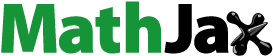
Abstract
We compare two aerosol surrogate tracers in aircraft cabins for breathing and coughing sources: tracer gas collected in the ACER Boeing 767 mock-up and fluorescent particles collected in an actual Boeing 767 aircraft by the US Transportation Command (TRANSCOM). Each source was located individually in window and middle seats. Exposure generally decreased with source distance. A window seat breathing source resulted in good agreement between datasets for exposure (as percent of release) for the TRANSCOM hangar-AFT testing mode, which corresponds to the 11-row cabin ACER laboratory space. Average tracer gas exposure for a middle seat breathing source was higher in the ACER study than the fluorescent particle tracer exposure in the TRANSCOM study. Using a coughing source in a window seat, the exposure for the TRANSCOM data was higher within the first two rows from the source before decreasing to and tracking with the ACER levels, until increasing after about 5 m away. A similar trend was recorded for a middle seat coughing source with higher overall exposure for the TRANSCOM data. Sources of exposure variation between the studies include particle deposition. This work helps optimize aerosol dispersion research in aircraft cabins and provides some validation to the existing studies.
Highlights
Laboratory cabin tracer gas (ACER) and real aircraft tracer particle results (TRANSCOM) differed significantly due to deposition and chaotic airflow patterns.
All individual seat measurements were below 0.3% of total released mass.
Tracer gas measurements were higher than particle results for a breathing source.
Particle results were higher than tracer gas results for a coughing source.
Measurements showed large variability near sources and general decrease with distance.
1. Introduction
The ongoing COVID-19 pandemic led to a 94% plunge in the number of airline passengers in the U.S during the first year of the pandemic due to travel restrictions and the public's uncertainty of aircraft cabin air quality according to Airlines for America (Citation2022). Even after almost two years of the pandemic, the number of flights departing and arriving domestically within the last two weeks of the year 2021 was down by 18% and 15% compared to pre-pandemic levels, respectively. The number of international passengers was also affected with 22% less for departing flights and 28% for arriving flights (Airlines for America, Citation2022). In terms of number of passengers, the total number of passengers for the year fell sharply from 2019, to a level the world’s airports had not seen since 1997 (Airports Council International, Citation2021). Industry experts had believed that the efforts to ensure the safety of all passengers and crews would not start showing impact on the industry recovery before the year 2024 (Moody's Investors Service, Citation2020). However, by the end of 2022, a return to profitability was forecast for 2023 (IATA, Citation2022).
According to Sun et al. (Citation2021), this pandemic has motivated more researchers to investigate disease transmission in aircraft cabins. Aircraft manufacturers, commercial airlines, government entities, and others have directed their efforts to study this topic with various goals. Several authors believe that, given the volume of commercial flights daily, the number of documented incidents of infectious disease transmission on board an aircraft is infrequent (Mangili & Gendreau, Citation2005; APHI, Citation2021). Some authors estimate those transmission incidents of SARS-Cov-2 to be as low as one case per 27 million travelers (Bielecki et al., Citation2021). Yet, even for relatively new diseases such as COVID-19, there have been well-documented flights describing multiple transmission events and over 2000 flights with known cases on board (Bielecki et al., Citation2021).
Various pre-COVID studies pointed to the possibility of disease spread particularly within rows. Many outbreaks of respiratory diseases that generally transmit via aerosols associated with air travel have been reported, and infectious agents transmitted by person-to-person droplet contact and airborne transmission of droplet nuclei are the main concern (Committee on Air Quality in Passenger Cabins of Commercial Aircraft, Board on Environmental Studies and Toxicology, National Research Council, Citation2002). Droplet nuclei can remain suspended in cabin air and can be distributed within an aircraft. Many authors studied the spread of different infectious substances in aircraft cabins. Several cases of actual on-board infection transmission have been documented. These include cases of cholera (Eberhart-Phillips et al., Citation2002), influenza (Baker et al., Citation2010; Foxwell et al., Citation2011; Ooi et al., Citation2010), measles (Hoad et al., Citation2013), norovirus (Kirking et al., Citation2010), SARS (Desenclos et al., Citation2004; Olsen et al., Citation2003), shigellosis, tuberculosis (Kenyon et al., Citation1996), and COVID-19 (Eldin et al., Citation2020; Hoehl et al., Citation2020; Qian et al., Citation2020; Chen et al., Citation2020; Eichler et al., Citation2021). In line with those studies, some authors think that a large number of the reported outbreaks appear to have occurred on aircraft that were likely in-service before 1990 when HEPA filters became standard equipment on most commercial aircraft (APHI, Citation2021). Regardless, useful information relevant to a pandemic similar to COVID-19 can be gleaned from such encounters. Since COVID-19 is a highly transmittable disease, the chance of flight-related transmission of different virus variants could be increased proportionately compared to other less transmittable diseases. The risk of SARS-CoV-2 infection during a flight has likely increased since the emergence of the higher transmissibility variants such as Delta and Omicron (Whitley, Citation2021). According to Stover et al. (Citation2018), ‘air travel can serve as a conduit for the rapid spread of newly emerging infections and pandemics’ due to the large number of passengers moving domestically and internationally every day.
Fluorescent particles are tracer particles containing any number of organic compounds named fluorophores and are widely used in medicine, biochemistry, and aerosol research (MilliporeSigma, Citation2022; Cospheric, Citation2022). Fluorescent particles have been used as a tracer in flow measurements of gases and liquids, using techniques such as laser doppler anemometry (LDA), particle dynamic analysis (PDA), planer laser-induced fluorescence (PLIF), and particle image velocimetry (PIV) (MilliporeSigma, Citation2022). The utilization of fluorescence for particle tracing in water and air could be traced back to the 1980's. Since then, it has been used widely in different research fields. Yet, particle simulation in general cannot ensure an accurate modeling of the dispersion of expiratory droplet nuclei between persons, and the knowledge deficiency and the difficulties attached may decrease the probability of obtaining reliable results (Ai et al., Citation2020). Freymuth (Citation1993) used PLIF to visualize contaminant transport inside a small-scale airliner cabin by injecting a dye of uranine into the flow and observing its fluorescence under laser illumination. Poussou et al. (Citation2010) modeled an aircraft cabin with a small-scale water model aiming at studying the influence of body movement on flow and contaminant distribution inside mock-up cabins, with and without ventilation. The authors used PIV to measure the velocity field and PLIF to visualize the contaminant concentration. Sze To et al. (Citation2009) used fluorescent particles to characterize the dispersion of expiratory droplets inside an aircraft cabin with mixing ventilation, as well as to understand the effects of the cabin air changes on the dilution and removal of these particles. Fluorescent dye was allowed to deposit onto surfaces throughout the aircraft cabin. The dye was then recovered from the surfaces and measured in a spectrophotometer. Interestingly, 60%–70% of dye mass was collected from within the cabin using the fluorescent method indicating that deposition may have a large factor in disease transmission. Rudnick et al. (Citation2009) used fluorescent particles to measure influenza virus titer before and after disinfection. Using an inverted fluorescent microscope, the authors counted the number of cells with fluorescent foci to determine the disinfection rate and found that a 2.5-min surface exposure to 10-ppm Hydrogen Peroxide vapor resulted in 99% inactivation.
Tracer gas has been used as a suitable surrogate of exhaled droplet nuclei for studying airborne transmission. Different tracer gases have been used to study dispersion in different applications, including aircraft cabins. Zhang et al. (Citation2021) used N2O as a tracer gas and a CFD model of a seven-row Airbus A320 aircraft to study SARS-CoV-2 concentration and distribution inside the cabin. The authors quantified passengers’ infection risk using the susceptible exposure index (SEI) method. The results showed that it took 50 s for the virus to spread from the breathing carrier to the ceiling of the cabin. For the same breathing carrier, the adjacent passengers, and the passengers in the back two rows were affected more than the others. Because of the higher mass fraction, a coughing carrier could spread the virus up to the front three rows on the same side of the aisle as the virus carrier. Wang et al. (Citation2006) used CO2 to simulate coughing and sneezing of a sick passenger in Boeing 767 mock-up and found that close proximity between the occupants increased the exposure risk, and that the location of the release source was an important factor affecting the airborne transmission. Yan et al. (Citation2009) conducted CO2 tracer gas experiments in a full-scale Boeing 767-300 mock-up to study the global transport process of contaminated air within the cabin. The gas was released at the breathing zone of a specified passenger at the rate of 4.5 L/min for 5 min and sampled for 1000s after the gas was released. The authors placed fourteen non-dispersive infrared (NDIR) CO2 sensors in two rows around the source to collect the tracer gas concentration measurements. Wang (Citation2006) used CO2 tracer gas to investigate the airborne transport dynamics of infectious diseases in a five-row section of a Boeing 767-300 aircraft cabin mock-up. To do so, Wang varied the distance between the release source and the receptors, changed the location of the release source, and examined the effect of changing the air supply rate.
The overall objective of this study is to provide information that furthers the understanding of aerosol dispersion research in aircraft cabins. More specifically, the issue of whether tracer gas and monodisperse inert particles can represent infectious droplets has been an important consideration during the pandemic. By comparing tracer gas data to monodisperse particle data, the current study seeks to make some progress toward addressing the issue. Because these data were from independent groups under only somewhat similar experimental conditions, any agreement should be thought of as only partial validation of the existing studies, in their use of the two tracer types as infectious aerosol surrogates.
2. Methods
2.1. Cabins description
The study conducted by TRANSCOM was performed in an actual Boeing 767 aircraft with 37 rows. Measurements were collected within 8-10 rows of the injection source location. The system was moved to other cabin sections for subsequent trials. The Airliner Cabin Environment Research (ACER) laboratory study was conducted in a Boeing 767 cabin mock-up of 11 rows. The mock-up cabin was constructed using parts from an actual salvaged Boeing 767 aircraft including the passenger seats, the cabin supply air system, linear diffusers, and personal ventilation gaspers. In addition, actual Boeing 767 cabin cross-section and outline were carefully considered while designing the mock-up to accurately resemble the aircraft cabin geometry.
The ACER cabin mock-up is enclosed within a 24.3 ft x 32.2 ft by 16 ft (7.4 m x 9.8 m by 4.9 m) wooden enclosure as shown in . The mock-up has a total inside length of 30.84 ft (9.41 m) and width of 15.48 ft (4.72 m), and a total volume of 2366 cubic feet (67 cubic meters). All measurement instruments were located in two hallways with the sample lines in the cabin leading out to the right and left sides of the mock-up cabin. Two hallways on each side of the mock-up allow access to the outside of the cabin walls and provide space to house equipment and instrumentation. This void, including hallways and areas above and below the mock-up chamber, serves as an exhaust air plenum for the cabin. Once exhausted from the cabin mock-up, air is pulled from the plenum through the exhaust fans. shows an inside view of the cabin. The cabin has 11 rows of seats numbered 32 to 42 to match the US Transportation Command (TRANSCOM) study and distributed longitudinally with 7 seats in each row. The seats are in a 2-3-2 configuration, separated by two aisles as designed in a standard Boeing 767 aircraft. The rows are numbered from the front of the cabin at the front wall to the back of the cabin at the rear wall. The 7 seats columns are labeled A through L from left to right. This naming style was adopted to match the one used in the TRANSCOM study (Kinahan et al., Citation2021) which was provided to them by United Airlines.
The study conducted by TRANSCOM was carried out in a functional Boeing 767-300 aircraft. The interior dimensions of the cabin cross-section, as reported in the manufacturer's manual (), are identical to the ACER mock-up cabin. shows the cabin during the TRANSCOM study. The aircraft, operated by United Airlines, normally has 37 rows of seats divided into 3 different cabin classes with a total cabin volume of 9320 cubic feet (264 cubic meters) (United Airlines, Citation2022). The business class (section) has 10 rows of seats with 30 seats total. The economy plus (FWD-MID section) has 7 rows of seats with 46 seats total. The economy class (AFT section) has 20 rows of seats with a total of 138 seats. Hence, the maximum capacity of the aircraft is 214 passengers.
Figure 3. Boeing 767 cross-section (The Boeing Company, Citation2005).

Figure 4. Inside view of the TRANSCOM aircraft (Kinahan et al., Citation2021).

2.2. Cabins supply air description
The ACER mock-up cabin, with 77 seats, was supplied with 1400 CFM (40 m3/minute) of conditioned air. Fresh air is drawn in from outside and passed through the dehumidifier unit into the air conditioning unit, which maintains the supply air at 60 °F (15.6 °C). The air is then delivered to the cabin through the main supply air duct running in the top centre, along the cabin length. The supply air is then delivered to the linear diffusers that run along the cabin interior length as shown in in the same configuration as the Boeing 767 aircraft. The air is exhausted at the floor level along the whole length of the cabin through the side walls to the side hallways. The supply air system achieves 35 ACH and keeps the average age of particles in the cabin at about 1.7 min. For these tests, the dehumidifier was not used. Additionally, only fresh single-pass air was used for these tests. Since HEPA filters are normally used on recirculation air, the air is believed to be pathogen free. However, tracer gas would not be removed by these filters if recirculation were employed in the mock-up. Therefore, using 100% outside air is the appropriate configuration for using tracer gas to accurately simulate pathogen dispersion.
For the tests conducted in the TRANSCOM study, the reported air exchange rate for the tested Boeing 767 airframe was 32 ACH, or 4979 CFM (141 m3/min). This yields an average age of particles in the cabin of about 1.9 min. The environment control system (ECS) achieved approximately 50% of the air exchange through HEPA-filtered recirculation, and 50% through fresh bleed air. The cockpits and cabins had separate supply systems with no mixing between them. The air was supplied to the cabin at a temperature between 51.5 and 67.0 °F (10.8 °C and 19.4 °C).
2.3. Tracer, source, and experimental methods description
The study conducted by ACER utilized a tracer gas source and several gas analyzers to record the gas concentrations in radial directions at different distances away from the source. Tracer gas was introduced as an aerosol surrogate at the breathing level at different hypothetical contagious passenger locations and sampled radially up to 3.35 m from the source for 30 min per location. Two different tracer gas injection methods were used. For the first method, a 1 inch (25.4 mm) inner diameter copper tube was used as a continuous injection source to represent a breathing source. For this source type, the tracer gas consisted of CO2 injected at 0.18 CFM (5 L/min) mixed with He injected at 0.11 CFM (3.07 L/min) to achieve neutral air buoyancy. The second tracer gas injection method was an automated coughing manikin designed to simulate a coughing passenger using a CO2 tracer gas. The coughing manikin has a 0.15 ft3 (4.2 L) CO2 gas coughing volume and a peak flowrate of 24.16 CFM (11.4 L/s) with the entire cough lasting approximately 1 s.
A sampling tree was used to decrease the number of required gas analyzers needed to sample the tracer gas at different locations inside the cabin. The sampling tree is an 11 ft (3.4 m) metal rod composed of 4 equidistant sampling ports as shown in and is used to collect samples at 4 different locations sequentially. The seats in the mock-up were equally spaced as shown in the cabin schematic in allowing for the usage of the sampling tree to measure at each consecutive seat row. Each port is controlled with a normally closed (NC) SMC Pneumatics NVKF334V-3G 2-way solenoid valve connecting each of the 4-sampling ports to a common outlet port that was connected to the inlet of a single CO2 analyzer. The ports were opened sequentially, one at a time, allowing for isolated sampling of 30 min in each location. Each sampling line was made of 304 stainless steel welded tubing with an inside diameter of 0.2 inch (5 mm). A fifth normally open solenoid valve was mounted to the manifold in reverse orientation. This allows the tube connected to the common rail of the manifold on one side and to the CO2 analyzer on the other side to constantly be pulling a sample through to the CO2 analyzer when all the sampling ports were closed. For each tracer gas injection location, the sampling tree was positioned in several orientations around the source. Several non-dispersive infrared (NDIR) gas analyzers (WMA-4 and WMA-5, PP Systems International, Inc., Amesbury, MA, USA) were used to sample the tracer gas inside the cabin. Three WMA-4 analyzers were calibrated to a full-scale of 2000 ppm and have an analog voltage output with the range 0-5 V which corresponds to 0-2000 ppm of CO2. The fourth WMA-4 analyzer and the WMA-5 analyzer were calibrated to a full scale of 3000 ppm with the range 0-2.5 V which corresponds to 0-3000 ppm of CO2. A sixth gas analyzer was a custom-made Edinburg Gascard analyzer with a 0-2000 ppm range and was used to measure the tracer gas concentration in the supply air. Each gas analyzer was operated at 1 lpm. The basic principle for all three analyzers is the same. The analyzer output signals were digitally converted using an Agilent 34970 A DAC. provides further instrumentation details.
Figure 5. Tracer gas sampling tree. Vertical copper sampling tubes are indicated by white circles. The pneumatic controller is shown on the left.

Table 1. CO2 sampling system uncertainty and range.
A typical test in the ACER study was conducted in the following sequence:
Mock-up cabin was operated until thermal steady state conditions were achieved.
A pre-test single port data collection period of 20 minutes, without tracer gas injection.
A steady state single port data collection period of 15 minutes, with tracer gas injection.
A testing data collection period of 30 minutes/sampling tree port, with tracer gas injection.
A post-test single port data collection period of 20 minutes, without tracer gas injection.
The TRANSCOM study was conducted in four days with two days reserved for ground testing and two days reserved for inflight testing. Of the two ground testing days, one was reserved for simulating inflight conditions on the ground to achieve more replicates in additional seats without the need to fly the aircraft. Hence, the only difference between hangar and inflight tests in the TRANSCOM study is the elevation at which the tests were conducted. The focus of the TRANSCOM study was to look at the potential for super spreader events that would result from aerosol transmission. In the TRANSCOM study, Fluoresbrite Plain yellow-green (YG) polystyrene latex (PSL) microspheres (Polysciences) sized at 1 μm were generated using a PulmoMate (fluorescent tracer). The flowrate was approximately 6 lpm (S. Kinahan, personal communication, June 6 2022). To sample the fluorescent tracer particles, a suite of instantaneous biological analyzer and collector (IBAC, FLIR Systems) discrete particle detectors was used. The instrument samples at 3.5 lpm, and reports tracer counts per second. The fluorescent tagged microspheres were generated for one minute in a breathing pattern using a timing circuit for 2 s on and 2 s off. The total number of released particles was 180 million particles for both breathing and coughing tests. The output of the nebulizer cup (Hudson Micro Mist) was plumbed through a tripod mounted manikin head and reached a velocity of 1.43 m/s at the manikin’s lips for breathing tests and 12.84 m/s for coughing tests. shows the number of tests included in this paper from both studies. While both studies included a larger number of conducted tests, only the tests with matching source location, and experimental conditions were selected for comparison and listed in . For example, the TRANSCOM study included terminal testing conditions with the jetway attached to study the exposure levels during passengers loading and loading. In addition, a ground air cart replaced the aircraft ECS in some tests to study different supply air mechanisms. The terminal tests configuration from the TRANSCOM work was not considered since the hangar and inflight tests are with the testing conditions that closely match these in the ACER tests. To conduct a realistic comparison, only the tests without the source mask from the TRANSCOM work were considered in this study. Furthermore, since the TRANSCOM study (Silcott et al., Citation2020) noted that ‘overhead gasper supply (on or off) does not make a significant impact on aerosol risk’, tests with gaspers on and off from the TRASNCOM study were considered.
Table 2. Total number of tests, cabin section, and source seat location for each experimental configuration considered in this work for both studies in comparison.
2.4. Cabin thermal load simulation
Thermal manikins were used in the ACER study to simulate passengers and cabin thermal load. Each manikin was wrapped with 10 m wire heater elements to generate about 102 W of heat to account for the average sensible thermal output of a resting adult, 70 W (ASHRAE, Citation2008), in addition to heat from inflight entertainment systems, avionics, and personal electronics such as laptops. On the other hand, cabin heat load was not considered in TRANSCOM tests performed in the hangar and inflight.
2.5. Tracer gas calculations
To establish a similar basis for comparison, some pre-analysis procedures were applied to each dataset. For the ACER data, the first step was to express the sampled tracer gas concentration data in terms of potential exposure as a percentage of mass released (%) rather than tracer gas concentration (ppm). The second was to normalize the data to a typical resting passenger inhalation flowrate of 7.5 lpm similar to the TRANSCOM study rather than the instruments sampling rate. EquationEq. (1)(1)
(1) was used to convert the ACER data to potential exposure percentage and to normalize the data to a resting passenger inhalation flowrate of 7.5 lpm.
(1)
(1)
The TRANSCOM dataset was expressed in terms of potential exposure percentage versus distance from the source rather than seat number to establish a source-distance decay comparison. To do so, a CAD model of a Boeing 767 cabin was used to measure the distance between tracer particles release and collection locations as shown in the appendix in . Airlines have control over modifying the interior of their aircraft cabins including seat pitch and width. The dimensions of the United Airlines Boeing 767 cabin seats were obtained from United Airlines website (United Airlines, Citation2022). Inspection of EquationEq. (1)(1)
(1) shows that the ratio of the inhalation rate of a person or the intake rate of a sampling device to the total emission rate of the tracer into the space will result in small values when expressed in units of percent. These low values do not indicate low risk, and this is easily illustrated by considering that the tracer quantity would have much larger values if reported in units of ppm. Therefore, small differences in percent exposure can fall above or below important exposure thresholds and should not be thought of as being ‘within the noise’ of an experiment.
shows a summary comparison between the two studies considered in this work.
Table 3. A summary comparison between the ACER study and the TRANSCOM study.
3. Results
The tracer gas dispersion results from the ACER study are compared quantitively and qualitatively versus the fluorescence tracer particles results from the TRANSCOM study. The objective of this comparison is to evaluate the two different tracer materials as a surrogate in aerosol research in aircraft cabins.
3.1. Directional measurements
To study the effect of the measurement direction around the source on the exposure inside the aircraft cabin, the measurements collected at the same distance and direction in the two datasets were compared. Since a sampling tree was used for the ACER dataset, the tracer gas measurements in each test were made in a single radial direction from the source. The sampling tree was rotated by multiples of 45° from the source to cover all possible radial directions. The TRANSCOM average exposure percentage at each distance and direction from the source was obtained from the raw data reported by TRANSCOM. The average exposure was calculated by averaging the exposure percentage of the measurements in the same sampling tree directions used in the ACER study. shows a sample comparison of the exposure percentage at the matching distance and orientation from the source (located at distance zero) between the two studies. In this figure, each sampling tree direction test in the ACER tests was repeated twice while each direction in the TRANSCOM dataset included three test replicas. In both studies, a breathing source was located in seat L37. The insert figure on the left in shows the five sampling tree orientations from the ACER dataset which were considered as basis for comparison. The insert figure to the right shows the matching measurement directions from the TRANSCOM dataset. The legends show the five measurement directions for each dataset. The solid legend lines with square markers represent the ACER dataset, and the dashed lines with circle markers represent the TRANSCOM dataset. By filtering the TRANSCOM dataset, only the measurements collected in those five directions, and at distances matching those used in the ACER dataset were considered. A similar comparison was constructed for all breathing and coughing source locations with the source in window and middle seats.
Figure 7. Exposure along each sampling tree direction for a breathing source in window seat 37 L for the TRANSCOM and ACER studies. The positive direction is always aligned with the tree, the source is at 0, and the negative position is in the direction opposite the tree.

shows the source-distance decay model for both tracer types. The lines connecting the average measurement for each tracer type are to demonstrate the source-distance decay trend and not a curve fit trendline. The figure shows the general exposure decay away from the source at all orientations for both tracer types. The exposure in the lateral direction was the best match between the two datasets. For the rest of the sampling tree directions, exposure is better matched between the two studies in the farfield compared to near the source.
3.2. Non-directional measurements
show the average exposure percentage versus distance from the source for both ACER and TRANSCOM datasets without regard to measurement directions. The experimental sets in are divided into two general categories: tests conducted using a breathing source and tests conducted using a coughing source. Each category is further broken down to two additional categories. Namely, tests conducted with the source in a window seat and tests conducted with the source in a middle seat. For this analysis, both datasets were filtered to include all the measurements collected at all distances in all directions from the source.
Figure 8. Non-directional average exposure from a window seat source vs. distance for each TRANSCOM and ACER test configuration. Insert highlights smaller scale variation between the hangar and the inflight modes at the FWD-MID section of the TRANSCOM dataset that are obscured in the larger figure.

Figure 9. Non-directional average exposure from a middle seat source vs. distance for each TRANSCOM and ACER test configuration.

Figure 10. Average exposure percentage per distance for TRANSCOM inflight tests in the FWD-MID section compared to the ACER hangar-AFT condition, each for a window seat source.

3.2.1. Breathing source tests
3.2.1.1. Window seat tests
Three replication tests were performed for each of the three TRANSCOM test configurations in this category: (a) inflight tests with the source in the forward part of the middle section of the cabin (FWD-MID) in seat 18 L, (b) hangar tests with the source in the FWD-MID section in seat 18 L, and (c) hangar tests with the source in the rear or aft section (AFT) in seat 37 L. On the other hand, two replication tests were performed for each of the tests conducted in the ACER hangar study covering different sampling orientations. Those tests were performed with the source in the AFT section of the cabin mock-up with the source in seat 37 L. shows the average exposure percentage vs. distance from the source for each test category in the TRANSCOM and ACER studies. The legends in shows each test configuration for each dataset. For visualization purposes, a third order polynomial was fitted through the data points of all the configurations to show the trend.
shows that the Hangar-AFT configuration from the TRANSCOM dataset is the one with the best match to the ACER configuration. This similarity might be due to the window source being farther away from the supply air diffusers, which run along the center of the Boeing 767 aircraft type, as shown in . The supply jet creates a somewhat unstable pair of counter-rotating vortices, and the window seat is farther from this influence that may create directional fluctuations in tracer measurements. The window seat source comparison is expected to show better agreement, due to smaller fluctuations, than the middle seat source, which is closer to the supply jet. By comparing potential exposure for hangar configurations in different cabin sections, there seems to be a variation in exposure for sources in the AFT and the FWD-MID sections. shows that for the same breathing source type, the exposure percentage in the AFT section of the cabin was higher than the FWD-MID section. The figure also shows that there was not a noticeable difference in the source-distance decay model between the inflight FWD-MID and the hangar FWD-MID configurations which could indicate that the elevation difference did not have a great influence on the exposure in the cabin for this particular test configuration.
3.2.1.2. Middle seat tests
Three replication tests were performed for each of the four TRANSCOM test configurations in this category. The test configurations were inflight and hangar tests with the source independently located in the FWD-MID section in seat 18E, and in the AFT section in seat 37E for both testing modes. Two replication tests were performed for each of the seven tests of different sampling tree orientations in the ACER study. Those tests were performed with the source in the AFT section of the cabin mock-up with source on seat 37E. shows the average exposure percentage vs. distance from the source for each test category in the TRANSCOM and ACER studies. For visualization purposes, a third order polynomial was fitted through the data points to show the trend.
shows that the exposure percentage for all TRANSCOM test configurations was lower than those of the ACER configuration. The ACER exposure had a uniform source-distance decay curve away from the source. Similar to the window seat source in , potential exposure from sources located in different cabin sections is not necessarily the same. By comparing potential exposure for hangar configurations in different cabin sections, there seems to be a variation in exposure when the source was placed in the AFT and the FWD-MID sections. For the same breathing source type in the hangar mode, the exposure percentage in the AFT section of the cabin was higher than the FWD-MID section. For the inflight test mode, an opposite observation is noticed for the nearfield measurements up to about 2.52 m (3 seat rows) away from the source. At further sampling locations, the exposures at the AFT section become higher than the FWD-MID section. This is possibly due to the bulkhead that exists at the FWD-MID section which affects the airflow within its vicinity. Compared to the window seat source in the TRANCOM data, changing the testing mode between inflight and hangar modes had an influence on the exposure inside the cabin. For the AFT cabin section, the exposures were higher in the hangar mode. On the other hand, the exposures were higher in the inflight mode for the FWD-MID section of the cabin. For example, the exposure percentage at 1 row away (0.84 m) from the source located in the FWD-MID section increased by 80% in the inflight mode compared with the hangar mode. Variations in replicates under the same testing conditions are expected, and more variation may be due to different weather conditions, and ECS heating or cooling modes. Furthermore, elevation, cabin pressurization and temperature gradients may cause different airflow patterns as indicated in the TRANSCOM study.
3.2.2. Coughing source tests
3.2.2.1. Window seat tests
The TRANSCOM study had a limited number of tests with a coughing source. Three replication tests were performed inflight with the source in the FWD-MID section in seat 18 A. Three hangar test replications were performed in the ACER study with the source located in seat 37 A. shows the average exposure percentage vs. distance from the source for each TRANSCOM and ACER test configurations. For visualization purposes, a third order polynomial was fitted through the data point of both studies to show the trend. The TRANSCOM data show an initial decrease in exposure with distance from the source and then an increase farther away. This suggests a large-scale circulation of the plume that removes contaminant from the nearfield by transporting it to a location in the farfield. The lab tracer gas results might indicate some of this circulation behaviour, with first a small increase with distance from the source, then a small decrease. The cough results are clearly higher in TRANSCOM, with a maximum of 0.011% compared to 0.0011% for the tracer gas, an order of magnitude.
3.2.2.2. Middle seat tests
Three inflight test replications were performed in the TRANSCOM study with the source in the AFT section in seat 37E. In the ACER study, 3 hangar test replications were performed with the source in the AFT section in seat 37E. shows the average exposure percentage vs. distance from the source for each TRANSCOM and ACER test configurations. For visualization purposes, a third order polynomial was fitted through the data point of both studies to show the trend. As with the window seat results in , the middle seat source results in show an initial decrease followed by an increase with distance in the TRANSCOM data. The tracer gas data do not show a clear trend other than approximate uniformity with distance, where a possible interpretation is more complete mixing of gas than of particles. The TRANSCOM data were clearly higher than the ACER lab tracer gas data.
4. Discussion
4.1. Particle deposition
Factors that could explain variations in gas exposures and aerosol dispersion characteristics in the two datasets include inherent differences between tracer gases and solid particles, along with other experimental conditions. The TRANSCOM study reported a maximum deposition rate of 0.005% of the emitted particles, scaled to a square foot for tests conducted in the inflight breathing mode in the Boeing 767 aircraft. For the same dataset, the minimum, average, and maximum exposures were 0.0003%, 0.0041%, and 0.0114%, respectively. The small numbers in and of themselves do not necessarily indicate low risk. On the other hand, due to the nature of gases, deposition was not a measurable quantity in the ACER study. Furthermore, the CO2 tracer gas was mixed with helium to achieve neutral buoyancy in the cabin air. The particle deposition effect clearly was a major factor in the exposure values being higher in the ACER study than in the TRANSCOM study.
4.2. Experimental independence
The ACER tracer gas study and the TRANSCOM study were conducted by independent groups. Data from the two studies were collected in a cabin with 11 rows at ground level (ACER) rather than a real operating plane (TRANSCOM), sometimes at ground level and sometimes in flight. Actual flights would include solar radiation, ambient air conditions, cabin pressurization and aircraft motion. Still, the TRANSCOM study deviated from actual passenger flights in not accounting for passenger thermal plume effects in tests conducted inflight and in the hangar, while acknowledging that ‘heating vs. cooling modes had the potential to drive airflow direction differently.’ The TRANSCOM study did use some heating blankets in their terminal testing mode, but these data are not considered in the current paper because some testing conditions differed greatly, such as the cabin door being open.
4.3. Experimental error
Experimental errors in each study could also explain differences in the reported exposure data. In the TRANSCOM study, in addition to the variability due to the statistical nature of particle movement, instrument error in the number of particles counted for the release and the seat sampling would of course directly affect the calculated exposure, although the current study presumes no knowledge of such errors, if any. Fluorescent particles were counted over an interval of six minutes. Based on the average residence time in the cabin, this might not be long enough for all the released particles to be either captured by the instruments, deposited on surfaces, or removed in the exhaust. Experimental procedures from the ACER study could also have created errors that influenced the results. Those might include a measured CO2 injection flowrate lower than the actual, a CO2 gas analyzer reading higher than the actual, and a supply airflow measurement lower or higher than actual lab conditions. Any of these possible errors would have affected the exposures, as source injection percentages. A total of 54 experiments were conducted, and a detailed error analysis is reported in Mahmoud (Citation2021). is included to summarize the uncertainty at the six positions along the sampling tree. Noteworthy is how the uncertainty was highest where the tracer gas measurements were lowest—farthest from the source.
4.4. Dispersion variability
While all the above factors could affect the comparison, the dominant factor likely is the inherent variability of the dispersion phenomena. From one test to the next for the same location and conditions in the ACER study, variations in results were far larger than could be explained by experimental errors were common. Additionally, for a given location, the concentration profile could be very different in different directions, especially front to back even though the cabin ventilation flows were designed to be balanced longitudinally and there are no intended net longitudinal flows. Additionally, smoke visualization was used to qualitatively study the flow patterns in the cabin mock-up (Shehadi et al., Citation2018). This visualization showed that the patterns are chaotic and unpredictable– unlike the animated flow arrows and laminar descriptions in some popular media stories (Grondahl et al., Citation2021; Read, Citation2020). For example, complete reversal in direction locally is not uncommon and can last for many seconds, even minutes. Local flow patterns, particularly those in the vicinity of the source can have a large impact on the local dispersion of tracers. Additionally, the visualization shows that the injected markers tend to flow in plumes rather than being uniformly dispersed. Given this plume nature of the dispersion, small differences in location can have large effects on the concentrations of injected markers. A cabin study emphasizing long measurement durations (Grote, Citation2023) observed extreme variation in tracer results among tests of the same injection types, notably in the continuous injection regime. These behavioral shifts in carbon dioxide response provide some evidence for the presence of what may be called metastable flow structures. For these reasons, it is unrealistic to expect close agreement between small numbers of tests conducted in different cabins at different times or in different facilities. However, general comparisons about the nature of the dispersion can be made.
The overall uncertainty, expressed as standard error of the time series average, in the ACER tracer gas samples ranged from 6% closest to the source to 20% at the farthest sampling points. The 42 IBACs used in the TRANSCOM study were pre-calibrated against a referee IBAC, and the fluorescent particle counts matched to within an average variance of ±10%. These samples had a standard error of the mean of 0.0014 percentage points in the economy cabin sections and 0.0010 percentage points in first class, as reported in Kinahan et al. (Citation2021). The standard error of the mean of the number of particles released (1.8x108), was 1x107, and the standard deviation in the number of released particles was 1.7x107. The TRANSCOM study did not report the overall uncertainty, which includes factors such as the supply air flowrate. Yet, the error could be within uncertainties expected in simulating particle dispersion using tracer gas, according to Ai et al. (Citation2020).
4.5. Gas and particle tracer emission
Despite both studies utilizing coughing manikins with a similar cough release velocity, the dispersion pattern and the calculated exposure percentage differed by tracer type. To achieve the cough velocity, TRANSCOM used a mouthpiece that accelerated the flow from the breathing velocity up to the coughing velocity, while using the same duration and cycle for coughing as for breathing: one-minute emission duration, cycling as two seconds on and two seconds off. Conversely, ACER used a coughing manikin that produced a cough every 75 s, with every cough lasting one second. Although both studies tried to get close to simulating an actual human cough, neither method generated tracer that accurately represents particles generated during a real cough.
4.6. Patterns of dispersion
By comparing the dispersion characteristics between the two datasets, a few points can be highlighted. The general source-distance decay model seems to be similar for both datasets, in that tracer concentration decreases as distance from the source increases. This behavior was evident in all directions around the source, as shown in . Notably, in some test conditions in both studies, tracer concentrations further away exceeded measurements nearby the source. An example is the measurements collected with a breathing source in the middle of the cabin, in the inflight-AFT mode in the TRANSCOM study, as shown in . Similarly, for both coughing source locations in both studies, and show a similar phenomenon. The cough momentum could explain increased concentrations at locations farther from the source in those tests. On the other hand, for the breathing source tests, the inherent airflow pattern inside aircraft cabins, which tends to have swirls and eddies, could direct the tracer gas in a specific direction causing local anisotropic elevated concentrations. A detailed analysis, including a smoke visualization of this phenomenon in the ACER cabin, is reported in Mahmoud (Citation2021), and a similar phenomenon was described in other studies, such as (Silcott et al., Citation2020).
For both studies, measurements collected at the same distance but in different orientations around the source were not necessarily the same, nor were they the same at a single location in subsequent trials. This is typical for measurements collected nearby the source and not farther away from the source where less variation between individual measurements were recorded. shows that the average exposures for two equally spaced seats (F39 and F35) from the source in the TRANSCOM study are 0.033% and 0.010%, respectively. Observations in the ACER study also varied at most of the source-distance values shown. Zhang et al. (Citation2021) used a CFD model of a seven-row Airbus A320 aircraft to look at SARS-CoV-2 spread in the cabin. They reported that the adjacent passengers and the passengers in the back two rows are affected more than the others when a source passenger breathes normally. Also, Lei et al. (Citation2017) showed that passengers in aisle seats had a higher norovirus infection risk from fomites than others when traveling with a virus carrier in the same aircraft cabin, and the predicted infection risk from the fomite route for aisle seat passengers was 2.2 times higher than that for non-aisle seat passengers. It is worth mentioning that variations between different airframes and testing conditions could result in different dispersion patterns. Hence, a generalized dispersion model would not likely describe all the transmission scenarios.
By looking at and , the exposure amounts and distribution patterns differ for window vs. middle sources, for both datasets. The authors of the TRANSCOM studybelieve that ‘while there is a measurable difference in middle vs aisle or window seat, results show that this relative exposure risk is not generalizable and depends on location and seat throughout the cabin’ (Kinahan et al., Citation2021). Yet, it might be essential to quantify the impact of these exposure differences in the case of highly communicable diseases, since large reductions in local particle counts compared to the total released is to be generally expected in indoor environments.
5. Conclusion
This paper analyzes two different tracer types used as aerosol surrogates in studying exposure in aircraft cabins by comparing two published datasets. The first dataset is from a tracer gas study conducted at the ACER laboratory in a Boeing 767 mock-up. The second dataset is from a fluorescent particles study conducted by TRANSCOM in an actual Boeing 767. The comparison between the two tracer types shows a general source-distance decay away from the source at all orientations for both tracer types. For a window seat breathing source, there was good agreement in exposure percentage when the TRANSCOM testing mode was the hangar-AFT. For a middle seat breathing source, the average exposure using tracer gas was higher than the four test configurations in the TRANSCOM study. Using a coughing source in a window seat, the TRANSCOM exposures were higher within the first two rows from the source, before coming down to the ACER levels up to about 5 m away. A similar trend was recorded for a middle seat coughing source with higher overall exposure for the TRANSCOM data.
The comparison showed three broad trends. Firstly, exposure generally yet inconsistently decreased with distance from the emission source. Secondly, the ACER laboratory tracer gas results were a higher percentage of the mass released at breathing velocity than were the TRANSCOM particle results; however, the TRANSCOM particle results were higher than the ACER gas results when the emission was at cough velocity. Thirdly, exposures at each specific measurement location were below approximately 0.3% of the total mass released but varied greatly within this range. There are several sources of variation in exposure estimation between the two studies including particle deposition. Given the chaotic nature of the local airflow patterns in the aircraft cabin and the resulting unpredictability of the patterns for a given locations, these differences between the two datasets are not unexpected. Perhaps the most important consideration is that, overall, the quantitative results are similar enough, even though very different experimental methods were used, i.e. DNA-tagged fluorescent particles vs tracer gas, to give some confidence that both studies, conducted by independent groups, generated reliable data. A recommendation for future research is the direct comparison of airborne exposure patterns for particles and tracer gas under otherwise identical conditions in air travel environments.
Author disclaimer
The findings and conclusions in this paper are those of the author(s) and do not necessarily represent the official position of the National Institute for Occupational Safety and Health, Centers for Disease Control and Prevention. Mention of any company or product does not constitute endorsement by the National Institute for Occupational Safety and Health, Centers for Disease Control and Prevention.
Disclosure statement
The authors have no conflicts of interest to report related to the current research.
Data availability statement
The TRANSCOM study data are publicly available at: https://figshare.com/articles/dataset/USTRANSCOM_767-Flight_Master_Spreadsheet/13093055. The ACER tracer gas data are publicly available through Seif Mahmoud’s dissertation at: https://krex.k-state.edu/handle/2097/41398. The data from every experimental trial, including those used in the current paper, appear as individual graphs in the dissertation. One reference (Grote) is a masters thesis publicly available at: https://krex.k-state.edu/handle/2097/43040.
Correction Statement
This article has been corrected with minor changes. These changes do not impact the academic content of the article.
Additional information
Notes on contributors
Seif Mahmoud
Seif Mahmoud is currently a Lead Aerodynamics Engineer at GE Aerospace. He recently served as an Oak Ridge Institute (ORISE) Fellow at the National Institute for Occupational Safety and Health, Centers for Disease Control and Prevention (CDC), where he researched aerosol and pathogens dispersion in different environments. He holds a PhD degree in Mechanical Engineering from Kansas State University with interests in fluid dynamics, aerodynamics, and heat transfer.
James Bennett
James Bennett is a research engineer with the National Institute for Occupational Safety and Health (NIOSH), which is one the federal Centers for Disease Control and Prevention (CDC). He has over 30 years of experience in industrial hygiene, particularly in the design and evaluation of engineering controls, in exposure quantification, and in contaminant transport modeling. His research areas include application of CFD and spatial models to the built and industrial environments. Dr. Bennett obtained a Bachelor Degree in Electrical Engineering from the University of Michigan and a Masters and Ph.D. in Environmental Health Sciences from the University of South Carolina. He is an ASHRAE member, former Chair of the Indoor Environmental Modeling Technical Committee, TC 4.10. and the current Chair of the Technical Activities Committee (TAC).
Byron Jones
Byron Jones is Professor Emeritus at Kansas State University, Department of Mechanical and Nuclear Engineering, where he has served for 45 years. He served as technical director for the six-university National FAA Airliner Cabin Environment Research Center of Excellence. Professor Jones is a Life Member of the American Society of Mechanical Engineers and is a Fellow and Life Member of the American Society of Heating Refrigerating and Air Conditioning Engineers. He also served as a distinguished lecturer for both ASME and ASHRAE making presentations at more than 40 local chapters throughout the U.S. and in several other countries.
Mohammad Hosni
Mohammad Hosni is a Professor of Mechanical Engineering and the Director of the University Engineering Alliance at Kansas State University. He holds a Ph.D. in mechanical engineering and his research interest areas include Fluid Mechanics, Heat Transfer, Air Distribution, Human Thermal Comfort, Experimental Techniques and Uncertainty Analysis. He is a Fellow of ASME, ASHRAE, and ABET.
References
- APHI. (2021). Assessment of Risks of SARS-CoV-2 Transmission During Air Travel and Non-Pharmaceutical Interventions to Reduce Risk; PhaseTwo Report: Curb-to-Curb Travel Through Airports, Aviation Public Health Initiative: Harvard T.H. Chan School of Public Health.
- Ai, Z., Cheuk, M. M., Gao, N., & Niu, J. (2020). How suitable is tracer gas as a surrogate of exhaled droplet nuclei for studying airborne transmission in the built environment [Paper presentation]. 16th Conference of the International Society of Indoor Air Quality and Climate: Creative and Smart Solutions for Better Built Environments, Indoor Air 2020.
- Airlines for America. (2022). Emerging From the Pandemic.
- Airports Council International. (2021). Pandemic resurgence and increasing uncertainty are delaying recovery. The Impact of COVID-19 on the Airport Business—and the Path to Recovery. https://aci.aero/2021/11/01/the-impact-of-covid-19-on-the-airport-business-and-the-path-to-recovery-3/
- ASHRAE. (2008). ASHRAE handbook – heating, ventilating and air-conditioning applications. Atlanta: ASHRAE. https://doi.org/10.31826/9781463212209-013
- Baker, M. G., Thornley, C. N., Mills, C., Roberts, S., Perera, S., Peters, J., Kelso, A., Barr, I., & Wilson, N. (2010). Transmission of pandemic A/H1N1 2009 influenza on passenger aircraft: Retrospective cohort study. BMJ (Online), 340, c2424–c2424. https://doi.org/10.1136/bmj.c2424
- Bielecki, M., Patel, D., Hinkelbein, J., Komorowski, M., Kester, J., Ebrahim, S., Rodriguez-Morales, A. J., Memish, Z. A., & Schlagenhauf, P. (2021). Air travel and COVID-19 prevention in the pandemic and peri-pandemic period: A narrative review. Travel Medicine and Infectious Disease. Elsevier Inc. https://doi.org/10.1016/j.tmaid.2020.101915
- Chan, H. T, School of Public Health. (2021). Assessment of Risks of SARS-CoV-2 Transmission During Air Travel and Non-Pharmaceutical Interventions to Reduce Risk.
- Chen, J., He, H., Cheng, W., Liu, Y., Sun, Z., & Chai, C. (2020). Potential transmission of SARS-CoV-2 on a flight from Singapore to Hangzhou, China: An epidemiological investigation.
- Committee on Air Quality in Passenger Cabins of Commercial Aircraft, Board on Environmental Studies and Toxicology, National Research Council. (2002). The airliner cabin environment and the health of passengers and crew. National Academies Press. https://doi.org/10.17226/10238
- Cospheric. (2022). Fluorescent microspheres - properties and applications.
- Desenclos, J.-C., van der Werf, S., Bonmarin, I., Levy-Bruhl, D., Yazdanpanah, Y., Hoen, B., Emmanuelli, J., Lesens, O., Dupon, M., Natali, F., Michelet, C., Reynes, J., Guery, B., Larsen, C., Semaille, C., Mouton, D., André, M., Escriou, N., Burguière, A., … Scheidegger, S. (2004). Introduction of SARS in France, March-April, 2003. Emerging Infectious Diseases, 10(2), 195–200. https://doi.org/10.3201/eid1002.030351
- Eberhart-Phillips, J., Besser, R. E., Tormey, M. P., Feikin, D., Araneta, M. R., Wells, J., Kilman, L., Rutherford, G. W., Griffin, P. M., Baron, R., & Mascola, L. (2002). An outbreak of cholera from food served on an international aircraft. Epidemiology and Infection, 118(1), 79–79. https://doi.org/10.1017/S095026889600948X
- Eichler, N., Thornley, C., Swadi, T., Devine, T., McElnay, C., Sherwood, J., Brunton, C., Williamson, F., Freeman, J., Berger, S., Ren, X., Storey, M., de Ligt, J., & Geoghegan, J. L. (2021). Transmission of severe acute respiratory syndrome coronavirus 2 during border quarantine and Air Travel, New Zealand (Aotearoa). Emerging Infectious Diseases, 27(5), 1274–1278. https://doi.org/10.3201/eid2705.210514
- Eldin, C., Lagier, J-C., Mailhe, M., & Gautret, P. (2020). Letter to the editor probable aircraft transmission of Covid-19 in-flight from the central african republic to france. Travel Medicine and Infectious Disease, 35, 101643. https://doi.org/10.1016/j.tmaid.2020.101643
- Foxwell, A. R., Roberts, L., Lokuge, K., & Kelly, P. M. (2011). Transmission of influenza on international flights, May 2009. Emerging Infectious Diseases, 17(7), 1188–1194. https://doi.org/10.3201/eid1707.101135
- Freymuth, P. (1993). Flow visualization in fluid mechanics. Review of Scientific Instruments, 64(1), 1–18. https://doi.org/10.1063/1.1144433
- Grondahl, M., Mzezewa, T., Fleisher, O., & White, J. (2021). How safe are you from Covid when you fly? https://www.nytimes.com/interactive/2021/04/17/travel/flying-plane-covid-19-safety.html
- Grote, J.-M. (2023). Experimental analysis of tracer gas injection methods simulating aerosols and their effects on dispersion in aircraft cabins. Mechanical and Nuclear Engineering. Manhattan, Kansas, Kansas State University. Master of Science: 163.
- Hoad, V. C., O'Connor, B. A., Langley, A. J., & Dowse, G. K. (2013). Risk of measles transmission on aeroplanes: Australian experience 2007-2011. The Medical Journal of Australia, 198(6), 320–323. https://doi.org/10.5694/mja12.11752
- Hoehl, S., Karaca, O., Kohmer, N., Westhaus, S., Graf, J., Goetsch, U., & Ciesek, S. (2020). Assessment of SARS-CoV-2 transmission on an international flight and among a tourist group. JAMA Network Open, 3(8), e2018044. https://doi.org/10.1001/jamanetworkopen.2020.18044
- IATA. (2022). Airlines Cut Losses in 2022; Return to Profit in 2023, International Air Transport Association.
- Kinahan, S. M., Silcott, D. B., Silcott, B. E., Silcott, R. M., Silcott, P. J., Silcott, B. J., Distelhorst, S. L., Herrera, V. L., Rivera, D. N., Crown, K. K., Lucero, G. A., & Santarpia, J. L. (2021). Aerosol tracer testing in boeing 767 and 777 aircraft to simulate exposure potential of infectious aerosol such as SARS-CoV-2. PLoS ONE, 16(12), e0246916. https://doi.org/10.1371/journal.pone.0246916
- Kirking, H. L., Cortes, J., Hall, A. J., Burrer, S., Fishbein, D. B., Lipman, H., Cohen, N. J., Daly, E. R., & Kim, C. (2010). Likely transmission of norovirus on an airplane, October 2008. Clinical Infectious Diseases, 50(9), 1216–1221. https://doi.org/10.1086/651597
- Lei, H., Li, Y., Xiao, S., Yang, X., Lin, C., Norris, S. L., Wei, D., Hu, Z., & Ji, S. (2017). Logistic growth of a surface contamination network and its role in disease spread. Scientific Reports, 7(1), 1–10. https://doi.org/10.1038/s41598-017-13840-z
- Mahmoud, S. (2021). Experimental analysis of aerosols dispersion and containment solutions in aircraft cabins. Kansas State University, Manhattan, Kansas. https://krex.k-state.edu/dspace/handle/2097/41398
- Mangili, A., & Gendreau, M. A. (2005). Transmission of infectious diseases during commercial air travel. Lancet (London, England), 365(9463), 989–996. https://doi.org/10.1016/S0140-6736(05)71089-8
- MilliporeSigma. (2022). Fluorescent microparticles and nanobeads. https://www.sigmaaldrich.com/US/en/technical-documents/technical-article/materials-science-and-engineering/biosensors-and-imaging/fluorescent-microparticles
- Moody's Investors Service. (2020). Airline industry disruption has far-reaching impact.
- Olsen, S. J., Chang, H.-L., Cheung, T. Y.-Y., Tang, A. F.-Y., Fisk, T. L., Ooi, S. P.-L., Kuo, H.-W., Jiang, D. D.-S., Chen, K.-T., Lando, J., Hsu, K.-H., Chen, T.-J., & Dowell, S. F. (2003). Transmission of the severe acute respiratory syndrome on aircraft. The New England Journal of Medicine, 349(25), 2416–2422. https://doi.org/10.1056/nejmoa031349
- Ooi, P. L., Lai, F. Y. L., Low, C. L., Lin, R., Wong, C., Hibberd, M., & Tambyah, P. A. (2010). Clinical and molecular evidence for transmission of novel influenza A(H1N1/2009) on a commercial airplane. Archives of Internal Medicine, 170(10), 913–915. https://doi.org/10.1001/archinternmed.2010.127
- Poussou, S. B., Mazumdar, S., Plesniak, M. W., Sojka, P. E., & Chen, Q. (2010). Flow and contaminant transport in an airliner cabin induced by a moving body: Model experiments and CFD predictions. Atmospheric Environment, 44(24), 2830–2839. https://doi.org/10.1016/j.atmosenv.2010.04.053
- Qian, G.-Q., Yang, N.-B., Ding, F., Ma, A. H. Y., Wang, Z.-Y., Shen, Y.-F., Shi, C.-W., Lian, X., Chu, J.-G., Chen, L., Wang, Z.-Y., Ren, D.-W., Li, G.-X., Chen, X.-Q., Shen, H.-J., & Chen, X.-M. (2020). Epidemiologic and clinical characteristics of 91 hospitalized patients with COVID-19 in Zhejiang, China: A retrospective, multi-centre case series. Monthly Journal of the Association of Physicians, 113(7), 474–481. https://doi.org/10.1093/qjmed/hcaa089
- Read, J. (2020). How clean is the air on planes? https://www.nationalgeographic.com/travel/article/how-clean-is-the-air-on-your-airplane-coronavirus-cvd
- Rudnick, S. N., McDevitt, J. J., First, M. W., & Spengler, J. D. (2009). Inactivating influenza viruses on surfaces using hydrogen peroxide or triethylene glycol at low vapor concentrations. American Journal of Infection Control, 37(10), 813–819. https://doi.org/10.1016/j.ajic.2009.06.007
- Shehadi, M., Hosni, M., & Jones, B. (2018). Airflow and turbulence analysis inside a wide-body aircraft cabin mockup. Indoor and Built Environment, 27(6), 766–785. https://doi.org/10.1177/1420326X16689720
- Silcott, D., Kinahan, S., Santarpia, J., Silcott, B., Silcott, R., Silcott, P., & Silcott, B. (2020). TRANSCOM/AMC commercial aircraft cabin aerosol dispersion tests.
- Stover, V., Weiss, H., Elon, L., Si, W., & Norris, S. L; FlyHealthy Research Team. (2018). Droplet-mediated respiratory diseases during transcontinental airline flights. Proceedings of the National Academy of Sciences of the United States of America 115(14), 3623–3627. https://doi.org/10.1073/pnas.1711611115
- Sun, X., Wandelt, S., Zheng, C., & Zhang, A. (2021). COVID-19 pandemic and air transportation: Successfully navigating the paper hurricane. Journal of Air Transport Management, 94, 102062. https://doi.org/10.1016/j.jairtraman.2021.102062
- Sze To, G. N., Wan, M. P., Chao, C. Y., Fang, L., & Melikov, A. (2009). Experimental study of dispersion and deposition of expiratory aerosols in aircraft cabins and impact on infectious disease transmission. Aerosol Science and Technology, 43(5), 466–485. https://doi.org/10.1080/02786820902736658
- The Boeing Company. (2005). 767 airplane characteristics for airport planning: Airplane description. http://www.boeing.com/commercial/airports/acaps/767sec2.pdf
- Kenyon, T. A., Valway, S. E., Ihle, W. W., Onorato, I. M., & Castro, K. G. (1996). Transmission of multidrug-resistant Mycobacterium tuberculosis during a long airplane flight. N Engl J Med. 334(15), 933–38. https://doi.org/10.1056/NEJM199604113341501
- United Airlines. (2022). Boeing 767-300 (763).
- Wang, Aijun, (2006). Quantifying Air Distribution, Ventilation Effectiveness and Airborne Pollutant Transport in an Aircraft Cabin Mockup. Doctoral dissertation, University of Illinois at Urbana-Champaign.
- Wang, A., Zhang, Y., Topmiller, J. L., Dunn, K. H., & Bennett, J. S. (2006). Tracer study of airborne disease transmission in an aircraft cabin mock-up. ASHRAE Transactions, 112, 697–706.
- Whitley, A. (2021). Omicron may double risk of getting infected on planes, IATA Says. https://www.bloomberg.com/news/articles/2021-12-22/omicron-at-least-doubles-risk-of-getting-infected-on-a-plane
- Yan, W., Yuanhui Zhang, Ã., Sun, Y., & Li, D. (2009). Experimental and CFD study of unsteady airborne pollutant transport within an aircraft cabin mock-up. Building and Environment, 44(1), 34–43. https://doi.org/10.1016/j.buildenv.2008.01.010
- Zhang, M., Yu, N., Zhang, Y., Zhang, X., & Cui, Y. (2021). Numerical simulation of the novel coronavirus spread in commercial aircraft cabin. Processes, 9(9), 1601. https://doi.org/10.3390/pr9091601