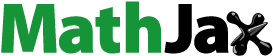
ABSTRACT
This study focuses on the optimization of geopolymer composites, considering the parameters of composition and performance. The research explores the integration of algorithm-built hybrid implementations and a hybrid intelligent system to solve complex optimization problems in geopolymer composite materials. Firstly, an algorithm-built hybrid implementation is proposed, combining experimental results with various data processing methods. This approach enables the utilization of composite algorithms, offering several advantages, such as scalability and adaptability to different loads. The models developed in this study provide a flexible and extensible architecture, allowing for efficient problem-solving in optimization tasks. Secondly, a hybrid intelligent system is introduced, comprising statistical simulation models that combine different control and design problem-solving approaches. Markov chains are employed to address the quantitative aspects of loosely structured tasks and process performance evaluation. Criterion methods are utilized for quantitative conclusions, ensuring the optimal adaptation of the results from both applications. The research culminates in the identification of the optimal composition, denoted as G + FC + CFI, with specific weight content. This composition consists of cement, activator, fireclay, and carbon fiber I, with 100 g, 90 g, 100 g, and 2.5 g, respectively. The findings from this study provide valuable insights into the optimization of geopolymer composites, employing algorithm-built hybrid implementations and a hybrid intelligent system. The proposed approaches offer enhanced efficiency and accuracy in solving complex optimization problems in the field of geopolymer composite materials. The identified optimal composition demonstrates the potential for improving performance in composition and weight content.
摘要
本研究的重点是优化地质聚合物复合材料,考虑成分和性能参数. 该研究探索了算法构建的混合实现和混合智能系统的集成,以解决地质聚合物复合材料中的复杂优化问题. 首先,将实验结果与各种数据处理方法相结合,提出了一种基于算法的混合实现方法. 这种方法能够利用复合算法,提供了几个优点,如可扩展性和对不同负载的适应性. 本研究中开发的模型提供了一个灵活和可扩展的体系结构,允许在优化任务中高效地解决问题. 其次,介绍了一种混合智能系统,该系统包括将不同的控制和设计问题解决方法相结合的统计仿真模型. 马尔可夫链用于解决松散结构任务和过程性能评估的定量方面. 标准方法用于定量结论,确保两种应用的结果的最佳适应. 研究最终确定了具有特定重量含量的最佳组成,表示为G+FC+CFI. 该组合物由水泥、活化剂、粘土和碳纤维I组成,分别为100 g、90 g、100 g和2.5 g. 这项研究的发现为利用算法构建的混合实现和混合智能系统优化地质聚合物复合材料提供了有价值的见解. 所提出的方法在解决地质聚合物复合材料领域的复杂优化问题时提供了更高的效率和准确性. 所确定的最佳组成证明了在组成和重量含量方面提高性能的潜力.
Introduction
Geopolymers are a new class of building materials that are an alternative to Portland cement. The production of Portland cement is associated with high carbon dioxide emissions. Despite this, the consumption of Portland cement has exceeded 4 million tons per year. At the same time, 1.5 million tons are emitted into the atmosphere. CO2. The production of geopolymers is free from this major drawback. Geopolymer materials are obtained by shellac processing of man-made or natural raw materials. The advantages of geopolymer technology in comparison with the technology of Portland cement production are the absence of high-temperature burning and carbon dioxide emissions during the dissociation of carbonate raw materials.
For the creation and sustainable development of the industry of geopolymer materials, it is necessary to accumulate and systematically analyze data on the influence of various factors of structure, chemical composition, and others on their various physical, mechanical, and technological properties.
The term geopolymer was proposed by French researcher J. Davidovits (Davidovits Citation1991, Citation1994), who obtained a material based on aluminosilicate material raw material as a heat-resistant alternative to traditional polymers. Due to their high strength, thermal and corrosion resistance, and low abrasion resistance, geopolymers are continuously capturing the materials market of the construction industry.
Traditionally, to optimize the composition of geopolymers, the limits of variation or ranges of variation of the obtained physical and mechanical properties of specific compositions, performed empirically, are used. The disadvantage of this approach is the fundamental impossibility of generalizing and specifying the formulation of the composition and properties of geopolymers corresponding to the maximum values of thermal and physical-mechanical properties. Another traditional approach to determine the composition of properties of geopolymers corresponding to the required values of physical and mechanical properties is the processing of experimental studies by the method of equality of weight content of criteria of physical and mechanical properties of different components of geopolymer matrices by establishing extrema. The disadvantage of this approach is the need to utilize the availability of volumes of input information with small step changes of input quantities. In a limited volume of measured quantities, the extremum point may be missed.
The random stochastic process of determining the optimal composition of geopolymer matrices based on reviewing and analyzing the available input information without its mathematical processing does not achieve the goal because it carries elements of randomness associated with the qualification of experts.
All over the world, there is an intensive search for the optimal formulation of geopolymer mixture, providing a set of specified thermal and mechanical properties.
In (Le et al. Citation2020; Van et al. Citation2019) the results of the determination of mechanical properties of foamed geopolymers at high temperatures are described. Optimization of parameters of the formulation of fly ash in geopolymers is presented in (Sharko et al. Citation2023). The creation of an intellectual system for studying the behavior of organizational and technical objects without human participation is presented in (Sharko et al. Citation2022). Modeling the development of technologies of different types is presented in (Duan, George, and Bullo Citation2019; Ludwig et al. Citation2021). The use of probabilistic models for estimating the trajectory of optimal systems development is presented in (Nosov et al. Citation2020; Odhiambo, Weke, and Ngare Citation2020; Sharko et al. Citation2022).
Criteria for calculating the durability of hybrid geopolymer bridges reinforced with carbon fiber are presented in (Hadigheh, Ke, and Fatemi Citation2022; Noushini and Castel Citation2018; Rama Seshu and Kumar Citation2019). A system for selecting sustainable criteria for fly ash based construction materials in optimizing the composition of geopolymer mixtures is presented in (Sandanayake et al. Citation2020). The study aims to reduce the amount of greenhouse gas emissions. Mechanical properties of high-strength geopolymer composites reinforced with hybrid fibers are presented in (Khan et al. Citation2018).
In (Fernández et al. Citation2023), natural fibers for 3D printed material are described that are capable of autogenous self-healing to heal possible cracks. The influence of the material structure on the mechanical properties of geopolymer composites reinforced with short fibers, obtained using additive technologies, and carbon-reinforced methods for obtaining geopolymer composites, is described in (Korniejenko, Kejzlar, and Louda Citation2022). The mechanical properties of short-fiber geopolymers made by injection molding and 3D printing are presented in (Korniejenko et al. Citation2020) showing that the mechanical properties of new composites made by injection are comparable to the mechanical properties of the traditional casting process. In (Sair et al. Citation2019), the development of biologically pure composite materials based on a geopolymer matrix and reinforced with outgoing fibers is presented. The influence of the pore structure on the strength characteristics of metakaolin-based geopolymer composites reinforced with particles and fibers is presented in (Akono, Koric, and Kriven Citation2019). It is shown that due to the presence of nanoporosity, the ratio of strength and total porosity is not unique. The optimization of the matrix compositions of Al2O3, SiO2, Caolin, and CaO for the mechanical properties of a geopolymer composite with short carbon fiber is described in (Akmal et al. Citation2019). It has been proven that the generation of geopolymer composites with the addition of silica powder and random short carbon fiber has good mechanical properties. Structure, composition, and properties of geopolymers from mineral wool waste (Erofeev et al. Citation2019). A correlation has been established between the structure, composition, and physical and mechanical properties of geopolymers obtained from mineral wool waste. The design of the mixture and the flexural impact strength of PVA fiber-reinforced volatile ash-geopolymer composites are presented in (Xu et al. Citation2017), the development of a hybrid geopolymer composite from coconut fiber for structural materials is presented in (Amalia and Akifah Citation2017), it was found that coconut fiber can improve the mechanical and microstructural properties of geopolymers composites. In (Akono et al. Citation2018), investigation delves into the behavior of cement-polymer composites across various scales, employing both computational and experimental methodologies. The primary objective of the researchers is to gain insights into how the micro- and nano-components of these composites exert influence upon their mechanical strength. Notably, the implications of their findings extend beyond the realm of cement-polymer composites, with potential applications in diverse engineered nano-composite materials. In (Wang et al. Citation2020), discourse centers on the utilization of the GTN model for the prediction of fractures in micro-forming processes. Nevertheless, it underscores limitations in the model’s effectiveness, particularly in specific operating conditions. In response, the researchers introduce an innovative model that accounts for the size-dependent effects on flow stress and incorporates a shear damage evolution law. This enhancement enhances the predictive accuracy of fracture occurrences across varying stress states and grain sizes. In (Lee, Vimonsatit, and Chindaprasirt Citation2016), a comprehensive examination is conducted on the properties of alkali-activated fly-ash cement (AAFA). This analysis is facilitated through statistical methodologies and nano-indentation testing. A noteworthy finding emerges, indicating that the quantity of sand incorporated into the cement mixture exerts the most significant influence on its density. Furthermore, the presence of certain additives is found to impart both favorable and adverse effects on the compressive strength of the AAFA cement. Additionally, this study sheds light on the principal reaction product within AAFA as sodium aluminosilicate hydrate (N-A-S-H), establishing a correlation between its presence and the cement’s strength characteristics.
The principal outcomes of this investigation have revealed that the incorporation of basalt and carbon fibers exerts a positive influence on both the mechanical strength and thermal conductivity of geopolymer composite materials. Furthermore, this study has laid the groundwork for a systematic approach to determining the ideal composition of geopolymers, with various optimization priorities considered, enabling the derivation of comparative assessments and the provision of pragmatic guidance. These findings hold significant implications for the construction industry, wherein geopolymer composites can be leveraged as viable substitutes for traditional building materials. Such composites exhibit remarkable attributes, including superior strength, thermal resilience, and corrosion resistance, although they may exhibit relatively lower abrasion resistance. Additionally, the optimization methodology established herein can serve as a valuable tool for formulating optimal geopolymer compositions across different priority configurations, thus facilitating the generation of comparative evaluations and practical directives.
Materials and method testing of investigation
Materials
The inorganic two-component aluminosilicate binder, commercially known as Baucis LK, is based on metakaolin (referred to as MK, part A) and activated by an aqueous alkaline activator (part B). Baucis LK is manufactured by České lupkové závody, a.s., located in Nové Strašecí, Czech Republic. The mixing ratio of part A and part B follows the manufacturer’s requirements, For Part A+B, we recommended a binder typically using five parts by weight of part A and four parts of the activator B. Silica sand, referred to as SS (ST 03/08), supplied by Sklopísek Střeleč, a.s., located in Újezd pod Troskami, Czech Republic, was used as the aggregate The silica sand used in the study exhibited a high SiO2 content of 99.4% and a low Fe2O3 content of 0.04%. The grain size ranged from 0.315 mm to 0.800 mm, with an average size of 0.570 mm. The bulk density of the sand was 2.65 g/cm3. The fireclay (České Lupkové Závody, Nové Strašecí, Czech Republic) was added to the geopolymer mortar reinforced basalt and carbon fibers (GMBC). The fireclay employed in the research was a sedimentary rock primarily composed of the clay mineral kaolinite. Clay minerals are aluminosilicates with varying degrees of contamination by metal oxides and organic substances. Fireclay’s high Al2O3 content and low melting admixture content contribute to its characteristic heat resistance. When fired and subsequently crushed, these high-quality raw materials result in a highly fire-resistant material used in the production of bricks and refractory concrete. The fireclay used in the tests had a bulk density of 2.48 g/cm3 and consisted of 57.8% SiO2, 36.4% Al2O3, 2.6% Fe2O3, and 3.2% other metal oxides. Chopped basalt and carbon fibers were utilized as reinforcement materials. The inclusion of basalt fiber has been found to enhance the mechanical properties and fire resistance of building materials. Therefore, both materials were incorporated to enhance the overall properties of all the samples under investigation. The raw materials used with magnification x50: (a)cement, (b) sand, (c) fireclay, (d) basalt, (d) carbon fiber I, and (e) carbon fiber II are depicted in .
Method testing
The mechanical properties of the geopolymer composites are investigated using impact, flexural, and compressive strength tests. The impact, flexural, and compressive strength tests were conducted according to ČSN EN 1015–11 standards. The mechanical strengths of the geopolymers were evaluated using the Instron model 4202 testing machine. Flexural strengths were assessed through three-point bending tests. Specimens measuring 30 by 30 by 150 mm3 and 30 by 30 by 30 mm3 were utilized for bending and compressive strength testing, respectively. The compression and bending tests were carried out at room temperature, with a load cell of 10 kN and a crosshead speed of 2.5 mm. The average values of compressive and flexural strengths were obtained by measuring three samples from each series. An impact Testing Machine (standard EN ISO 148–1:2010) was utilized. Five samples measuring sizing 20 by 20 by 80 mm3 were subjected to these tests, which were carried out at ambient temperature. The setup of stress tests is presented in .
Figure 2. Setup of stress testing (a) Instron model 4202 testing machine, (b) setup of bending testing, (c) setup of compression testing.

Figure 3. Setup of charpy impact test (a) universal testing machine, (b) setup for specimen testing.

The physical properties such as bulk density and porosity were measured according to the ČSN EN 1936 standard. Thermal conductivity properties were determined with the instrument model HFM436 Lambda (Netzsch, a.s., Selb, Germany). The physical properties of GMBC were primarily analyzed in terms of average density and thermal conductivity. The measurement of these properties was conducted using GMBC samples measuring 150 by 150 by 30 mm3.
X-ray diffraction, scanning electron microscopy, and energy-dispersive X-ray spectroscopy are used to investigate the physical and microstructural properties of the geopolymer composites.
Sample preparation
The raw materials were mixed according to the weight ratios specified in . Additionally, a parametric study was conducted to examine the influence of filler proportion on sample performance. Initially, metakaolin powder was mechanically stirred in an aqueous alkaline solution containing KOH for 5 minutes at high speeds using the mixer. Subsequently, the aggregates of the silica sand or fireclay were introduced into the geopolymer mortar, and the mixing process continued until a homogeneous blend was achieved. Finally, various fillers (such as basalts and or carbon fibers) were added to the geopolymer mortar to create distinct geopolymers. The resulting geopolymer mortar was poured into molds and securely covered with plastic wraps. The samples were then cured for 28 days at room temperature.
Table 1. The component for the mixture of GMBC.
Results and discussion
In its implementation mapping the optimization of geopolymer compositions taking into account fillers in the form of fibers, the main phases are problem identification, its semantic description, selection of the subject area, the establishment of a hierarchy of relationships between optimization parameters and consideration of their dynamic changes and possible trends. Conceptual model, i.e. transformation of declarative values and procedural knowledge for computer processing and obtaining the conclusion. It is shown in .
The presented scheme is a tool for making collective decisions, combining a quantitative and qualitative description of unstructured and semi-structured tasks.
The problem of choosing the optimal solution in the presence of several optimality criteria is associated with the development of methods, mathematical models, and algorithms that ensure the transition from subjective to objective optimal solutions (Buketov et al. Citation2017). The optimization criteria are not extreme, but some compromise values, which together correspond to the main goal of optimization. Decision-making under conditions of uncertainty and risk begins with the construction of a matrix of relative deviations of the analyzed parameters from the target:
where – geopolymer composition,
– analyzed physical and mechanical parameters,
– relative deviation of the j-th parameter from the target.
To define elements matrix (1) the vector argument convolution function is applied
to scalar
, which is formed about the extreme values of features
where under deviation from the ideal goal by the j-th feature, we understand the absolute value of the difference
, in which the ideal goal when maximizing the jth feature is defined as
, and when minimizing the jth feature, as
. The condition for the correct use of a function (2) is the use of a common absolute scale for measuring all features.
Under the sample we mean a class of objects, characterized by a generalized goal . Let us introduce a measure of the generalized deviation from the target, which allows not only to find the object closest to the sample, but also to order the objects according to their distance from the target. Consider a sample whose properties are formed by equality constraints (
). Deviation of the j-th feature in any direction from the point
determines the degree of remoteness of the object from the target by this attribute. Then the relative deviation of the jth feature from the target is defined as
where i – is the line number; j – is the number of the column in the matrix (1).
As parameters it is necessary to choose the best values of the analyzed parameters from the point of view of the problem being solved. Based on expert assessments, the optimal values of the parameters were established (maximum or minimum values from ):
Table 2. Data mechanical and physical properties of GMBC.
Density, Thermal conductivity – minimal;
Bending strength, Compressive strength, Impact strength – maximum.
With this approach, formula (3) will convert the dimensional values into relative ones within the scale (0.1). However, this choice of parameters Cj will necessarily be observed coinciding with the value corresponding matrix elements (1), which will lead to
. When using additive convolution (4), this leads to the loss of the corresponding feature from the overall assessment of the object, and when using multiplicative convolution (5), to its zeroing. An obvious way to avoid such situations is to expand the upper (for maximum) or lower (for minimum) limit of each feature
in the same percentage. Below are the maximum (minimum) values of each of the analyzed parameters
were increased (decreased) by 1%.
In the theoretical analysis, the following generalizing multicriteria convolution functions of the vector argument were used.
Additive convolution
where – importance (weight coefficient) of the j-th feature,
.
Power multiplicative convolution
Additional multiplicative convolution
Wald criterion (minimum maximum)
Laplace criterion (minimum minimum)
Hurwitz criterion
where – the indicator of pessimism, in the calculations was taken equal to 0.5.
The best object is considered to have the minimum values of functions (4–9).
The presented aspects of the physical and mechanical properties of geopolymers and their relationships through the mechanism of structure formation were taken as the basis for constructing the matrix of transition probabilities (). The processing of this matrix by the method of calculating Markov chains makes it possible to determine the values of the weight coefficients ωj, included in formulas (4–6).
Table 3. Conditional probability matrix.
There is no functional relationship between the main components of the mechanism of formation structure in geopolymers, so it makes sense about conditional probabilities of their contribution to different stages of the process dynamics ()
Each state of the parameters characterizing the information situation of determining the physical and mechanical properties of geopolymers with a given recipe for their preparation is assigned a certain probability, which is written as a line of the state matrix. The wandering of the system over its states is described by the matrix of intensities or transitions of the system. The matrix is compiled in such a way that the sum of the probabilities in the rows of the matrix is always equal to 1. In the process of analyzing the state matrix, all possible states of the parameters are enumerated with their probabilities, i.e. we are dealing with a stochastic transition matrix, the set of vectors inside which reflects the values of probabilities between gradations. These iterations are made for various combinations of parameters. Since the processes of obtaining the mechanical properties of geopolymers by changing their formulations do not have a constant time reference, we will use the stages that characterize the successive approximation of the approach of the states to the achievement of the intended goal as time. In this case, we will replace the time with the step number.
The initial state vector in can be written in the form:
The transition probability matrix has the following form:
Multiplying the initial state vector Р(0) onto the matrix of transition probabilities Т, we obtain the probability distribution at the first stage of decision-making Р(1). By the methodology for calculating Markov chains, this probability will be equal to:
Multiplying the state vector Р(1) onto the matrix of transition probabilities Т, we will obtain the probability distribution at the next decision-making stage Р(2):
Multiplying the state vector Р(2) onto the matrix of transition probabilities Т we will obtain the probability distribution at the next decision-making stage Р(3):
We repeat similar calculations until constant stationary values of the state vector are reached:
Starting from the fifth step, the values of the state vector stop changing. Thus, we have the following set of weight coefficients for the physical and mechanical parameters of geopolymers:
Density ω = 0.0893
Bending strength ω = 0.2419
Compressive strength ω = 0.3897
Impact strength ω = 0.1541
Thermal conductivity ω = 0.1250
The calculations were carried out in the Maple computer mathematics system. presents the results of calculations by formulas (3–9).
Table 4. The matrix of non-integer values of deviations of the mechanical and physical parameters of GMBC from the optimal values, as well as the values of convolutions and criteria.
In , the following designations are adopted: ya — additive convolution (4);
yms — multiplicative convolution (5); ymd — additional multiplicative convolution (6); Vald – Vald criterion (7); Laplas – Laplace criterion (8); Hurwitz – Hurwitz criterion (9). The optimal values of the criteria and convolutions are highlighted in bold and in color.
An analysis of the results obtained allows us to state that the minimization of convolutions (additive, multiplicative and additional multiplicative), as well as the Wald and Laplace criteria, give an unambiguous conclusion – the optimal composition G + FC + CFI. This means the composition of cement, activator, fireclay, and carbon fiber I with 100 g, 90 g, 100 g, and 2.5 g, respectively content by weight.
The values of the convolutions and the Laplace criterion closest to the minimum indicate the G + FC composition, which can be put in second place in terms of optimal physical and mechanical properties.
Conclusions
Algorithm-built hybrid implementation of criterion-based estimation and probabilistic dynamics of weakly structured data. It is proposed to use composite algorithms for solving complex optimization problems, combining experimental results with their processing by different methods. Such transformational models have several advantages as a general model is exploited. The architecture of the models is easily extensible and adaptable to loads through scaling.
A hybrid intelligent system is a set of statistical simulation models combining different classes of control and design problem-solving. The quantitative side of loosely structured tasks and process performance evaluation is solved using Markov chains, while criterion methods are used for quantitative conclusions, and the final result best adapts the results of both applications.
The optimal composition G + FC + CFI (), which means the composition of cement, activator, fireclay, and carbon fiber I with 100 g, 90 g, 100 g, and 2.5 g, respectively content by weight.
The principal outcomes of this investigation have revealed that the incorporation of carbon fibers exerts a positive influence on both the mechanical strength and thermal conductivity of geopolymer composite materials.
Highlights
The study proposes an algorithm-built hybrid implementation to optimize geopolymer composites based on composition and performance parameters.
The hybrid intelligent system includes statistical simulation models that combine different control and design problem-solving approaches, using Markov chains to address quantitative aspects of loosely structured tasks and process performance evaluation.
The optimal composition, denoted as G + FC + CFI, is identified with specific weight content consisting of cement, activator, fireclay, and carbon fiber I, with 100g, 90g, 100g, and 2.5g, respectively
The proposed approaches provide enhanced efficiency and accuracy in solving complex optimization problems in the field of geopolymer composite materials and valuable insights into their optimization.
Disclosure statement
No potential conflict of interest was reported by the author(s).
Additional information
Funding
References
- Akmal, J., M. Badaruddin, M. K. Ismoyo, and S. D. Yuwono. 2019. “Optimization of Matrix Compositions of Al2O3, SiO2, Caolin, and CaO on the Mechanical Properties of a Geopolymer Composite with Short Carbon Fiber.” In IOP Conference Series: Materials Science and Engineering, 602:012079. IOP Publishing. https://doi.org/10.1088/1757-899X/602/1/012079
- Akono, A. T., Y. Cui, A. Kataruka, K. Anderson, and P. Kabir. 2018. “Intrinsic Mechanical Properties of Calcium Aluminate Crystals via the Linear Comparison Composite Method Coupled with Nano-Indentation.” Mechanics of Materials 118 (March 1): 74–14. https://doi.org/10.1016/j.mechmat.2017.12.007.
- Akono, A. T., S. Koric, and W. M. Kriven. 2019. “Influence of Pore Structure on the Strength Behavior of Particle-And Fiber-Reinforced Metakaolin-Based Geopolymer Composites.” Cement and Concrete Composites 104:103361. https://doi.org/10.1016/j.cemconcomp.2019.103361.
- Amalia, F., and N. Akifah. 2017. “Development of Coconut Trunk Fiber Geopolymer Hybrid Composite for Structural Engineering Materials.” In IOP Conference Series: Materials Science and Engineering, 180:012014. IOP Publishing. https://doi.org/10.1088/1757-899X/180/1/012014
- Buketov, A. V., A. V. Sharko, D. A. Zinchenko, and D. M. Stepanchikov. 2017. “To the Problem of Ingredients Optimization of Composite Materials Based on Epoxy Resin.” Bulletin of the Karaganda University-Mathematics 86 (2): 37–44. https://doi.org/10.31489/2017M2/37-44.
- Davidovits, J. 1991. “Geopolymers: Inorganic Polymeric New Materials.” Journal of Thermal Analysis and Calorimetry 37 (8): 1633–1656. https://doi.org/10.1007/BF01912193.
- Davidovits, J. 1994. “Geopolymers: Man-Made Rock Geosynthesis and the Resulting Development of Very Early High Strength Cement.” Journal of Materials Education 16:91–91.
- Duan, X., M. George, and F. Bullo. 2019. “Markov Chains with Maximum Return Time Entropy for Robotic Surveillance.” IEEE Transactions on Automatic Control 65 (1): 72–86. https://doi.org/10.1109/TAC.2019.2906473.
- Erofeev, V. T., A. I. Rodin, V. V. Yakunin, and M. N. Tuvin. 2019. “Structure, Composition, and Properties of Geopolymers from Mineral Wool Waste.” Magazine of Civil Engineeringno 6 (90): 3–14.
- Fernández, F., R. Jarabo, E. Asensio, and A. Guerrero. 2023. “Natural Fibers for a 3D Printable Eco-ECC Material.” In International RILEM Conference on Synergising Expertise towards Sustainability and Robustness of CBMs and Concrete Structures, 683–694. Cham: Springer Nature Switzerland.
- Hadigheh, S. A., F. Ke, and H. Fatemi. 2022. “Durability Design Criteria for the Hybrid Carbon Fibre Reinforced Polymer (CFRP)-Reinforced Geopolymer Concrete Bridges.” In Structures, 325–339. Vol. 35. Elsevier.
- Khan, M. Z. N., Y. Hao, H. Hao, F. U. A. Shaikh, and K. Liu. 2018. “Mechanical Properties of Ambient Cured High-Strength Plain and Hybrid Fiber Reinforced Geopolymer Composites from Triaxial Compressive Tests.” Construction and Building Materials 185:338–353. https://doi.org/10.1016/j.conbuildmat.2018.07.092.
- Korniejenko, K., P. Kejzlar, and P. Louda. 2022. “The Influence of the Material Structure on the Mechanical Properties of Geopolymer Composites Reinforced with Short Fibers Obtained with Additive Technologies.” International Journal of Molecular Sciences 23 (4): 2023. https://doi.org/10.3390/ijms23042023.
- Korniejenko, K., M. Łach, S.-Y. Chou, W.-T. Lin, A. Cheng, M. Hebdowska-Krupa, S. Gądek, and J. Mikuła. 2020. “Mechanical Properties of Short Fiber-Reinforced Geopolymers Made by Casted and 3D Printing Methods: A Comparative Study.” Materials 13 (3): 579. https://doi.org/10.3390/ma13030579.
- Lee, H., V. Vimonsatit, and P. Chindaprasirt. 2016. “Mechanical and Micromechanical Properties of Alkali Activated Fly-Ash Cement Based on Nano-Indentation.” Construction and Building Materials 107 (March 15): 95–102. https://doi.org/10.1016/j.conbuildmat.2015.12.013.
- Le, V. S., M. M. Szczypinski, P. Hájková, V. Kovacic, T. Bakalova, L. Volesky, L. C. Hiep, and P. Louda. 2020. “Mechanical Properties of Geopolymer Foam at High Temperature.” Science and Engineering of Composite Materials 27 (1): 129–138. https://doi.org/10.1515/secm-2020-0013.
- Ludwig, R., B. Pouymayou, P. Balermpas, and J. Unkelbach. June 10, 2021. “A Hidden Markov Model for Lymphatic Tumor Progression in the Head and Neck.” Scientific Reports 11 (1): 12261. https://doi.org/10.1038/s41598-021-91544-1.
- Nosov, P., A. Ben, S. Zinchenko, I. Popovych, V. Mateichuk, and H. Nosova. 2020. “Formal Approaches to Identify Cadet Fatigue Factors by Means of Marine Navigation Simulators.” In ICTERI Workshops, 823–838.
- Noushini, A., and A. Castel. 2018. “Performance-Based Criteria to Assess the Suitability of Geopolymer Concrete in Marine Environments Using Modified ASTM C1202 and ASTM C1556 Methods.” Materials and Structures 51 (6): 146. https://doi.org/10.1617/s11527-018-1267-z.
- Odhiambo, J., P. Weke, and P. Ngare. 2020. “Modeling Kenyan Economic Impact of Corona Virus in Kenya Using Discrete-Time Markov Chains.” Journal of Finance and Economics 8 (2): 80–85.
- Rama Seshu, D., and B. S. Kumar. 2019. “Binder Index as Criteria for Assessing the Strength of Geopolymer Concrete.” Journal of Structural Engineering 46 (1): 12–16.
- Sair, S., B. Mandili, M. Taqi, and A. El Bouari. 2019. “Development of a New Eco-Friendly Composite Material Based on Gypsum Reinforced with a Mixture of Cork Fibre and Cardboard Waste for Building Thermal Insulation.” Composites Communications 16:20–24. https://doi.org/10.1016/j.coco.2019.08.010.
- Sandanayake, M., C. Gunasekara, D. Law, G. Zhang, S. Setunge, and D. Wanijuru. 2020. “Sustainable Criterion Selection Framework for Green Building Materials–An Optimisation Based Study of Fly-Ash Geopolymer Concrete.” Sustainable Materials and Technologies 25:e00178. https://doi.org/10.1016/j.susmat.2020.e00178.
- Sharko, M., O. Liubchuk, G. Krapivina, N. Petrushenko, O. Gonchar, K. Vorobyova, and N. Vasylenko. 2022. “Information Technology to Assess the Enterprises’ Readiness for Innovative Transformations Using Markov Chains.” In International Scientific Conference “Intellectual Systems of Decision Making and Problem of Computational Intelligence”, 197–213. Cham: Springer International Publishing.
- Sharko, A., P. Louda, V. V. Nguyen, K. E. Buczkowska, D. Stepanchikov, R. Ercoli, P. Kascak, and V. S. Le. 2023. “Multicriteria Assessment for Calculating the Optimal Content of Calcium-Rich Fly Ash in Metakaolin-Based Geopolymers.” Ceramics 6 (1): 525–537. https://doi.org/10.3390/ceramics6010031.
- Sharko, M., N. Petrushenko, O. Gonchar, N. Vasylenko, K. Vorobyova, and I. Zakryzhevska. 2022. “Information Support of Intelligent Decision Support Systems for Managing Complex Organizational and Technical Objects Based on Markov Chains.”
- Van, S., P. Hájková, V. Kovacic, T. Bakalova, V. Lukáš, C. H. I. H. Le, K. C. Seifert, A. P. Peres, and P. Louda. 2019. “Thermal Conductivity of Reinforced Geopolymer Foams.” Ceramics-Silikáty 63 (4): 365–373. https://doi.org/10.13168/cs.2019.0032.
- Wang, J., C. Li, Y. Wan, C. Zhang, J. Ran, and M. W. Fu 2020. “Size Effect on the Shear Damage Under Low Stress Triaxiality in Micro-Scaled Plastic Deformation of Metallic Materials.” Materials and Design 196:109107. https://doi.org/10.1016/j.matdes.2020.109107.
- Xu, F., X. Deng, C. Peng, J. Zhu, and J. Chen. 2017. “Mix Design and Flexural Toughness of PVA Fiber Reinforced Fly Ash-Geopolymer Composites.” Construction and Building Materials 150:179–189. https://doi.org/10.1016/j.conbuildmat.2017.05.172.