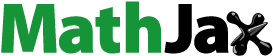
ABSTRACT
In Indian subcontinent, pineapple (Ananas Comosus) leaves are left unused after fruits are harvested resulting in a waste of natural resources that contain textile-graded fibers. The present study intended to develop a pineapple leaf fiber extractor to chip off the waxy layer of the leaf. The machine comprised feeding conveyor, feed rollers, scratching roller, combing roller, frame and motor as a power source with a transmission unit. The performance was evaluated under different independent variables, i.e. rpm of the scratching roller (100, 150 and 200 rpm), types of secondary roller (combing, serration and without secondary roller) and moisture content of leaf (85 ± 3, 78 ± 3 and 71 ± 2%). Response surface method of optimization was employed to optimize the operational parameters. Best combination of operating parameters was found to be 150 rpm scratching rollers, combing type secondary rollers and moisture content of 85 ± 3%. It was found that the retting duration, processing efficiency, and fiber yield were 4 days, 88.9%, and 2.41% at optimized parameters, respectively. Further, throughput capacity and power requirement were found to be 33 ± 2 kg/hand 1.5 kWh respectively. The developed machine found to be effective in extracting waxy layer on leaves with minimal damage to useable fiber.
摘要
在印度次大陆,菠萝(Ananas Comosus)的叶子在果实收获后被闲置,导致含有纺织级纤维的自然资源浪费. 本研究旨在开发一种菠萝叶纤维提取器,以去除菠萝叶的蜡质层. 该机器由给料输送机、给料辊、刮花辊、梳理辊、机架和带传动装置的电机组成. 在不同的自变量下评估性能,即刮花辊的转速(100、150和200rpm)、二次辊的类型(梳理、锯齿和无二次辊)和叶片的含水量(85 ± 3、78 ± 3和71 ± 2%). 采用响应面优化方法对运行参数进行了优化. 最佳操作参数组合为150rpm刮花辊、梳型二次辊和含水量为85 ± 3%. 研究发现,在优化的参数下,脱胶时间、加工效率和纤维产量分别为4d、88.9%和2.41%. 此外,吞吐量和功率要求分别为33 ± 2公斤/手1.5千瓦时. 开发的机器被发现可以有效地提取叶片上的蜡质层,对可用纤维的损害最小.
Introduction
The pineapple, a perennial member of the Bromeliaceae family (Ananas Comosus), is grown primarily in humid tropical and subtropical climates. A history of its cultivation suggests that it was originally cultivated in Central and South America, then spread to Europe and other parts of the world. Globally, Costa Rica demonstrates the highest production with 328.1 metric tons, followed by the Philippines, Brazil, Indonesia, China, and India (FAO Citation2019). The pineapple acreage in India is 105t ha, with production and productivity of 1740.00 MT and 17.04 MT/ha respectively. In terms of area under cultivation in India, Assam (17.85 t ha) state dominates, followed by Meghalaya (12.36), Manipur (12.29) and West Bengal (11.85 t ha) (Agricultural Statistics at a Glance Citation2022). After harvesting pineapple fruits, leaves are left unused, causing severe environmental pollution due to burning and landfills. Leaves of pineapple account for most of the wet plant residues generated per hectare, which amounts to about 250 metric tons (Saini et al. Citation2022). The pineapple leaves contain a certain amount of fiber, called pineapple leaf fiber (PALF), which comprises about 2 to 3.5% of the fresh leaves. Thus, conversion of pineapple leaf into fiber provides additional income to the growers. According to estimates, fiber extracted from pineapple leaf can augment income by 10–15% per ha for growers (Nayak, Nag, and Banik Citation2016). The extracted fiber can be used for manufacturing of yarns and handicrafts. Having similar properties to other natural fibers, pineapple leaf fiber can also be blended and used to produce furnishings and ornamental fabrics, thus expanding their use in various clothing and textile fields (Jalil et al. Citation2021).
Fibers from pineapple leaves can be extracted by manual, mechanical or chemical retting process. In the manual method immersion of leaf in water, removal of waxy layer followed by washing and drying are most prevalent steps and observed as an easy way to extract fibers. But being a tedious, time-consuming and less productive process, it also produces 50% more waste materials than mechanical extraction (Yahya Citation2016). Further, manual method of extraction involves the kneeling posture of a human being for a prolonged period which leads to knee bursitis. The extraction of fibers from leaves through retting by using DAPS or urea 0.5% has been studied in several research studies, but it is constraint to industrial application for large scale (Kengkhetkit and Amornsakchai Citation2012). As a result of the constraints of the aforementioned methods, mechanical extraction methods have been developed. A mechanical extraction method offers advantages such as reduced PALF waste, timeliness of operation and faster processing time (Yusof, Yahya, and Adam Citation2016).In China and Malaysia, there are commercial pineapple decorticator/extractor machines that employ beating and scraping method to extract fiber (Yusof, Yahya, and Adam Citation2015; Zhang et al. Citation2002). Due to the inherent softness and delicacy of pineapple fiber, these processes result in significant fiber breakage, leading to a reduced fiber yield. Moreover, extracted fibers are coarser in nature posing challenges to blend with other natural fibers (Rafiqah et al. Citation2020). The limitation of the aforementioned machines has prompted the development of a pineapple fiber waxy scraping machine. Banik et al. (Citation2011) reported about the development of a pineapple leaf extraction machine which comprised feed rollers, scratching rollers and serrated rollers. The function of the scratching and serration roller was to remove the waxy layer and to crush leaves for better retting by microbes. Das et al. (Citation2010) and Nayak et al. (Citation2016) studied the retting of machine-extracted pineapple leaves in bacterial cultures and found reduced retting time (6–8 days) compared to the control (10 days). Previous research on machines that remove waxy layers on the leaves followed by retting underscores the advantageous aspects of this process interms of retting duration and fiber quality. However, these machines are currently facing a bottleneck due to loss of usable fiber since pineapple leaf fiber is less fibrous than other fibers and is low energy efficient. Also, low processing efficiency (removal of waxy layer), which is a key parameter for retting and fiber quality.Optimal performance of a fiber extractor can be achieved when it is operated under optimal conditions. There is a lack of detailed analysis of these parameters in the studies mentioned above.Therefore, pineapple growers need mechanical extractors that reduce fiber loss and remove waxy layer more effectively. Hence, the research work was carried out to develop a pineapple leaf extractor that will aid in the retting of pineapple leaves and the production of quality fiber. Further, its performance was evaluated, operational parameters were optimized and then the results were compared with the conventional method of extraction.
Materials and methods
Morphological study of pineapple leaf
For designing of extracting unit of the pineapple leaf fiber extractor, a morphological study was carried out on five hundred pineapple leaves of locally grown (West Bengal) “queen” variety and their values were considered. Morphological parameters of leaf include weight, thickness, thickness of waxy layer, length and width at three positions (basal, middle and tip) were measured. Length and width were measured using scale of 1000 mm and 300 mm. The thickness of leaf and waxy layer was measured using a digital vernier caliper (Model: Mitutoyo, least count: 0.01 mm). In order to measure the thickness of the waxy layer, it was manually removed with the help of a sharp knife and its thickness was measured. The weight of leaves was measured by digital weighing balance (Model: F100,Avery Accuracy: ±1 g). The moisture content of fresh leaves was measured using the standard method as described in ASTM (Citation1992), in which sample was made into small pieces and placed into the oven and dried at 105°C for 24 hours.
Design consideration for pineapple leaf extractor
Before developing the extractor, based on the requirement of stakeholders, following factors were considered in the design i.e., a) lightweight, easy to construct, and powered by single phase motor; b) simultaneous processing of three to four leaves; c) processing of leaves with the combination of scratching and combing action; and d) provision for adjustment of different type and size of pineapple leaf. The extraction process involves the removal of the waxy layer and the combing of scratched material. As the pineapple leaf is positioned between an L-shaped blade on the scratching roller and a breast plate, the scratching roller systematically beats the leaf at closely spaced intervals, effectively stripping away the waxy layer (). The material clinging to the leaf after passing through the scratching roller is then eliminated by the pins on the combing roller.
To effectively remove the waxy layer from the pineapple leaves, it is essential for the scratching roller to rotate at the recommended speed, enabling the L-shaped blade’s tip to generate angular momentum. The operational principle of pineapple leaf fiber extractor is similar to sisal/banana fiber extractor, and calculation of angular momentum was carried out using Equationequation 1(1)
(1) .
Where L= angular momentum (kg. m2/s), ms=mass of scratching roller (kg), vb=linear velocity of blade tip (m/s), rs = radius of the scraping roller from origin to blade tip (m).
One of the important performances of indices of the extractor is amount and extent of waxy removed from the pineapple leaf. In this investigation, the degree of waxy removal was quantified as processing efficiency, with higher processing efficiency correlating to improved retting and fiber quality. Two key design factors influencing the amount of material scraped are the length of the scrapping cylinder and the pitch of the blades employed. The length of scrapping roller using Equationequation 2(2)
(2) as suggested by Shrivastava et al., (Citation2023) and Snyder et al. (Citation2006).
Where, LS = Length of scrapping roller (m), qm = material feed rate (kg/s), t = thickness of the pineapple leaf (m), η = coefficient of cylinder length utilization (0.7–0.9), ρ = bulk density of pineapple leaf and vp = velocity of plant mass entering (05–1 m/s assumed).
The pitch indicates the quantity of leaf material that is extracted as a scraping blade interacts with pineapple leaves. The following formula as suggested by Snyder et al. (Citation2006) was used to determine.
Where, LP = Pitch of scrapping roller blade (m), vp = Velocity of leaf (m/s), L= Angular momentum (rad/s), N= Number of blades on the periphery of scrapping roller.
The diameter of the scrapping roller plays an important role in processing efficiency. The following formula suggested by Snyder et al. (Citation2006) was used to calculate diameter.
Where, VC =Peripheral speed of scrapping roller (m/s), NC = Rotational speed of scrapping roller (rpm).
The number of blades and width of blades were decided based on the morphological characteristics of the leaves. Further, the height and working zone of the machine were decided based on the anthropometric data of both men and women of west Bengal as recommended by Shambhu et al. (Citation2023).
Development of pineapple leaf extractor
Based on the theoretical design of the decorticator, a 3D model of the pineapple leaf extractor was developed using Creo Parametric software (). The prototype comprises feeding conveyor, feed rollers, scratching roller, combing roller, frame and motor as a power source with a transmission unit (). A canvas feeding conveyor placed before the feed rollers with a length of 800 mm and a width of 300 mm so that leaves are directly fed to them. The feed rollers with a diameter of 320 mm and a length of 300 mm are fabricated by M.S. sheet of 4 mm with shaft of 25 mm and periphery of the rollers was covered with rubber 30 mm. There is a provision to adjust gap between two rollers. The main function of the feed rollers is to flatten the trough shaped pineapple leaf and provide constant rate of feeding to scratching roller. To remove the waxy layer on leaf, scratching roller having diameter of 400 mm with 10 L-sharp blades fastened on the periphery of roller made from mild steel provided. The function of combing roller, having diameter of 400 mm with pins on the periphery of the roller is to comb the waxy layer on leaves that has been scathed. Underneath the scratching and combing, breastplate made from MS sheet of 3 mm are provided and gap can be adjusted. One horse power single phase electric motor having rated rpm 1400 was selected as prime mover based on the power requirement to process leaves. Through belts and pulleys, power was transmitted to all the moving components of the machine. To support all the components, frame of the machine with an overall dimension of 1500 × 1100 × 700 mm was fabricated using ASTM A36 mild steel.
Evaluation of the developed pineapple leaf extractor
In order to select operational parameters, preliminary tests were conducted on the developed pineapple leaf extractor. The test revealed that, with a speed higher than 200 rpm, more short fibers were observed, while if the speed was lower than 100 rpm, low processing efficiency was observed. Hence, the speed of scratching roller was varied from 100 to 200 rpm by changing the transmission ratio (by changing belt and pulley). The role of providing a secondary roller was to comb the waxy layer on leaves. It was observed that secondary roller also played an important role in producing the rich quality of fiber. Therefore, three kinds of secondary roller i.e., combing roller, serration and without secondary roller were selected. To vary the moisture content, harvested leaves were allowed to dry in the field for three days (78 ± 3%) and five days (71 ± 2%). Freshly harvested leaves having moisture content of 85 ± 3% were processed in the machine.
Performance of the developed pineapple leaf extractor was assessed in terms of three independent parameters, such as speed of the scratching roller (100, 150 and 200 rpm), type of secondary roller (combing, serration and without secondary roller (WOSR)), and moisture content (85 ± 3, 78 ± 3 and 71 ± 2%) of the leaf and for the response parameters like fiber yield (%), processing efficiency (%) and retting duration (days) were observed.
Retting duration (days)
The scratched pineapple leaves were placed in a plastic tray with bacteria such as Bacillus cereus TNA-2 and Bacillus cereus TNA-15 having high petinolytic and xylanolytic enzyme activity. The bacterial inoculum of both the bacteria in a ratio of 1:1 was prepared and 250 ml/5 liter of retting liquorwas added (). The pectin and xylan present in pineapple leaves act as cementing material in between the fibers and also provide roughness to fiber with helps TNA-2 and TNA-15 bacterial inoculation these cementing material get hydrolyzed and pineapple get loosened from biomass and fiber is easily separated from leaves. The pineapple leaves were regularly monitored to determine the proper retting of pineapple and timely washing of retting pineapple leaves.
Fibre yield (%)
To calculate the fiber yield, the processed leaves were retted with bacterial culture, after that retted leaves were cleaned and sun dried till moisture content reached to less than 10% (Gebino and Muhammed Citation2018). The fiber in percentage was calculated by using following equation
Where: = weight of dried fiber obtained, g and;
= weight of the fresh pineapple leaf, g.
Processing efficiency (%)
The processing efficiency of the machine indicates the extent of waxy layer removed. It was expressed in terms of percentage and calculated using Equationequation 6(6)
(6) .
Where: = Weight of processed leaf-weight of waxy layer remain, g;
= weight of the fresh pineapple leaf, g; and
= weight of the basal portion, g.
Once the operational parameters were optimized, its comparative evaluation with conventional method of extraction was carried out. In conventional method, harvested pineapple leaf are immersed in the water and fiber extracted by manual scrapping method using ceramic plate followed by drying. The quality parameters of the extracted fiber from both methods i.e., fiber bundle strength (gm/tex), fineness (Tex) and color (%) were tested using bundle strength tester, air flow fineness tester and color meter, respectively. Five samples for each parameter were tested in both bundle strength tester and air flow fineness tester, while color values of the fibers were measured at five different locations. The fiber testing was conducted in a laboratory with humidity and temperature set at 65 ± 2 and 27 ± 2, respectively.
Furthermore, to analyze the shape and morphology of the extracted fibers, scanning electron microscopy (SEM) was performed using the SEM-Philips XL-30SEM in the Netherlands. The analysis was conducted at 500× magnification with an acceleration voltage of 10 KV.
Statistical analysis
Symmetrical three-factorial analysis was conducted in Stat-Ease Design Expert V 13.0.0 to analyze the significance of effects of different independent variables on response parameters. Numerical optimization was carried out to identify the optimum condition which results in faster retting duration, high processing efficiency and fiber yield. Duncan’s Multiple Range (DMRT) test (using IBM® SPSS® Statistics 25.0) was also applied to observe the difference in performance between conventional and developed machine.
Artificial neural network
To predict performance parameters, artificial neural network(ANN) model was also employed along with the factorial analysis. In this study, feed-forward back propagation neural network was utilized to predict the performance of pineapple leaf extractor in termsof retting duration, processing efficiency and fiber yield. In the model, network architecture using three input nodes, three hidden layers, and three output nodes was established (). The data set was trained using the levernberg-marquardt back propagation method. Experimental data consists of 27 runs that were replicated three times to obtain 81 training, testing, and validation runs. For the entire modeling process Matlab 2020a software was used.
Results and discussion
Morphological parameters of pineapple leaf
The morphological results of 500 samples of pineapple leaves are presented in . The length of leaves was found in the range of 95 cm to 120 cm whereas width of leaves varied from 4 to 4.62 cm, 4.8 to 5.5 cm, and 1.5 to 2.7 cm at the base, middle, and top, respectively. Thickness of the leaves ranged from 0.18 cm to 0.27 cm in the middle portion. Based on leaf thickness, gap between breast plate and scratching roller, combing roller was set. Further gaps between feed rollers are set. Based on the width of leaf, feed rate (No. of leaves) is maintained. For the present study, three leaves are fed simultaneously.
Table 1. Morphological results of pineapple leaf.
Retting duration
The processed leaf from the extractor was immersed in water with pectinolytic and xylanolytic bacterial culture for retting and the results of retting duration are presented in and its corresponding ANOVA is presented in . The slowest retting period was observed for 100 rpm scratching roller, 71 ± 2 moisture content of leaf and serration type of secondary roller whereas fastest retting was observed for 200 rpm, 85 ± 3% MC and combing type of secondary roller. It was observed from the , that the retting period decreased with an increase in scratching roller speed whereas it increased with a decrease in moisture content. The ANOVA results revealed that amongst the three independent parameters and their interaction, except speed and moisture content, none had a significant effect on retting duration (p > .0001). The relation between retting time and speed of the scratching roller was inversely proportional. With the increment of speed from 100 rpm to 200 rpm, retting duration was faster as when the speed increased, blades progressively beat the leaf at closely spaced intervals and chips off the waxy layer resulting in higher bacterial attack and led tofast retting. Further, under higher scratching speed, all biochemical components of leaves were easily leached to water and available for bacteria which leads to faster multiplication of inoculated bacteria. Hence, it leads to faster retting under high range of speed with higher moisture level. Similar observations are reported by Baniket al. (Citation2011) for pineapple leaf fiber extracted leaf. Compared to different types of secondary roller, in case of combing and serration roller, leaf gets disturbed and waxy layer removed which helps increasing of surface and penetration of inoculated bacteria resulting in faster retting as compared to WOSR.
Table 2. ANOVA of response parameters.
The regression results of ANN model for retting duration using Levernberg-Marquardt back propagation method is shown in . The high degree of correlation coefficients (R) for training, validation, test, and all are 0.9486, 0.87678, 0.83409, and 0.91537, respectively between observed and predicted values. It also implies that developed model could explain the at least 91% of overall variability of response variable.
Processing efficiency
Effect of operational parameters i.e., rpm of scratching roller, type of secondary roller and moisture content of leaf on processing efficiency are shown in and corresponding ANOVA is presented in . The highest processing efficiency (93.1%) was obtained with the combination of 200 rpm of scratching roller, combing type of secondary roller and MC of 85 ± 3%. The lowest processing efficiency was found to be 35.9% for 100 rpm scratching roller with no secondary roller at MC of 71 ± 2%. The processed leaf under different types of secondary roller is shown in . According to , processing efficiency increased with increasing speed of scratching roller, while it decreased with decrease of moisture content of leaf and changingof secondary roller from combing roller to WOSR. Naik and Kar (Citation2020) observed similar phenomenon while extracting sisal fiber from a decorticator. Increase of processing efficiency with increased rpm of scratching roller may be attributed to better removal of waxy layer on pineapple leaf. Moisture content of leaf plays important role in extracting fiber from leaf. The waxy layer on dried leaves is difficult to remove, and chipping it off takes more energy. This indicates that for better processing efficiency, the fiber extraction must be carried out immediately after the harvest of pineapple leave.The ANOVA results revealed that independent parameters with their interactions had significant effect on processing efficiency at 95% confidence level. The F-value of the model (1562.99) that model is significant and there are only 0.01% chance that this value could large due to noise.
Figure 9. Processed leaf under three different types of secondary roller(a. combing roller b. serration roller c. WOSR).
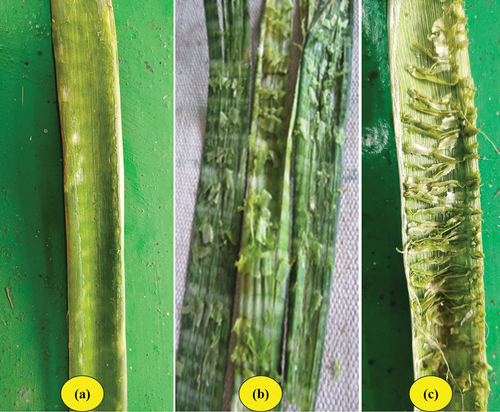
presents results of regression analysis using ANN Levernberg-Marquardt back propagation method for processing efficiency. There is a strong positive correlation between the independent variables and dependent parameter processing efficiency, which is 0.65883, 0.74523, 0.8727, and 0.67281 in training, validation, and test, respectively. Moreover, it indicates that the developed artificial neural network model could explain 65% and 87% of the variability in the measured processing efficiency across training and test datasets (Pareek et al. Citation2021).
Fibre yield
Results of fiber yield obtained after retting of processed leaf using bacteria culture are shown in . The highest fiber yield (2.81%) was observed for 150 rpm of scratching roller, 78 ± 3% MC and without secondary roller while lowest fiber yield (1.6%) observed for combing type of roller at 100 rpm of scratching roller with 85 ± 3% MC. In the figure, it is evident that with an increase in roller speed from 100 to 150 rpm, the field yield increased, and with an increase from 150 to 200 rpm, it decreased. This can be attributed to loss of fiber with waxy layer. Similar observation was reported by Vishnu et al. (Citation2023) for multifibre extractor. Among the independent parameter rpm of scratching roller had significant effect on fiber yield followed by MC and type of secondary roller. The interaction between rpm of scratching roller and type of secondary roller did not significantly affected the fiber yield (p > .001) (). Type of roller had significant effect on fiber yield (p < .001). Among type of secondary rollers, fiber loss was more in serration type of roller due to maceration and breaking of leaf surface.
shows the results of regression analysis of ANN for fiber yield. There was a small variability between target and output values, indicating that the network was reliable in predicting fiber yield. The coefficient of correlation values of training, validation, test and all are 0.99091, 0.99872, 0.98747 and 0.9907, respectively. A high R value in various phases of the ANN model also indicates a strong correlation between the experimental and model-predicted fiber yield.
Optimization of operational parameters
Numerical method was employed to optimize the operational parameters of pineapple leaf extractor and the goal set with importance are given in . Minimizing retting duration, maximizing processing efficiency and fiber yield were objective functions that were set to achieve targeted goal. Results of the optimized combination are shown in . The optimized combination was found to be 150 rpm of scratching roller, combing type secondary roller and with MC of 85 ± 3%. At optimized parameter, retting duration, processing efficiency and fiber yield were 4 days, 88.9% and 2.41% with desirability of 0.802. After setting the optimized parameters, performance of the developed prototype was again tested and retting duration, processing efficiency and fiber yield were observed as 4, 89.20% and 2.53% respectively. Further, throughput capacity of prototype, waste generated during extraction and power required were measured and observed as 33 ± 2 kg/h, 2 kg/h and 1.5 kWh respectively.
Table 3. Constraints for optimization.
Comparative evaluation of pineapple leaf extractor with conventional method
shows the fiber yield, retting time and extraction capacity under traditional method of fiber extraction and pineapple leaf extractors. The pineapple leaf extractor took four days on average to ret the leaves compared to traditional method. This may be because of the absence of a waxy layer and the action of bacteria, Similar results were observed by Nayak et al. (Citation2016) for machine extracted pineapple leaf. The dry fiber extraction capacity (7.68 kg/h) was found to be four times higher than the conventional extraction method (1.86 kg/h). It can be seen from the table that there is a significant difference in fiber yield between conventional and pineapple leaf fiber extractor. The conventional method yielded higher fiber output compared to the pineapple leaf fiber extractor, consistent with findings reported by Rafiqah et al. (Citation2020).
Table 4. Fibre yield, retting time and extraction capacity under different methods.
After retting, fiber quality was also assessed and the results are presented in . The conventional method produced fibers with a higher bundle strength than the fiber extracted after processed in pineapple leaf extractor. As compared to the conventional method, pineapple leaf fiber extracted fiber had a brighter color. Furthermore, it produced finer fiber than conventional method. Overall, fiber quality from developed fiber extractor machine was superior to the previously reported pineapple leaf scratching machine by Banik et al. (Citation2011) where the bundle strength was 9.6 gm/tex and the fineness was 5.7 tex.
Table 5. Fibre quality under different treatment.
From , it was observed that conventional method of extracted pineapple fiber has shown rough structure with the presence of nodes throughout the structure. It is also visible that depth of up and down plane inside the structure was comparatively more as compared to the microbial treated fiber surface. Moreover, machine extracted fiber with microbial treated surface is comparatively smoother and less number of rough nodes are present throughout the structure. Further, reduced diameter of fiber suggests that finer fibers
Conclusion
The present study was aimed at developing pineapple leaf fiber extractor to remove waxy layer of the leaf. An evaluation was conducted under various operational parameters and optimization was carried out using design expert software. The study found that moisture content and rpm of scratching roller had significant effects on retting duration among the independent variables. The processing efficiency was varied from 35.9% to 93.1% under selected parameters. Among chosen independent variables rpm of scratching roller had significant effect on fiber yield followed by MC and type of secondary roller. Results of feed-forward back propagation neural network (ANN) indicate that input variables had strong correlation with dependent parameters. Numerical method was employed to optimize the operational parameters of pineapple leaf extractor and optimized combination was found to be 150 rpm of scratching roller, combing type secondary roller and with MC of 85 ± 3%. At optimized parameters, retting duration, processing efficiency and fiber yield were found to be 4 days, 88.9% and 2.41%. Compared with conventional methods, the developed pineapple leaf extractor reduced retting duration, increased extraction capacity and improved fiber quality. The SEM revealed show that the conventional method of extracted fiber had nodes throughout the structure and machine extracted fiber exhibited a smoother and less number of rough nodes. The extracted fiber can be used to manufacture of fabric and other diversified products. The waste generated during extraction and after retting can be used to make vermicomposting and crop mulching. The developed machine not only provides additional income to growers but also ease the distress of waste discarding. Total cost incurred to fabricate pineapple leaf fiber extractor was $ 1684.With minor modifications, it can also be adapted for processing other leaf fiber plant like sisal.
Highlights
Design and development of Mechanical Extractor for Utilization of Pineapple Leaf Agro-Waste for Textile Application.
Performance evaluation of the developed machine interms of retting duration, processing efficiency and fiber yield.
Retting of processed leaf with bacteria such as Bacillus cereus TNA-2 and Bacillus cereus TNA-15.
Statistical analysis using three factorial analysis and ANN model.
Disclosure statement
No potential conflict of interest was reported by the author(s).
References
- Agricultural Statistics at a Glance. 2022. Government of India, Ministry of Agriculture & Farmers Welfare, Department of Agriculture & Farmers Welfare. Economics & Statistics Division. https://agricoop.gov.in/Documents/CWWGDATA/Agricultural_Statistics_at_a_Glance_2022_0.pdf.
- ASTM, A. 1992. Standard Test Methods for Direct Moisture Content Measurement of Wood and Wood-Base Materials. West Conshohocken, PA: ASTM.
- Banik, S., D. Nag, and S. Debnath. 2011. “Utilization of Pineapple Leaf Agro-Waste for Extraction of Fibre and the Residual Biomass for Vermicomposting.” Indian Journal of Fibre & Textile Research 36 (2): 172–17.
- Das, P. K., D. Nag, S. Debnath, and L. K. Nayak. 2010. “Machinery for Extraction and Traditional Spinning of Plant Fibres.” Indian Journal of Traditional Knowledge 9 (2): 386–393.
- FAO. 2019. FAOSTAT. Food and Agricultural Commodities Production. Rome: Food and Agricultural Organization of the United Nations-FAO.
- Gebino, G., and N. Muhammed. 2018. “Extraction and Characterization of Ethiopian Pineapple Leaf Fiber.” Current Trends in Fashion Technology & Textile Engineering 4 (4): 77–83.
- Jalil, M. A., M. Moniruzzaman, M. S. Parvez, A. Siddika, M. A. Gafur, M. R. Repon, and M. T. Hossain. 2021. “A Novel Approach for Pineapple Leaf Fiber Processing As an Ultimate Fiber Using Existing Machines.” Heliyon 7 (8): e07861. https://doi.org/10.1016/j.heliyon.2021.e07861.
- Kengkhetkit, N., and T. Amornsakchai. 2012. “Utilisation of Pineapple Leaf Waste for Plastic Reinforcement: 1. A Novel Extraction Method for Short Pineapple Leaf Fiber.” Industrial Crops and Products 40:55–61. https://doi.org/10.1016/j.indcrop.2012.02.037.
- Naik, R. K., and G. Kar. 2020. “Development and Testing of a Portable Sisal Fibre Extractor for Small Scale Growers.” International Journal of Current Microbiology and Applied Sciences 9 (10): 2467–2472. https://doi.org/10.20546/ijcmas.2020.910.295.
- Nayak, L. K., D. Nag, and S. Banik. 2016. “Extraction, Characterization and Application of Pineapple Leaf Fibre.” Journal of the Indian Society of Coastal Agricultural Research 34 (1): 77–80.
- Pareek, C. M., V. K. Tewari, R. Machavaram, and B. Nare. 2021. “Optimizing the Seed-Cell Filling Performance of an Inclined Plate Seed Metering Device Using Integrated ANN-PSO Approach.” Artificial Intelligence in Agriculture 5:1–12. https://doi.org/10.1016/j.aiia.2020.11.002.
- Rafiqah, A., K. Abdan, M. Nasir, and M. Asim. 2020. “Effect of Extraction on the Mechanical, Physical and Biological Properties of Pineapple Leaf Fibres.” In Pineapple Leaf Fibers. Green Energy and Technology, edited by M. Jawaid, M. Asim, P. Tahir, and M. Nasir, 41–54. Springer, Singapore. https://doi.org/10.1007/978-981-15-1416-6_3.
- Saini, R., C. W. Chen, A. K. Patel, J. K. Saini, C. D. Dong, and R. R. Singhania. 2022. “Valorization of Pineapple Leaves Waste for the Production of Bioethanol.” Bioengineering 9 (10): 557. https://doi.org/10.3390/bioengineering9100557.
- Shambhu, V. B., P. Shrivastava, T. Nageshkumar, M. Jagadale, L. K. Nayak, and D. B. Shakyawar. 2023. “Development of Gender-Friendly Power Ribboner for Extraction of Green Ribbon/Bast from Jute Plants.” Journal of Natural Fibers 20 (2): 2250076. https://doi.org/10.1080/15440478.2023.2250076.
- Shrivastava, P., T. Nageshkumar, M. Jagadale, V. B. Shambhu, and L. K. Nayak. 2023. “Design and Simulation of Banana Pseudo-Stem Fibre Extracting Raspador (Cylinder) Using FEA Technique.” Journal of Natural Fibers 20 (2): 2200986. https://doi.org/10.1080/15440478.2023.2200986.
- Snyder, B. J., J. Bussard, J. Dolak, and T. Weiser. 2006. “A Portable Sisal Decorticator for Kenyan Farmers.” International Journal Service Learning English 2 (1): 92–116. https://doi.org/10.24908/ijsle.v1i2.2087.
- Vishnu, V., M. P. Harikrishnan, A. S. Warrier, N. K. Mahanti, M. Basil, T. Venkatesh, R. Pandiselvam, and A. Kothakota. 2023. “Design Consideration and Optimization of Process Parameters in Fiber Extraction Unit via Modelling Studies.” Journal of Food Process Engineering 46 (4): e14298. https://doi.org/10.1111/jfpe.14298.
- Yahya, S. A. 2016. “Characterizations of Malaysia PALF Properties Between Hand Scrapping and PALF M1.” Doctoral dissertation, Universiti Tun Hussein Onn Malaysia.
- Yusof, Y., S. A. Yahya, and A. Adam. 2015. “Novel Technology for Sustainable Pineapple Leaf Fibers Productions.” Procedia CIRP 26:756–760. https://doi.org/10.1016/j.procir.2014.07.160.
- Yusof, Y., S. A. Yahya, and A. Adam. 2016. “Pineapple Leaf Fibre Extractions: Comparison Between PALF M1 and Hand Scrapping.” ARPN Journal of Engineering Applied Science 11 (3): 2125–2129.
- Zhang, J., L. Mingfu, O. Zhongqing, and Y. Xinmao. 2002. “Pineapple fibre scraping machine.” CN2474549Y. https://patents.google.com/patent/CN2474549Y/en.