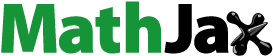
ABSTRACT
To solve the problem of the grade evaluation of foreign fiber content in cotton, this paper divided the problem into multi-criteria decision-making field and proposed an interval-valued Pythagorean fuzzy set foreign fiber content grade evaluation method. Taking into account the various properties of foreign fibers, the multi-criteria evaluation of single foreign fiber content and comprehensive foreign fiber content is carried out to improve the quality of cotton textiles. Based on the Pythagorean fuzzy set, we used AHP and TOPSIS methods to determine the relevant weight information, combined with the grade evaluation index of foreign fiber content and the interval-valued Pythagorean fuzzy weighted average or geometric operators to construct a fiber multi-criteria decision model. Then, the evaluation index function is improved to make the score function more consistent with the facts, and the digitization and intelligence methods required for foreign fiber content detection and grade evaluation are developed. Further, an example is given to verify the feasibility and effectiveness of the model. In addition, the multi-criteria decision-making method adopted has high theoretical innovation and application value for the textile industry.
摘要
针对棉花中异纤含量等级评价问题,将问题分解为多准则决策域,提出了一种区间值勾股模糊集异纤含量等级评价方法. 考虑到异纤的各种性能,进行单一异纤含量和综合异纤含量的多指标评价,以提高棉纺织品的品质. 以勾股模糊集为基础,采用层次分析法和TOPSIS方法确定相关权重信息,结合异纤含量等级评价指标和区间值勾股模糊加权平均或几何算子构建纤维多准则决策 模型. 然后,改进评价指标函数,使评分函数更符合事实,开发了异纤含量检测和等级评价所需的数字化、智能化方法. 进一步,给出了一个例子来验证模型的可行性和有效性. 此外,所采用的多准则决策方法对纺织行业具有较高的理论创新和应用价值.
Introduction
Multi-criteria decision-making (MCDM) determines the optimal selection by considering multiple attributes of the evaluation target, which is the most dominant and feasible procedure from the decision-making strategy to deal with intricate data in authentic life scenarios (Ye and Chen Citation2021; Yue and Zhang Citation2020). To express uncertainty and fuzzy information, Zadeh first proposed the related concepts of fuzzy set (FS) in 1965, and then extended the scope of fuzzy sets by introducing interval fuzzy (Zadeh Citation1973). Next, a series of fuzzy set expansion operators have been proposed, such as intuitionistic fuzzy sets (IFSs) (Yang, Wang, and Xu Citation2019), interval-valued intuitionistic fuzzy sets (IVIFSs) (Yuan, Yi, and Zhang Citation2021), Pythagorean fuzzy sets (PFSs) (Khan et al. Citation2019; Ye and Chen Citation2021), interval-valued Pythagorean fuzzy sets (IVPFSs) (Peng, Liu, and Sun Citation2021), q-rung orthopair fuzzy sets (q-ROFSs) (Yager Citation2016), interval-valued q-rung orthopair fuzzy sets (IVq-ROFSs) (Garg Citation2021; Wang et al. Citation2019), and so on. It can be seen that q-ROFS is more widely applicable than IFS and PFS because IFS and PFS are special cases of q-ROFS for q = 1 and q = 2, respectively. However, Pythagorean fuzzy information has a wide application space and is widely used in multi-criteria decision problems because it has a wider constraint range than IFS and lower complexity than the q-ROFS. Furthermore, Jan (Citation2019) used a numerical method to demonstrate the significance of the interval-valued fuzzy concept relative to the non-interval-valued fuzzy. Peng and Yang (Citation2016) and Li, Wei, and Gao (Citation2018) proposed the interval-valued Pythagorean fuzzy weighted aggregation operators for MCDM problems. In addition, linear Diophantine fuzzy and spherical linear Diophantine sets are also important components of fuzzy sets. Kamacı (Citation2021) and Riaz et al. (Citation2021) studied the theory and properties of relevant linear Diophantine and spherical linear Diophantine fuzzy aggregation operators, respectively.
The analytic hierarchy process (AHP) is a simple, flexible, and practical MCDM method for quantitative analysis of qualitative problems. Huang (Citation2021) developed a new AHP method to solve the correlation effect between criteria. Ayyildiz and Gumus (Citation2021) and Çalık (Citation2021) applied the AHP method in IVPFS to determine the criteria weights. The technique for order preference by similarity to an ideal solution (TOPSIS) method can realize the comprehensive evaluation of multiple input arguments. Alshammari et al. (Citation2022) constructed the LDFSS-TOPSIS technique as a robust extension of TOPSIS for multi-criteria decision-making problems. Moreover, Tirkolaee et al. (Citation2021) and Riaz et al. (Citation2022) presented a new MCDM technique based on AHP and TOPSIS methods.
Foreign fiber refers to impurities in cotton, which will cause yarn breakage and defects during the spinning and weaving process, reduce the quality of yarn and textiles, and affect the efficiency of enterprises (Ren et al. Citation2022). In the Chinese national standard, the foreign fiber content is an important indicator of cotton quality. Different levels of foreign fiber content cause different degrees of damage to yarns and fabrics and affect the appearance and quality of cotton products. Therefore, it is necessary to grade the content of foreign fiber in cotton. In this paper, the problem of grade evaluation of the multi-type and multi-criteria foreign fiber content is divided into MCDM fields, and an evaluation model of foreign fiber content is established by using IVPFSs information. At present, most detection methods are based on machine vision technology. By using a CCD camera to scan cotton, the collected images were processed, and the foreign fibers in cotton were statistically analyzed, to realize the content-level evaluation. Taylor (Citation1990) used imaging technology to determine the quantity and particle size of foreign matter in cotton and designed an analyzer that could determine the cotton impurity content. Fortier et al. (Citation2011) noted that HVI and other conventional instrument-based methods cannot measure fibers. Further, Du et al. (Citation2017) developed a fiber color model with characteristic parameters alongside a classification and recognition algorithm based on cluster analysis. Zhang, Li, and Zhang (Citation2021) realized cotton impurity content detection based on an improved Yolo v4. Moreover, Das and Ghosh (Citation2021) presented a fuzzy MCDM approach for grading raw jute.
The purpose of this study is to improve the construction of IVPFS-based decision methods and related application extensions for information fusion and data aggregation analysis. Through the optimization of the score function and the qualitative and quantitative description of the weight determination, the MCDM method based on IVPF information is constructed. The proposed method is then applied to the content evaluation of various types and attributes of fibers, and the quality grade of different batches of cotton is determined, which is very important to reduce the cost of cotton textile enterprises and improve the quality of textiles.
The objective of the paper is given as follows:
The superiority of interval-valued Pythagorean fuzzy sets is analyzed, the weighted average and geometric operators are derived, and the desirable properties are studied.
AHP and TOPSIS methods are introduced into IVPFS to determine weight information, which provides a solution to the unknown weight problems in the MCDM.
The score function is improved to make it more consistent with the facts, and then the MCDM method based on interval-valued Pythagorean fuzzy sets is proposed.
Characteristics of different foreign fiber content parameters and grade evaluation interval characteristics are studied, and the MCDM method is used to evaluate foreign fiber content, and then a hybrid decision method is designed.
Foreign fiber content grade evaluation system
In this section, the evaluation algorithm of foreign fiber content is studied by combining interval-valued fuzzy and Pythagorean fuzzy MCDM methods. Firstly, the theoretical derivation and desirable properties of the MCDM method are discussed to deepen the understanding of the related algorithms. The method is then applied to the evaluation of foreign fiber content in cotton, and a content evaluation model is constructed. Finally, the effectiveness of the proposed algorithm is verified by numerical analysis and method comparison.
The evaluation system includes establishing and verifying evaluation models of single and comprehensive foreign fiber content. The characteristic parameters of various foreign fibers in cotton were obtained by image detection technology, and then the content decision matrix was constructed. The AHP method was used to determine the attribute weight vector of each fiber, and the aggregation operators were run to calculate the score and accuracy values. Further, the grade evaluation of single fiber content is realized according to the evaluation criteria. Among them, the single foreign fiber evaluation indexes are score and accuracy function values based on the PFS-weighted operator. However, the evaluation model of comprehensive foreign fiber content is extended based on a single model, and combined with the TOPSIS method to determine the weight vector of various fibers to achieve the evaluation of the comprehensive fiber content. Then, the influencing factors of the comprehensive fiber evaluation include weighted fiber grade membership and comprehensive score function values.
Pythagorean fuzzy sets
The Pythagorean fuzzy set is widely used in MCDM problems because of its low complexity and wide space range. It requires the sum of squares of the membership degree and non-membership degree to be less than or equal to 1, which has a wider space than intuitionistic fuzzy sets. In this paper, interval-valued Pythagorean fuzzy information is used to determine foreign fiber content grade evaluations. The introduction of interval values further expands the fuzzy set application range (Gul and Ak Citation2021; Liang et al. Citation2018).
Interval-valued Pythagorean fuzzy sets
Studying the literature on Pythagorean fuzzy sets allows one to understand relevant basic concepts. Let X be the domain; then, an interval-valued Pythagorean fuzzy set can be denoted as follows: , where
and
denote the degrees of membership and non-membership of the element x∈X in the set I, respectively. For convenience, an IVPFN is denoted by
.
Let ,
, and
be three IVPFNs, and then we can define basic operations (Li, Wei, and Gao Citation2018): ①
②
④
⑤
For IVPFN, the related arithmetic is as follows (Li, Wei, and Gao Citation2018):
① ②
④
Based on the above basic concepts, we propose improved IVPFS score and accuracy functions, as well as an evaluation criteria (Li, Wei, and Gao Citation2018). The improved score function expresses the relative membership degree of IVPFS, and it can be denoted as follows:
Let be the IVPFN. The score and accuracy functions are defined as follows:
From the score and accuracy functions defined above, let and
be two IVPFNs. The evaluation criteria are as follows (Li, Wei, and Gao Citation2018):
The first evaluation criterion: If , then
; If
, then
;
The second evaluation criterion: If ; If
, then
; If
, then
; if
, then
.
Interval-valued Pythagorean fuzzy weighted average operator
Let be any IVPFN, where
is its weight vector and
. IVPFWA can be denoted as follows (Peng and Yang Citation2016):
Proof: By mathematical induction:
(1) When n is equal to 1, the formula holds.
(2)
(3) We suppose to hold for n=k, that is
Then, if n=k +1, we have
According to the definition of the IVPFWA operator, its desirable properties are discussed.
Property (1): Idempotency
Let ,
is the weight vector of
,
,
, and
, then
.
Proof:
Property (2): Monotony
Let ,
be two IVPFNs,
is the weight vector of
,
,
, and
. If
,
, then
Proof:
Let ,
,
, then
,
,
Thus, we can also prove the relationship between other terms, so that this desirable property holds.
Property (3): Boundedness
Let be an IVPFNs,
,
,
is the weight vector of
,
,
, and
, according to Properties 1 and 2, we can obtain
.
Property (4): Commutativity
Let be a collection of IVPFNs, and
be any permutation of
, then
Proof:
Since set ai’ is any permutation of set ai, it indicates that any element in ai’ can be found in ai, we can obtain:
In addition, the interval-valued Pythagorean fuzzy average (IVPFA) operator is induced by the IVPFWA operator when , thus,
Interval-valued Pythagorean fuzzy weighted geometric operator
Let be any IVPFN,
is the weight vector, and
. IVPFWG can be denoted as follows (Peng and Yang Citation2016):
The proof process and related ideal properties of the IVPFWG operator are similar to the IVPFWA operator, which is not proved here. In addition, the interval-valued Pythagorean fuzzy geometric (IVPFG) operator is induced by the IVPFWG operator, when, then
Evaluation of the single foreign fiber content grade
Single foreign fiber content grade evaluation method
In this section, we considered the MCDM method with interval-valued Pythagorean fuzzy information. We used IVPFWA and IVPFWG operators to evaluate the grade of the single foreign fiber content, and determine the weight vector of the attribute set by the AHP method. Let the set of options be and the set of attributes be
,
is the weight vector of Cn,
, and
. The decision matrix
is obtained through the performance probability distribution. The probabilities A(ɑij) of the foreign fiber attributes are obtained using formula (7). In the PFS environment, the paper combined the concept of interval error to deal with the probability A(ɑij) and obtained the decision matrix
. The aggregated results are then compared by evaluation criteria to obtain the optimal alternative set. Finally, the evaluation grade of single foreign fiber content was obtained.
where ,
represents the number of occurrences of a single attribute under each decision, and
represents the number of samples.
Analytic hierarchy process
The AHP is a combination of qualitative and quantitative decision-making methods. The steps are as follows: arrange m evaluation factors into an m × m order matrix and then determine the matrix values according to the importance of the factors. The maximum eigenvalue and corresponding eigenvector of the matrix are calculated and the normalized maximum eigenvector that satisfies the consistency test is the weight vector. The scale and meaning of the judgment matrix are shown in .
Table 1. The scale and meaning of the judgment matrix.
We used the judgment matrix to solve for the corresponding eigenvalue and eigenvector. The consistency test formula is as follows:
where Ci is the random consistency index of the judgment matrix,
represents the number of attribute sets, and Ri represents the average random consistency index of the judgment matrix, as shown in . Moreover, the judgment matrix is modified according to the degree of consistency of the judgment matrix
Table 2. Average random consistency index.
Application of the single foreign fiber content grade evaluation method
Decision matrix
The single foreign fiber content grade evaluation is performed using hair as an example under the IVPFS environment. We calculated the probability of hair attributes according to the fiber probability formula (7) to obtain the value of the membership degree. The principle of maximum membership degree and maximum non-membership degree was used to obtain the value of the non-membership degree. Considering the existence of errors, the error of 0.05 was used for scaling to obtain the interval-valued Pythagorean fuzzy decision matrix , as shown in . Where C1, C2, C3, and C4 represent the diameter, length, area, and weight of the hair, and A1, A2, A3, and A4 represent the grades first, second, third, and fourth, respectively.
Table 3. Interval values for the Pythagorean fuzzy decision matrix for hair .
Results of the analytic hierarchy process
The four attributes of hair are placed in a 4 × 4 matrix, and the importance of any two factors is compared to obtain a judgment matrix, as shown in . Where C1, C2, C3, and C4 represent the diameter, length, area, and weight of the hair, respectively.
Table 4. The judgment matrix of the hair.
The consistency index of the hair and the random consistency ratio of the hair judgment matrix
. The attribute weight vector can be obtained by normalizing the largest eigenvector
,
.
Application of the interval-valued Pythagorean fuzzy aggregation operators
The AHP method is used to determine the attribute weight vector of hair. The decision matrix of hair is operated to obtain the integrated matrix
using IVPFWA and IVPFWG operators, as shown in . Where A1, A2, A3, and A4 represent the grades first, second, third, and fourth, respectively. It can be seen that under the two aggregation methods, the membership interval of aggregation vector A2 is significantly better than that of A1, A3, and A4, and the size of A3 and A4 is similar, indicating that there is little difference between them.
Table 5. Hair integration matrix under the IVPFWA and IVPFWG operators .
The score and accuracy functions are run on the integrated matrix to obtain the function value images, as shown in and 2. It can be seen from that the value of the score function does not appear to be equal, and the first evaluation criterion is used to divide the single fiber content evaluation grade. The result is
and thus the grade evaluation result under the IVPFWA operator is II. Then, we can see from the figure that the larger the score function value, the smaller the accuracy function value, which is mainly affected by the membership degree and non-membership degree. Moreover, we can also conclude from that they are consistent with . However, by comparing and analyzing , it can be found that the value of the evaluation function under the IVPFWA aggregation model is slightly larger than that under the IVPFWG aggregation model.
The influence of attribute set on the evaluation result
According to the variable control theory, the two attributes of control remain constant, and the influence of the other two attributes (diameter or length) on the score function and the accuracy function is studied. The relationship diagram is shown in . From figure (a), it can be seen that the value of the score function increases with the increase in diameter or length, that is, the IVPFN determines the value of the score function. It can be seen from Figure (b) that the relationship between the accuracy function and attributes is complex. When using the second criterion to evaluate the grade of foreign fiber, it is necessary to precisely calculate the accuracy function value of each measuring point.
Comprehensive foreign fiber content grade evaluation
Comprehensive foreign fiber grade evaluation method
The comprehensive evaluation method of foreign fiber content is an extension of the single evaluation method, and the comprehensive content evaluation model is proposed through linear analysis and comparison. Then, foreign fibers are constructed into the decision-making set, and the TOPSIS method is used to determine the weight information of various foreign fibers. Next, this is combined with the comprehensive score function shown in formula (9) to determine the comprehensive score function value. Finally, we obtain the optimal comprehensive evaluation grade of foreign fibers by using the evaluation criteria.
where represent the comprehensive score value,
represents the score value of each decision, is the weight vector of
, and
.
Decision matrix of comprehensive foreign fibers
Common foreign fibers are hair, wool, feathers, hemp rope, polypropylene yarn, nylon rope, plastic film, silk, camel hair, and yak hair, and the corresponding weight information is . Similar to the method used to determine the weight vector of hair attributes, the AHP method is used to determine the weight information of other foreign fiber attributes, as shown in .
Table 6. Attribute weight information for various foreign fibers.
Similar to the process of determining the decision matrix of a single foreign fiber hair, we used the attribute probability formula of foreign fiber to solve the attribute probability of various foreign fibers. The obtained attribute membership degree was mapped to the IVPFS information, and then the decision matrix of various foreign fibers was obtained. The decision matrix is shown in .
Table 7. Interval values Pythagorean fuzzy decision matrix of fibers.
TOPSIS method
TOPSIS method was adopted to determine the weight vector of fibers. It has the following advantages: no sample data is required, it is more in line with the actual evaluation situation, and alternatives can be ranked in order of merit. According to the decision matrix , the positive ideal decision matrix
, the negative ideal matrices
,
, and
, and the normalized projections are calculated; then, the closeness degree RCk of different decision objects can be obtained according to the projection of the decision matrices on the ideal matrices. Furthermore, the weight vectors of different objects are obtained by standardizing the closeness degrees. The closeness degree RCk and the weight vector wk are shown in Formula (10) and (11), respectively.
We used the TOPSIS method to determine the weight information for fibers based on the decision matrix. Through the comprehensive foreign fiber decision matrix in , the weight coefficient wj of various fibers is obtained, .
Comprehensive grade evaluation model of foreign fiber
The various above-mentioned foreign fiber decision matrices are run with IVPFWA and IVPFWG operators to obtain the fiber integration matrices
, respectively. The results are shown in , respectively. It can be seen that the membership degree of the aggregation vector of various foreign fibers under the index A2 is significantly greater than A3 and A4, but there is no absolute sequential relationship between A1 and A2, indicating that A2 is quite different from A3 and A4, while A1 and A2 are less distinguishable.
Table 8. IVPFWA foreign fiber integration matrix .
Table 9. IVPFWG foreign fiber integration matrix .
We used the integrated decision matrix in to determine the score function value and obtained the evaluation grade of each fiber according to the evaluation criteria. Then, we find that the decision results of for A3 and A4 of fiber 9 (camel hair) are equal under the IVPFWA and IVPFWG operators, which is mainly caused by the decision data. Thus, if the decision matrix
under the IVPFWA operator runs at the same level of fibers, the result after processing by the IVPFWG operator usually remains consistent.
Considering the closeness degree of the decision matrix of different foreign fibers, the TOPSIS method is used to determine the weight information of foreign fibers. The content grade of each type of fiber is determined by a single foreign fiber content evaluation model, the fibers grade matrix , and each Ai in the matrix represents the evaluation grade of the corresponding fiber. Then, combined with the evaluation model of comprehensive foreign fiber content, the comprehensive evaluation result is obtained, as shown in . It can be seen that the comprehensive score function achieves the optimal solution at II, that is, the comprehensive fibers content level is II. Then, the decision result of the IVPFWA operator is larger than that of the IVPFWG operator on the whole, which is also consistent with the decision result of the evaluation model of the single foreign fiber content.
Comparison with existing methods
Some existing decision-making methods were used to solve the above MCDM problems. The effectiveness and superiority of the proposed method are then illustrated by numerical analysis. Li, Wei, and Gao (Citation2018) proposed IVPFWHM and IVPFWDHM aggregation operators and analyzed the sensitivity of the decision model. We applied the two decision aggregation operators to the decision problem in this paper to analyze the influence of different parameters on the decision results, as shown in . It can be seen that the decision results of different parameters in are consistent with the results of the proposed decision method, indicating the effectiveness of the proposed method. However, the difference between the comprehensive score function values under IVPFWHM and IVPFWDHM operators is small, which is not conducive to the subsequent optimal decision, and the selection of the k value will also increase the workload.
Table 10. Score function values under various operators.
Moreover, Liang et al. (Citation2018) introduced Bonferroni mean (BM) operator into IVPFS and proposed the WIVPFGBM operator, and then we applied this operator to this paper. When p = 1 and q = 1, the aggregation results have S(A1) = 0.9579, S(A2) = 0.9628, S(A3) = 0.9484, S(A4) = 0.9271, and the ranking result is A2 > A1 > A3 > A4. Furthermore, Wang et al. (Citation2019) introduced q-RIVOFWHM and q-RIVOFWDHM operators, and then we applied these operators to this paper. When q = 3, k = 1 the q-RIVOFWHM aggregation results have S(A1) = 0.9557, S(A2) = 0.9639, S(A3) = 0.9444, S(A4) = 0.9091, when q = 3, k = 1 the q-RIVOFWDHM aggregation results have S(A1) = 0.0074, S(A2) = 0.0099, S(A3) = 0.0048, S(A4) = 0.0023. To facilitate the comparison of multiple decision-making methods, we put the aggregated results in the same image for analysis and comparison, as shown in .
First of all, through the comparison of various decision-making methods, we can see that the best decision-making scheme is consistent, that is, the optimal evaluation of the comprehensive foreign fiber content is II. Then, we find that the evaluation results of the proposed two decision methods are distinguishable in each scheme, and other methods cannot achieve this effect. Furthermore, the proposed IVPFA and IVPFG operators have low complexity, which is conducive to the calculation of aggregate results, while other decision methods need to consider the influence of parameters and have high computational complexity. Therefore, the proposed decision-making method has certain advantages for MCDM problems.
Conclusion
Since Pythagorean fuzzy information has a wider application range than intuitionistic fuzzy set and a lower complexity than q-rung orthopair fuzzy set, it is widely used in multi-criteria decision-making problems, and the application scope of PFS is further expanded by introducing interval-valued. In this paper, a decision method based on IVPF information is proposed for MCDM problems with unknown weights.
In this paper, the advantages of Pythagorean fuzzy sets are studied, and a decision model based on IVPFWA and IVPFWG with unknown weights is proposed by extending IVPFS. Then, we improve the score function and study some desirable properties. Next, by combining AHP and TOPSIS methods to determine the weight information, a decision-making method is proposed. Further, we study the characteristics of different foreign fiber content parameters and grade evaluation interval characteristics and divide the multi-type and multi-criteria foreign fiber content evaluation problem into the category of MCDM problems. Finally, the effectiveness of the proposed method is illustrated by the analysis and comparison of decision methods. Thus, it has important theoretical innovation and engineering application value and has guiding significance for related grade evaluation research.
In the future research, we will further generalize these operators using Choquet integral and power operator to solve the multi-variable multi-classification problem under hesitant fuzzy sets. Then, linear Diophantine fuzzy sets, and spherical and complex spherical fuzzy sets have been research hotspots in recent years, which have certain frontiers and representativeness. By combining them with the weighted average and geometric operators mentioned in this paper, more representative operators can be developed. Further, by studying the weight determination method of attribute set and decision set, a decision-making method for specific functions is constructed, which can be targeted to solve the decision problem in real life. We hope that this study will provide a useful perspective for researchers engaged in decision-making.
Highlights
The characteristics of different foreign fiber content parameters and grade evaluation interval characteristics were studied, and a multi-attribute decision-making method was used to evaluate foreign fiber content.
AHP and TOPSIS methods were introduced into IVPFS to determine the weight information, which provided a solution to the unknown weight problem of multi-attribute decision-making.
In this paper, the scoring function is improved to make it more consistent with the facts.
Development of a new method for multi-attribute decision-making based on interval-valued Pythagorean fuzzy set.
The two decision methods presented in this paper are applied to the grade evaluation of single or comprehensive foreign fiber content and the grade evaluation of synthetic foreign fiber content.
Acknowledgements
We would like to submit the enclosed manuscript entitled “Development and application of an interval-valued Pythagorean fuzzy set-based grade evaluation method to foreign fiber content”, which we wish to be considered for publication in “Journal of Natural Fibers”. No conflict of interest exists in the submission of this manuscript, and the manuscript is approved by all authors for publication. I would like to declare on behalf of my co-authors that the work described was original research that has not been published previously, and not under consideration for publication elsewhere, and in addition, there is no legal or ethical problem with this article.
Disclosure statement
No potential conflict of interest was reported by the authors.
Additional information
Funding
References
- Alshammari, I., M. Parimala, C. Ozel, M. Riaz, and R. Kammoun. 2022. New MCDM algorithms with linear Diophantine fuzzy soft TOPSIS, VIKOR and aggregation operators. Mathematics 10 (17):3080. doi:10.3390/math10173080.
- Ayyildiz, E., and A. T. Gumus. 2021. Interval-valued Pythagorean fuzzy AHP method-based supply chain performance evaluation by a new extension of SCOR model: SCOR 4.0. Complex & Intelligent Systems 7 (1):559–17. doi:10.1007/s40747-020-00221-9.
- Çalık, A. 2021. A novel Pythagorean fuzzy AHP and fuzzy TOPSIS methodology for green supplier selection in the Industry 4.0 era. Soft Computing 25 (3):2253–65. doi:10.1007/s00500-020-05294-9.
- Das, S., and A. Ghosh. 2021. A fuzzy multi-criteria decision-making approach for grading of raw jute. Journal of Natural Fibers 18 (5):685–93. doi:10.1080/15440478.2019.1645788.
- Du, Y. H., T. Ma, C. W. Yang, and X. M. Yang. 2017. Detection clustering analysis algorithm and system parameters study of the near-point multi-class foreign fiber. The Journal of the Textile Institute 108 (6):1022–27. doi:10.1080/00405000.2016.1212467.
- Fortier, C. A., J. E. Rodgers, M. S. Cintron, X. Cui, and J. A. Foulk. 2011. Identification of cotton and cotton trash components by Fourier transform near-infrared spectroscopy. Textile Research Journal 81 (3):230–38. doi:10.1177/0040517510383620.
- Garg, H. 2021. A new possibility degree measure for interval‐valued q‐rung orthopair fuzzy sets in decision‐making. International Journal of Intelligent Systems 36 (1):526–57. doi:10.1002/int.22308.
- Gul, M., and M. F. Ak. 2021. A modified failure modes and effects analysis using interval-valued spherical fuzzy extension of TOPSIS method: Case study in a marble manufacturing facility. Soft Computing 25 (8):6157–78. doi:10.1007/s00500-021-05605-8.
- Huang, J. J. 2021. Analytic hierarchy process with the correlation effect via WordNet. Mathematics 9 (8):872. doi:10.3390/math9080872.
- Jan, N., M. Aslam, K. Ullah, and T. Mahmood. 2019. An approach towards decision making and shortest path problems using the concepts of interval-valued Pythagorean fuzzy information. International Journal of Intelligent Systems 34 (10):2403–28. doi:10.1002/int.22154.
- Kamacı, H. 2021. Linear Diophantine fuzzy algebraic structures. Journal of Ambient Intelligence and Humanized Computing 12 (11):10353–73. doi:10.1007/s12652-020-02826-x.
- Khan, M., S. Abdullah, A. Ali, and F. Amin. 2019. Pythagorean fuzzy prioritized aggregation operators and their application to multi-attribute group decision making. Granular Computing 4 (2):249–63. doi:10.1007/s41066-018-0093-6.
- Liang, D., A. P. Darko, Z. S. Xu, and W. Quan. 2018. The linear assignment method for multicriteria group decision making based on interval-valued Pythagorean fuzzy Bonferroni mean. International Journal of Intelligent Systems 33 (11):2101–38. doi:10.1002/int.22006.
- Li, Z., G. Wei, and H. Gao. 2018. Methods for multiple attribute decision making with interval-valued Pythagorean fuzzy information. Mathematics 6 (11):228. doi:10.3390/math6110228.
- Peng, Y., X. H. Liu, and J. B. Sun. 2021. A multi-attribute group decision making method considering both the correlation coefficient and hesitancy degrees under interval-valued intuitionistic fuzzy environment. Applied Soft Computing 104:107187. doi:10.1016/j.asoc.2021.107187.
- Peng, X. D., and Y. Yang. 2016. Fundamental properties of interval-valued Pythagorean Fuzzy aggregation operators. International Journal of Intelligent Systems 31 (5):444–87. doi:10.1002/int.21790.
- Ren, W. J., Y. H. Du, X. L. Li, and R. W. Yuan. 2022. Design optimization and flow field analysis of the nozzle structure of a foreign fiber sorter. Textile Research Journal 92 (11–12):1987–98. doi:10.1177/00405175221076046.
- Riaz, M., M. R. Hashmi, D. Pamucar, and Y. M. Chu. 2021. Spherical linear Diophantine fuzzy sets with modeling uncertainties in MCDM. Computer Modeling in Engineering & Sciences 126 (3):1125–64. doi:10.32604/cmes.2021.013699.
- Riaz, M., S. Tanveer, D. Pamucar, and D. S. Qin. 2022. Topological data analysis with spherical fuzzy soft AHP-TOPSIS for environmental mitigation system. Mathematics 10 (11):1826. doi:10.3390/math10111826.
- Taylor, R. A. 1990. Estimating the size of cotton trash with video images. Textile Research Journal 60 (4):185–93. doi:10.1177/004051759006000401.
- Tirkolaee, E. B., Z. Dashtian, G. W. Weber, H. Tomaskova, M. Soltani, and N. S. Mousavi. 2021. An integrated decision-making approach for green supplier selection in an agri-food supply chain: Threshold of robustness worthiness. Mathematics 9 (11):1304. doi:10.3390/math9111304.
- Wang, J., H. Gao, G. Wei, and Y. Wei. 2019. Methods for multiple-attribute group decision making with q-rung interval-valued orthopair fuzzy information and their applications to the selection of green suppliers. Symmetry 11 (1):56. doi:10.3390/sym11010056.
- Yager, R. R. 2016. Generalized orthopair fuzzy sets. IEEE Transactions on Fuzzy Systems 25 (5):1222–30. doi:10.1109/tfuzz.2016.2604005.
- Yang, Y., X. Wang, and Z. S. Xu. 2019. The multiplicative consistency threshold of intuitionistic fuzzy preference relation. Information Sciences 477:349–68. doi:10.1016/j.ins.2018.10.044.
- Ye, J., and T. Y. Chen. 2021. Selection of cotton fabrics using Pythagorean fuzzy TOPSIS approach. Journal of Natural Fibers 19 (14):9085–100. doi:10.1080/15440478.2021.1982439.
- Yuan, R., L. Yi, and P. Zhang. 2021. Interval-valued intuitionistic fuzzy generalised Bonferroni mean operators for multi-attribute decision making. International Journal of Fuzzy Systems 23 (6):1728–54. doi:10.1007/s40815-021-01064-3.
- Yue, Q., and L. Zhang. 2020. Two-sided matching for hesitant fuzzy numbers in smart intelligent technique transfer. Mechanical Systems and Signal Processing 139:106643. doi:10.1016/j.ymssp.2020.106643.
- Zadeh, L. 1973. Outline of a new approach to the analysis of complex systems and decision processes. IEEE Transactions on Systems, Man, and Cybernetics Smc-3 (1):28–44. doi:10.1109/tsmc.1973.5408575.
- Zhang, C., T. Li, and W. Zhang. 2021. The detection of impurity content in machine-picked seed cotton based on image processing and improved YOLO V4. Agronomy 12 (1):66. doi:10.3390/agronomy12010066.