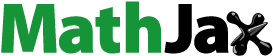
ABSTRACT
Questionnaires in educational research assessing students’ attitudes and beliefs are low-stakes for the students. As a consequence, students might not always consistently respond to a questionnaire scale but instead provide more random response patterns with no clear link to items’ contents. We study inter-individual differences in students’ intra-individual random responding profile across 19 questionnaire scales in the TIMSS 2015 eighth-grade student questionnaire in seven countries. A mixture IRT approach was used to assess students’ random responder status on a questionnaire scale. A follow-up latent class analysis across the questionnaire revealed four random responding profiles that generalized across countries: A majority of consistent nonrandom responders, intermittent moderate random responders, frequent random responders, and students that were exclusively triggered to respond randomly on the confidence scales in the questionnaire. We discuss the implications of our findings in light of general data-quality concerns and the potential ineffectiveness of early-warning monitoring systems in computer-based surveys.
A large research base in the educational sciences is built on studies using questionnaires to survey students’ values, beliefs, and attitudes toward school subjects such as mathematics and science (Eccles & Wigfield, Citation2002; Linnenbrink & Pintrich, Citation2002; Osborne et al., Citation2003; Potvin & Hasni, Citation2014). This research base includes both smaller-scale individual research-team studies and larger-scale international comparative studies such as OECD’s Program for International Student Assessment (PISA) or IEA’s Trends in International Mathematics and Science Study (TIMSS). The research results are typically used to evaluate and contextualize educational practice and inform educational policy.
Yet, research on such attitudinal constructs is low-stakes for the students as it has no direct consequences or relevance for themselves (in contrast to, for instance, cognitive achievement tests or exams). At the heart of educational and psychological measurements, even before Cronbach’s (Citation1946) treatise on response sets and validity, there is a core concern that students might not always respond accurately or thoughtfully to a questionnaire but instead shift to responding with the lowest effort (e.g., Curran, Citation2016; Eklöf, Citation2010; Huang et al., Citation2012) such that their item responses and scale scores might no longer accurately reflect the constructs that the questionnaire scales were intended to assess (e.g., Messick, Citation1984). One way this can express itself is that instead of the expected consistent item response pattern on a questionnaire scale, a more random response pattern is provided with no clear link to items’ contents.
Individuals engaging in random response behavior on a questionnaire scale can potentially distort inferences on basic item statistics, reliability, dimensionality, and intercorrelations within and between constructs (e.g., Credé, Citation2010; Huang et al., Citation2012; Liu et al., Citation2019; Maniaci & Rogge, Citation2014; Meade & Craig, Citation2012). Random responding can be seen as a type of nonresponse; even though responses are observed, genuine information on the actual response that the individual would have given, if they had responded in a regular nonrandom fashion, is missing. Hence, the underlying process giving rise to the nonresponse is crucial both for understanding the phenomenon and for assessing its expected impact and how to handle it in data analyses (cf. M(C/N)AR missingness framework, Rubin Citation1976). Typically, scale and item means are biased toward their midpoint and residual item variances tend to be inflated, whereas scale and item covariances within and between other constructs can be biased in either direction or remain unaffected. Higher impact can be expected with increased prevalence and when regular consistent responders tend to score further away from the midpoint.
Random responding is speculated to occur due to, among others, carelessness, insufficient effort, disengagement, or lack of motivation and seriousness on behalf of the respondent (e.g., Huang et al., Citation2012). To the extent that they are almost always the same individuals that are random responding on scales throughout the questionnaire, the validity threat is mostly located within the student responding. By implication, random responding would then be mostly beyond our reach unless we manage to figure out individualized incentives that convince these students to genuinely engage with the survey in a low-stakes context. On the other hand, to the extent that individuals systematically vary the extent of their random response behavior throughout the questionnaire, the validity threat could be due to specific triggers in their questionnaire progress or scale content or type. The latter aspects would provide possible pathways to redesign and modify the questionnaire to dampen the triggers and reduce the general validity threat. In contrast, when random responding occurs more incidentally among individuals throughout the questionnaire, there are no clear levers for reducing its prevalence, but the impact of such random responding can also be expected to be minimal as it would conform to a completely-at-random nonresponse pattern (cf. MCAR, Rubin, Citation1976).
Thus, the distinct patterns in within-person variation in random responding become especially important (for a more general call for within-person research, see also Molenaar, Citation2004) if we want to extend conclusions about individuals based on limited information as in a data quality monitoring or screening system. For example, if a student is identified as a random responder on one scale, what does this imply for the rest of the questionnaire? Can their responses on other scales in the questionnaire still be trusted or are they all to be considered invalidated? By studying the inter-individual differences in intra-individual random response behavior across the questionnaire scales in a survey, we aim to further clarify to what extent random responding would be a systematic biasing factor that potentially threatens and distorts inferences made from the survey data and shed further light on potential factors triggering such random response behavior.
Individual differences in random responding across questionnaire scales
There are different ideas of how response behavior is actualized over the course of a questionnaire at the individual level. Most of these ideas can be traced back to ancient-old discussions in the general field of individual differences such as the trait versus state (e.g., Schmitt & Blum, Citation2020) or person versus situation (e.g., Fleeson, Citation2004) debates.
Trait perspective
A person-central or trait perspective would prescribe that individuals have the tendency to respond to a questionnaire in a consistent manner (e.g., favoring a certain response option, speeding, or guessing) and that this response behavior is reflective of underlying personality traits and relatively stable across time (e.g., Messick, Citation1991). Cronbach (Citation1950) indicates that especially within a singular-content questionnaire, this consistency of response behavior should become clear. This does not imply that individuals will respond perfectly consistent, as it has generally been considered that no trait is perfectly stable. Yet, from this viewpoint, it is expected that if respondents had had engaged in random responding on one scale, they would also have an increased probability of being identified as a random responder on any of the other scales in the questionnaire, and limited within-person variability in random responding across the questionnaire is implied.
Bowling et al. (Citation2016) provide tentative evidence on temporal stability and correlations with personality traits, which would be consistent with a trait-based individual differences perspective. Other findings in the literature cast doubt on whether such individual consistency in random responding across the questionnaire is a realistic pattern to expect. For a sequence of achievement tests in a US context, Soland and Kuhfeld (Citation2019) conclude that rapid guessing is not longitudinally stable, but some cross-sectional correlations with other trait measures were observed. For a low-stakes scientific reasoning test, Wise et al. (Citation2009) note that only a small percentage of college students appeared to have engaged in random response behavior on the majority of the questionnaire. Similarly, self-reports in personality research indicated that of the 52% of college students who reported themselves to have engaged in some level of random responding on the MMPI-2 questionnaire, only 3% indicated to have responded randomly to “many” or “most” of the items (Berry et al., Citation1992). Given such findings and the fact that most surveys in large-scale educational research are also non-singular in contents, we expect that only for a limited number of individuals a universally applicable “random responder” trait applies in an educational survey context.
State perspective
Alternatively, random response behavior could be more of a temporary state that expresses itself only in specific parts of a questionnaire regardless of the content. In the personality assessment literature, the perspective of so-called “back-random” responding has especially gained much attention (e.g., Clark et al., Citation2003; Gallen & Berry, Citation1997; Pinsoneault, Citation2007). Once an individual’s internal “cognitive resources” have been depleted and/or they are no longer willing to actively engage with the questionnaire, the individual switches from regular response behavior to expressing random response behavior and carries on to do so for the remainder of the questionnaire (Bowling et al., Citation2021; Clark et al., Citation2003). Notions of boredom, disinterest, inattentiveness, or fatigue are indicated as potential underlying drivers of the phenomenon. From this viewpoint, it is expected that once individuals are identified as random responders on a scale, they will also have a higher probability to be identified as random responders on the scales following that scale in the questionnaire.
For a low-stakes information literacy test, Wise (Citation2006) found that several participants switched to random response behavior over the course of the test and persisted to do so for most of the remainder of the test (see also, Cao & Stokes, Citation2007). Similarly, self-reports in personality research asked about “the proportion of test questions which you were unable to pay attention to and answered randomly” (Berry et al., Citation1992, p. 341) and 42–52% of the individuals across different samples indicated the most common place for them was toward the end of the questionnaire (from response options: mostly in the first part; mostly in the middle part; mostly in the last part; scattered throughout). For achievement testing, Ackerman and Kanfer (Citation2009) concluded that subjective test fatigue was better predicted by individual differences in personal motivation than by mere physical differences in test length. If back-random responding is indeed more of a personal motivation issue, then the low-stakes character of many assessments in educational research can be considered to be a facilitating factor for back-random responding. Whether and the point at which this within-person shift to random response behavior can be observed will vary across the questionnaire from person to person depending on their general engagement with the questionnaire. Thus, good questionnaire design would target the survey to the intended population, inquire about aspects that speak to this population, and allow generous time to complete the survey; In such an ideal situation, back-random responding should theoretically be a rare phenomenon.
Situation perspective
Whereas the previous perspectives seek the triggers for random response behavior mostly internal to a person, one could also posit that external triggers could play a role. Cronbach (Citation1950) indicated that response sets are more stable within singular-contents questionnaires and that response sets become more influential as items become more difficult or ambiguous. Similarly, Baer et al. (Citation1997) indicated that the two main reasons for giving random responses were difficulty in understanding the question or in deciding on the response alternative. From this viewpoint, it is expected that if respondents had engage in random responding on one scale, they would also have an increased probability of being identified as a random responder on a similar scale in the questionnaire, but not a dissimilar scale; where similarity is either in contents or response type. This implies limited within-person variability across similar scales but large within-person variability between dissimilar groups of scales.
Idiosyncratic perspective
Another alternative is that random response behavior is more unsystematic in nature, an extremely volatile state, meaning that the reasons for random responding on one scale and not on another are rather idiosyncratic to the individual. This would be reflected by individuals switching behavior multiple times and engaging in random responding rather haphazardly throughout the questionnaire. Switching behavior might not be uncommon in practice. Baer et al. (Citation1997) observed that of the 73% of young adolescents who reported themselves to have engaged in some level of random response behavior on a personality inventory, the majority of respondents indicated this behavior to be scattered across the questionnaire. Similarly, Berry et al. (Citation1992) found that 18–32% of the individuals across different samples reported having engaged in random responding in a random fashion. From this viewpoint, the probability of being identified as a random responder on one scale would be independent of someone’s response behavior on the other scales and consequently, it would not be possible to make any predictions about the validity of the complete set of responses on all scales in the questionnaire.
The literature is scarce and inconclusive on which patterns of within-person variability will be dominant, or even present or absent. Hence, the core research question is exploratory in nature and can be regarded as a step in charting this unknown territory. To study this research question, we selected the 2015 cycle of the Trends in Mathematics and Science Study (Martin et al., Citation2016) as it collected responses from large random samples of students, in multiple educational systems across the world, to a large-scale student survey with many questionnaire scales covering students’ attitudes and beliefs toward relevant subjects that are popular with educational researchers and policymakers alike.
The many methodological approaches to detect random response behavior that rely on auxiliary resources or require long scales with many items (e.g., Rupp, Citation2013) are not applicable to most educational survey research as both features are typically impractical and as a result are absent. In achievement testing, an operationalization utilizing reaction time information on the item level to identify what is labeled a “rapid guess” (see, e.g., Wise et al., Citation2009) has gained traction. A rapid guess is framed as a response given within such a limited time span that it is clear that the individual did not spend sufficient time to consider and process the question asked, and as a consequence provided an essentially random response. Unfortunately, even when the survey is computer-based, item-level reaction times are unattainable as items of a questionnaire scale are typically presented all at once on the screen. Given that there is also no single correct response on a survey item, aberrant responses are less obvious unless they form a very systematic pattern (e.g., diagonal responding across the items of a questionnaire scale) and we can for instance also not identify students that perform below chance level. The use of bogus items or instructed response items (Breitsohl & Steidelmüller, Citation2018; Leiner, Citation2019) is also not commonplace in educational survey research, and the debate is not yet settled on whether these tools are even an ethical practice or effective. In the end, one needs to resort to the pattern of actual item responses given to the different questionnaire scales in the survey. Given the absence of auxiliary elements for the TIMSS 2015 student questionnaire, we will employ a mixture item response theory (IRT) approach (van Laar & Braeken, Citation2022) to explicitly model the possibility of two underlying yet unobserved groups in the population, students engaging in regular response behavior versus students engaging in more random response behavior across the items of a questionnaire scale. The mixture approach is able to classify respondents based on a relative comparison between how likely a student’s item response pattern is under the measurement model for the majority population versus under the independent random responses model. This means that random response behavior is operationalized model-based and at scale-level, directly based on item responses given on that scale by the student, and in normative relative comparison to what the other students responded. This scale-level analysis is perhaps a more coarse operationalization than what would be possible otherwise with auxiliary information at the item level, but this is compensated by the presence of up to 19 scales in the TIMSS 2015 student questionnaire allowing for a sufficient range to explore within-person variability.
Method
TIMSS is an international large-scale assessment of mathematics and science, which has been conducted every 4 years since 1995. TIMSS 2015 provides the sixth assessment of trends in the fourth grade and/or eighth grade of 57 educational systems and seven benchmarking participants. TIMSS 2015 includes assessments of mathematics and science achievement as well as context questionnaires collecting background information (Martin et al., Citation2016). The data used in this study stem from the student questionnaire for the eighth-grade students. The student questionnaire covers not only basic background questions about the students and their home situation but also questionnaire scales about the students’ school experiences, attitudes, and beliefs with respect to school subjects and homework. TIMSS’s target sample size for the number of students to be reached within an educational system is (if student population size and other practicalities permit).
The assessment of the students in TIMSS 2015 was separated into three sections. The students first have the achievement tests, with 45 minutes of testing time per section (i.e., mathematics and science) with a 30-minute break in between. After the achievement tests, a second break was provided; the student questionnaire was administered right after the break. The testing time for the student questionnaire was set at 30 minutes. The total testing time for an eighth-grade student in the TIMSS 2015 assessment (i.e., all 3 sections) is then 120 minutes in total plus the time for the two breaks. The times were set such that in principle students do not need to rush to complete a section. Students were not allowed to leave the room or start with a new section even if they had already completed the task within the set time frame (Martin et al., Citation2016). Hence, there is no reward for rushing through the assessment as students had to remain seated in class and everyone also gets the same break time.
Outcome: random responder status
A mixture item response theory model framework (van Laar & Braeken, Citation2022) was adopted to operationalize and define the target outcome variable of interest, the random responder status of a student on a particular scale in the TIMSS 2015 student questionnaire. The approach assumes that there are two distinct yet unobserved latent groups of responders in the population expressing different response behavior on a questionnaire scale: regular or nonrandom responders and random responders (see ).
Figure 1. Mixture IRT model framework to define and operationalize random responders in terms of independence and uniformity of item responses.

The regular responders are expected to respond consistently according to their own opinions and beliefs related to the questionnaire content of the items on the scale, in line with a traditional latent variable measurement model (see , the “circle” is the common cause of the “squares”) such as the graded response model (Samejima, Citation1969). In contrast, the random responders are expected to provide responses that do not reflect their opinions and beliefs but are more haphazard, in line with a null model implying mutually independent item responses that have an equal chance of falling in either of the possible response categories (see , the “squares” are mutually disconnected, nor influenced by the “circle”; all squares are divided into uniformly equal category parts).
Under the mixture IRT model, the likelihood of a person ‘s item response pattern
(see EquationEquation 1
(1)
(1) ) is written as a weighted sum of the two mentioned model expressions: the joint probability of the observed item response pattern given the person’s latent trait value under the graded response model multiplied by
the prior probability for a person to be a member of the regular responder group plus the joint probability of the observed item response pattern under the null model multiplied by
the prior probability for a person to be a member of the random responder group.
Notice that this mixture model has only one additional to-be-estimated parameter compared to the regular measurement model. The part of the model accommodating the possibility of random responders in the population only has fixed parameters as item response probabilities are known and assumed to be uniformly equal across categories and items. Given that the mixture weights sum up to one by definition (i.e., ), only one extra parameter needs to be estimated.
can be interpreted as a model-based estimate of the prevalence of random responders on the questionnaire scale. The resulting estimated model can be used to classify individuals according to their individual item response pattern in one of the two classes based upon their maximum posterior class membership probability. Thus, on the particular questionnaire scale, an individual student is (classified as) a random responder
if
, and
otherwise. For each scale in the questionnaire, such a mixture IRT model will be estimated and used to compute the random responder status of the individual students having responded to that scale, resulting in a binary profile of random responder status across scales in the questionnaire for each individual.
Quality criteria for use of the mixture classification
If the mixture model for a questionnaire scale failed either of two quality checks, the corresponding random responder status for that scale was set to missing for all students: (1) When the measurement model of the regular responder class had two or more standardized item discrimination parameters (i.e., factor loadings) below .40, the scale was considered unscalable for the majority population (i.e., no clean unidimensional scale structure). (2) When classification entropy dropped below .70 we concluded that the mixture model was unable to provide a good enough distinction between the two latent groups of responders. The first loading-quality criterion requires a strong “clean” measurement model when using the approach to detect random responders because the contrast between the measurement model and the random model is at the crux of the mixture approach. The second entropy-quality criterion requires a good crisp classification (maximum posterior class membership probabilities are high), which in its turn ensures that classification uncertainty is not too prominent when the random responder label is used as an outcome in further analyses.
Study design: sample & student questionnaire
Sample
Inclusion criteria
We study the students participating in TIMSS 2015 in the set of countries that have a so-called separated science program where all four subjects (i.e., biology, chemistry, earth science, and physics) are taught as independent subjects in the curriculum (instead of as part of one big integrated science subject). This choice is motivated by the useful features it brings to our study design: The student questionnaires in these countries contain extra scales and additional structure, as now students’ values and attitudes were asked toward the four different science subjects instead of the single integrated science subject in other countries. The higher number of scales is beneficial for the study of intra-individual variability across scales, and the additional questionnaire structure allows investigating whether subject matter or scale-specifics could be potential triggers for random responding.
Exclusion criteria
Although Malta and Sweden follow a separated science program, their students do not necessarily follow all four science subjects, and hence these countries were excluded from our sample. Lebanon and Morocco were excluded from the sample as the random responder mixture classification did not meet the required quality criteria for the majority of questionnaire scales. The latter points to larger discrepancies in those countries such as the scales not being unidimensional and/or specific items being unscalable.
Effective sample
Applying inclusion and exclusion criteria to TIMSS 2015 results in the following set of seven countries (ISO-code) in our study: Armenia (ARM), Georgia (GEO), Hungary (HUN), Kazakhstan (KAZ), Lithuania (LTU), Russia (RUS), and Slovenia (SVN). In both Armenia and Georgia, a single (but different) questionnaire scale did not meet the classification quality criteria, and here the random responder status was set to missing for all students on that scale in the corresponding country (i.e., Confidence in Chemistry for Armenia and Like Learning Earth Science for Georgia).
TIMSS student questionnaire
The random responder status of a student will be estimated for 19 scales in the TIMSS student questionnaire (see ). These scales were each intended to reflect a unidimensional construct and contained between 7 and 10 Likert items for which a student needed to indicate to what extent s/he agrees with the given statement or indicate how often a specific situation has occurred to them on a 4-point response scale, ranging from 1 (agree a lot or at least once a week) to 4 (disagree a lot or never). The scales cover constructs such as students’ sense of belonging, bullying, value of mathematics, value of science, and a set of three scales on like learning, views on engaging teaching, and confidence in each of five school subjects (mathematics, biology, earth science, chemistry, and physics). Note that the set of starting questions on students’ background and home educational resources will not be considered in our analyses as those were single items of varying response formats that did not form a reflective scale.
Table 1. Overview of scales in the TIMSS 2015 student questionnaire.
Statistical analysis
To determine the random-responder status of each student on different scales, the confirmatory mixture IRT model of van Laar and Braeken (Citation2022) for ordered polytomous indicators was run independently per scale-by-country combination (for sample Mplus syntax, see Appendix A). To determine latent class random responder profiles across the whole questionnaire, an exploratory sequence of unstructured latent class models with the 19 scale-specific random-responder status outcomes from the previous analysis step as binary indicators, was fitted independently per country. The number of classes (i.e., profiles) was determined by means of BIC (e.g., Nylund et al., Citation2007). For generalization and interpretability, we match-aligned the resulting classes across countries. The expectation was that each country would show at the minimum a majority class with a close-to-consistent profile of nonrandom responding across the questionnaire, whereas expectations for the number and profile-type of additional classes were less clear.
Model-implied random responder rates were computed to visualize the latent classes, and these profiles were supplemented by class-specific within-person statistics summaries. For the latter, we first computed for each individual a set of statistics based on their random responder profile (i.e., the binary sequence of their random responder status across scales in the questionnaire). The number of runs (i.e., a sequence of constant random responder status across subsequent scales) and the maximum run length would inform about the individual within-questionnaire consistency. Their switching behavior was more directly quantified through the first-order transition probabilities giving the probability of (not) being a random responder on the current scale given that you were (not) a random responder on the previous scale, i.e., and
. The number of Guttman errors (Guttman, Citation1950) in the binary sequence formed by the profile informed about the level of within-person sequential inconsistency and a high number of errors would be incompatible with the earlier mentioned back-random responding profile.
Both the mixture IRT models and the latent class models were estimated using full-information maximum likelihood in Mplus Version 8.2 (Muthén & Muthén, Citation1998–2017) through the MplusAutomation package for R version 0.7–3 (Hallquist & Wiley, Citation2018), with robust standard errors and the expectation-maximization acceleration algorithm with a standard of 400 random starts, 100 final-stage optimizations, and 10 initial-stage iterations. All analyses accounted for the TIMSS sampling design by applying the total student weights in Mplus for the models and through the survey R package (Lumley, Citation2020) for the descriptive statistics. Analysis scripts were run under R version 4.0.0 (R Core Team, Citation2020).
Results
Descriptives
Quality criteria for use of the mixture classification
In general, a solid unidimensional measurement model surfaced for all but one of the scale-country combinations. The grand average of the average and minimum loading on a scale amounts to .825 and .661, respectively. presents the cross-countries average of the average loading per scale. The loading criterion was not satisfied for the “Students Like Learning Earth Science” scale in Georgia (GEO), with items 2 and 3 having standardized factor loadings of only around .30 (the other items having loadings between .765 and .963).
Similarly, for all but one of the 133 scale-country combinations, the resulting classification by means of the mixture model leads to a crisp classification. The entropy criterion was not satisfied for the “Students Confident in Chemistry” scale in Armenia, with entropy = .695, just below the a priori set threshold. For combinations meeting the quality criteria, we report in the cross-country average of (i) the entropy of the mixture classification, (ii) the average of the students’ maximum posterior class membership probability, and (iii) the percentage of students having a maximum posterior class membership probability below .60. The latter are the individuals that balance between being assigned to the random responder or regular responder class. Overall, with the grand average of the entropy and the maximum posterior class membership probability being .901 and .973, respectively, and a grand average of only 1.40% of students having a maximum posterior class membership probability below .60, the mixture approach leads to a solid and crisp classification in random and regular responders.
Given that out of the 133 scale-country combinations, there are only two cases that do not meet the quality criteria, their in/exclusion status will in principle not heavily impact the results of further analyses. Still, both cases will be excluded from further analyses, because we feel it is important to signal and communicate conservative proper practice when implementing an approach to detect random responders.
Random responder (
) prevalence
About 4500 students filled in the TIMSS 2015 student questionnaire in each of the seven countries, with sample sizes ranging from in Georgia to
in Armenia. The prevalence of random responders among students in these countries varied across the scales, with the minimum
prevalence observed on the Student Bullying scale (across-countries median prevalence = 0%) and the maximum
prevalence on the Students Confident in Physics scale (across-countries median prevalence = 17%). On average, Georgia had the highest median within-person
proportion across scales (11% or 2 out of 18 scales), while at least half of the students were not identified as
on any scale (median within-person
proportion = 0%) in 4 out of 7 countries (i.e., Kazakhstan, Lithuania, Russia, and Slovenia).
Missing random responder status
When no item responses on an entire scale were observed for an individual student, there was also no data to assign a posterior class membership to the student and the student’s random responder status on that scale was set to missing. About 4% of the students did not have a random responder status on 1 to 2 scales, and for another 4% this was the case on 3 or more scales. Across countries, slightly higher missingness percentages were observed for Georgia (12% missed 1 to 2 scales and 6% missed 3 or more scales) and Armenia (6% missed 1 to 2 scales and 6% missed 3 or more scales). Reasons underlying the missing responses are unknown and could be ascribed to a multitude of factors leading the student to either purposefully or accidentally skipping a page and as such an entire scale of the questionnaire. The missingness was generally observed to be randomly distributed across scales with missingness percentages on average below 6%. The exception was Georgia where missingness was concentrated on scales linked to the earth science subject (up to 10% of students were missing their random responder status on these scales). Yet, across all countries, the majority of students, on average about 92% (ranging from 82% in Georgia to 97% in Lithuania and Russia), had a random responder status () for each of the administered scales in the TIMSS 2015 student questionnaire. Thus, for the subsequent latent class analyses modeling random responder profiles across scales, a student’s missing random responder status on a scale will be treated as missing at random. When computing the within-person descriptive statistics, a student’s random responder status pattern across scales is used as is, skipping missing classifications (i.e., this implies that a missing status on a scale does not break a run). This treatment brings a slight within-person consistency bias, but given the low amount and randomness of missings, the inferential impact can be expected to be limited.
Latent class profiles of random responder status across the questionnaire
When determining the number of latent classes, the normalized BIC plot showed uniformly across countries a huge drop after one class followed by a quadratic inverse U-shape with the minimum at 4 (see ). To complement the relative perspective offered by the normalized BIC plot, we also computed model-weights based on raw BIC values (Wagenmakers & Farrell, Citation2004). Model weights for the 4-class solution were close to the boundary value of 1, clarifying that the 4-class solution is also the single preferred solution. Entropy values for the 4-class models ranged from .73 to .82, indicating that students could be classified in a rather clear crisp fashion across the classes. These model comparison results support the hypothesis of population heterogeneity in terms of random responding across the scales in the student questionnaire and suggest there to be four distinct latent profiles in each of the seven countries.
Figure 2. Normalized Bayesian information criteria as a function of the number of latent classes.

displays the resulting random responder status probability profiles for the four-class solution in each of the seven countries. For each class, the vertical axis represents the probability of having a positive random responder status on a given scale in the student questionnaire. On the horizontal axis, the respective scales are listed in order of occurrence in the questionnaire and can be grouped (cf. dotted gray vertical lines) in terms of the particular subject domain (cf. single gray letter) they relate to.
Figure 3. Random responder status probability profiles for the four-class solution.

The four classes corresponded to four distinct random responder across-scales profiles that could be neatly matched across countries. provides per class an overview of relevant within-person statistics that can help to further characterize what type of within-person random responder across-scales patterns can be observed in each of the four classes.
Table 2. Class sizes and class averages of within-person statistics across seven countries.
Consistent nonrandom responders
The profile for the majority class (: lightest gray with diamond points) indicates close to zero probabilities of a positive random responder status for all scales, suggesting that this class bundles students that are (almost) never classified as a random responder on any of the scales. This is further corroborated by an across-students average within-person maximum run length of a zero random responder status close to the total of scales in the questionnaire, a first-order transition probability close to 1, and hardly any Guttman errors (). All these average within-person statistics imply large within-person consistency and point to generally (close-to) all-zeroes random responder status patterns for students in this class.
Random responders triggered exclusively by the confidence scales
The profile for a second class runs rather parallel with the majority class, were it not for the substantially higher probabilities of positive random responder status on the five subject-specific confidence scales (: darkest gray with square points). Notice that this pattern indeed repeats across countries, although the peaks at the confidence scales do vary in height across countries (in Armenia the Confidence in Chemistry scale is absent as it did not meet quality criteria). This profile suggests that this class bundles students that are exclusively classified as random responders on the confidence scales, and not elsewhere in the questionnaire. This is further corroborated by students having on average a positive random responder status on 57% of the Confidence scales, but on only 6% of the other scales in the questionnaire.
Frequent random responders
The response probability profile for the minority class (: dark gray with circle points) has the highest probabilities of a positive random responder status for all scales, suggesting that this class bundles students that are frequently classified as a random responder on any of the scales (i.e., on average on about 43% of the scales). The individual student patterns in this class are far from consistent, with transition probabilities close to 50/50 in either way, and many Guttman errors (see ).
Intermittent moderate random responders
The profile for the third class indicates non-zero but low probabilities of a positive random responder status for most scales (: light gray with triangle points). Individual students have on average on about 3 scales (i.e., 16% of the questionnaire) a positive random responder status, spread out across two runs, and result in on average 4 Guttman errors (see ). Together with the response profile of this class, these within-person statistics results imply that random responding in this class is more intermittent across the questionnaire and without clear systematic trends across students in this class.
Back-random responders
None of the class profiles corresponded to the pattern you would expect under back-random responding. Among all 32,086 students, there were only 590 students who showed a non-zero proportion of random responding in combination with zero Guttman errors, a set of within-person statistics that would surface under back-random responding. Yet of those 590 students, 506 responded randomly only to the last scale (and 55 to the two last scales). Similarly, among all 32,086 students, only 34 and 114 students had 4 out of the last 6 scales and 3 out of the last 5 scales a random responder status and a non-responder status elsewhere. These findings seem to suggest that the occurrence of back-random responding is at most a rarity in our sample with the TIMSS 2015 student questionnaire.
Discussion
In this study, we explored intra-individual variability in random responding behavior across questionnaire scales in the TIMSS 2015 student questionnaire. The objective was to clarify to what extent random responding is a more systematic trait-like behavior or more state-like as in activated once a personal threshold has been breached (cf. back-random responding) or when triggered by specific contents or type of a scale, or more haphazard due to more idiosyncratic instances.
Our latent class analyses uniformly converged on a four-class solution with four distinct random responding profiles that generalized well, both in class size and in profile character, across the seven countries under study. Do note that the sample contains eighth-grade students in countries with a separated science program in their school curriculum and that are mostly located in Eastern Europe. Hence, there are potentially some shared contextual influences that need to be taken into account when extrapolating results outside this age group or toward other countries elsewhere.
The identified majority class reflects within-person profiles in which no random responding occurs on scales throughout the questionnaire. Although the students have nothing to gain or lose from filling in the low-stakes questionnaire, the majority appear to respond to the scales in a rather construct-consistent manner. This is a reassuring finding for TIMSS 2015, and by extension also for the potential of other low-stakes educational surveys.
In contrast, the identified minority class reflects within-person profiles that randomly responded on almost half of the scales in the questionnaire. This class profile can be speculated to correspond well to explanations of random responding in terms of carelessness, insufficient effort, or lack of motivation and seriousness on behalf of the respondent (e.g., Huang et al., Citation2012). The relatively high frequency of random responding also questions the general trustworthiness of the delivered responses on the questionnaire by those students, even on scales for which the student was not classified as a random responder.
A slightly larger class shows an intermittent random responding pattern across the questionnaire with a much more moderate frequency of occurrence, about 3 scales or 16% of the questionnaire. Here, random responding can be considered more incidental due to undefined idiosyncratic features and occasional lapses of engagement by the student. To the extent that this is indeed a reflection of completely-at-random events, data quality and inferences should remain relatively unharmed.
In contrast to the former classes for which there were no obvious systematic observable triggers for the random response behavior, a fourth class reflected within-person profiles where the students responded randomly but exclusively to the confidence scales. Such a systematic pattern cannot be ascribed to momentary lapses in engagement or insufficient effort, nor to response type artifacts (4-point Likert items were used uniformly across the questionnaire), but we ought to look at item contents. Participants in a study by Baer et al. (Citation1997) also reported that their core reasons for random responding were not lapses of concentration or boredom, but mostly difficulties in understanding items or deciding on the response. A similar phenomenon could be at play here. Perhaps the students in this latent class genuinely find it uncomfortable to publicly disclose their confidence in school subjects? Examples of items on such a confidence scale are for instance “mathematics is harder for me than any other subject” or “mathematics is more difficult for me than for many of my classmates.” Students’ perceptions about themselves are always made in comparison to some standard, either internally (i.e., own performance in one subject with own performance in another subject) or externally (own performance with the performance of other students) (e.g., Marsh & Hau, Citation2004). Items that require comparisons, with additional changing or ambiguous standards and definitions of self, might just be more difficult to answer or could result in internal inconsistencies in perception for certain individuals. Hence, one cannot exclude the possibility that these students in fact provided genuine valid responses from their individual viewpoints. This type of more systematically triggered random responding could be considered more harmful than the intermittent random responding and, in our particular case, this raises questions for the validity and data quality of the confidence scales in the questionnaire.
We found no support for the so-called back-random responding profiles (e.g., Clark et al., Citation2003; Gallen & Berry, Citation1997), where students are assumed to switch from regular responding to random responding once they reached their “threshold.” The lack of back-random responding implies that explanations in terms of a full-blown depletion of internal cognitive resources or alternatively a firm conscious decision to no longer actively engage with the questionnaire are not applicable. TIMSS states that there is ample time for the student to fill in the questionnaire, that the general task demands of the assessment are not out of bounds, and that there is also no benefit in rushing through the survey (one has to stay in class anyhow for the allotted time). Hence, this non-speeded no-rush low-stakes character of the questionnaire potentially sets (part of) the context to the null-finding on back-random responding, and we caution against generalizing this finding to educational surveys under more rushed speededness conditions.
Currently, we were restricted to implementing a scale-level mono-method assessment of random responding (van Laar & Braeken, Citation2022), which operationalized random responding as providing item responses on a scale more alike patterns resulting from an independent random response model than alike the response patterns consistent with the measurement model for the “regular” population. Hence, the effectivity of the method in detecting random responders relies on the adequacy of this measurement model for capturing individual differences in item response behavior. If a scale would for instance be bidimensional for the majority group, the measurement model would need to be modified to accommodate this. There is also the possibility that the population heterogeneity is even more complex and that multiple classes with different measurement models would need to be integrated in the model. In our study, the scales considered all have a theoretical basis for being unidimensional and fulfill the basic loading quality criterion empirically indicating uniformly strong measurement models in the mixture. Hence, we have not pursued further model comparisons with respect to the measurement model used beyond the unidimensional model in the mixture approach.
With the granularity of the method at the scale level, we cannot say which particular items have been randomly responded to and might have missed respondents who would systematically randomly respond to particular individual items regardless of the scale. For instance, respondents who would only answer randomly to the last item of each scale or who are triggered by the presence of a certain word in an item regardless of the scale contents. At the inferential level, the current approach also ignores the classification uncertainty in random responder status assessment as the maximum posterior binary membership classification is used as a binary outcome. To allow a broader grip and strengthen the detection of random responders, auxiliary data is needed that allows for a multi-method approach using for instance bogus items (e.g., “I am not in grade eighth”), instructed-response items (e.g., “Please mark slightly agree”), duplicate items (cf. so-called lie-scales in personality questionnaires), and the provision of individual survey completion speed indicators (e.g., Leiner, Citation2019).
Conclusion
Whereas noncompliance and manipulation checks have become more and more a default part of experimental design, similar data quality checks or monitoring procedures are not yet commonplace in educational science research using questionnaires and surveys. Ethical questions remain as this monitoring needs to be communicated to the participants and potentially creates an atmosphere of mistrust and ambiguity, which might also aversively affect the quality of response. From a perspective of implementing a monitoring system, the intermittent character of random response behavior in one of the classes does not hold much promise for the value of an early warning monitoring system and one might even wonder whether the added value of monitoring outweighs the potential negative impact of the “big brother is watching you” impression that such a monitoring system might bring along to the students. The confidence-exclusive class also sketches that, in a questionnaire context where there is no objectively correct answer, the label “random” might also be a misnomer; let alone imagine the potential mishap when one would ascribe it to insufficient effort and actively communicate it as such to a participant. Given these practical complications and the finding that the majority of students do not end up as random responders in the survey, we would advise against implementing an active monitoring system with early warnings for the students. Instead, we would advocate for a passive monitoring system, including survey completion speed indicators, to support post-survey response data quality checks and including student-level diagnostic indicators in the publicly available datasets of the survey to allow secondary data analysts to run proper sensitivity checks to assure robustness of their research findings. Next to enabling such sensitivity checks, we should also not underestimate proper survey design and here one can step things up in educational research by valuing the power of cognitive labs and the feedback of survey panels that are not filled with “academic experts” on the constructs to be measured, but with members of the actual target group. The information these panels bring can potentially help detect, before any large-scale implementation, the unintended triggers to random response behavior in the questionnaire.
Acknowledgments
This study was supported by a research grant [FRIPRO-HUMSAM261769] for young research talents of the Norwegian Research Council.
Disclosure statement
No potential conflict of interest was reported by the author(s).
Additional information
Funding
References
- Ackerman, P. L., & Kanfer, R. (2009). Test length and cognitive fatigue: An empirical examination of effects on performance and test-taker reactions. Journal of Experimental Psychology: Applied, 15(2), 163–181. https://doi.org/10.1037/a0015719
- Baer, R. A., Ballenger, J., Berry, D. T. R., & Wetter, M. W. (1997). Detection of random responding on the MMPI–A. Journal of Personality Assessment, 68(1), 139–151. https://doi.org/10.1207/s15327752jpa6801_11
- Berry, D. T. R., Wetter, M. W., Baer, R. A., Larsen, L., Clark, C., & Monroe, K. (1992). MMPI-2 random responding indices: Validation using a self-report methodology. Psychological Assessment, 4(3), 340–345. https://doi.org/10.1037/1040-3590.4.3.340
- Bowling, N. A., Gibson, A. M., Houpt, J. W., & Brower, C. K. (2021). Will the questions ever end? Person-level increases in careless responding during questionnaire completion. Organizational Research Methods, 24(4), 718–738. https://doi.org/10.1177/1094428120947794
- Bowling, N. A., Huang, J. L., Bragg, C. B., Khazon, S., Liu, M., & Blackmore, C. E. (2016). Who cares and who is careless? Insufficient effort responding as a reflection of respondent personality. Journal of Personality and Social Psychology, 111(2), 218–229. https://doi.org/10.1037/pspp0000085
- Breitsohl, H., & Steidelmüller, C. (2018). The impact of insufficient effort responding detection methods on substantive responses: Results from an experiment testing parameter invariance. Applied Psychology, 67(2), 284–308. https://doi.org/10.1111/apps.12121
- Cao, J., & Stokes, S. L. (2007). Bayesian IRT guessing models for partial guessing behaviors. Psychometrika, 73(2), 209–230. https://doi.org/10.1007/s11336-007-9045-9
- Clark, M. E., Gironda, R. J., & Young, R. W. (2003). Detection of back random responding: Effectiveness of MMPI-2 and personality assessment inventory validity indices. Psychological Assessment, 15(2), 223–234. https://doi.org/10.1037/1040-3590.15.2.223
- Credé, M. (2010). Random responding as a threat to the validity of effect size estimates in correlational research. Educational and Psychological Measurement, 70(4), 596–612. https://doi.org/10.1177/0013164410366686
- Cronbach, L. J. (1946). Response sets and test validity. Educational and Psychological Measurement, 6(4), 475–494. https://doi.org/10.1177/001316444600600405
- Cronbach, L. J. (1950). Further evidence on response sets and test design. Educational and Psychological Measurement, 10(1), 3–31. https://doi.org/10.1177/001316445001000101
- Curran, P. G. (2016). Methods for the detection of carelessly invalid responses in survey data. Journal of Experimental Social Psychology, 66, 4–19. https://doi.org/10.1016/j.jesp.2015.07.006
- Eccles, J., & Wigfield, A. (2002). Motivational beliefs, values and goals. Annual Review of Psychology, 53(1), 109–132. https://doi.org/10.1146/annurev.psych.53.100901.135153
- Eklöf, H. (2010). Skill and will: Test-taking motivation and assessment quality. Assessment in Education: Principles, Policy & Practice, 17(4), 345–356. https://doi.org/10.1080/0969594X.2010.516569
- Fleeson, W. (2004). Moving personality beyond the person-situation debate: The challenge and the opportunity of within-person variability. Current Directions in Psychological Science, 13(2), 83–87. https://doi.org/10.1111/j.0963-7214.2004.00280.x
- Gallen, R. T., & Berry, D. T. R. (1997). Partially random MMPI-2 protocols: When are they interpretable? Assessment, 4(1), 61–68. https://doi.org/10.1177/107319119700400108
- Guttman, L. (1950). The basis for scalogram analysis. In S. A. Stouffer, L. Guttman, E. A. Suchman, P. Lazarsfeld, S. A. Star, & J. A. Clausen (Eds.), Measurement and prediction (pp. 66–90). Princeton University Press.
- Hallquist, M. N., & Wiley, J. F. (2018). MplusAutomation: An R package for facilitating large-scale latent variable analyses in Mplus. Structural Equation Modeling: A Multidisciplinary Journal, 25(4), 621–638. https://doi.org/10.1080/10705511.2017.1402334
- Huang, J. L., Curran, P. G., Keeney, J., Poposki, E. M., & DeShon, R. P. (2012). Detecting and deterring insufficient effort responding to surveys. Journal of Business and Psychology, 27(1), 99–114. https://doi.org/10.1007/s10869-011-9231-8
- Leiner, D. J. (2019). Too fast, too straight, too weird: Non-reactive indicators for meaningless data in internet surveys. Survey Research Methods, 13(3), 229–248. https://doi.org/10.18148/srm/2019.v13i3.7403
- Linnenbrink, E. A., & Pintrich, P. R. (2002). Motivation as an enabler for academic success. School Psychology Review, 31(3), 313–327. https://doi.org/10.1080/02796015.2002.12086158
- Liu, T., Sun, Y., Li, Z., & Xin, T. (2019). The impact of aberrant response on reliability and validity. Measurement: Interdisciplinary Research and Perspectives, 17(3), 133–142. https://doi.org/10.1080/15366367.2019.1584848
- Lumley, T. (2020). Survey: Analysis of complex survey samples. [R package version 4.0].
- Maniaci, M. R., & Rogge, R. D. (2014). Caring about carelessness: Participant inattention and its effects on research. Journal of Research in Personality, 48, 61–83. https://doi.org/10.1016/j.jrp.2013.09.008
- Marsh, H. W., & Hau, K. -T. (2004). Explaining paradoxical relations between academic self-concepts and achievements: Cross-cultural generalizability of the internal/external frame of reference predictions across 26 countries. Journal of Educational Psychology, 96(1), 56–67. https://doi.org/10.1037/0022-0663.96.1.56
- Martin, M. O., Mullis, I. V. S., & Hooper, M. (2016). Methods and procedures in TIMSS 2015. TIMSS & PIRLS International Study Center.
- Meade, A. W., & Craig, S. B. (2012). Identifying careless responses in survey data. Psychological Methods, 17(3), 437–455. https://doi.org/10.1037/a0028085
- Messick, S. (1984). The psychology of educational measurement. Journal of Educational Measurement, 21(3), 215–237. https://doi.org/10.1111/j.1745-3984.1984.tb01030.x
- Messick, S. (1991). Psychology and methodology of response styles. In R. E. Snow & D. E. Wiley (Eds.), Improving inquiry in social science: A volume in honor of Lee J. Cronbach (pp. 161–200). Lawrence Erlbaum.
- Molenaar, P. C. M. (2004). A manifesto on psychology as idiographic science: Bringing the person back into scientific psychology, this time forever. Measurement: Interdisciplinary Research and Perspectives, 2(4), 201–218. https://doi.org/10.1207/s15366359mea0204_1
- Muthén, L. K., & Muthén, B. O. (1998–2017). Mplus user’s guide (8th ed.). Muthén & Muthén.
- Nylund, K. L., Asparouhov, T., & Muthén, B. O. (2007). Deciding on the number of classes in latent class analysis and growth mixture modeling: A Monte Carlo simulation study. Structural Equation Modeling: A Multidisciplinary Journal, 14(4), 535–569. https://doi.org/10.1080/10705510701575396
- Osborne, J., Simon, S., & Collins, S. (2003). Attitudes towards science: A review of the literature and its implications. International Journal of Science Education, 25(9), 1049–1079. https://doi.org/10.1080/0950069032000032199
- Pinsoneault, T. B. (2007). Detecting random, partially random, and nonrandom Minnesota multiphasic personality inventory-2 protocols. Psychological Assessment, 19(1), 159–164. https://doi.org/10.1037/1040-3590.19.1.159
- Potvin, P., & Hasni, A. (2014). Interest, motivation and attitude towards science and technology at K-12 levels: A systematic review of 12 years of educational research. Studies in Science Education, 50(1), 85–129. https://doi.org/10.1080/03057267.2014.881626
- R Core Team. (2020). R: A language and environment for statistical computing. R Foundation for Statistical Computing.
- Rubin, D. B. (1976). Inference and missing data. Biometrika, 63(3), 581–592. https://doi.org/10.1093/biomet/63.3.581
- Rupp, A. A. (2013). A systematic review of the methodology for person fit research in item response theory: Lessons about generalizability of inferences from the design of simulation studies. Psychological Test and Assessment Modeling, 55(1), 3–8. https://www.psychologie-aktuell.com/fileadmin/download/ptam/1-2013_20130326/01_Rupp.pdf
- Samejima, F. (1969). Estimation of latent ability using a response pattern of graded scores. Psychometrika, 34(1), 1–97. https://doi.org/10.1007/BF03372160
- Schmitt, M., & Blum, G. S. (2020). State/Trait interactions. In V. Zeigler-Hill & T. K. Shackelford (Eds.), Encyclopedia of personality and individual differences (pp. 5206–5209). Springer International Publishing.
- Soland, J., & Kuhfeld, M. (2019). Do students rapidly guess repeatedly over time? A longitudinal analysis of student test disengagement, background, and attitudes. Educational Assessment, 24(4), 327–342. https://doi.org/10.1080/10627197.2019.1645592
- van Laar, S., & Braeken, J. (2022). Random responders in the TIMSS 2015 student questionnaire: A threat to validity? Journal of Educational Measurement, 59(4), 470–501. https://doi.org/10.1111/jedm.12317
- Wagenmakers, E. -J., & Farrell, S. (2004). AIC model selection using Akaike weights. Psychonomic Bulletin & Review, 11(1), 192–196. https://doi.org/10.3758/BF03206482
- Wise, S. L. (2006). An investigation of the differential effort received by items on a low-stakes computer-based test. Applied Measurement in Education, 19(2), 95–114. https://doi.org/10.1207/s15324818ame1902_2
- Wise, S. L., Pastor, D. A., & Kong, X. J. (2009). Correlates of rapid-guessing behavior in low-stakes testing: Implications for test development and measurement practice. Applied Measurement in Education, 22(2), 185–205. https://doi.org/10.1080/08957340902754650