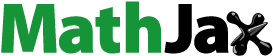
ABSTRACT
Exploring the differences of cotton quality among the four production areas in Aksu, Xinjiang and tracing the origin can improve cotton quality and cotton blending efficiency. In this study, nine quality indicators of 2000 cotton samples from four regions were analyzed by one-way analysis of variance to determine the differences of cotton from different regions. Principal component analysis (PCA) and Fisher discriminant analysis (DA) were used to establish the origin traceability model. Results show that there were significant differences in the quality of cotton from different regions. PCA extracted the first five principal components, and the cumulative variance contribution rate reaches 82.11%, 17.89% information are lost in the PCA model. The origin traceability model established by discriminant analysis shows that the accuracy of sample verification reaches 85.4% and the accuracy of cross-validation reaches 85.1%. Therefore, the Fisher discriminant model based on cotton quality indicators can effectively discriminate cotton from different regions in Xinjiang, China.
摘要
探讨新疆阿克苏四个产区棉花品质的差异,追溯产地,可以提高棉花品质和混棉效率. 本研究采用单向方差分析法对四个地区 2000 个棉花样品的9项质量指标进行了分析,以确定不同地区棉花的差异. 采用主成分分析(PCA)和Fisher判别分析(DA)建立原产地可追溯性模型. 结果表明,不同地区棉花品质存在显著差异. 主成分分析提取了前五个主成分,累积方差贡献率达到82.11%,主成分分析模型中信息丢失17.89%. 通过判别分析建立的原产地溯源模型表明,样本验证的准确率达到85.4%,交叉验证的准确度达到85.1%. 因此,基于棉花质量指标的Fisher判别模型可以有效地判别新疆不同地区的棉花.
Introduction
Cotton (Gossypium hirsutum L.) is the main raw material for textile (Kamalha et al. Citation2017). All kinds of textiles made of cotton fiber are closely related to people’s life. The quality of cotton fiber is judged by some quality indicators, such as Moisture, Micronaire, Upper half mean length, and Strength. These quality indicators are influenced by many factors, mainly climate (Luo, Bange, and Johnston Citation2016; UI-Allah et al. Citation2021), variety (Carvalho et al. Citation2022; Hussain et al. Citation2022), harvesting methods, and processing conditions. China is the significant producer of cotton, and most of China’s cotton are produced in northwest region, Xinjiang Uygur Autonomous Region, which is the largest province in China and shares nearly 90% of China’s total production. The cotton production in the Aksu, Xinjiang has always been at a high level, which is closely related to its unique natural conditions. Among which Kuche City, Wensu County, Xinhe County and Shaya County are representative areas for producing high-quality cotton. At present, there are many cotton varieties in Xinjiang and there are significant differences in cotton quality among different varieties (), which allows to select high-quality cotton varieties matching to local conditions, but it also led to low consistency of cotton performance and increased difficulties in cotton blending in the downstream spinning enterprises. Therefore, comparing the quality characteristics of cotton from different regions and establishing a reliable origin tracing model can not only improve the efficiency of cotton blending but also improve the spinning quality.
Table 1. Average values of main fiber characteristics of different cotton varieties.
At present, the origin tracing of agricultural products has been carried out by using near-infrared spectroscopy technology (Feng et al. Citation2021), stable isotope technology (Suzuki Citation2021), and mineral element fingerprint technology. The combination of Fourier transform near-infrared spectroscopy and stoichiometry has achieved in origin traceability of various food and agricultural products (Girolamo et al. Citation2019; Innamorato et al. Citation2019; Qian et al. Citation2022; Silva et al. Citation2021). Stable isotope method also has good effects on origin tracing. Gong et al. (Citation2018) combined stable isotope ratio and fatty acid analysis to trace the source of giant squid. There are many studies which has analyzed the content of mineral elements in agricultural products to identify their sources (Chen et al. Citation2022; Li et al. Citation2022; Ye et al. Citation2016). It can be seen that the origin tracing of agricultural products has been mainly achieved by analyzing the characteristic indicators of different sources of agricultural products.
In recent years, the study of origin traceability has been the focus of relevant scholars, and most methods still have limitations in achieving the identification of agricultural product origins. In this study, nondestructive identification of cotton production areas was achieved by using multivariate statistical analysis method based on the differences in cotton quality among different production areas in Aksu, Xinjiang. At the same time, we also verified the accuracy of the cotton origin traceability model. This research will provide theoretical support for the accuracy of cotton origin traceability.
Materials and methods
Test material
All data in this study were sourced from China’s cotton fair inspection data. In order to ensure the comparability of differences in cotton production from different regions and the accuracy of origin traceability, this study used prefecture-level cities and counties in the Aksu, Xinjiang as the basic units () and selected 2000 cotton samples from 4 different ginning factories randomly for statistical analysis. The detailed information of cotton samples are shown in .
Table 2. Table of geographical information on cotton samples.
According to the current Chinese national standard GB1103 ”Cotton Gossypium hirsutum L.,” there are many indicators that describe the quality of cotton. This study selected nine indicators, including Moisture (MSTR), Micronaire (Mic), Upper half mean length (UHML), short fiber index (SFI), strength (Str), color grade: whiteness (Rd), color grade: yellowness (+b), trash particle (count) (TrCnt), and uniformity index (UI) to analyze the quality differences of cotton from different production areas in Aksu and conduct a study on cotton production traceability.
Fiber testing
In this study, the quality indicators of cotton fibers from different regions were tested using the same method. The tests were mainly conducted using USTER HVI900. Mic, UHML, Str, UI, and SFI were tested according to the Chinese national standard GB/T 20,392–2006. The test results of Mic were retained to two decimal places, and the test results of UHML, Str, UI, SFI were retained to one decimal place. Rd and +b were tested by selecting inspection samples according to the standard GB/T 20,392–2006. TrCnt was tested according to the standard GB/T 6499–2012, and the results were retained as integers. In addition, the MSRT was tested using a bake oven, and the results were retained to one decimal place (SAC Citation2006).
Statistical and analytical methods
One-way analysis of variance
Analysis of variance (ANOVA) is a statistical method used to determine the impact of categorical independent variables on numerical dependent variables (Jia Citation2015). ANOVA is usually used to test the significance of differences in the mean of two or more samples. The ANOVA that only considers the impact of one factor on the observed data is called one-way analysis of variance.
Let there be i treatments (such as Kuche, Wensu, and Shaya) for factor A (such as Mic, MSTR, and +b), and the one-way ANOVA can be represented in the following model:
where, yij represents the j-th observation value in the i-th (i = 1, 2, …, I) treatment; represents the total average of the observed values without considering the influence of factors;
represents the error caused by the observation value when the treatment is i (i = 1, 2, …, I);
represents the random error of the j-th observation in the i-th treatment. Therefore, to test whether there is a significant effect of different origins on cotton quality indicators is to test the following hypothesis:
(different origins have no significant impact on cotton quality indicators).
At least one is not equal to 0 (different origins have a significant effect on cotton quality indicators).
Principal component analysis
Principal component analysis is a commonly used analysis method in multivariate statistical analysis, which converts multiple indicators into several comprehensive indicators through dimension reduction. The core idea of principal component analysis is to reduce the dimension of the data, so that each principal component can reflect most of the information in the original data, and the information in each part are not repeated.
Discriminant analysis
Discriminant analysis is a supervised classification method that determines the attribution of a research object based on its eigenvalues during classification determination. Its basic principle is to establish a discriminant function according to certain criteria, determine the coefficients of the discriminant function using the information data of the research object, and calculate the discriminant indicators.
Data analysis
SPSS software was used to analyze data. Origin software was used to visualize the analysis results.
Results and analysis
Quality analysis of cotton from different producing areas
SPSS software was used to conduct significance tests for major quality indicators of the selected 2000 cotton samples. Given the test level , the test results are shown in . As shown in , the
-values of MSTR, Mic, UHML, SFI, Str, Rd, +b, TrCnt and UI of cotton samples from different regions were all less than 0.05. Therefore, the original hypothesis has been rejected, which means that there are significant differences in the quality indicators of cotton from different regions.
Table 3. One-way ANOVA test results.
In order to clarify the specific differences in quality indicators of cotton from different regions, Duncan multiple comparisons were used to analyze the quality indicators of cotton samples from different regions. shows the specific differences in quality indicators of cotton samples from four regions, with different letters indicating significant differences (Zhang et al. Citation2017). The MSTR of cotton samples from four production areas ranged from 5.5% to 6.4%, and the MSTR of cotton samples from Xinhe County was significantly higher than that of other regions. There was no significant difference in the MSTR of cotton samples from Kuche City and Shaya County. The Mic was mainly concentrated between 4.2 and 4.9, and the Mic of cotton samples in Xinhe County was significantly higher than those in other regions. The range of the UHML was concentrated between 27.8 mm and 28.3 mm, and the UHML of the cotton sample in Shaya County was significantly higher than that in other regions. There was no significant difference in the UHML between the cotton samples in Kuche City and Wensu County. The SFI of the sample was concentrated between 16.1% and 22.1%, and the SFI of the cotton sample in Xinhe County was significantly higher than that of the other three regions. There was no significant difference in the SFI between the cotton samples in Kuche City and Shaya County. The range of Str was concentrated between 25.0cN/tex and 27.6cN/tex. There was no significant difference in the Str of cotton samples from Kuche City and Wensu County, but which was significantly higher than the other two regions. The Rd of samples from various regions ranged from 80.6% to 81.6%, and the Rd of cotton samples from Xinhe County was significantly higher than that of the other three regions. The +b was concentrated between 7.9 and 8.2, and there was no significant difference in the +b between the cotton samples from Kuche City and Wensu County, but which was significantly higher than the other two regions. There was also no significant difference in the +b between the cotton samples from Xinhe County and Shaya County. The TrCnt was concentrated between 9.7 and 14.7, and the TrCnt of cotton samples in Xinhe County was significantly higher than that in other regions. There was no significant difference in the TrCnt between cotton samples in Kuche City and Shaya County. The UI was concentrated between 80.6% and 82.4%, and the UI of the cotton sample in Wensu County was significantly higher than that of the other three regions.
Origin traceability model based on principal component analysis
Principal component analysis was used to compare nine quality indicators of 2000 cotton samples. As shown in , the top five principal components were extracted, and the cumulative variance contribution rate of the top five principal components was as high as 82.11%, which includes most of the effective information required for classification. The explanatory power of principal components PC1, PC2, PC3, PC4, and PC5 on total variance were 26.94%, 21.16%, 13.31%, 11.59%, and 9.11%. This study also analyzes indicators that contribute significantly to each principal component. As shown in , the contribution of SFI and UI to the first principal component shared the greatest; The second principal component combines three indicators: UI, Str and Mic; The third principal component was mainly influenced by two indicators: Rd and +b; The fourth principal component was most affected by the MSTR; The TrCnt was the maximum load on the fifth principal component.
Table 4. Explanation of total variance in principal component analysis.
The two-dimensional scatter plots of cotton samples from four regions are shown in . The total cumulative variance contributions of the scatter plots for PC1-PC2, PC1-PC3, and PC2-PC3 were 48.1%, 40.3%, and 34.5%. From the scatter plot, it can be seen that the cotton samples from Xinhe County, Wensu County, and Shaya County showed a certain clustering trend on the graph, and their place of origin differentiation was obvious. However, the cotton samples from Kuche City has overlapped with those from other regions, which makes it difficult to distinguish. In the scatter plot of PC1-PC2, cotton from Xinhe County, Wensu County, and Shaya County can be well distinguished; In the PC1-PC3 scatter plot, there was a partial overlap in the samples from Xinhe County, Wensu County, and Shaya County; In the PC2-PC3 scatter plot, there was severe overlap in the samples of Kuche City, Xinhe County, Wensu County, and Shaya County. This may be due to the low cumulative contribution of PC1-PC3 and PC2-PC3 to the total variance, with 59.7% and 65.5% of information lost in the PCA model. Essentially, unsupervised principal component analysis cannot reliably distinguish cotton from four production areas. This was mainly due to the low resolution of PC1, PC2, and PC3, and the total variance contribution rate of the first five principal components was only 82.11%. Therefore, in order to accurately trace the origin of cotton from different regions, other multivariate statistical methods should be adopted to improve identification accuracy.
Origin traceability model based on discriminant analysis
The difference in quality indicators of cotton from different regions is the basis for identifying their origin. This study used MSTR, Mic, UHML, SFI, Str, Rd, +b, TrCnt, UI as characteristic variables to establish four Fisher discriminative model for cotton producing areas and obtain four discriminant functions.
Fisher linear discriminant function:
In the formula, represent the numerical values of MSTR, Mic, UHML, SFI, Str, Rd, +b, TrCnt, UI.
Fisher function and general discriminant analysis were used to verify the results of cotton sample origin discrimination. From , it can be seen that this model has achieved the differentiation of cotton production areas successfully, with a discrimination accuracy of 85.4% and a cross-validation accuracy of 85.1%. The correct discrimination rate of cotton samples in Kuche City has reached 67.2%, which has a high probability of misjudgment; The discrimination accuracy of cotton samples in Wensu County has reached 82%, with 18% of the samples being misjudged; The discrimination accuracy of cotton samples in Xinhe County has reached 95.8%, with 4.2% of the samples being misjudged; The discrimination accuracy of cotton samples in Shaya County has reached 95.2%, with 4.8% of the samples being misjudged. This model has a high probability of misjudgment of cotton in Kuche City, which is consistent with the results of one-way ANOVA mentioned earlier. The quality indicators of cotton samples in Kuche City have small differences compared to other regions. It can be seen that the establishment of Fisher discriminative model based on cotton quality indicators can realize the traceability of cotton origin in Aksu, Xinjiang.
Table 5. Specific discrimination results of origin discrimination model.
Discussion
The physical properties of cotton fibers are influenced by multiple indicators, and cotton seed is one of the main factors causing differences in cotton fiber quality. The quality indicators of cotton fibers were used by Addis et al. (Citation2022) to categorize cotton in Ethiopia and distinguish cotton varieties. Teodoro et al. (Citation2019) chosed the best cotton genotypes by evaluating the fiber quality of 19 cotton cultivars. According to earlier research, cotton fiber from various sources has varying quality. In this study, one-way ANOVA was used to confirm that there were variations in cotton fiber quality from different producing areas, which was consistent with the findings of earlier research. It also served as a foundation for identifying cotton producing areas based on the variations in cotton quality in subsequent studies.
Traceability of agricultural products is a challenging issue. In recent years, many scholars have used different methods to identify the geographical origin of products. Jin et al. (Citation2023) studied the spectral information of Cornus officinalis from 11 origins, combined principal component analysis with support vector machine to achieve the identification of the producing areas of Cornus officinalis. Berriel et al. (Citation2019) successfully distinguished honey produced in two regions of Uruguay using discriminant analysis based on the mineral composition in honey. In order to identify the origin of the cotton, this study used principal component analysis and discriminant analysis. The model loses a significant amount of information as a result of the restricted main component information that PCA can extract, making it ineffective at different cotton sources. The model established by discriminant analysis has higher accuracy.
At present, many varieties of cotton are planted in Xinjiang, which is the main reason for the differences in cotton quality among different producing areas. The performance indicators of cotton already exist in the existing public inspection system, and these indicators can show the characteristics of the origin in some extent. So, it is possible to distinguish the cotton producing areas in Xinjiang by analyzing indicators of varieties cotton. Thus, this method solves the problem of poor cotton consistency caused by abundance of cotton varieties in Xinjiang effectively and will improve the yarn quality in subsequent spinning processes.
Conclusion
This study conducted one-way ANOVA on the quality indicators of 2000 cotton samples from 4 production areas in Aksu, Xinjiang. It was found that there were significant differences in the quality indicators of cotton samples from different production areas. These differences provided data support for subsequent research on the traceability of cotton production areas in Xinjiang.
Principal component analysis was used to analyze the first five principal components and two-dimensional scatter plots of the first three principal components were drawn. Results show that the clustering effect of the scatter plots from the four production areas has no differences, and there was a serious overlap of samples from individual production areas. Therefore, the accuracy of principal component analysis in distinguishing cotton production areas is not high.
This study used the general discriminant analysis to establish the discriminant model with nine quality indicators of cotton. The model correctly classified 85.4% of the modeling sample data and 85.1% of the cross-validated data, which indicates that using the quality indicators of cotton to establish a Fisher discriminant model can effectively identify the origin of cotton in Xinjiang.
Highlights
Differences in cotton quality indicators from different regions.
Traceability of origin can be achieved based on differences in cotton quality.
A cotton origin traceability model with high accuracy was established.
Author contributions
The manuscript was written through contributions of all authors. All authors have given approval to the final version of the manuscript.
Consent
All participants in the manuscript consent to publish.
Ethical approval
I confirm that all the research meets ethical guidelines and adheres to the legal requirements of the study country.
Acknowledgments
We are especially grateful to the Xinjiang Uygur Autonomous Region Fiber Quality Monitoring Center for its help in this study.
Disclosure statement
No potential conflict of interest was reported by the author(s).
Additional information
Funding
References
- Addis, T., A. Kachi, N. T. Akankwasa, and J. Wang. 2022. “Analysis of the Cotton Varieties Under Production in Ethiopia.” Journal of Natural Fibers 19 (4): 1522–11. https://doi.org/10.1080/15440478.2020.1780181.
- Berriel, V., P. Barreto, and C. Perdomo. 2019. “Characterisation of Uruguayan Honeys by Multi-Elemental Analyses as a Basis to Assess Their Geographical Origin.” Foods 8 (1): 24. https://doi.org/10.3390/foods8010024.
- Carvalho, L. P., F. J. C. Farias, R. G. Santos, L. P. R. Teodoro, and P. E. Teodoro. 2022. “Genotype Selection for Fiber Quality Traits in Cotton in the Brazilian Northeast.” Agronomy Journal 114 (6): 3068–3073. https://doi.org/10.1002/agj2.21176.
- Chen, M. M., L. X. Fu, D. W. Li, F. Zuo, and L. L. Qian. 2022. “Mineral Element Fingerprints Verified the Geographical Origin of Years and Quantities of Rice.” Journal of Food Composition and Analysis 114:104803. https://doi.org/10.1016/j.jfca.2022.104803.
- Feng, L., B. H. Wu, S. S. Zhu, Y. He, and C. Zhang. 2021. “Application Of visible/Infrared Spectroscopy And hyperspectral Imaging with Machine learning Techniques for Identifying food Varieties and Geographicalorigins.” Frontiers in Nutrition 8:680357. https://doi.org/10.3389/fnut.2021.680357.
- Girolamo, A. D., M. Cortese, S. Cervellieri, V. Lippolis, M. Pascale, A. F. Logrieco, and M. Suman. 2019. “Tracing the Geographical Origin of Durum Wheat by FT-NIR Spectroscopy.” Foods 8 (10): 450. https://doi.org/10.3390/foods8100450.
- Gong, Y., Y. K. Li, X. J. Chen, and L. Chen. 2018. “Potential Use of Stable Isotope and Fatty Acid Analyses for Traceability of Geographic Origins of Jumbo Squid (Dosidicus Gigas).” Rapid Communications in Mass Spectrometry 32 (7): 583–589. https://doi.org/10.1002/rcm.8071.
- Hussain, A., M. Sajid, D. Iqbal, M. L. Sarwar, M. A. Farooq, A. Siddique, M. Q. Khan, and I.-S. Kim. 2022. “Impact of Novel Varietal and Regional differences on Cotton Fiber Qualitycharacteristics.” Materials 15 (9): 3242. https://doi.org/10.3390/ma15093242.
- Innamorato, V., F. Longobardi, V. Lippolis, M. Cortese, A. F. Logrieco, L. Catucci, A. Agostiano, and A. D. Girolamo. 2019. “Tracing the Geographical Origin of Lentils (Lens Culinaris Medik.) by Infrared Spectroscopy and Chemometrics.” Food Analytical Methods 12 (3): 773–779. https://doi.org/10.1007/s12161-081-1406-8.
- Jia, J. P. 2015. Statistics. 1rd ed. Beijing, China: China Renmin University Press.
- Jin, Y. Q., B. Liu, C. N. Li, S. S. Shi, and N. A. Mahat. 2023. “Origin identification of Cornus Officinalis Based on PCA-SVM Combined Model.” PLOS ONE 18 (2): e0282429. https://doi.org/10.1371/journal.pone.0282429.
- Kamalha, E., J. Kiberu, I. Nibikora, J. I. Mwasiagi, and E. Omollo. 2017. “Clustering and Classification of Cotton Lint Using Principle Component analysis, Agglomerative Hierarchical Clustering, and K-Means Clustering.” Journal of Natural Fibers 15 (3): 425–435. https://doi.org/10.1080/15440478.2017.1340220.
- Li, Q. H., C. F. Li, S. Y. Xiao, H. Wang, C. Chen, X. Wei, and X. F. Wen. 2022. “Tracing the Origins of Blueberries by Their Mineral Element Contents and 87Sr/86Sr Ratios.” Biological Trace Element Research 200 (2): 920–930. https://doi.org/10.1007/s12011-021-02701-6.
- Luo, Q. Y., M. Bange, and D. Johnston. 2016. “Environment and cotton fibre quality.” Climatic Change 138 (1–2): 207–221. https://doi.org/10.1007/s10584-016-1715-0.
- Qian, L. L., D. W. Li, X. J. Song, F. Zuo, and D. J. Zhang. 2022. “Identification of Baha’sib Mung Beans Based on Fourier Transform Near Infrared spectroscopy and Partial Least Squares.” Journal of Food Composition and Analysis 105:104203. https://doi.org/10.1016/j.jfca.2021.104203.
- SAC (Standardization Administration of China). 2006. GB/T 6102.1-2006 Test method for moisture regain in raw cotton by oven drying.
- Silva, L. K. R., J. C. Jesus, R. R. V. Onelli, D. G. Conceição, L. S. Santos, and S. P. B. Ferrão. 2021. “Discriminating Coalho Cheese by Origin Through near and Middle Infrared Spectroscopy and Analytical Measures. Discrimination of Coalho Cheese Origin.” International Journal of Dairy Technology 74 (2): 393–403. https://doi.org/10.1111/1471-0307.12767.
- Suzuki, Y. 2021. “Achieving Food Authenticity and Traceability Using an Analytical Method Focusing on Stable Isotope Analysis.” Analytical Sciences 37 (1): 189–199. https://doi.org/10.2116/analsci.20SAR14.
- Teodoro, P. E., F. J. C. Farias, L. P. de Carvalho, L. P. Ribeiro, M. Nascimento, C. F. Azevedo, C. D. Cruz, and L. L. Bhering. 2019. “Adaptability and Stability of Cotton Genotypes Regarding Fiber Yield and Quality Traits.” Crop Science 59 (2): 518–524. https://doi.org/10.2135/cropsci2018.04.0250.
- UI-Allah, S., A. Rehman, M. Hussain, and M. Farooq. 2021. “Fiber Yield and Quality in Cotton Under Drought: Effects and Management.” Agricultural Water Management 255:106994. https://doi.org/10.1016/j.agwat.2021.106994.
- Ye, X. H., S. Jin, D. H. Wang, F. Zhao, Y. Yu, D. Y. Zheng, and N. X. Ye. 2016. “Identification of the Origin of White Tea Based on Mineral Element Content.” Food Analytical Methods 10 (6): 191–199. https://doi.org/10.1007/s12161-016-0568-5.
- Zhang, X., D. P. Hu, Y. Li, Y. Chen, H. M. A. A. Eltayib, Z. D. Dong, D. H. Chen, and L. Zhang. 2017. “Developmental and hormonal regulation of fiber quality in two natural-colored cotton cultivars.” Journal of Integrative Agriculture 16 (8): 1720–1729. https://doi.org/10.1016/S2095-3119(16)61504-6.