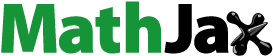
ABSTRACT
The study, comprised seventeen genotypes, was conducted during 2016 to 2019 growing seasons. The design was Randomized Complete Block Design (RCBD) with three replications to identify adaptable cotton genotypes. Parametric and non-parametric measures, principal component analysis (PCA) and correlation among the ranks of the parameters computed using R software. The environment, genotype and genotype-environment interaction contributed 79.58, 3.2 and 15.46% of the variation, respectively, and were significant (p < 0.001). The highest (1510.7 kg/ha) and lowest (1080.1 kg/ha) seed cotton yield was recorded from G12 and G8 genotypes respectively. Coefficients of determination (R2) and superiority index (Pi) recognized G13, G11, G10 and G11, G12, G7 as the top three stable genotypes correspondingly. G10 was selected three times as superior by stability variance (σ2), wricke’s ecovalence (Wi) and deviation from regression (S2di). Seed cotton yield positively correlated with GAI, Kang’s rank-sum (KRS), Huhn and Nassar S(1), S(6) S(3) while negatively correlated with Thennarasu stability measures NP(2), NP(3) and NP(4 . The first, second and third clusters of the PCA biplot comprised, 4, 4 and 10 parameters respectively. G12, with highest seed cotton yield and good stability, was the best genotype and recommended for variety verification.
摘要
这项研究包括17种基因型,在2016年至2019年的生长季节进行. 该设计采用随机完全块设计(RCBD),进行三次重复,以鉴定适应性棉花基因型. 参数和非参数测量,主成分分析(PCA)和使用R软件计算的参数等级之间的相关性. 环境、基因型和基因型-环境相互作用分别贡献了79.58%、3.2和15.46%的变异,具有显著性(p<0.001). G12和G8基因型的籽棉产量最高(1510.7公斤/公顷),最低(1080.1公斤/ha). 决定系数(R2)和优势指数(Pi)分别认定G13、G11、G10和G11、G12、G7为前三个稳定基因型. G10被稳定性方差选为三倍优 (σ2),wricke的生态价(Wi)和回归偏差(S2di). 籽棉产量与GAI、Kang秩和(KRS)、Huhn和Nassar s(1)、s(6)s(3)呈正相关,而与Thennarasu稳定性指标NP(2)、NP(3)和NP(4)负相关. PCA双图的第一、第二和第三聚类分别包括4个、4个和10个参数. G12是棉花产量最高、稳定性好的最佳基因型,推荐用于品种验证.
Introduction
Cotton (Gossypium hirsutum L.) belongs to the family Malvaceae, and the genus Gossypium comprised about 50 species of which only four (G. hirsutum, G. herbaceum, G. barbadense, and G. arboreum) are cultivated while the remaining 46 are wild growing in the tropics and sub-tropics (Gotmare, Singh, and Tule Citation2000). The upland cotton (Gossypium hirsutum) is the most popular under cultivation more than any other of the cultivated species (Liu et al. Citation2013). Moreover, the tetraploid Gossypium hirsutum and Gossypium barbadense are the most widely cultivated cotton species nowadays (Deng et al. Citation2018). A total of 52 flavonoids, that play a role in cotton fiber quality and color, from cotton, Gossypium hirsutum (Nix, Paull, and Colgrave Citation2017). Asia (68.7%), America (21.1%) and Africa (6.3%) are the largest seed cotton producing continents and China, India, United States of America, Pakistan and Brazil are the top seed cotton yield producing countries in the world (Faostat Citation2021). In terms of cotton production area, 87% of the world’s coverage occurs in developing countries (Nix, Paull, and Colgrave Citation2017). Cotton is currently growing worldwide in approximately 75 countries mainly in the warmer and hotter dry areas of the tropics subtropics and temperate regions (Ayana et al. Citation2016). Cotton is the most important source of fiber and edible oil covering 40% of the global textile need and 3.3% of edible oil and its seed contains 16% to 24% oil (Khan et al. Citation2010). Cotton is among the most important seed fiber crops in the world using as a raw material in many spinning and weaving industries, where the fibers from cotton covers 85–90% of the total fibers production and it contains 18–26% oil of the weight of the seeds (Al-Obaidi and Al-Jubouri Citation2023). As a result, it is called the king of natural fiber crops (Saleem et al. Citation2009).
Environmental variations among growing environments result in poor yield performance in cotton (Boyer Citation1982). According to Allard and Bradshaw (Citation1964) environmental variation can be divided into two components as predictable and unpredictable. The former one includes, cropping system, management, long-term climatic condition and others. The latter includes like amount and distribution of rainfall, temperature, established population and others. The lint quality and the seed cotton yield particularly is highly sensitive to agro-climatic conditions (Abro, Rizwan, Deho, et al. Citation2022) indicating the execution of Multi-Environment Trial (MET) in cotton to identify adaptable genotypes is vital. The presence of a significant genotype-environment interaction (GEI) in yield and yield components and lint quality of seed cotton yield is a common phenomenon (Abro, Rizwan, Rajput, et al. Citation2022; De Assis Machado et al. Citation2002; Peixoto et al. Citation2021; Riaz et al. Citation2019; Sadabadi et al. Citation2018; Satish, Jain, and Chhabra Citation2009; Shahzad et al. Citation2019; Teodoro et al. Citation2019). Hence, it is important to conduct a MET to develop stable and high yielding varieties. The existence of significant GEI interaction is both a good opportunity and a challenge for plant breeders (Baraki, Tsehaye, and Abay Citation2014) and in such situations it is important to combine the yield performance and stability (M. S. Kang and Magari Citation1995). To identify the stable ones three different methods, viz. parametric univariate, non-parametric univariate, and multivariate methods, can be used (Flores, Moreno, and Cubero Citation1998). As a result, most breeders and agronomists faced a problem in selecting the most suitable measures to identify desirable genotype and it seems about the breeders’ choice to select the appropriate methods based on different aspects. In this study, different parametric and non-parametric stability measures are used and similarly, Kamali et al., (Citation2009);Kamali et al., (Citation2011) on seed cotton yield and Ahmadi et al., (Citation2018);Cenci and Saavedra (Citation2019);Kılıç (Citation2012) on other crops used parametric and non-parametric stability statistics to identify desirable genotypes.
It is widely cultivated because of it is important raw material for a different products, like textiles, paper, livestock feed, edible oil, medicinal products and many others and hence, the world production is increasing from time to time reached about 41.3 million tons in 2020 (FAOSTAT Citation2021). Cotton is a good source of cash for the producers in addition to its role as an export item in the national economic development of the country. Furthermore, it offers considerable employment opportunity on farms, industry, and commercial trade, input and service sectors. However, the productivity especially in developing countries is very low (Ayana et al. Citation2016). Gebregergis et al., (Citation2020) reported that the seed cotton yield in Northern Ethiopia is very low ranging from 1720–2610 kg/ha because of different intermingled constraints. The absence of high yielding varieties is among the most important constraints in Ethiopia that resulted in low productivity and production. Such problems can be solved and desired varieties can be investigated by executing MET using many genotypes. Hence, the objective of this study is to identify high seed cotton yielding and stable cotton genotypes to boost productivity in the growing areas of western Tigray, Ethiopia.
Materials and methods
Description of the material and designs
The present experiment was conducted in the Western Tigray, Northern Ethiopia, during the 2016–2019 cropping seasons under rainfed conditions in 8 environments and 17 cotton genotypes, obtained from breeding program in werer agricultural research center, were used in the trial (). The design was randomized complete block design (RCBD) with three replications and each plot consisted of 5 meter long five rows with 90 cm and 20 cm spacing between rows and plants respectively. Data on seed cotton yield were taken from the middle rows of each plot and total seed cotton yield (kg/ha) was calculated for each genotype at each test environment.
Table 1. Genotype designation, growing environment and testing sites description.
Statistical analysis
AMMI Analysis of variance
The seed cotton yield was subjected to a combined analysis of variance for seed yield and AMMI model, which combines the conventional ANOVA and PCA (Zobel Citation1990). This was used to determine the Genotype (G), environment and genotype by environment interaction (GEI) as well as to further partition the GEI into interaction principal components (IPCs). Tukey HSD was performed to explain the significant differences among mean seed cotton yields of the 17 cotton genotypes. The AMMI model for the yield of the ith genotype in the jth environment is computed as:
; Where: Yij: the observed mean yield of ith genotype in the jth
environment; µ: the grand mean; gi: the ith genotypic effect (G); ej: the jth environment effect (Kaiser); is the GEI;
the eigenvector for the ith genotype from nth IPCA;
the eigen vector for the jth environment from the nth IPCA;
the GE interaction; N: the number of IPCA retained in the model; and
the random error term.
Moreover, different parametric and non-parametric stability measures described in () were also computed.
Table 2. Parametric and non-parametric stability measures and their descriptions.
Principal Component Analysis and Association of the Stability Measures
Finally based on the ranks of genotypes in the different environments spearman’s rank correlation analysis (Piepho and Lotito Citation1992) was undertaken to estimate the correlation among the different stability statistics. For better visualization of the association of the stability measures PCA was computed to detect interrelationships among the stability statistics using the “factoextra” package (Kassambara and Mundt Citation2020) of R software 4.2.2. While all other data were analyzed with the assistance of R statistical environment version 4.2.2 (R Core Team Citation2022) using “metan” package (Olivoto, Lúcio, and Jarman Citation2020).
Results
Combined AMMI Analysis of Variance and genotypic performance
The seed cotton yield data were subjected to AMMI analysis, which combines analysis of variance (ANOVA) with additive and multiplicative parameters in to a single model (Gauch Citation2013). The results of the combined ANOVA for seed cotton yield showed that the genotypes (G), environments and GEI were significant (p < 0.001). This confirms the genotypes were different in their genetic potential and the environments in which the genotypes were grown were different. The E, GEI and G effects contributed 79.58%, 15.46%, and 3.2% of the total variation respectively and the GEI was partitioned further to seven statistically significant PCA with 35.8 and 27.9% of PCA1 and PCA2 respectively (). The E effect was more than five times of the GEI effect and more than 28 time of the G effect. There was statistical significant difference in seed cotton yield among the cotton genotypes and the highest seed cotton yield was recorded from G12 and G11 with 1510.75 and 1474.9 kg/ha respectively while the lowest seed cotton yield (1080.14 kg/ha) was recorded from G8 and the grand mean seed cotton yield was 1325.28 kg/ha. Regarding to the locations and growing environments, there was a statistically significant difference among the environments and locations (). The highest (2320.5 kg/ha) and the lowest (602.5 kg/ha) seed cotton yield were recorded from E1 and E6 correspondingly.
Table 3. Combined AMMI ANOVA of 17 cotton genotypes.
Table 4. Seed cotton yield (Kg/ha) across the growing environments and locations.
Parametric Stability Measures
Since the effect of genotypes on seed cotton yield was found highly significant, mean of the seed cotton yield was taken into account and for this reason a total of 17 parametric and non-parametric stability measures and seed cotton yield rank were calculated to identify the stable genotypes. According to the seed cotton yield rank (Yld) G12, G11 and G7 were the top three high yielding and G8, G2 and G3 were the low yielding cotton genotypes (). According to Eberhart and Russell (Citation1966), regression coefficients (bi) genotypic values near to unity (bi = 1) lowest deviation from regression (S2di = 0) is considered as the most stable. Accordingly, bi and S2di identified G9, G17, G15, and G10, G13 and G15 as the most stable genotypes respectively (). The coefficients of determination (R2) (Pinthus Citation1973) and Superiority index (Pi) (Lin and Binns Citation1988) recognized G13, G11, G10 and G11, G12 and G7 as the top three stable genotypes correspondingly while coefficient of variation (Francis and Kannenberg Citation1978) identified genotypes G16, G12, G10 as the top three stable genotypes. G10 was selected more than any other genotype (three times) as the most stable one by Shukla stability variance (σ2) (Shukla Citation1972), Ecovalence (Wi) (Wricke Citation1962) and the Eberhart and Russell (Eberhart and Russell Citation1966) deviation from regression (S2di). Exceptionally, geometric adaptability index (GAI) (Mohammadi and Amri Citation2008), Superiority index (Pi) (Lin and Binns Citation1988) and seed cotton yield rank selected three genotypes viz. G7, G11 and G12 as the top three stable ones and these genotypes are statistically non-significant and are the high yielding genotypes (). Both the seed cotton yield rank and GAI are the only stability measures which identified G12 as the most stable one ranked first.
Table 5. Values and ranks of parametric stability measures for 17 cotton genotypes.
Non-parametric stability measures
Nassar and Huehn’s (Hühn and Nassar Citation1989) statistics, Thennarasu’s (Thennarasu Citation1995) statistics and Kang’s (M. Kang Citation1988) rank-sum and ranks for seed cotton yield of 17 cotton genotypes are depicted in (). Based on the Nassar and Huehn stability measures of S(1), identified G1, G17, G7, G10 and G15 as the most stable while G3, G16, G6, G5 and G11 as unstable genotypes. G15 had the lowest values of S(2), S(3) and S(6) stability measures and as a result was selected as the most stable genotype and the latter two stability measures also ranked G10 as the second stable genotype. Generally, S(2), S(3) and S(6) stability measures also recognized for G10, G13 and G15 as the most stable (with in top five) genotypes and for genotypes G3, G6, G8 and G9 as unstable genotypes. Based on the second non-parametric stability statistics (Thennarasu’s stability) it is clear from the () that genotypes with the lowest values are recognized as more stable while those with highest value as unstable. The lowest value of NP(1) was recorded from G15 followed by G13, G4, G2 and G1 and so that these genotypes are the most stable ones respectively according to this stability statistics. NP(2) selected G2, G15, G4, G8 and G15 as stable ones in that order and G12, G17 and G7 as unstable ones. Similar to NP(2), NP(3) also selected G2 as the top stable genotype followed by G15, G8 and G4. G1 had the lowest NP(4) and as a result selected as the most stable while G12 with the highest value was selected as the unstable genotype ranked in the 17th. G11, G13, G15, G7 and G10 had the lowest values of Kang’s rank-sum stability statistics and hence, are the most stable genotypes and these genotypes had greater than the average seed cotton yield. On the other hand, G9, G8 and G16 had the highest value of this stability statistics and declared as the unstable genotypes while G11, G13 and G15 with smallest value as the highly stable.
Table 6. Values and ranks of non-parametric stability measures for 17 cotton genotypes.
Correlation among stability parameters
The mean seed cotton yield was positively and significantly correlated with GAI, KRS, Pi and S(6) (p < 0.001), with Wi (p < 0.01) and with S(3) (p < 0.05) but it was negatively and significantly (p < 0.005) correlated with NP(2) and NP(3) (). The seed cotton yield rank also had negative and positive, but non-significant, correlation with NP(4) and NP(1) respectively. S2 di and bi had non-significant correlation among each other and GAI had a positive and significant correlation with Yld, Wi, Pi, KRS, and S(6) (p < 0.001), S(3) (p < 0.005), with S(3) (p < 0.005) and CV (p < 0.05) while significantly negative (p < 0.05) correlation with NP(2) and NP(3). Most of the Nassar and Huehn stability measures had a positive and significant correlation among each other except that of S(1) which showed non-significant but positive correlation with S(2) and S(6). Among the Thennarasu’s stability measures only NP(3) had a positive and significant correlation with NP(1) and NP(2) and all the others had a positive but non-significant correlation to each other. Exceptionally, all of these Thennarasu’s stability parameters had non-significant correlation with the Kankg’s rank sum. Kang’s rank sum had a positive and significant correlation with Yld, σ2 (Sh), Wi, R2, Pi, GAI, S(3) and S(6) (p < 0.001), with S2 di (p < 0.005) and with S(2) (p < 0.05) while it was negatively but non-significantly correlated with NP(2), NP(3) and bi.
Principal component analysis
Based on the rank correlation matrix a principal component analysis was executed. The first five PCs accounted 92.38% of the total variation of the stability parameters. The first PC accounted 44.9% of the total variation and it was significantly associated with KRS, S6, S3, S2, GAI, Pi, S2di, Wi, Sh, and Yld. PC2 (20.3%) was mainly influenced by NP3, NP2 and NP1. PC3 (13.8%) was associated with NP4, bi, S1 and R2. PC5 that accounted only 5.9% of the total variation was associated only with CVP while there was no considerable correlation between PC4 (7.3%) and the stability statistics (). PC1 and PC2 revealed the highest (65.3%) percent of variance and hence, these two PCs were used to create a PCA-based biplot. In the biplot the stability statistics and the seed cotton yield clustered in to three clusters: cluster I comprised of NP1, NP2, NP3 and S2; cluster II consisted of NP4, S1, R2 and bi; and cluster III included Yld, GAI, Pi, KRS, CV, Wi, S6, S3, Sh, S2di. Seed cotton yield showed strong positive correlation with the stability statistics in cluster II and cluster III.
Discussion
There is a high possibility of the occurrence of significant GEI in many MET which can be occurred if the different genotypes performed differently across the given environments and it is familiar to most researches (Allard and Bradshaw Citation1964). Such interactions may be with no interaction, quantitative interaction or qualitative interaction (Ceccarelli Citation2015). The combined ANOVA of the AMMI model in this study demonstrated that, both the environment, genotype (G) and genotype x environment interaction (GEI) were significant with 79.58%, 3.2% and 15.46% of the total variation correspondingly (). This corroborates with the findings of Farias et al., (Citation2016);Maleia et al., (Citation2010);Shahzad et al., (Citation2019) who found a significant E, G and GEI using AMMI model ANOVA. Campbell and Jones (Citation2005);Killi and Harem (Citation2006);Riaz et al., (Citation2019);Unay et al., (Citation2004) also conducted studies in cotton genotypes and found a significance of E, G and GEI. Specifically, the former three authors also reported that the significant variation was because of E, GEI and G accordingly. There was statistical significant difference among the genotypes evaluated across the eight environments in their seed cotton yield (). Ten cotton genotypes viz. G12, G11, G7, G17, G14, G15, G13, G5, G10 and G1 are high yielding genotypes with above the average mean seed cotton yield while the remaining seven genotypes yielded lower than the grand mean (). G12, G11 and G7 genotypes with mean seed cotton yield of 1510.75, 1474.9 and 1460.73 kg/ha were the high yielding genotypes respectively while G8 was the lowest yielding genotype. The productivity of seed cotton yield varies from 1855–3174 kg/ha (Ali, Aslam, and Hussain Citation2005), 1445–2877 kg/ha (Riaz et al. Citation2019), 1720–2612 kg/ha (Gebregergis et al. Citation2020) and 1960–3096 kg/ha (Shahzad et al. Citation2019). However, the productivity obtained in this study is lower than earlier reports which may be due to genetic variability of the study materials and the environments under which the experiments were conducted. However, Zeng et al., (Citation2014) and Vineeth et al., (Citation2022) reported a seed cotton yield of lower than 1275.5 kg/ha and 1100 kg/ha respectively which is lower than the average seed cotton yield of genotypes in this study.
Yield stability refers to a genotype’s capability to perform consistently across a wide range of environments (Annicchiarico Citation2002). Regarding to the stability, genotypes with the smallest variance for a studied trait across the growing environments are considered stable, considered as a biological or static concept of stability (Becker and Leon Citation1988). Based on the second concept of stability, the dynamic or agronomic concept of stability, a given genotype is recognized as stable if its response to the growing environments is parallel to the average response of all genotypes in the trial (Becker and Leon Citation1988). The former stability concept is not acceptable by most agronomists and breeders, since they desire genotypes having higher under favorable conditions or improved agronomic practices (Robert, Citation2002). On the other hand, Simmonds (Citation1991) reflected that the static stability may be more important than the dynamic stability in different situations especially in agricultural production with limited agronomic practices. Both parametric and non-parametric stability statistics have their own strengths and limitations (Delic, Stankovic, and Konstantinov Citation2009) and hence, most breeders are integrating both parametric and non-parametric stability measures. Furthermore, some others are also integrating different multivariate analyses in addition to these stability measures. As a result, Heravani et al., (Citation2005);Kamali et al., (Citation2009) used different parametric and non-parametric stability measures in their study in cotton crop.
The variability in seed yield of genotype may be predictable (regression) and unpredictable (variance deviation) (Eberhart and Russell Citation1966). As a result, regression method was executed for the stability analysis of the genotypes. Accordingly, G9 with the bi value equal to unity (bi = 1) is the most stable one and hence this bi can be considered as biological or static concept of stability (Eberhart and Russell Citation1966). Riaz et al., (Citation2019) used bi to identify stable and adapted cotton for its seed cotton yield and suggested the importance of using this stability measure. Although this G9 was selected as the stable genotype by bi this genotype was low yielder (1209 kg/ha) which is below the average seed cotton yield. The high seed cotton yielder genotype G12 (1510.7 kg/ha) was identified among the unstable genotypes together with G4, G5, G11 and G16 since their bi values had larger variation from unity. On the other hand, this G12 was recognized as it is among the top four stable ones according to CV, Wi, Pi, GAI, S(3) and S(6) indicating there is a bit controversy with the bi. Similar result was also reported by Vaezi et al., (Citation2019) in selection of stable barley genotypes while this is in contradiction to the findings of Temesgen et al., (Citation2015) in faba bean where this bi could recognized for the high yielding genotypes as the stable ones. Furthermore, Shahbazi (Citation2019) also reported that, CV, Pi and GAI are the stability measures used to choose the high yielding genotype. According to the deviation from regression, G10, G13 and G15 with the lowest values of S2 di are considered as the most stable genotypes and all produced above the average mean yield (). Kang’s, Citation1988 (KRS), considers the seed cotton yield rank and the Shukla stability variance (σ2) rank so as to recognize genotypes with lowest value as stable while those with highest value as unstable. Accordingly, G11, G13 and G15 are declared as the most stable while G9, G8 and G16 declared as unstable. The stable genotypes according to this parameter had a seed cotton yield above the average mean and those declared as unstable had below the average seed cotton yield and accordingly this study suggest that KRS may be among the important parameters to select desirable genotypes which is similar to the findings of (Farshadfar, Sabaghpour, and Zali Citation2012).
Non parametric stability measures of Thennarasu (N(i)) and Huhn stability statistics of Si(1), Si(2), Si(3) and Si(6) computed based on the genotype rankings in the different environments () distinguishing genotypes with minimum change of rank across environments are known as stable genotypes while those of with higher changes of ranks as unstable. The Huhn stability statistics of Si(1), Si(2), Si(3) and Si(6) identified genotypes G6, G8 and G9 among the unstable genotypes while G10 and G15 among the most stable genotypes. This is in contrast to the findings of Kilic et al., (Citation2010);Temesgen et al., (Citation2015) who reported these parameters were able to recognize for high yielding genotypes as unstable while for low yielding genotypes as stable. Similar to the bi, Thennarasu’s stability of N(2), N(3) and N(4) selected G12, with the highest values of these statistics, as most unstable genotype. This is because of the performance of this genotype highly varies across environments ranging from 758.4–2528.4 kg/ha in E6 and E7 respectively () confirming there was high variability among the growing environments and this is in line with the findings of Kaya and Taner (Citation2003).
Among the parametric stability measures, GAI, Pi and Wi had positive and significant correlation with seed cotton yield while the remaining had non-significant correlation. Hence, GAI, Pi and Wi are among the best parametric measures to identify superior genotypes and this is in accordance with Kaya and Taner (Citation2003). Related to the Huehn stability measures Si(3) and Si(6) had a positive and significant correlation with the seed cotton yield rank and these parameters are associated with the agronomic stability. According to Hühn and Nassar (Citation1989) these stability measures combines mean yield and stability based on stability ranks in the growing environments. Si(2) had a positive and significant (p < 0.001) correlation with Si(3) and Si(6) which is in line with the findings of Farshadfar et al., (Citation2012), Furthermore, Si(1) had significant correlation with Si(3) and similarly Scapim et al., (Citation2000) also reported a significant correlation among Si(1) and Si(3). N(1) had significant correlation with Si(2), Si(3) and Si(6). Similarly, Abdulahi et al., (Citation2008);Farshadfar et al., (Citation2012) also found significant correlation of N(1) with Si(2) and Si(3). NP(1) and NP(4) had positively and negatively but non-significant correlation with the seed cotton yield rank. On the other hand, NP(2) and NP(3) had a negatively significant correlation with the yield rank and this study indicates they are not appropriate measures to select high yielding genotypes. Moghaddam and Pourdad (Citation2009) also reported that both the Huehn and Thennarasu’s stability measures had a negative correlation with the seed cotton yield rank, indicating there is no complete similarity of these parameters in prioritizing the genotypes. Unlike to this study, Kadhem et al., (Citation2010) reported that all of these parameters showed negative correlation with the seed cotton yield rank suggesting these are not important to select stable rice genotypes. However, in contrast to this, Vaezi et al., (Citation2018) reported that all of these stability measures showed positive correlation with seed cotton yield rank suggesting as they are important to select stable barley genotypes. Among the Thennarasu’s stability measures it was only NP(3 which had highly and positively correlation with NP(1) and NP(2).which is different from the findings of Vaezi et al., (Citation2018) who reported all of these parameters had a positive and significant correlation among each other. KRS had a positive and significant correlation with Yld, σ2, Wi, R2, Pi, S(3), S(6), S2 di and S(2) indicating it is important to incorporate KRS with these stability measures to select a desirable genotype. However, Khalili and Pour (Citation2016) reported that this Kang’s rank sum had a negative and significant correlation with seed cotton yield rank, S(3), and all the Thennarasu’s stability measures. Generally, the seed cotton yield was positively and significantly correlated with GAI, KRS, Pi, S(6), Wi, and S(3), S(6) and this study suggests these parameters may be important in selecting high yielding and stable genotype. The different stability measures used in this study are not consistent in identifying the stability of the genotypes since some genotypes become stable according to some parameters while unstable according to other parameters. Such phenomenon is a common challenge in GEI studies and similar suggestions were forwarded by Khalili and Pour (Citation2016);Vaezi et al., (Citation2018).
A PCA biplot using the rank correlation matrix of 17 parametric and non-parametric stability measures in seed cotton yield was executed. Kaiser (Citation1960) suggested that PCs greater than a unity is considered as significant and accordingly five PCs. The first five PCs accounted 92.38% of the total variation of the stability statistics. The first PC accounted for 44.9% of the total variation and it was significantly correlated with KRS, S6, S3, S2, GAI, Pi, S2di, Wi, Sh, and Yld. The second PC contributed 20.3% of the total variation and it was mainly influenced by NP3, NP2 and NP1. PC3 explained 13.8% of the total variation and it was associated with NP4, bi, S1 and R2. PC5 that accounted only 5.9% of the total variation was associated only with CV while there was no considerable correlation between PC4, which accounted 7.3%, and the stability statistics (). This corroborates with the findings of Pour-Aboughadareh et al., (Citation2023) who reported that most of the stability statistics were associated with the first six PCs and similarly, Pour-Aboughadareh et al., (Citation2021) also reported that most of the physiological traits were associated with the first five PCs.
PC1 and PC2 were used to create a PCA-based biplot, which is vital to investigate the relationships between seed cotton yield and stability statistics; and the association between the stability statistics. In the biplot, a cosine angle between two statistics vectors shows a strong positive correlation; where as a large cosine angle between the statistics shows a weak correlation. On the other hand, no correlations and strong negative correlations were displayed at cosine 90° and 180° respectively. Accordingly, seed cotton yield showed strong positive correlation with the stability statistics in cluster II and cluster III (). Hence, these stability statistics express a dynamic concept of stability due to their close correlation with the seed cotton yield. Similarly, Moghaddam and Pourdad (Citation2009) also clustered the seed cotton yield with the likes of Pi, KRS and bi while this is in contrast to the results of Kadhem et al., (Citation2010);Temesgen et al., (Citation2015) who reported seed yield was negatively correlated with these parameters. On the other hand, it was not correlated with NP1 and S2 while it was strongly and negatively correlated with NP2 and NP3. According to the biplot the stability statistics and the seed cotton yield grouped in to three clusters (I-III). Cluster I comprised of four statistics viz. NP1, NP2, NP3 and S2; cluster II consisted of four parameters namely NP4, S1, R2 and bi; and cluster III included Yld and other nine stability parameters viz., GAI, Pi, KRS, CV, Wi, S6, S3, Sh and S2di. Seed cotton yield (Yld) belongs to the third cluster confirming it had positive association with the stability statistics in this cluster. This result corroborates with the findings of Pour-Aboughadareh et al., (Citation2023) and Verma et al., (Citation2018).
Conclusion and recommendation
In the current study, a significant GEI was found confirming there was a variation among the genetic potential of the genotypes, the environments and their interaction confirming the seed cotton yield rank variation across environments. In such situations, selecting desirable genotypes, integrating parametric, non-parametric and other graphical measures in a given study seems ideal for agronomists and plant breeders. Seed cotton yield was positively associated with nine stability parameters viz., GAI, Pi, KRS, CV, Wi, S6, S3, Sh and S2di indicating these stability statistics express a dynamic concept of stability because of their positive association with the seed cotton yield. Both the seed cotton yield rank, GAI, Pi and Wi identified G12 (the high yielder genotype) as most stable genotype ranked first and this genotype was also moderately stable according to some other stability parameters. Hence, this genotype considered as superior genotype and recommended for variety verification to get recognition from the Ministry of Agriculture of the country and to be used as improved variety in the country. Finally, this study recommends to developing a new cotton variety in the study areas, it is crucial to evaluate a range of genotypes across years and locations to see the spatial, temporal and their interaction, which enables to evaluate their performances and stabilities simultaneously.
Highlight
Genotype “Delcero x dellopile 90 f5-5-4-z-2” (G12) is the high yielder and moderately stable genotype and hence, this genotype is recommended in the region and the country as well to be used as new variety.
Cotton seed is positively associated with nine stability parameters viz., GAI, Pi, KRS, CV, Wi, S6, S3, Sh and S2di indicating these stability statistics express a dynamic concept of stability.
This study recommends to develop a new variety it is crucial to evaluate genotypes across years and locations to see the spatial, temporal and their interaction.
This study suggests integrating parametric, non-parametric and other graphical measures is vital to select stable and high yielding genotype.
Authors’ contributions
FB and ZG designed the study; FB, YB, ZG, GT, ZG, AA, AA and WN conducted the study at field level and collected the required data. FB analyzed the data and wrote the initial draft of the manuscript. ZG, YB and GT further developed the manuscript and all authors read and approved the final manuscript.
Acknowledgments
The authors would like to sincerely acknowledge to Tigray Agricultural Research Institute for the financial support, and they would like to extend their special thanks to all Humera Agricultural Research Center researchers for their commitment during conducting the present study.
Disclosure statement
No potential conflict of interest was reported by the author(s).
References
- Abdulahi, A., S. Pourdadand, and R. Mohammadi. 2008. “Study on Relationship Among Nonparametric Stability Statistics in Safflower.” Proceedings 7th International Safflower Conference, Wagga Wagga.
- Abro, S., M. Rizwan, Z. A. Deho, S. A. Abro, and M. A. Sial. 2022. “Identification of Heat Tolerant Cotton Lines Showing Genetic Variation in Cell Membrane Thermostability, Stomata, and Trichome Size and Its Effect on Yield and Fiber Quality Traits.” Frontiers in Plant Science 12:1–18. https://doi.org/10.3389/fpls.2021.804315.
- Abro, S., M. Rizwan, M. T. Rajput, M. A. Sial, and Z. A. Deho. 2022. “Evaluation of Upland Cotton Genotypes for Stability Over Different Locations Using Ammi and GGE Biplot Analysis.” Pakistan Journal of Botany 54 (5): 1733–1739. https://doi.org/10.30848/PJB2022-5(1).
- Ahmadi, J., B. Vaezi, A. Shaabani, K. Khademi, S. Fabriki Ourang, and A. Pour-Aboughadareh. 2018. “Non-Parametric Measures for Yield Stability in Gras S Pea (Lathyrus Sativus L.) Advanced Lines in Semi Warm Regions.” Journal of Agricultural Science and Technology 17 (7): 1825–1838.
- Ali, Y., Z. Aslam, and F. Hussain. 2005. “Genotype and Environment Interaction Effect on Yield of Cotton Under Naturally Salt Stress Condition.” International Journal of Environmental Science & Technology 2 (2): 169–173. https://doi.org/10.1007/BF03325872.
- Allard, R. W., and A. D. Bradshaw. 1964. “Implications of Genotype-Environmental Interactions in Applied Plant Breeding 1.” Crop Science 4 (5): 503–508. https://doi.org/10.2135/cropsci1964.0011183X000400050021x.
- Al-Obaidi, O. N. J., and J. M. A. Al-Jubouri. 2023. “Stability Study of Cotton Genotypes Cultivated in Different Environments.” IOP Conference Series: Earth and Environmental Science, United Kingdom, 1–10/062030. United Kingdom: IOP Publishing. https://doi.org/10.1088/1755-1315/1158/6/062030.
- Annicchiarico, P. 2002. Genotype X Environment Interactions: Challenges and Opportunities for Plant Breeding and Cultivar Recommendations. Rome, Italy: Food & Agriculture Org. https://books.google.com.et/books?hl=en&lr=&id=so4UJSzWNbIC&oi=fnd&pg=PR8&dq=Annicchiarico+P.,+(2002).+Genotype+x+environment+interactions:+challenges+and+opportunities+for+plant+breeding+and+cultivar+recommendations,+Food+%26+Agriculture+Org.&ots=dDZonBscX4&sig=8PITRYbaL574ivef-JGr8jZDDK4&redir_esc=y#v=onepage&q=Annicchiarico%20P.%2C%20(2002).%20Genotype%20x%20environment%20interactions%3A%20challenges%20and%20opportunities%20for%20plant%20breeding%20and%20cultivar%20recommendations%2C%20Food%20%26%20Agriculture%20Org.&f=false.
- Ayana, G., A. Abdo, Y. Merine, T. Jobie, A. Bekele, D. Mekonnen, F. Mekibib, G. Tabor, M. Amare, and M. Eshete. 2016. Plant Variety Release. Protection and Seed Quality Control Directorate. Addis Ababa, Ethiopia: Ministry of Agriculture and Natural Resources.
- Baraki, F., Y. Tsehaye, and F. Abay. 2014. “AMMI Analysis of Genotype× Environment Interaction and Stability of Sesame Genotypes in Northern Ethiopia.” Asian Journal of Plant Sciences 13 (4–8): 178–183. https://doi.org/10.3923/ajps.2014.178.183.
- Becker, H., and J. Leon. 1988. “Stability Analysis in Plant Breeding.” Plant Breeding 101 (1): 1–23. https://doi.org/10.1111/j.1439-0523.1988.tb00261.x.
- Boyer, J. S. 1982. “Plant productivity and environment.” Science 218 (4571): 443–448. https://doi.org/10.1126/science.218.4571.443.
- Campbell, B., and M. Jones. 2005. “Assessment of genotype× Environment Interactions for Yield and Fiber Quality in Cotton Performance Trials.” Euphytica 144 (1–2): 69–78. https://doi.org/10.1007/s10681-005-4336-7.
- Ceccarelli, S. 2015. “Efficiency of Plant Breeding.” Crop Science 55 (1): 87–97. https://doi.org/10.2135/cropsci2014.02.0158.
- Cenci, S., and S. Saavedra. 2019. “Non-Parametric Estimation of the Structural Stability of Non-Equilibrium Community Dynamics.” Nature Ecology & Evolution 3 (6): 912–918. https://doi.org/10.1038/s41559-019-0879-1.
- De Assis Machado, J. R., J. C. V. Penna, J. Fallieri, P. G. Santos, and M. A. Lanza. 2002. “Stability and Adaptability of Seedcotton Yields of Upland Cotton Genotypes in the State of Minas Gerais, Brazil.” Crop Breeding and Applied Biotechnology 2 (3): 401–410. https://doi.org/10.12702/1984-7033.v02n03a10.
- Delic, N., G. Stankovic, and K. Konstantinov. 2009. “Use of Non Parametric Statistics in Estimation of Genotypes Stability.” Maydica 54 (2): 155.
- Deng, F., X. Zhang, W. Wang, R. Yuan, and F. Shen. 2018. “Identification of Gossypium Hirsutum Long Non-Coding RNAs (lncRnas) Under Salt Stress.” BMC Plant Biology 18 (1): 1–14. https://doi.org/10.1186/s12870-018-1238-0.
- Eberhart, S. A., and W. Russell. 1966. “Stability Parameters for Comparing Varieties 1.” Crop Science 6:36–40. https://doi.org/10.2135/cropsci1966.0011183X000600010011x.
- FAOSTAT. 2021. “Food and Agriculture Organization of the United Nations Data, Production Quantities of Cotton Seed by Country.” https://www.fao.org/faostat/en/#data/QCL/visualize.
- Farias, F., L. De Carvalho, J. Da Silva Filho, and P. Teodoro. 2016. “Biplot Analysis of Phenotypic Stability in Upland Cotton Genotypes in Mato Grosso.” Genetics & Molecular Research 15 (2): 1–10. https://doi.org/10.4238/gmr.15028009.
- Farshadfar, E., S. H. Sabaghpour, and H. Zali. 2012. “Comparison of Parametric and Non-Parametric Stability Statistics for Selecting Stable Chickpea (Cicer Arietinum L.) Genotypes Under Diverse Environments.” Australian Journal of Crop Science 6 (3): 514–524.
- Flores, F., M. Moreno, and J. Cubero. 1998. “A Comparison of Univariate and Multivariate Methods to Analyze G× E Interaction.” Field Crops Research 56 (3): 271–286. https://doi.org/10.1016/S0378-4290(97)00095-6.
- Francis, T., and L. Kannenberg. 1978. “Yield Stability Studies in Short-Season Maize. I. A Descriptive Method for Grouping Genotypes.” Canadian Journal of Plant Science 58 (4): 1029–1034. https://doi.org/10.4141/cjps78-157.
- Gauch, H. G. 2013. “A Simple Protocol for AMMI Analysis of Yield Trials.” Crop Science 53 (5): 1860–1869. https://doi.org/10.2135/cropsci2013.04.0241.
- Gebregergis, Z., F. Baraki, G. Teame, and M. Tejada Moral. 2020. “Planting Geometry of Cotton Under Rain Fed Condition in the Dry Land Areas of Western Tigray.” Cogent Food & Agriculture 6 (1): 1–12. https://doi.org/10.1080/23311932.2020.1771113.
- Gotmare, V., P. Singh, and B. Tule. 2000. Wild and Cultivated Species of Cotton. Nagpur, India: Technical Bulletin; Central Institute for Cotton Research. 5.
- Heravani, J. L., N. Nemati, R. Bozorgipour, and Z. Hosseini-Negad. 2005. “Yield Stability of Some Promising Cultivars and Hybrids of Cotton (Gossypium hirsutum) in Northern Khorasan and Moghan Plain.” JWSS-Isfahan University of Technology 8 (4): 87–98.
- Hühn, M., and R. Nassar. 1989. “On Tests of Significance for Nonparametric Measures of Phenotypic Stability.” Biometrics Bulletin 45 (3): 997–1000. https://doi.org/10.2307/2531698.
- Kadhem, F. A., I. S. Al-Nedawi, S. D. Al-Atabe, and F. Y. Baktash. 2010. “Association Between Parametric and Nonparametric Measures of Phenotypic Stability in Rice Genotypes (Oryza Sativa L.).” Diyala Journal of Agricultural Sciences 2 (2): 20–33.
- Kaiser, T. 1960. “The Absorption Spectrum of Calcium Vapour in the Vacuum Ultra-violet.” Proceedings of the Physical Society 75 (1): 152–159. https://doi.org/10.1088/0370-1328/75/1/425.
- Kamali, S., O. Alishah, and N. Jelodar. 2009. “The Assessment of genotype× Environment Interaction Effect on and Yield Stability of Cotton Cultivars Using Uniparametric and Non-Parametric Methods.” Journal of Science and Technology of Agriculture and Natural Resources 13 (48 (B)): 41–51.
- Kamali, S., N. Jeloudar, and O. Alishah. 2011. “The Assessment of Adaptability and Stability of Yield on Cotton Cultivars by Using Uniparametric, Non-Parametric Methods and AMMI Model.” Iranian Journal of Field Crop Science 42 (2): 397–407.
- Kang, M. 1988. “A Rank-Sum Method for Selecting High-Yielding, Stable Corn Genotypes.” Cereal Research Communication 16 (1/2): 113–115.
- Kang, M. S., and R. Magari. 1995. “STABLE: A BASIC Program for Calculating Stability and Yield‐Stability Statistics.” Agronomy Journal 87 (2): 276–277. https://doi.org/10.2134/agronj1995.00021962008700020023x.
- Kassambara, A., and F. Mundt. 2020. “Factoextra: Extract and visualize the results of multivariate data analyses.” R package version, 1.0.7.
- Kaya, Y., and S. Taner. 2003. “Estimating Genotypic Ranks by Nonparametric Stability Analysis in Bread Wheat (Triticum Aestivum L.).” Journal of Central European Agriculture 4:47–54. https://hrcak.srce.hr/ojs/index.php/jcea/article/view/158.
- Khalili, M., and A. A. Pour. 2016. “Parametric and Non-Parametric Measures for Evaluating Yield Stability and Adaptability in Barley Doubled Haploid Lines.” Journal of Agricultural Science and Technology 18:789–803. https://scholar.google.com/scholar?hl=en&as_sdt=0%2C5&q=Khalili+M.%2C+Pour+A.A.%2C+(2016).+Parametric+and+non-parametric+measures+for+evaluating+yield+stability+and+adaptability+in+barley+doubled+haploid+lines.+J.+Agr.+Sci.+Tech.%2C+18%2C+789-803.&btnG=.
- Khan, A. I., F. S. Awan, B. Sadia, R. M. Rana, and I. A. Khan. 2010. “Genetic Diversity Studies Among Coloured Cotton Genotypes by Using RAPD Markers.” Pakistan Journal of Botany 42 (1): 71–77.
- Kılıç, H. 2012. “Assessment of Parametric and Nonparametric Methods for Selecting Stable and Adapted Spring Bread Wheat Genotypes in Multi–Environments.” Journal of Animal & Plant Sciences 22 (2): 390–398.
- Kilic, H., M. Akçura, and H. Aktaş. 2010. “Assessment of Parametric and Non-Parametric Methods for Selecting Stable and Adapted Durum Wheat Genotypes in Multi-Environments.” Notulae Botanicae Horti Agrobotanici Cluj-Napoca 38 (3): 271–279.
- Killi, F., and E. Harem. 2006. “Genotype X Environment Interaction and Stability Analysis of Cotton Yield in Aegean Region of Turkey.” Journal of Environmental Biology 37 (2): 427–430.
- Lin, C.-S., and M. R. Binns. 1988. “A superiority measure of cultivar performance for cultivar× location data.” Canadian Journal of Plant Science 68 (1): 193–198. https://doi.org/10.4141/cjps88-018.
- Liu, C., D. Yuan, X. Zhang, and Z. Lin. 2013. “Isolation, Characterization and Mapping of Genes Differentially Expressed During Fibre Development Between Gossypium Hirsutum and Gossypium Barbadense by cDNA-SRAP.” Journal of Genetics 92 (2): 175–181. https://doi.org/10.1007/s12041-013-0238-y.
- Maleia, M. P., P. S. Vidigal Filho, M. V. Kvitschal, and M. Vidigal. 2010. “Stability and adaptability of commercial cotton cultivars (Gossypium hirsutum L. race latifolium H.) in Mozambique.” African Journal of Agricultural Research 5 (7): 539–550. https://doi.org/10.5897/AJAR09.244.
- Moghaddam, M., and S. Pourdad. 2009. “Comparison of Parametric and Non-Parametric Methods for Analysing genotype× Environment Interactions in Safflower (Carthamus Tinctorius L.).” The Journal of Agricultural Science 147 (5): 601–612. https://doi.org/10.1017/S0021859609990050.
- Mohammadi, R., and A. Amri. 2008. “Comparison of Parametric and Non-Parametric Methods for Selecting Stable and Adapted Durum Wheat Genotypes in Variable Environments.” Euphytica 159 (3): 419–432. https://doi.org/10.1007/s10681-007-9600-6.
- Nix, A., C. Paull, and M. Colgrave. 2017. “Flavonoid Profile of the Cotton Plant, Gossypium hirsutum: A Review.” Plants 6 (4): 1–14. https://doi.org/10.3390/plants6040043.
- Olivoto, T., A. D. C. Lúcio, and S. Jarman. 2020. “Metan: An R Package for Multi‐Environment Trial Analysis.” Methods in Ecology and Evolution 11 (6): 783–789. https://doi.org/10.1111/2041-210X.13384.
- Peixoto, M. A., J. S. P. C. Evangelista, R. S. Alves, F. J. C. Farias, L. P. Carvalho, L. P. R. Teodoro, P. E. Teodoro, and L. L. Bhering. 2021. “Models for Optimizing Selection Based on Adaptability and Stability of Cotton Genotypes.” Ciência Rural 51 (5): 1–9. https://doi.org/10.1590/0103-8478cr20200530.
- Piepho, H.-P., and S. Lotito. 1992. “Rank Correlation Among Parametric and Nonparametric Measures of Phenotypic Stability.” Euphytica 64 (3): 221–225. https://doi.org/10.1007/BF00046052.
- Pinthus, M. J. 1973. “Estimate of genotypic value: A proposed method.” Euphytica 22 (1): 121–123. https://doi.org/10.1007/BF00021563.
- Pour-Aboughadareh, A., A. Barati, A. Gholipoor, H. Zali, A. Marzooghian, S. A. Koohkan, K. Shahbazi-Homonloo, and A. Houseinpour. 2023. “Deciphering Genotype-By-Environment Interaction in Barley Genotypes Using Different Adaptability and Stability Methods.” Journal of Crop Science and Biotechnology 26 (5): 1–16. https://doi.org/10.1007/s12892-023-00199-z.
- Pour-Aboughadareh, A., S. Sanjani, H. Nikkhah-Chamanabad, M. R. Mehrvar, A. Asadi, and A. Amini. 2021. “Identification of Salt-Tolerant Barley Genotypes Using Multiple-Traits Index and Yield Performance at the Early Growth and Maturity Stages.” Bulletin of the National Research Centre 45 (1): 1–16. https://doi.org/10.1186/s42269-021-00576-0.
- R Core Team. 2022. “R: A Language and Environment for Statistical Computing. R Foundation for Statistical Computing.” Vienna, Austria. https://www.rproject.org/.
- Riaz, M., J. Farooq, S. Ahmed, M. Amin, W. S. Chattha, M. Ayoub, and R. A. Kainth. 2019. “Stability Analysis of Different Cotton Genotypes Under Normal and Water-Deficit Conditions.” Journal of Integrative Agriculture 18 (6): 1257–1265. https://doi.org/10.1016/S2095-3119(18)62041-6.
- Robert, N.2002. “Comparison of Stability Statistics for Yield and Quality Traits in Bread Wheat.” Euphytica 128: 333–341.
- Sadabadi, M. F., G. Ranjbar, M. Zangi, S. Tabar, and H. N. Zarini. 2018. “Analysis of Stability and Adaptation of Cotton Genotypes Using GGE Biplot Method.” Trakia Journal of Sciences 16 (1): 51–61. https://doi.org/10.15547/tjs.2018.01.009.
- Saleem, M. F., S. A. Anjum, A. Shakeel, M. Y. Ashraf, and H. Z. Khan. 2009. “Effect of Row Spacing on Earliness and Yield in Cotton.” Pakistan Journal of Botany 41 (5): 2179–2188.
- Satish, Y., P. Jain, and B. Chhabra. 2009. “Stability Analysis for Yield and Its Component Traits in American Cotton (Gossypium Hirsutum L.).” Journal of Cotton Research and Development 23 (2): 175–182.
- Scapim, C. A., V. R. Oliveira, C. D. Cruz, C. A. D. B. Andrade, M. C. G. Vidigal, and M. C. G. Vidigal. 2000. “Yield Stability in Maize (Zea Mays L.) and Correlations Among the Parameters of the Eberhart and Russell, Lin and Binns and Huehn Models.” Genetics and Molecular Biology 23 (2): 387–393. https://doi.org/10.1590/S1415-47572000000200025.
- Shahbazi, E. 2019. “Genotype Selection and Stability Analysis for Seed Yield of Nigella Sativa Using Parametric and Non-Parametric Statistics.” Scientia Horticulturae 253:172–179. https://doi.org/10.1016/j.scienta.2019.04.047.
- Shahzad, K., T. Qi, L. Guo, H. Tang, X. Zhang, H. Wang, X. Qiao, et al. 2019. “Adaptability and Stability Comparisons of Inbred and Hybrid Cotton in Yield and Fiber Quality Traits.” Agronomy 9 (9): 1–16. https://doi.org/10.3390/agronomy9090516.
- Shukla, G. 1972. “Some Statistical Aspects of Partitioning Genotype Environmental Components of Variability.” Heredity 29 (2): 237–245. https://doi.org/10.1038/hdy.1972.87.
- Simmonds, N. 1991. “Selection for Local Adaptation in a Plant Breeding Programme.” Theoretical and Applied Genetics 82 (3): 363–367. https://doi.org/10.1007/BF02190624.
- Temesgen, T., G. Keneni, T. Sefera, and M. Jarso. 2015. “Yield Stability and Relationships Among Stability Parameters in Faba Bean (Vicia Faba L.) Genotypes.” The Crop Journal 3 (3): 258–268. https://doi.org/10.1016/j.cj.2015.03.004.
- Teodoro, P. E., F. J. C. Farias, L. P. De Carvalho, L. P. Ribeiro, M. Nascimento, C. F. Azevedo, C. D. Cruz, and L. L. Bhering. 2019. “Adaptability and Stability of Cotton Genotypes Regarding Fiber Yield and Quality Traits.” Crop Science 59 (2): 518–524. https://doi.org/10.2135/cropsci2018.04.0250.
- Thennarasu, K. 1995. “On certain non-parametric procedures for studying genotype-environment interactions and yield stability.” Ph. D. thesis. New Delhi, India: PJ School, IARI.
- Unay, A., H. Basal, A. Erkul, and Z. Yuksekkaya. 2004. “Stability Analysis of Upland Cotton Genotypes to the Aegean Region in Turkey.” Asian Journal of Plant Sciences 3 (1): 36–38. https://doi.org/10.3923/ajps.2004.36.38.
- Vaezi, B., A. Pour-Aboughadareh, A. Mehraban, T. Hossein-Pour, R. Mohammadi, M. Armion, and M. Dorri. 2018. “The Use of Parametric and Non-Parametric Measures for Selecting Stable and Adapted Barley Lines.” Archives of Agronomy and Soil Science 64 (5): 597–611. https://doi.org/10.1080/03650340.2017.1369529.
- Vaezi, B., A. Pour-Aboughadareh, R. Mohammadi, A. Mehraban, T. Hossein-Pour, E. Koohkan, S. Ghasemi, H. Moradkhani, and K. H. Siddique. 2019. “Integrating Different Stability Models to Investigate genotype× Environment Interactions and Identify Stable and High-Yielding Barley Genotypes.” Euphytica 215 (4): 63. https://doi.org/10.1007/s10681-019-2386-5.
- Verma, A., V. Kumar, A. Kharab, R. Chatrath, and G. Singh. 2018. “Parametric Vis-A-Vis Non Parametric Measures Describing G X E Interactions for Salt Salinity Tolerant Barley Genotypes in Multi-Environment Trials.” Journal of Applied and Natural Science 10 (2): 557–563. https://doi.org/10.31018/jans.v10i2.1736.
- Vineeth, T., I. Prasad, A. R. Chinchmalatpure, B. Lokeshkumar, S. Kumar, K. Ravikiran, and P. C. Sharma. 2022. “Weighted Average Absolute Scores of BLUPs (WAASB) Based Selection of Stable Asiatic Cotton Genotypes for the Salt Affected Vertisols of India.” Indian Journal of Genetics and Plant Breeding 82 (1): 104–108. https://doi.org/10.31742/IJGPB.82.1.15.
- Wricke, G. 1962. “Uber eine Methode zur Erfassung der okologischen Streubreite in Feldverzuchen. Z.” pflanzenzuchtg 47:92–96.
- Zeng, L., J. Meredith, B. T. Campbell, J. K. Dever, J. Zhang, K. M. Glass, A. S. Jones, G. O. Myers, and F. M. Bourland. 2014. “Genotype-By-Environment Interaction Effects on Lint Yield of Cotton Cultivars Across Major Regions in the US Cotton Belt.” Journal of Cotton Science 18 (1): 75–84.
- Zobel, R. 1990. “A Powerful Statistical Model for Understanding Genotype by Environment Interaction.” Genotype by Environment Interaction and Plant breeding’ (Ed MS Kang) 126–140. https://www.researchgate.net/profile/Richard-Zobel/publication/285717060_Ammi_analysis_of_yield_trials_In_Genotype-by-environment_interaction_kang_ms_and_hg_gauch_eds/links/56b4c2b408aebbde1a77922e/Ammi-analysis-of-yield-trials-In-Genotype-by-environment-interaction-kang-ms-and-hg-gauch-eds.pdf.