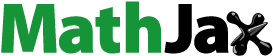
ABSTRACT
To improve the fuel cell durability of the hydrogen Electric Multiple Units, this paper proposes a novel multi-stack fuel cell hybrid system energy management strategy in consideration of fuel cell degradation. The top layer of the method distributes the power of fuel cells and lithium batteries reasonably according to the Equivalent Consumption Minimization Strategy, and adjusts the fuel cell power through the feedback power regulation module. The bottom layer distributes the power of different stacks according to the degradation degree. The proposed layering method improves the durability of fuel cells by reducing the fuel cell degradation degree. The hardware-in-the-loop (HIL) experiments results show that, compared with the traditional equivalent hydrogen consumption minimum energy management strategy, the proposed method is effective in lowering the degradation degree and operating pressure of the fuel cell by 25.77% and 35.73% respectively.
1. Introduction
Global warming and air pollution caused by the use of fossil energy have a devastating impact on human life. Hydrogen energy can be used to replace traditional fossil energy. The current development of hydrogen fuel cell has been relatively mature, and is gradually approaching industrialization and commercialization (Huangfu et al. Citation2018; Li et al. Citation2021; Zhou et al. Citation2018).
Urban Electric Multiple Units (EMUs) are new rail transit products developed for urban railways. It not only has the advantages of large passenger capacity, fast start and stop of metro vehicles, but also has the advantages of high-speed EMU with high speed and comfort. The application of fuel cells to EMUs improves the application of hydrogen energy in intercity rail transportation, thus the research on the fuel cell for EMUs provides new ideas for the development of intercity transportation (Chen et al. Citation2018; Meng and; Li et al. Citation2021).
However, due to the limited output power, poor durability, and high cost of fuel cells, multi-stack fuel cell system (MFCS) is usually used to make up for the above deficiencies in the applications of EMUs. Meanwhile, because of the unidirectional nature of fuel cell output power as well as poor dynamic response characteristics, it is often necessary to form an electric-hydrogen hybrid system together with auxiliary power sources such as batteries and supercapacitors (Li et al. Citation2022; Peng and Bucknall Citation2020).
For the fuel cell hybrid power system, from the perspective of system durability, the fuel cell output power should be maintained in the operation process of the smooth output, from the perspective of economy, the fuel cell should work as much as possible in the high-efficiency point, so the durability of the hybrid power system and the economy is actually a game of two goals. Reasonable energy management strategy can not only ensure that the train has enough power to run, but also optimize the power distribution between the fuel cell and the lithium battery, thus improving the economy and durability of the power system. In recent years, rule-based, optimization-based and artificial intelligence-based strategies are dominant types in power allocation (Li et al. Citation2019, Citation2023; Marx et al. Citation2014).
Rule-based power allocation strategies are widely used in the engineering field because of their simplicity and real-time characteristics (Diana, Javier, and Loïc Citation2020; Duan et al. Citation2017). The setting of rules usually relies on engineering experience or experimental data, and the system operation state is divided into different sub-modes according to the parameters such as fuel cell power and SOC of lithium battery, and then the rules of power allocation are set in each sub-mode. These rules are usually based on experience, so they are often not the optimal solutions. Besides, the rules can be specifically classified as deterministic rules and fuzzy rules. Thereof, fuzzy rules energy management strategies are more robust and often optimize the affiliation function by genetic algorithms or neural networks to reduce the operating cost of hybrid systems. Ridong Zhang and Tao (Citation2018) optimized a fuzzy control strategy containing a low-pass filter with a genetic algorithm to smooth the power oscillations and hydrogen consumption of fuel cells.
Optimization-based power allocation strategies can better compensate for the drawbacks of rule-based energy management strategies. The strategies optimize the control objective by defining an energy cost function and minimizing it in combination with constraints. Usually, the hydrogen consumption of the hybrid system is used as the control objective to form a single-objective control under the constraints, but there are also multi-objective optimization controls that use fuel cell durability, lithium-ion battery durability, and driving performance as the control objectives at the same time. Further, optimization-based energy management strategies can be classified into instantaneous optimization-based and global optimization-based strategies depending on the optimization period. The optimization-based energy management strategies mainly include Pontryagin Minimum Principle (PMP), Equivalent Consumption Minimization Strategy (ECMS), Dynamic Programming (DP), and Model Predictive Control (MPC) (Anselma et al. Citation2020; Kandidayeni et al. Citation2020; Peng et al. Citation2021; Zheng, Xu, and Park Citation2014). Yalian Yang et al. (Citation2016) evaluated the economy of different powertrain configurations of vehicles using DP algorithms. Xiyun Li et al. (Citation2019) improved the traditional Markov chain prediction method so that it is more accurate for all cycle conditions.
With the development of artificial intelligence (Legala, Zhao, and Li Citation2022; Wang et al. Citation2023), a machine learning algorithm, Reinforcement Learning Algorithm (RL), is applied to energy management strategies. RL is an algorithm in which the agent learns by “trial and error.” This algorithm guides the action of the agent through the rewards it receives from interacting with the environment, and its goal is to maximize the rewards the agent receives. Reinforcement learning is used for hybrid tracked vehicle energy management to achieve better fuel economy (Liu et al. Citation2015; Qi, Luo, and Wu Citation2017) designed a deep reinforcement learning-based real-time energy management strategy that combines reinforcement learning and deep neural networks.
Currently, although more work has turned to the study of fuel cell deterioration, the amount of the maximum power fluctuation of fuel cells is still considered a constant value (Wang, Advani, and Prasad Citation2020). In addition, there are fewer studies on the overall system endurance degradation caused by the performance differences between different power stacks (Zhou, Ravey, and Pera Citation2020).
Aiming at bridging the above gaps, this paper develops a novel hierarchical energy management strategy of the multi-stack fuel cell hybrid system for EMUs in consideration of fuel cell degradation (HEM-FCD). The main contributions of the paper are listed as follows:
In order to characterize fuel cell degradation, a novel index, the fuel cell degradation degree, is defined by analyzing the main factors contributing to fuel cell degradation
HEM-FCD is proposed. The top layer of the proposed method achieves optimal economics based ECMS. Besides, a method for power adaptive correction is applied to delay the influence of variable load power on the performance of multi-stack fuel cell hybrid systems. The bottom layer defines the fuel cell degradation rate to characterize the performance difference between different stacks and achieves power allocation between different electrical stacks based on this index to reduce fuel cell degradation.
Based on the RT-LAB hardware-in-the-loop experimental platform, the proposed power allocation method is compared with the traditional Finite-State Machine (FSM) and the ECMS to prove the effectiveness and superiority of the method.
In terms of the structure of this paper, the parameters of multi-stack fuel cell hybrid power system and power source characteristics are introduced in section 2. Section 3 defines the fuel cell system degradation degree. HEM-FCD is introduced in section 4. Section 5 analyzes the experimental results and summarizes the conclusions.
2. Fuel cell hybrid system
shows the topology of the multi-stack fuel cell hybrid system for urban EMUs in this paper. The main power source of the EMU is composed of two sets of hydrogen fuel cells, providing power to the EMU and linked to the bus with a unidirectional DC/DC converter. As an auxiliary energy source, the lithium titanate battery cooperates with the fuel cell by connecting to the system through a bidirectional DC/DC converter. Moreover, the DC/DC converter mainly serves to manage the power of the hydrogen fuel cell and the battery.
2.1. Fuel cell system
The fuel cell system used in this paper consists of two sets of Ballard HD6 V2 type 150 kW PEMFC stacks, and its main technical parameters are shown in . Based on the data in , the polarization curve of the fuel cell is shown in .
Table 1. Fuel cell parameters.
2.2. Battery model
In this paper, the lithium titanate battery is selected as the auxiliary power source of the hybrid system, and the equivalent circuit model is shown in (1).
where, Uocv is the open circuit voltage of the battery(V); Ibat is the output current of the battery(A); Rinternal is the internal resistance of the battery(Ω). Uocv and Rinternal are both related to battery state of charge (SOC) and can be fitted as a polynomial function of the SOC. However, since Rinternal varies less with SOC, Rinternal is taken as a constant in this paper.
In addition, the Uocv -SOC is fitted with first-order functions to ninth-order functions, respectively, and the fitting accuracy is evaluated with the fitting factor R2. The closer the R2 is to 1, the higher the accuracy of the fit is. In this paper, it is found that the highest fitting accuracy is achieved when the third-order function is used to fit, and the value of R2 reaches 0.9486 as shown in .
The charging and discharging efficiency ηchg and ηdis of the lithium battery can be derived as shown in (2) based on the Rint model.
where, Pbat is the charging and discharging power of the battery(W).
3. Fuel cell system degradation analysis
The main phenomenon of the hydrogen fuel cell degradation is the significant reduction of fuel cell voltage (Zhou et al. Citation2017). Analyzing the main factors causing fuel cell degradation, this paper defines a degree of fuel cell degradation. This indicator can represent the current performance status of the fuel cell in real time and the influence of different operating conditions on the fuel cell system, and then a real-time adjustment can be made through a power allocation strategy.
The operating conditions of the fuel cell system for EMUs are usually classified into four main loads: start-stop load, variable load, high-power load, and low-power load. Under the above working circumstances, the start-stop load leads to extremely significant degradation of the fuel cell. Therefore, to prevent unnecessary degradation, the whole operation will not switch off the fuel cell system in this paper (Gazdzick et al. Citation2016). Analyzing the above operating conditions, the degree of the fuel cell degradation L is calculated as (3).
where, e1, e2, and e3 are the coefficients derived from PEMFC aging tests; Pfc is the instantaneous output power of the fuel cell(W); Pfcmin and Pfcmax are the minimum and maximum values of fuel cell output power respectively(W); Phighlim and Plowlim are the bottom limit of the high-power range and the top limit of the low-power range respectively(W); represents the voltage decay variation rate of fuel cells; Lhigh and Llow are the high power degradation factor and low power degradation factor(%), which represent the fuel cell degradation when it operates in the high power range and low power range respectively; Lchange is the variable load degradation factor(%); Lstart/stop is the start/stop load degradation factor, which represents the voltage degradation caused when the fuel cell starts and stops(%), and it is considered to be a constant value as the hybrid system starts and stops only once during the driving cycle.
During the electrochemical reaction of fuel cells, three kinds of voltage losses lead to the performance degradation of fuel cells, which are ohmic polarization voltage, activation polarization voltage, and concentration difference polarization voltage. When the operating conditions of the fuel cell fluctuate frequently, the increase in ohmic polarization voltage is the main cause of fuel cell degradation (W., F. and C. et al. 2002). At this time, the voltage loss is mainly created by the internal resistance divider, and the violent fluctuation of the current is the main reason for the increase of the internal resistance. Therefore, the standard deviation of the output power of the fuel cell is used to characterize its output power fluctuation. Thus, the variable load degradation factor is calculated as shown in (4).
where, Pfc|t is the fuel cell output power at the current moment(W); Pfc|t-1、Pfc|t-2、Pfc|t-3 and Pfc|t-4 are the fuel cell power in the last four time steps(W); δ is the standard deviation function to get the standard deviation of the fuel cell power in five time steps; uδ is the voltage loss per unit standard deviation(V).
Therefore, during regular fuel cell operation, the degradation degree L is composed of the corresponding degradation factors under different operating conditions, and it can be calculated as shown in (5).
where, Videal is the ideal fuel cell output voltage(V); β is the fuel cell degree of degradation when it is retired(%) (Zhang et al. Citation2014); t1 and t2 represent the beginning and finishing times of the fuel cell operating at the high-power load respectively(s); t3 and t4 represent the beginning and finishing times of the fuel cell operating between the bottom limit of high-power and the top limit of low-power respectively(s); t5 and t6 represent the starting and ending times of the fuel cell operating at the low-power load respectively(s).
Among the four degradation factors, the variable load degradation factor and high-power degradation factor are the main contributors to the growth of fuel cell degradation. Therefore, this paper focuses on improving the performance of MFCS by reducing the variable load degradation factor and high-power degradation factor.
4. Hierarchical energy management strategy in consideration of fuel cell degradation
Considering the impact of fuel cell systems degradation, a hierarchical energy management strategy is developed to enhance the overall durability of the MFCS in this paper. The objective of the top layer is to minimize the hydrogen consumption of the system and to decrease fluctuations of the power output as much as possible, while the bottom layer allocates the power among the different stacks according to their degradation degree. The durability of the MFCS is optimized by the variable load degradation factor Lchange and the high-power load degradation factor Lhigh in the top and bottom layers respectively, to reduce the operating pressure and degradation of the fuel cell system. shows the HEM-FCD flowchart.
4.1. Top layer of the energy management strategy
The aim of the top layer is to minimal the consumption and maintain the battery SOC. The optimal fuel cell power Pfc can be solved through establishing relevant rules as well as objective functions. Considering the real time and economic performance, ECMS is selected as the energy management strategy to distribute the power between different power supplies.
ECMS enables the allocation of power to the system based on numerical optimization principles. Although lithium battery energy consumption is generally measured in electrical energy. ECMS allows for the equivalence of electrical energy to hydrogen consumption facilitating the establishment of an objective function. Under the physical constrains, the optimal solution of the objective function can be obtained. This minimizes the total equivalent hydrogen consumption of the system per unit cycle and improves the economy and efficiency of the system.
Based on the analysis above, the system hydrogen consumption can be calculated by (6).
where, Cfc and Cbat represent the hydrogen consumption of the fuel cell and the lithium battery respectively(g/s); κ is the correction factor, which aims at limiting the fluctuation of the battery’s SOC within a certain range, as shown in (7).
where, SH and SL represent the upper and lower limits of SOC of lithium battery(%); μ is the correction factor of the SOC, which takes a value in the range of 0–1, and the value needs to be selected according to the actual working conditions. The equivalent hydrogen in the operating condition in this paper is calculated to be minimal when the value of μ is 0.6.
Cfc and Cbat are linear functions of the fuel cell output power Pfc, but it needs to consider the influence of the charging and discharging efficiency of the lithium battery when calculating Cbat. The calculation of Cfc and Cbat is shown in Equations (8) and (9).
where, represent the average charging efficiency and discharging efficiency of the lithium battery, both of which are constants(%).
The demand power of the system load is calculated as shown in (10).
where, ηd represents the efficiency of the motor(%); Pm represents the power of the motor(W); Paux represents the auxiliary power of the hybrid system(W); Pbat represents the power of the lithium battery(W).
Combining (6), (8), (9), and (10), the minimum hydrogen consumption during the sampling cycle can be calculated by (11).
The optimal power distribution of the system can be solved by limiting the parameters with boundary conditions, and the boundary conditions of the system are shown in (12).
where, Ubatmin and Ubatmax represent the maximum and minimum value of the operating voltage of the lithium battery(V); Pfcmax represents the maximum output power of the fuel cell(W).
The optimal output power of the lithium battery can be obtained by solving for the objective function, and the optimal output power of the fuel cell is solved as shown in (13).
where, Pfcmax and Pfcmin represent the maximum and minimum output power of the fuel cell respectively(W).
According to the analysis in section 3, the variable load degradation factor is the main cause of increased fuel cell degradation. Fixed maximum power fluctuations in fuel cells can cause non-negligible power fluctuations in the event of sudden increases/decreases in demand power, which increases the degradation of the fuel cell. Therefore, this section reduces the degradation factor of the variable load by optimizing the maximum power fluctuation and thus the degradation of MFCS.
According to (4), the smaller the change of fuel cell power in adjacent moments, the lower the fluctuation of fuel cell output power. Nevertheless, the amount of fluctuation in the output power of a fuel cell varies and different power correction values are required to correct its output power to reduce its degradation. The value of power correction can be calculated by (14).
where, ∆Pmax represents the largest power fluctuation of the fuel cell(W), and it takes the maximum value when the power fluctuation is the most severe during the whole operation, denoted as εmax. With the value of εmax as the standard, the power fluctuations are represented by a variable load degradation factor at each moment, so that the value of the power correction is adaptively adjusted to adjust the output power under different operating conditions.
When solving for the adaptive power correction value, it is also necessary to consider optimizing the fuel cell power without changing the fuel cell variation trend and without exceeding the fuel cell operating interval. Specifically, the value of ∆Pfc_modify is larger when Pfc fluctuates more, and conversely, the value of ∆Pfc_modify is smaller. The direction of change in Pfc(k) is determined by the sign of the difference between Pfc(k) and Pfc(k-1), and Pfc(k) is reduced or increased as appropriate to reduce fluctuations in output power. When Pfc increases, the increase in power should be reduced significantly when fluctuations are sharp, while when fluctuations are small, the increase in power should be reduced by a small amount. Conversely, when Pfc drops, the power drop should be adjusted moderately, in a similar process to that described above.
Real-time adjustment of maximum power fluctuations can be effective in reducing the degradation factor of variable loads, but has limited effect in smoothing local power peaks in fuel cells. Therefore, a smoothing module is set up in this paper to improve this problem. The specific smoothing strategy is shown in (15).
In the driving cycle, the system operating conditions are divided into m different parts according to the fluctuation degree l of the system operating conditions, and different correction coefficients w are selected for different conditions. The following is an analysis of one of the power smoothing processes.
The amount of power change in the adjacent sampling interval is noted as ∆. Set Pfc|t-2=P, so Pfc|t-1=P+∆,Pfc|t=P +2∆. Therefore, the difference between the power before and after smoothing is (w3+w)-∆. From this equation, regardless of whether the fuel cell power increases or decreases, it is known that the adjusted power will change in its opposite direction as expected. The constraint of w is shown as (16).
When the fuel cell power fluctuates drastically, l tends to 1. Otherwise, it tends to 0. l is set according to the system power demand.
4.2. Bottom layer of the energy management strategy
The reference power Pfcref3 optimized by the top level of the energy management strategy is required to be assigned to each fuel cell stack. Given that the overall performance of MFCS depends on its worst performing stack, the output power load of the worst performing stack should be reduced. Therefore, the bottom layer aims to optimize the high-power load degradation factor to ensure the overall performance of MFCS.
For the convenience of description, this paper defines the fuel cell degradation rate ξfci, and this parameter represents the degree of the difference between current performance and initial performance of the i-th fuel cell.
where, Li is the degradation degree of the i-th fuel cell(%).
A new stack and a stack with 1600 hrs aging degree are chosen to jointly constitute the fuel cell system, setting the former as the #1 stack and the latter as the #2 stack. In order to minimize the overall degradation of the fuel cell system, it is necessary to subject the better performing stacks to larger loads and the poorer performing stacks to smaller loads, and reduce the high-power load degradation factor of the poorly performing stacks (Wang et al. Citation2020).
When the power of each stack satisfies the limit, the power distribution of different fuel cell stacks is derived in this paper, as shown in (18).
where, Pload is the required power of hydrogen fuel cell systems(W); Pfc1, Pfc2 represent the output power of #1 stack and #2 stack respectively(W); Pfci_min and Pfci_max are the minimum and maximum of the i-th fuel cell stack power respectively(W).
5. Results and discussion
5.1. Hardware-in-the-loop platform introduction
To demonstrate the viability and reliability of the suggested method, a HIL experiment platform is established in this paper. The experimental platform consists of a DSPTMS28335 controller, RT-LAB, and a host computer. First, RT-LAB processes the fuel cell hybrid system model built in Simulink in real-time. Next, the processed simulation model is assigned to different processing cores of the target machine through IP communication for real-time simulation. Besides, the DSP controller is responsible for completing the verification of the entire method, and the host computer performs the tasks of code writing, model building, control of the whole system, and analysis of the results.
This paper conducts the HIL experiment and compares the improvement of fuel cell lifetime with different energy management strategies to demonstrate the feasibility as well as reliability of the suggested method.
5.2. Analysis of results
To verify the superiority of the proposed method, FSM and ECMS are compared with the proposed method.
shows the power distribution of the MFCS when the HEM-FCD method is applied, which satisfies the power demand of the EMU.
A comparison of the standard deviation of power for ECMS and HEM-FCD is shown in . It might be derived that HEM-FCD can decrease the variable load degradation factor.
A comparison of the degradation degree of MFCS under the three different methods is shown in . It can be concluded that the overall degradation of MFCS is much less under HEM-FCD, which means a significant increase in the durability of the system and a great reduction in the economic cost of the system.
From the frequency domain analysis of the hybrid system, it is clear that the fuel cell should be subject to fewer high-frequency components while meeting the system power requirements. Therefore, the Haar wavelet is used to analyze the high-frequency component of the fuel cell output power in this paper, while the standard deviation of the high-frequency component is to characterize the fuel cell pressure. The result is shown in . With the HEM-FCD method, the pressure on both the #1 stack and the #2 stack is much less than the pressure with the other methods. This indicates an improvement in the overall operating pressure of the MFCS.
Figure 8. Fuel cell operating pressure. (a) 1# fuel cell operating pressure based on FSM, (b) 1 # fuel cell operating pressure based on ECMS, (c) 1# fuel cell operating pressure based on HEM-FCD, (d) 2# fuel cell operating pressure based on HEM-FCD.

compares the equivalent hydrogen consumption of the MFCS under three different energy management strategies. It can be concluded that the HEM-FCD-based equivalent hydrogen consumption is the smallest, 11.40% lower than the FSM-based strategy. shows the SOC variation curve of the lithium battery under the three energy management strategies. The result shows that the HEM-FCD has the smallest variation of 0.59% in the lithium battery SOC at the beginning and end.
In summary, a comparison of performance indicators of three energy management strategies is shown in .
Table 2. Fuel cell parameters.
6. Conclusion
In order to prolong the life of fuel cells, this paper proposes a novel hierarchical energy management strategy for the multi-stack fuel cell hybrid system for EMUs considering hydrogen fuel cell degradation. Under the hierarchical architecture, the method adaptively adjusts the maximum power fluctuation of the fuel cell and distributes the power of the different stacks according to the fuel cell degradation degree. To verify the effect of the proposed method on improving the durability of fuel cells, this paper conducts experimental on the HIL platform. The experimental results show that HEM-FCD can effectively reduce the degradation and operational stress of fuel cell systems compared to conventional energy management strategies. It is shown that the proposed method is effective in improving the lifetime of the fuel cell and the economic cost of the system.
Author contributions
Conceptualization, D.J. and Y.L.; methodology, D.J.; software, D.J.; Writing – original draft, P.F. and C.G.; Project administration and funding acquisition, Y.T.; validation, D.J., Y.L., P.F., and H.H. Writing – review and editing, D.J. and Y.T. All authors have read and agreed to the published version of the manuscript.
Acknowledgements
This paper is supported by The State Key Laboratory of Heavy duty and Express High-power Electric Locomotive.
Disclosure statement
No potential conflict of interest was reported by the author(s).
Additional information
Funding
References
- Anselma, P., A. Biswas, G. Belingardi, and A. Emadi. 2020. Rapid assessment of the fuel economy capability of parallel and series-parallel hybrid electric vehicles. Applied Energy 275:115319. doi:10.1016/j.apenergy.2020.115319.
- Chen, R., L. Zhou, Y. Yue, J. Tang, and C. Lu. 2018. The integrated optimization of robust train timetabling and electric multiple unit circulation and maintenance scheduling problem. Advances in Mechanical Engineering 10 (3):168781401876869. doi:10.1177/1687814018768694.
- Diana, S., S. Javier, and B. Loïc. 2020. Energy management strategy to optimise regenerative braking in a hybrid dual-mode locomotive. IET Electrical Systems in Transportation 10 (4):391–400. doi:10.1049/iet-est.2020.0070.
- Duan, B., Q. Wang, J. Wang, J. Li, and T. Ba. 2017. Calibration efficiency improvement of rule-based energy management system for a plug-in hybrid electric vehicle. International Journal of Automotive Technology 18:335–44. doi:10.1007/s12239-017-0034-4.
- Gazdzick, P., J. Mitzel, D. Sanchez, M. Schulze, and K. Friedrich. 2016. Evaluation of reversible and irreversible degradation rates of polymer electrolyte membrane fuel cells tested in automotive conditions. Journal of Power Sources 327:86–95. doi:10.1016/j.jpowsour.2016.07.049.
- Huangfu, Y., S. Zhuo, F. Chen, S. Pang, D. Zhao, and F. Gao. 2018. Robust voltage control of floating interleaved boost converter for fuel cell systems. IEEE Transactions on Industry Application 54:665–74. doi:10.1109/TIA.2017.2752686.
- Kandidayeni, M., A. Macias, L. Boulon, and S. Kelouwani. 2020. Investigating the impact of ageing and thermal management of a fuel cell system on energy management strategies. Applied Energy 274. doi:10.1016/j.apenergy.2020.115293.
- Legala, A., J. Zhao, and X. Li. 2022. Machine learning modeling for proton exchange membrane fuel cell performance. Energy and AI 10:2666–5468. doi:10.1016/j.egyai.2022.100183.
- Li, Q., L. Cai, L. Yin, T. Wang, L. Li, S. Xie, and W. Chen. 2023. An energy management strategy considering the economy and lifetime of multi-stack fuel cell hybrid system. IEEE Transactions on Transportation Electrification 9 (2):3498–507. doi:10.1109/TTE.2022.3218505.
- Li, Q., P. Liu, X. Meng, G. Zhang, Y. Ai, and W. Chen. 2022. Model prediction control-based energy management combining self-trending prediction and subset-searching algorithm for hydrogen electric Multiple Unit train. IEEE Transactions on Transportation Electrification 8 (2):2249–60. doi:10.1109/TTE.2022.3149479.
- Li, Q., B. Su, Y. Pu, Y. Han, T. Wang, L. Yin, and W. Cheng. 2019. A state machine control based on equivalent consumption minimization for fuel cell/supercapacitor hybrid tramway. IEEE Transactions on Transportation Electrification 5 (2):552–64. doi:10.1109/TTE.2019.2915689.
- Liu, T., Y. Zou, D. Liu, and F. Sun. 2015. Reinforcement learning of adaptive energy management with transition probability for a hybrid electric tracked vehicle. IEEE Transactions on Industrial Electronics 62 (12):7837–46. doi:10.1109/TIE.2015.2475419.
- Li, Q., L. Yin, H. Yang, T. Wang, Y. Qiu, and W. Chen. 2021. Multiobjective optimization and data-driven constraint adaptive predictive control for efficient and stable operation of PEMFC system. IEEE Transactions on Industrial Electronics 68 (12):12418–29. doi:10.1109/TIE.2020.3040662.
- Marx, N., L. Boulon, F. Gustin, D. Hissel, and K. Agbossuo. 2014. A review of multi-stack and modular fuel cell systems: Interests, application areas and on-going research activities. International Journal of Hydrogen Energy 39 (23):12101–11. doi:10.1016/j.ijhydene.2014.05.187.
- Peng, W., and R. Bucknall. 2020. Hybrid fuel cell and battery propulsion system modelling and multi-objective optimisation for a coastal ferry. International Journal of Hydrogen Energy 45 (4):3193–208. doi:10.1016/j.ijhydene.2019.11.152.
- Peng, H., H. Cao, S. Dirkes, Z. Chen, K. Deng, J. Gottschalk, C. Ünlübayir, A. Thul, L. Löwenstein, D. Sauer and, et al. 2021. Validation of robustness and fuel efficiency of a universal model-based energy management strategy for fuel cell hybrid trains: From analytical derivation via simulation to measurement on test bench. Energy Conversion and Management 229:113734. doi:10.1016/j.enconman.2020.113734.
- Qi, X., Y. Luo, and G. Wu. 2017. Deep reinforcement learning-based vehicle energy efficiency autonomous learning system. IEEE Intelligent Vehicles Symposium (IV) 1228–33. doi:10.1109/IVS.2017.7995880.
- Wang, Y., S. Advani, and A. Prasad. 2020. A comparison of rule-based and model predictive controller-based power management strategies for fuel cell/battery hybrid vehicles considering degradation. International Journal of Hydrogen Energy 45 (58):33948–56. doi:10.1016/j.ijhydene.2020.09.030.
- Wang, J., H. Jiang, G. Chen, H. Wang, L. Lu, J. Liu, and L. Xing. 2023. Integration of multi-physics and machine learning-based surrogate modelling approaches for multi-objective optimization of deformed GDL of PEM fuel cells. Energy and AI 14:100261. doi:10.1016/j.egyai.2023.100261.
- Wang, T., Q. Li, X. Wang, W. Chen, E. Breaz, and F. Gao. 2020. A power allocation method for multistack PEMFC system considering fuel cell performance consistency. IEEE Transactions on Industry Applications 56 (5):5340–51. doi:10.1109/TIA.2020.3001254.
- Yang, Y., X. Hu, H. Pei, and Z. Peng. 2016. Comparison of power-split and parallel hybrid powertrain architectures with a single electric machine: Dynamic programming approach. Applied Energy 168:683–90. doi:10.1016/j.apenergy.2016.02.023.
- Zhang, R., and J. Tao. 2018. GA-Based fuzzy energy management system for FC/SC-Powered HEV considering H2 consumption and load variation. IEEE Transactions on Fuzzy Systems 26 (4):1833–43. doi:10.1109/TFUZZ.2017.2779424.
- Zhang, X., R. Yang, T. Zhang, S. Xu, Y. Shen, and H. Ni. 2014. The characteristics of voltage degradation of a proton exchange membrane fuel cell under a road operating environment. International Journal of Hydrogen Energy 39 (17):9420–29. doi:10.1016/j.ijhydene.2014.03.239.
- Zheng, C., G. Xu, and Y. Park. 2014. Prolonging fuel cell stack lifetime based on pontryagin’s minimum principle in fuel cell hybrid vehicles and its economic influence evaluation. Power Sources 248:533–44. doi:10.1016/j.jpowsour.2013.09.110.
- Zhou, D., A. Al-Durra, I. Matraji, A. Ravey, and F. Gao. 2018. Online energy management strategy of fuel cell hybrid electric vehicles: A fractional-order extremum seeking method. IEEE Transactions on Industrial Electronics 65 (8):6787–99. doi:10.1109/TIE.2018.2803723.
- Zhou, D., F. Gao, E. Breaz, A. Ravey, and A. Miraoui. 2017. Degradation prediction of PEM fuel cell using a moving window based hybrid prognostic approach. Energy 138:1175–86. doi:10.1016/j.energy.2017.07.096.
- Zhou, Y., A. Ravey, and M. Pera. 2020. Multi-mode predictive energy management for fuel cell hybrid electric vehicles using Markov driving pattern recognizer. Applied Energy 258:114057. doi:10.1016/j.apenergy.2019.114057.