Sydney.
- Ryan, A. M., and C. L. Spash. 2012. “The Awareness of Consequences Scale: An Exploration, Empirical Analysis, and Reinterpretation.” Journal of Applied Social Psychology 42 (10): 2505–2540. https://doi.org/10.1111/j.1559-1816.2012.00951.x.
- Scarpa, R., and J. M. Rose. 2008. “Design Efficiency for Non-Market Valuation with Choice Modelling: How to Measure It, What to Report and Why.” Australian Journal of Agricultural and Resource Economics 52 (3): 253–282. https://doi.org/10.1111/j.1467-8489.2007.00436.x.
- Sinden, J. A. 1988. “Empirical Tests of Hypothetical Bias in Consumers’ Surplus Surveys.” Australian Journal of Agricultural Economics 32 (2-3): 98–112. https://doi.org/10.22004/ag.econ.22956.
- South Australia Health. 2021. “Beaches, Lakes and Rivers and Being Safe Around Them.” South Australia.
- Swallow, S. K., and M. P. McGonagle. 2006. “Public Funding of Environmental Amenities: Contingent Choices Using New Taxes or Existing Revenues for Coastal Land Conservation.” Land Economics 82 (1): 56–67. https://doi.org/10.3368/le.82.1.56.
- Thomas, M., and N. Stratis. 2002. “Compensating Variation for Recreational Policy: A Random Utility Approach to Boating in Florida.” Marine Resource Economics 17 (1): 23–33. https://doi.org/10.1086/mre.17.1.42629346.
- Unsworth, R. K. F., L. C. Cullen, J. N. Pretty, D. J. Smith, and J. J. Bell. 2010. “Economic and Subsistence Values of the Standing Stocks of Seagrass Fisheries: Potential Benefits of no-Fishing Marine Protected Area Management.” Ocean & Coastal Management 53 (5-6): 218–224. https://doi.org/10.1016/j.ocecoaman.2010.04.002.
- Vij, A., and J. L. Walker. 2016. “How, When and Why Integrated Choice and Latent Variable Models Are Latently Useful.” Transportation Research Part B: Methodological 90: 192–217. https://doi.org/10.1016/j.trb.2016.04.021.
- Wielgus, J., N. E. Chadwick-Furman, N. Zeitouni, and M. Shechter. 2003. “Effects of Coral Reef Attribute Damage on Recreational Welfare.” Marine Resource Economics 18 (3): 225–237. https://doi.org/10.1086/mre.18.3.42629397
- Windle, J., and J. Rolfe. 2014. “Estimating the Nonmarket Economic Benefits of Beach Resource Management in Southeast Queensland, Australia.” Australasian Journal of Environmental Management 21 (1): 65–82. https://doi.org/10.1080/14486563.2013.875953.
- Wu, Z., G. Keremane, and J. McKay. 2016. “Sustainable Urban Water Management Through the Lens of Community – A Photostory Exercise in Metropolitan Adelaide.” Australasian Journal of Environmental Management 23 (2): 141–156. https://doi.org/10.1080/14486563.2015.1084488.