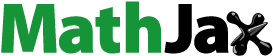
ABSTRACT
Using a multivariate drought index that incorporates important environmental variables and is suitable for a specific geographical region is essential to fully understanding the pattern and impacts of drought severity. This study applied feature scaling algorithms to MODIS time-series imagery to develop an integrated Multivariate Drought Index (iMDI). The iMDI incorporates the vegetation condition index (VCI), the temperature condition index (TCI), and the evaporative stress index (ESI). The 54,474 km2 Vietnamese Central Highlands region, which has been significantly affected by drought severity for several decades, was selected as a test site to assess the feasibility of the iMDI. Spearman correlation between the iMDI and other commonly used spectral drought indices (i.e. the Drought Severity Index (DSI–12) and the annual Vegetation Health Index (VHI–12)) and ground-based drought indices (i.e. the Standardized Precipitation Index (SPI–12) and the Reconnaissance Drought Index (RDI–12)) was employed to evaluate performance of the proposed drought index. Pixel-based linear regression together with clustering models of the iMDI time-series was applied to characterize the spatiotemporal pattern of drought from 2001 to 2020. In addition, a persistent area of LULC types (i.e. forests, croplands, and shrubland) during the 2001–2020 period was used to understand drought variation in relation to LULC. Results suggested that the iMDI outperformed the other spectral drought indices (r > 0.6; p < 0.005). The analysis revealed an increase in drought risk in some provinces of the Central Highlands including Gia Lai, Kon Tum, and Dak Lak. It was also found that changes in LULC patterns could minimize (reforestation) or exacerbate (deforestation) the impacts of drought. Our study suggests that applying a multivariate drought index enables a better understanding of drought patterns at the local scale. This provides valuable information for the development of appropriate land and environmental management practices that can affect and mitigate climate change effects.
1. Introduction
Drought is a natural disaster, which can cause serious impacts on human health and well-being, economy, and the environment (Sherval, Askew, and McGuirk Citation2014; NDMC Citation2022; WMO Citation2022). The phenomenon occurs when rainfall is lower than average over an extended period (WMO Citation2016). This process results in significant water shortages, including the supply of surface water and groundwater. Drought severity has manifold impacts on the environment and socio-economic development in more than 130 countries worldwide (Son et al. Citation2021; UNDRR Citation2021). The United Nations estimated that about 55 million people worldwide are affected by severe drought and predicted worsening droughts could displace up to 700 million people by 2030 (UNDRR Citation2021). They also reported that the potential impact of drought on people’s livelihoods might be comparable with the damage caused by the COVID-19 pandemic (UNDRR Citation2021). Of those negative effects of drought, agricultural production, especially crop and livestock sectors, are the most affected groups. For instance, loss of agricultural productivity originated from drought occupies almost 86% of the total impacts of climate-related disasters (Wu, Ma, and Yan Citation2020; FAO, F. and A.O. of the U.N. Citation2021). Hence, understanding drought severity is valuable for the management of drought-related practice.
Drought can be classified into different types, subject to the causes and effects of drought, geographical regions, or field of study (WMO and GWP Citation2016; West, Quinn, and Horswell Citation2019; Wu, Ma, and Yan Citation2020). Thornthwaite (Citation1947), for example, categorized droughts into four types: permanent (water demand is always higher than supplied precipitation), seasonal (rainy and dry), contingent (irregular and changeable rainfall), and invisible (occurs at any time). Wilhite and Glantz (Citation1985), on the other hand, classified drought into four groups: meteorological drought, hydrological drought, agricultural drought, and socio-economic drought. In addition, several studies suggested other types of drought such as ecological drought (Crausbay et al. Citation2017; Bradford et al. Citation2020) and flash drought (Christian et al. Citation2019; Hu et al. Citation2021). In practice, all drought types are connected to each other and it is hard to identify the onset and end of each drought type (West, Quinn, and Horswell Citation2019; Son et al. Citation2021; Ha et al. Citation2022). For instance, a decline in precipitation over time is the primary factor affecting meteorological drought. If the precipitation deficiencies persist, the stream flow, lake, and groundwater levels will be reduced, which will lead to hydrological drought. Soil moisture deficits are caused by low precipitation and water supply and are associated with agricultural drought. Finally, a socioeconomic drought occurs when water demand for socioeconomic activity exceeds water supply because of a weather-related deficit, including the impacts of meteorological, agricultural, or hydrological droughts (Elhag and Zhang Citation2018).
Conventionally, ground meteorological data were collected to monitor and predict drought events. However, these ground-based stations are often sparsely distributed making regional drought monitoring challenging (Jiao, Wang, and McCabe Citation2021; Ha et al. Citation2022). In the recent years, remote sensing has been heavily utilized in predicting drought severity improving the drawbacks of traditional approaches in relation to cost, time, and spatial distribution (West, Quinn, and Horswell Citation2019; Jiao, Wang, and McCabe Citation2021). Remote sensing that is capable of providing multiple spatial and temporal resolution of data acquiring from various sensors at different spectral regions (wavelengths), has significantly contributed to drought studies over the previous several decades (West, Quinn, and Horswell Citation2019; Jiao, Wang, and McCabe Citation2021). Remotely sensed products of drought (mostly using drought indices) have commonly utilized information of land surface temperature (LST), rainfall, vegetation greenness, evapotranspiration (ET), and soil moisture (WMO and GWP Citation2016; Jiao, Wang, and McCabe Citation2021) [see comprehensive reviews by Jiao, Wang, and McCabe (Citation2021), Mu et al. (Citation2013), West, Quinn, and Horswell (Citation2019), Wu, Ma, and Yan (Citation2020) and Yu et al. (Citation2019)]. According to these studies, each remotely sensed drought index is generated to investigate a specific type of drought based on its source, which may be utilized as input elements. For other types of drought, meteorological drought often utilizes climatic data (e.g. precipitation, air temperature) (Spinoni et al. Citation2019; Yu et al. Citation2019), whereas hydrological drought employs streamflow or groundwater data (Myronidis et al. Citation2018; Jiao, Wang, and McCabe Citation2021). Furthermore, vegetation condition or evapotranspiration are commonly used to characterize drought patterns in agricultural drought studies (West, Quinn, and Horswell Citation2019; Sun et al. Citation2020). Although drought indices have been produced for several decades, the majority of them are region-specific, and so these indices may not be relevant to covering various geographical settings and environmental conditions such as climate zones, land cover types, and biome types (Hao and AghaKouchak Citation2013; Son et al. Citation2021).
Several integrated drought indices have been developed to better monitor and characterize drought conditions (Hao, Zhang, and Yao Citation2015; Cao et al. Citation2019; Shah and Mishra Citation2020). The Palmer Drought Severity Index (PDSI) may be the first multivariate indicator that combined precipitation, temperature, and soil moisture data to detect agricultural drought (defined as soil moisture deficit) patterns (Guttman Citation1998; Mu et al. Citation2013), but its parameters heavily depend on regional geographical features (Guttman Citation1998; Paulo and Pereira Citation2006; Yu et al. Citation2019). Kogan (Citation1995) proposed Vegetation Health Index (VHI) that combines Vegetation Condition Index (VCI) and Temperature Condition Index (TCI) to examine the dryness condition associated with the water-energy balance of the land surface. However, utilizing VHI to examine drought conditions may not be appropriate due to the difference in spatial distribution, contribution, and intensity of moisture and temperature to vegetation phenology, which are major factors influencing the weight of VCI and TCI (Karnieli et al. Citation2006; Bento et al. Citation2020; Sun et al. Citation2020). Additionally, VHI does not directly respond to the evapotranspiration process, which is one of the major factors influencing drought conditions on cultivated land in semi-arid areas (Bento et al. Citation2020; Zeng et al. Citation2022). Mu et al. (Citation2013) developed the global-scale Drought Severity Index (DSI), a well-known index, using standardized combinations of the MOD16 ET/PET (Evapotranspiration/potential ET) and the MOD13 NDVI (the Normalized Difference Vegetation Index) products of MODIS (the Moderate Resolution Imaging Spectroradiometer) to monitor drought conditions. The ratio of actual ET and PET behaved similarly to precipitation-based indices (Anderson et al. Citation2011) using evaporation from canopy interception,Footnote1 wet and moist soil surfaces, and transpiration from canopy stomata (Mu et al. Citation2007; Mu, Zhao, and Running Citation2011; Mu et al. Citation2013). However, DSI does not consider the use of land surface temperature (LST) which may lead to missed information of the land surface balance of water, energy, and carbon dioxide. To fill this gap, Tran et al., (Citation2019) added LST to Enhanced Drought Severity Index (EDSI) and applied it to monitor drought at regional scale of the Mekong River Delta. In this study, we integrated additional potentially related factors such as vegetation, temperature, and evapotranspiration to better quantify and understand drought patterns.
The use of time series remotely sensed data may provide an additional temporal dimension to further decipher the complex drought pattern (Frazier et al. Citation2018; Jönsson et al. Citation2018; Ghil et al. Citation2002). However, the differences in ranges of features (i.e. the difference between the lowest and highest values) within an input time-series dataset are relatively high and, in most cases, data used to develop drought indices are measured in different units (e.g. millimeter versus degree Celsius) (Heo and FitzHugh Citation2000; Lokman et al. Citation2019; Zakaria Citation2019). As a result, feature scaling (e.g. data normalization) – a method used to normalize the range of independent variables or features of data – is widely employed as a pre-processing stage to standardize or improve the quality of the input dataset (Heo and FitzHugh Citation2000; Lokman et al. Citation2019). Among several feature scaling techniques that have been employed such as linear regression, logistic regression, or neural networks, normalization and standardization are commonly used methods for drought monitoring and assessment (McKee, Doesken, and Kleist Citation1993; Kogan Citation1995; Rhee, Im, and Carbone Citation2010; Mu et al. Citation2013; Hao, Zhang, and Yao Citation2015; Tran et al. Citation2019). The primary difference between normalization and standardization is that the standardized data follows a Gaussian distribution (Aniruddha Citation2020). Also, in multivariate linear regression, all features need to ensure having similar ranges of values for standardized performance (Dzierżak Citation2019; Wan Citation2019). However, for DSI (Mu et al. Citation2013) and EDSI (Tran et al. Citation2019), there is a difference in range between indicators of NDVI and ET/PET ([−1; 1] and [0;1], respectively), while maximum LST value is not defined. Therefore, applying feature scaling algorithms is necessary to have a similar range before the parameter integration.
Our study aims to develop an integrated multivariate drought index (iMDI) from MODIS data for monitoring agricultural drought. The iMDI employed a combination of vegetation condition, LST, and evapotranspiration based on feature scaling algorithms (i.e. normalization and standardization). The normalized approach helps to homogenize the range of variables between 0 and 1 before combining these parameters (Shumway and Stoffer Citation2017; Aniruddha Citation2020). The standardized method assists in rescaling the integrated values such that they are centered around the mean with a unit standard deviation, as in a normal distribution (Shumway and Stoffer Citation2017; Aniruddha Citation2020). The approach allows filling data gaps in the proposed drought indices of DSI (Mu et al. Citation2013) and EDSI (Tran et al. Citation2019) with respect to variable min – max values. The combination of three previously published drought indices (i.e. VCI, TCI, and ESI) enables us to understand vegetation and moisture conditions in relation to water stress conditions. As such, it will be an effective index for agricultural drought monitoring. The Central Highlands, one of the major agricultural regions of Vietnam, was selected as a case study to demonstrate how the proposed drought index can be applied to quantify the pattern of drought severity over time (i.e. 2001–2020) (Nguyen et al. Citation2016; Dinh et al. Citation2017).
2. Materials and methods
2.1. Study site
The Central Highlands in Vietnam (11°12”00”–15°27”15” N and 107°12–108°59’37” E) consists of five administrative provinces including Kon Tum, Gia Lai, Dak Lak, Dak Nong, and Lam Dong with an area of 54,474 km2 (). It is considered as a “green lung” of Vietnam with a total forested area of nearly 26,000 km2, which is 17.5% of the country’s forested area (Trieu, Pham, and Dao Citation2021). The study region is comprised of several plateaus with elevation ranging from 500 m to 1,500 m. These plateaus are covered by basaltic soils and bordered by high mountain ranges and massifs to the east (known as the South Annamite Range). The study area has a typical tropical monsoon climate with a rainy (May-October) and a dry (November-April) season (). The study region receives 90% of rainfall in the rainy season with a minimum of 100 mm/month. Severe drought events commonly occur in the dry season. Mean annual temperature is about 20 ºC with low seasonality for tropical region. Nevertheless, due to topography, the climate are characterized into three types of sub-regions, which are North Highlands, Central Highlands, and South Highlands () (Toan and Dac Citation1975).
Figure 1. (A) Location of the study area, with (b) elevation, provincial boundaries, and meteorological stations. The source of the digital elevation model is from ASTER Global Digital Elevation Model.

Figure 2. Monthly mean temperature (T, the primary y-axis) and precipitation (P, the secondary y-axis) acquired from five meteorological stations in the study area from 50 years of climate data (1972 –2021) from the National Centre for Hydro-Meteorological Forecasting. Consecutive months receiving 100+ mm of precipitation (above the standard lines) is defined as the rainy season.

Coffee plantation occupies 85% of the cultivated area of the Central Highlands with the remaining crops being rubber (5.1%), pepper (1.4%), and others (8.5%). Coffee plantations in this area account for 90% of the production for the entire Vietnam (GSO Citation2021). The flowering season of coffee trees begins in March requiring sufficient water for producing seeds, but rainfall could only provide 25% of the demand and additional water supply from irrigation is sometimes necessary (Amarasinghe et al. Citation2015). However, deforestation created 20% decline of natural forests between 1990 and 2019 (from 60% to less than 40%) (Chuyen Citation2020; MARD, M. of A. and R.D. Citation2021), leading to an increase in surface runoff, reduction in the infiltration of rainwater, and decrease in the amount of stored groundwater to provide irrigation water for crops in the dry season (Chuyen Citation2020). Consequently, drought has severe impacts on water supply for coffee, rice and other cash crops (Dinh et al. Citation2017; Nguyen et al. Citation2017; Vu, Vu, and Trinh Citation2019). However, the limitation on the number of meteorological stations, where each province only has one station, has created a challenge for broadly monitoring drought in the Highlands. Therefore, to overcome these issues in the region, there is an emerging need for large-scale, long-term, and cost-effective monitoring and mapping techniques that employ remotely sensed data that are available at no or low cost.
2.2. Data preparation
The monthly and annual time-series MODIS LST (MOD11A1), NDVI (MOD09 GA) and ET/PET (MOD16A2) data acquired between 2001 and 2020, were obtained via Google Earth Engine (GEE). GEE, a cloud-based platform, is commonly used to perform deep learning approaches in image analysis with the “JavaScript API (application programming interface) and an online Integrated Development Environment (IDE) code editor” (Gorelick et al. Citation2017). The GEE is a flexible platform which enables users to perform online analysis of satellite images without downloading the images (https://earthengine.google.com/). This platform allows the users to utilize all GEE data and functions to perform high-performance computing analysis to detect changes and monitor trends on the Earth’s surface using big data (e.g. long-term time series satellite images) (Erickson Citation2014; Gorelick et al. Citation2017). In this study, we retrieved the monthly mean, maximum, and minimum values of LST, NDVI, ET, and PET in the 2001–2020 period (). Afterward, all the parameters were exported at a spatial resolution of 1000 m for further processing (see the workflow in ). In addition to the satellite data, the concurrent ground precipitation and temperature data, provided by the National Hydrometeorological Center, was collected from five meteorological stations in the study region () to quantify ground-based annual drought (12-month drought) for consideration of the iMDI performance. Notably, the 500-m annual MODIS Land Cover Type 1 Product (MCD12Q1) for 2000–2019 was utilized to monitor land use and land cover change and access its impacts on drought patterns as anthropogenic factors in the study area (Sulla-Menashe and Friedl Citation2018) (please see description of land classes in ).
Table 1. Description of the land cover classes derived from MODIS product (Sulla-Menashe and Friedl Citation2018).
2.3. Integrated multivariate drought index (iMDI)
In this study, iMDI is proposed as an extension of VHI through an addition of ESI for agricultural drought characterization (see for the workflow of iMDI), and details follow:
1) The VCI (Equationeq. 1(1)
(1) ) was obtained from pixel-based normalization of NDVI (Kogan Citation1995; Elhag and Zhang Citation2018):
where Vmean, Vmax and Vmin are the mean, maximum and minimum NDVI of a period (2001–2020) respectively, and I is the year. This vegetation greenness metric accounts for local differences in ecosystem productivity because the drought implications for vegetation cannot be easily detected from NDVI directly (Kogan Citation1995; Tran et al. Citation2017). VCI assumes that climate variance influences vegetation vigor. Extreme drought may decrease vegetation growth and result in the lowest NDVI in a multi-year observation in certain climate region and season. Conversely, the highest NDVI represents an optimal climatic condition for plant growth. Therefore, VCI is superior to NDVI in drought stress detection (Tran et al. Citation2017; Elhag and Zhang Citation2018). The range of VCI is from 0 to 1, and when there is a drought in a particular year, vegetation vigor is low (low Vi,mean); hence, the numerator of the Equationeq.1(1)
(1) is low (low VCIi).
(2) The TCI (Equationeq. 2(2)
(2) ) is used to assess vegetation stress induced by high temperatures and excessive precipitation (Kogan and Sullivan Citation1993; Kogan Citation1995):
where Tmean, Tmax and Tmin are the mean, maximum and minimum LST of a period (2001–2020) respectively, and i is the year. In relation to the effects on vegetation growth, high LST in the growing season of vegetation presents unfavorable conditions (i.e. drought), whereas low LST mostly shows favorable conditions (Singh, Roy, and Kogan Citation2003). This demonstrates the opposite effect compared to the VCI, which is generated from NDVI. The range of TCI is limited in [0;1] and when there is a drought in a particular year, temperature in a particular year is high and hence TCIi is low.
(3) Drought indices involved in evapotranspiration estimation are mainly based on the ratio of ET/PET, also known as Evaporative Stress Index (the ESI, Equationeq. 3(3)
(3) ):
The ET and PET, directly derived from MODIS ET products, has been demonstrated to be effective in several drought studies (Anderson et al. Citation2011; Mu et al. Citation2013; Dorjsuren, Liou, and Cheng Citation2016) and is rigorously validated with daily ET estimations based on flux tower eddy covariance measurements on a worldwide scale (Jung et al. Citation2010). MODIS ET also includes NASA Soil Moisture Active Passive (SMAP) within its algorithm framework which is effective for agricultural drought monitoring (Running, Mu, and Zhao Citation2019; Brust et al. Citation2021). As such, the ESI (ET/PET) can be used as an approximate proxy for soil moisture which can provide a direct interpretation for a water stress condition. Using other methods such as precipitation data or hydrologic modeling is not efficient as it often requires extensive information (e.g. management strategies and water table distribution). In contrast to precipitation indices, we will likely be able to acquire information on where stress is reduced by active water management or other non-precipitation water inputs (Anderson et al. Citation2007a, Citation2007b, Citation2011). In addition, precipitation and soil moisture data that are available on a global scale are too coarse or uncertain for regional analysis, and thus, were not used for iMDI computation in our study (Mu et al. Citation2013; Kerr et al. Citation2016; Peng et al. Citation2017). The range of ESI is limited in [0;1] and when ESI is low, there is likely to be an association with low soil moisture or dryness, with consequential negative impacts on vegetation.
Lastly, (4) we used mathematical functions to combine these parameters as an integrated index to come up with the proposed index (iMDI) (Equationeq. 4(4)
(4) ) since their values range from 0 to 1 without any unit. Afterward, we standardized iMDI as an anomaly to reduce the dissimilarity with missing data of local mean values (Equationeq. 5
(5)
(5) ).
where and iMDImean are the iMDI’s standard deviation and average, respectively. The
score is dimensionless and varies in
for dryness to wetness conditions, respectively. When there is a drought in a particular year all three indices are low, hence the iMDIi is low. Conditions of drought are shown by values less than zero, while wet conditions are presented by values above zero. Similar to drought indices that were developed in previous studies (e.g. DSI, EDSI, and SPI), the iMDI anomaly is available to detect abnormally wet periods, whereas our study mainly focused on drought detection. To provide a consistent interpretation of the drought assessment, we classified the drought conditions in the study area into five categories that are similar to the classification in the SPI (McKee, Doesken, and Kleist Citation1993) and SDI (Mu et al. Citation2013). Specific threshold values for each category were adjusted to meet the local conditions ().
Table 2. The drought categories based on iMDI dynamic range and relative categories (McKee, Doesken, and Kleist Citation1993; Mu et al. Citation2013; Tran et al. Citation2019).
The combination of VCI, TCI, and ESI, obtained from LST, NDVI, and ET products offer valuable information on drought effects in connection to local conditions. Both VCI and TCI are efficient for early drought warning because these indices incorporate spectral profiles of vegetation, which can reveal vegetation response to the influence of environmental conditions (Seiler, Kogan, and Sullivan Citation1998; Ontel et al. Citation2021; Zeng et al. Citation2022). The ESI enables the moisture content to be detected (Running, Mu, and Zhao Citation2019), and this index is also a valuable supplement that can be incredibly useful in areas where rainfall data is sparse or inconsistent (Anderson et al. Citation2011). Therefore, an integration of three proposed indices provides effective information about vegetation and water content as an efficient indicator of agricultural drought monitoring. Notably, there are two critical aspects to emphasize in this phase: (i) standardization improves minor fluctuations or seasonal variation, and (ii) standardization does not eliminate any existing trend in the time series. Furthermore, it does not change the statistical significance of trends in any sense (de Carvalho Júnior et al. Citation2015; Orvos et al. Citation2015). As only five meteorological stations have been used to observe weather conditions in the Central Highlands across an area of 54,474 km2, remotely sensed data should be applied for drought monitoring.
2.4. Performance assessment
To assess the performance of iMDI, Spearman correlation analyses were conducted to test the correlation between iMDI and the annual field-derived Standardized Precipitation Index (SPI–12) (McKee, Doesken, and Kleist Citation1993) and Reconnaissance Drought Index (RDI–12) (Tsakiris and Vangelis Citation2005; Tsakiris, Pangalou, and Vangelis Citation2007). These indices are calculated from data measured from weather stations and have been widely used to evaluate the performance of spectral drought indices (Cao et al. (Citation2019), Shah and Mishra (Citation2020), and Wu, Ma, and Yan (Citation2020). The annual mean air temperature and rainfall data from five weather stations in the Central Highlands from 2001 to 2020 were used to calculate the ground-based drought indices (i.e. SPI and RDI). Correlation between ground-based indices and some commonly used multivariate drought indices that are VHI (Kogan Citation1995) and DSI (Mu et al. Citation2013) were carried out to evaluate the performance of iMDI compared to other spectral drought indices. Results from the Spearman correlation analysis between remotely sensed drought indices and ground-based drought information from 2001 to 2020 provided a basis to justify the effectiveness of the proposed drought index.
2.4.1. Ground-based drought indices
The SPI (Equationeq.6(6)
(6) ) is based on the standardized approach to rainfall probability over a certain time period (McKee, Doesken, and Kleist Citation1993). It describes the change in precipitation using the joint (
) probability distribution and normalizes the rainfall of skewed probability distribution (McKee, Doesken, and Kleist Citation1993; Tigkas, Vangelis, and Tsakiris Citation2013; Um, Kim, and Park Citation2018). The formula for SPI calculation is as follows:
In Equationequation 6(6)
(6) , c0 = 2.515517; c1 = 0.802853; c2 = 0.010328; d1 = 1.432788; d2 = 0.189269; and d3 = 0.001308 are the calculation parameters,
, where
is the rainfall distribution probability associated to the
function;
presents the precipitation sample; and S indicates the positive and negative probability density coefficient. When
is more than 0.5 and S is equal to 1, or when
is equal and less than 0.5 and S is equal to-1, the probability density integral formula of
distribution function is used to determine
(Equationeq.7
(7)
(7) ):
where, γ and β are the shape and scale parameters, respectively, of the distribution function.
The RDI (Equationeq.8(8)
(8) ) is conceptualized as a water deficit, representing the water balance deficit between input (precipitation) and output (reference evapotranspiration) (Tsakiris and Vangelis Citation2005).
where Rij and PETij are the rainfall and potential evapotranspiration (Equationeq. 8(8)
(8) ) of the j-th month of the i-th year, respectively; k and N is the total number of months and years of the available data, respectively. The PET in the Equationequation 8
(8)
(8) was obtained using Hargreaves equation (Equationeq. 9
(9)
(9) ) (Tigkas, Vangelis, and Tsakiris Citation2013, Citation2015) that requires monthly average geo-datasets of mean temperature (T – mean, degree Celsius), daily temperature range (TD, degree Celsius), and extra-terrestrial radiation (RA, radiation on top of atmosphere expressed in mm/month as equivalent of evaporation). In this study, the annual field drought indices (i.e. SPI–12 and RDI–12) and PET (Equationeq.9
(9)
(9) ) from 2001 to 2020 were measured using DrinC software (Tigkas, Vangelis, and Tsakiris Citation2013, Citation2015).
2.4.2. Remotely sensed drought indices
The VHI (Equationeq. 10(10)
(10) ) (Kogan Citation1995) and DSI (Equationeqs. 11
(11)
(11) and Equation12
(12)
(12) ) (Mu et al. Citation2013) were calculated to provide information for a comparison between iMDI and these spectral drought indices. The equations for these indices are presented as follows:
where α is the weighted coefficient between VCI and TCI. The value of α varies according to moisture and temperature conditions. In the case of uncertain moisture conditions, α is set to 0.5, indicating that VCI and TCI are equally weighted for annual VHI computation (Kogan Citation1995; Tran et al. Citation2017). For the annual DSI,
where Zi is sum of the RT and NDVI and i is the year; RTi and Vi are ET/PET and NDVI, respectively; ,
,
, Zmean, RTmean, and Vmean are the standard deviations and means of Z, RT, and NDVI in the 2001–2020 period, respectively.
2.5. Time-series clustering analysis
A time-series clustering analysis consists of two parts: measuring similarity and performing clustering algorithm (Aghabozorgi, Shirkhorshidi, and Wah Citation2015). Clustering is commonly used in data mining, and details of cluster analyses can be found in Aghabozorgi, Shirkhorshidi, and Wah (Citation2015), Guijo-Rubio et al. (Citation2020), and Zhang et al. (Citation2014). In this study, two commonly utilized clustering algorithms, K-Means and Hierarchical clustering, were applied to group drought datasets of the Central Highlands (Guyet and Nicolas Citation2016). K-Means clustering is defined as an algorithm that “uses the Euclidean distance to separate n observations into k clusters” (Guijo-Rubio et al. Citation2020)(Equationeq. 11(11)
(11) ).
The distance measurements compare time point i of time-series x with the same time point i of time-series y, and thus, both time series must be of equal length (n = 20). The measurement process of K – Means clustering is followed by the steps described below (Grabusts and Borisov Citation2009): (i) choose an iMDI value for K; (ii) initialize the K cluster centers at random (if necessary); (iii) determine the class memberships of the N objects by assigning them to the nearest cluster center or centroid; (iv) re-estimate the K cluster centers’ (means) by assuming the memberships discovered above are valid; (v) exits if the N objects in the previous iteration is at 95% or if some nominated threshold of members remaining stable is exceeded. Otherwise, return to step (iii) to repeat the process.
In addition to the K-Mean clustering, the L-moments based heterogeneity measure was applied to analyze whether the regions might be considered homogeneous. According to Hosking and Wallis (Citation1997), L – moments summarize the properties or geometries of theoretical probability distributions and observed samples. First, these data are examined in order to identify some problems with the homogeneity of the single time series. Then, 500 homogeneous regions with population parameters equal to the regional average sample L – moment ratios were generated using a four-parameter Kappa distribution-based Monte Carlo simulation approach. Finally, the target region’s characteristics were compared with those of the homogenous simulation region. For the sample and simulated regions, the heterogeneity measures H statistic and V statistic can be defined as follows Equationeqs.12(12)
(12) and Equation13
(13)
(13) (Yoo et al. Citation2012):
Where and
are the average and the standard deviation of simulated Vi values, respectively; ni and
are the record length and the sample L-moment at site i, respectively; and
presents the regional averaged sample L-moment. The L-coefficient of variation, the L-skewness, and the L-kurtosis are used as the L-moment in Equationeq. (12)
(12)
(12) to calculate each homogeneity [H(1), H(2), and H(3)], respectively. A region is regarded as acceptably homogeneous if H ≤ 2, possibly heterogeneous if 2 < H ≤ 3, and certainly heterogeneous if H > 3 (Yoo et al. Citation2012).
2.6. Trend analysis
A pixel-based time-series trend analysis of iMDI was performed to estimate the drought spatial variation in the study area from 2001 to 2020. The slope of the linear regression line was calculated using Equationeq. 13(13)
(13) .
where, a is slope of the regression line; iMDIi and Yi represent the drought index as a response variable and year as an explanatory variable, respectively, during the period of 20 years. Afterward, only pixels with values that are statistically significant at the 95% level are investigated using a 2-tailed t-test. Negative values of the slope indicate an environmentally stressed or increasing trend in drought intensity since the drought indices decrease toward negative infinity. Conversely, a slope value greater than 0 suggests a decline in drought level over time. To present the true drought conditions in the study site, we multiplied the resulting drought slope values by minus 1. This enables providing basis information to identify whether each of these measurements has a significant change either positively or negatively (increasing or decreasing trend of drought) over time.
3. Results
3.1. The performance of the iMDI
The results from Spearman correlation analysis between iMDI, DSI, VHI, and in-situ drought measurements (i.e. SPI and RDI) at five weather stations are shown in . Although all spectral indices are significantly correlated with SPI and RDI at the five weather stations (p < 0.005), r–values obtained from iMDI are significantly higher than that of VHI and DSI. For instance, correlation coefficients in iMDI range between 0.16–0.68, while r-values in VHI and DSI are only between 0.10–0.55 and 0.05–0.67, respectively (). This information in addition to the highest r-value indicates the performance of iMDI and therefore, demonstrates the suitability of using this index for the examination of drought condition in the study area.
Table 3. The correlation coefficients between in situ indices and remotely sensed indices upon meteorological stations (p <0.005) during the 2001–2020 period.
3.2. Trend analysis of each cluster
The time-series clustering analysis showed five clusters of drought patterns (i.e. A, B, C, D, and E) in the study area (). The values of heterogeneity measures (H1, H2 and H3) among five clusters were less than 2 () and the probability density boxplot of each cluster showed a normal distribution with the mean value of iMDI centered around zero (). These information demonstrates that grouping drought into five clusters is appropriate to present the drought pattern in the study area (Zhang et al. Citation2014).
Figure 4. (A) the time-series clusters of drought patterns (A, B, C, D, and E) and (b) the distribution of iMDI values for each cluster in the study region. Boxes, whiskers, bar line, and crosses are range, maximum and minimum, median, and mean of iMDI values, respectively.

Table 4. Descriptive statistics for average time-series of the iMDI ().
shows that clusters A and D were mainly concentrated in Gia Lai and Kon Tum provinces. Cluster B was found in Gia Lai and Lam Dong provinces. Whereas clusters C and E were located in the middle and east of the Central Highlands, respectively. Comparing the trend of changes among five clusters ( and ), clusters B, C, and E presented an increase in drought value (slope >0), whereas cluster D showed a decrease trend (slope <0). This indicates that the risk of drought declined in B, C, and E regions, but increased in D region. In cluster A, an insignificant trend was investigated since the p-value was more than 0.1 despite the slope being less than zero.
3.3. Spatiotemporal variations of drought pattern during the 2001–2020 period
The annual patterns showed both decreasing and increasing trends in drought risk, with a dominance of decreasing trend across the study area. However, the change varies across space and season, showing that an increase in drought risk was mainly found in Gia Lai and Kon Tum provinces (). In the early dry season (November–December), an increase in drought risk was observed in the central part of the study area (e.g. a contiguous region between Kon Tum, Dak Lak, and Gia Lai). This trend expanded toward the east part of the study area in the following months and reached the maximum extent in April (the end of the dry season) (). The increasing trend continued in the east region of the Central Highlands, and this reduced significantly in August (). In contrast, the lowest extent of decreasing trend was recorded in the middle of rainy season (August) and the highest value was discovered in December ().
Figure 7. Monthly variation of affected-drought area trend in area extent over the period 2001–2020.

The annual affected area by drought categories between 2001 and 2020 is shown in . It is noted that D0 was not included in the analysis because it is a non-drought category. The temporal change of the iMDI demonstrates that the drought intensity reached a peak in 2004 (Severe Drought) and 2005 (Extreme Drought), which is illustrated by the largest affected area in moderate and above drought categories (D2-D4). The areas influenced by severe and extreme drought (D3-D4) were also high in 2002, 2008, 2011, and 2016. A decrease in drought risk pattern was seen in the periods of 2006–2010 and 2012–2015, and the drought-affected area was the lowest in 2017.
Figure 8. Areal extent by drought categories between 2001 and 2020. Note: D1 = near drought; D2 = moderate drought; D3 = severe drought; D4 = extreme drought.

In general, drought events occur every year, but mostly concentrated in the dry season. The near drought pattern (D1) started from August/September surrounded the South Annamite Range and gradually expanded to other regions in accordance with increasing drought levels (i.e. D2, D3, and D4) in the following months (). The drought intensity tended to be higher and more severe toward the end of the dry season (April). During this time, the drought risk is found to be more severe in the areas between Gia Lai and Dak Lak, south of Kon Tum, Dak Nong, and Lam Dong.
3.4. The pattern of drought variation through land use and land cover
The LULC map shows the dominant cover of forests, shrubs, and croplands, whilst residential areas and water bodies occupy only a small proportion (). From 2001 to 2020, forested land cover (i.e. evergreen, and deciduous broadleaf forests) declined by 684,444 km2 (i.e. 657,478 km2 of evergreen and 26,966 km2 of deciduous), whereas other LULC types that are residence, shrub, and water bodies increased 3,383 km2, 495,020 km2, and 9,406 km2respectively ( and ). In terms of agricultural land, the area of annual croplands increased by 83,451 km2, while perennial croplands decreased 538 km2 in 2000–2010 period but increased by 93,165 km2 in 2011–2019.
Table 5. Areas of LULC in the study area between 2001 and 2019, performed from LULC data in (km2).
Although LULC change occurred in most of LULC types, a large area remained stable over time. To exclude the drought trends that were impacted by LULC change, we focused on the persistent LULC areas where there was not having a conversion from one LULC type to another. An intersection of LULC layers of 20 years was employed to consider the persistent LULC category at a resolution of 1 km2. Five vegetated LULC classes (i.e. evergreen broadleaf forests, deciduous broadleaf forests, annual croplands, perennial croplands, and shrubs) were considered to assess the variations of annual and seasonal drought index by LULC. The change in drought patterns and its relation to LULC between 2001 and 2020 is presented in . Most lines tend to have a negative trend from 2001 to 2006 and then a positive trend afterward. However, an abnormal pattern was found in deciduous broadleaf forests, followed by annual croplands and perennial croplands. The iMDI < − 1.4 (extreme drought, ) was explored in connection to these land uses in 2004 and 2005, while the iMDI >1 was found in 2017. The drought patterns in evergreen broadleaf forest and shrub ranged from −1 and above.
The increasing trend in drought-affected areas in deciduous broadleaf forest types was not explored for all months (), while this trend varied across seasons in evergreen broadleaf forests and shrublands classes (). For the croplands (i.e. perennial and annual), an increase in drought-affected areas was observed in rainy season, mainly concentrated in July and August, while the decreasing pattern was found in the dry season, especially in December ().
Figure 12. The trends in monthly drought area based on persistent LULC classes between 2001 and 2020.

In addition, a decreasing trend was discovered in an area of 8,131 km2 of the evergreen broadleaf forests, followed by shrubs and perennial croplands with a decrease of 7,062 km2 and 1,113 km2, respectively (). Whereas the increasing trend in drought-affected areas was mainly seen in annual croplands (171 km2) and shrubs (1,510 km2). Additionally, the difference between the increased and decreased areas was found to be greater in forests and perennial croplands than that of annual croplands and shrubs.
Table 6. Increasing or decreasing trend in annually affected-drought area (km2) for unchanged land use classification in the study area during 2001–2020 period.
5. Discussion
Various drought indices have been developed to detect drought intensity and severity worldwide (West, Quinn, and Horswell Citation2019; Ha et al. Citation2022). Despite their valuable contributions, the suitability and performance of these indices for regional drought analysis are different and significantly dependent on the climate process, local LULC characteristics, and associated anthropogenic activities (Hao and AghaKouchak Citation2013; Hao, Zhang, and Yao Citation2015). Although the integration of multiple factors such as rainfall, soil moisture and temperature, and vegetation greenness is seen as an effective approach to develop a regional drought index, a comprehensive drought index that considers all these factors is neglected in previous studies (Rhee, Im, and Carbone Citation2010; Yao et al. Citation2010; Anderson et al. Citation2011; Hao, Zhang, and Yao Citation2015; Cao et al. Citation2019; Sun et al. Citation2020). Compared to drought indices that were developed and applied in previous studies, the iMDI developed in our study is seen to be more effective as it integrates more factors such as vegetation, temperature, and evapotranspiration to quantify and understand drought patterns at local scale. An integration of three published drought indices (i.e. VCI, TCI, and ESI), obtained from these environmental factors, enables the vegetation, soil moisture, and water deficit conditions to be taken into account. The information derived from LST and NDVI reflects the vegetation conditions in relation to temperature, while ESI presents moisture status and actual water stress conditions (Kogan Citation1995; Mu et al. Citation2013; Running, Mu, and Zhao Citation2019; Tran et al. Citation2019). The use of normalization algorithms in this study created a standardize measurement (from 0 to 1 scale) for each input factor before combining these to create drought index allows the iMDI to be appropriately used for multivariate linear regression (Wan Citation2019). Notably, using iMDI and time-series analysis methods enable better understanding of the changes in drought intensity in relation to LULC pattern. For example, a significant reduction in natural drought severity was found to be associated with a rapid expansion of perennial croplands ().
In the Central Highlands, a few studies applied remotely sensed data to monitor and assess drought intensity (Dinh et al. Citation2017; Le et al. Citation2021; Thuong et al. Citation2022). Dinh et al. (Citation2017) applied the Normalized Difference Drought Index (NDDI) to examine the drought variation in the past three decades in the study area. The NDDI was calculated using NDVI and NDWI that permits more detailed information to be observed in respect to the drought pattern (i.e. higher spatial resolution) than ground-based drought indices (e.g. SPI). However, the aforementioned study only focused on drought analysis in March, while drought intensity should be continuously investigated by season or year. Bushra et al. (Citation2019) suggested that using NDWI is unable to differentiate the stress to vegetation canopies occurred due to drought. Alternatively, Le et al. (Citation2021) and Thuong et al. (Citation2022) used remote sensing- based VHI to examine the drought variation in the study area in a 20-year time period. The VHI has significantly improved the major NDDI drawbacks in relation to applications of NDVI and LST based on the normalized algorithm. However, some limitations of VHI have been described within the introduction section (Bento et al. Citation2020; Sun et al. Citation2020; Zeng et al. Citation2022). More importantly, previous studies in the region have not included LULC, while changes in LULC significantly influence drought conditions.
In this study, we examined both annual and seasonal patterns of the drought conditions and integrated the trend of drought and pattern of LULC in drought-affected areas. The trend and pattern of drought within the LULC types can support the development of appropriate land use management plans to minimize the effects of drought. Our study discovered that evergreen broadleaf forests help to reduce affected-drought areas because trees could store water in rainy seasons and provide water balance in dry seasons. High density forest cover or trees with large canopy enable a significant decrease in heat flux, resulting in the reduction of soil evapotranspiration (Rhee, Im, and Carbone Citation2010). However, a decrease in forest area (i.e. evergreen broadleaf forests and deciduous broadleaf forests), mostly replaced by croplands, was exposed from 2000 to 2019. Changing from woody vegetation cover to cropland could negatively affect the level of water table in relation to reduction of infiltration processes due to increase in surface flow resultant of deforestation. Hence, converting land use types in the Central Highlands should be of concern for forest protection. Within the deciduous broadleaf forests, the seasonal change in drought pattern is clearly presented because these forests shed their leaves in dry season and grow back in rainy season. This change facilitates explaining the insignificant pattern of cluster A () because this region is mostly covered by shrubs and deciduous broadleaf forest types.
Besides, understanding the long-term trends in drought patterns under the impacts of human activities also provides valuable information to develop appropriate land and environmental management practices which can adapt and mitigate the climate change effects. It has been shown that the drought intensity becomes significantly high in El Niño years (e.g. 2002, 2004), while in the La Nina events (e.g. 2008, 2011), the iMDI value represents non-drought conditions (). However, developing irrigation systems to produce crops in the dry season can reduce drought severity, as a result of increasing soil moisture and expanding leaf area (Rey, Holman, and Knox Citation2017). The drought severity was not significant in the dry season of 2015–2016 despite a historical El Niño event because of a substantial enhancement to the irrigation system for crop cultivation that enabled a greater supply of water in the dry season in the study area (Vu, Vu, and Trinh Citation2019). In fact, the croplands often consume a large amount of water, especially in the dry season, in order to achieve high productivity. The amount of water supply for perennial croplands (e.g. coffee) is much higher than that of annual croplands (e.g. rice). For example, coffee normally consumes 390–520 litters/root with a cycle of 25–27 days/time in the granulation stage, while paddy rice needs only 175–200 litter/root for the whole growth stage (Hieu and Hao Citation2000; Tan et al. Citation2018). Also, coffee trees require adequate soil moisture conditions during the flowering and fruiting stages, so that a significant amount of water provided by irrigation often added to the soil in dry season (Tan et al. Citation2018). As such, this helps minimize the drought severity in the areas where perennial croplands are cultivated.
Considering that major findings obtained from this study provide spatially explicit information (e.g. the trend and pattern of drought severity are presented on maps), spatial-based management and mitigation strategies can be developed to better adapt to local variations in drought and environmental conditions. The applications mentioned above demonstrate that our study is directly linked to the Goal 13 (climate action) and Goal 15 (life on land) among 17 of UN Sustainable Development Goals adopted in 2015 (General Assembly of the UN Citation2015; Honeck et al. Citation2018). Supporting these SDGs is considered as an efficient solution to achieve the long-term sustainable development of the region.
6. Conclusions
This research proposed an integrated multivariate drought index (iMDI) using monthly normalized ESI (ET/PET), LST, and NDVI from the MODIS products during 2001–2020 period. The iMDI synthesized the moisture deficits, soil thermal stress, and vegetation growth status in drought processes and makes it favorable for comprehensive drought monitoring. The results from this study revealed that the influence of drought severity mainly focused on the boundary regions of Gia Lai and Kon Tum provinces. Additionally, changes in drought conditions upon five persistent land use types (i.e. evergreen broadleaf forests, deciduous broadleaf forests, annual croplands, perennial croplands, and shrubs) were explored for 20 years in the study area. An increasing trend in affected-drought areas was discovered in cropland and shrubland areas.
This study demonstrated that the proposed approach that uses multi-source remote sensing data enables the achievement of more accurate drought information. This approach does not only cover the meteorological drought information but also reveals the impacts of drought on agriculture. The natural hazard associated with drought is a complex phenomenon and it is a slow process, not an event, that always begins with a precipitation deficit and progresses to drought conditions if the shortage persists for an extended period of time. Various effects of drought have been discovered, including a reduction in soil moisture, an increase in LST, and a negative impact on vegetation growth. As such, it is important that information related to evapotranspiration, soil, and vegetation is incorporated in the monitoring and assessment of drought condition to better understand this process, we must consider the spheres of evapotranspiration, soil, and vegetation.
Given that necessary MODIS data that are used to develop iMDI are globally available, the approach proposed here can be applied to any other areas in the world. In order to achieve better results for local scale application, we suggest that future studies employ higher spatial resolution data to develop drought indices using a similar approach we developed here. For instance, the continental monthly product for AET available in Australia (Guerschman et al. Citation2022; McVicar et al. Citation2022), in addition to Landsat LST and NDVI that can be utilized to develop iMDI at 30 m resolution for this region. In addition, a higher spatial resolution of LULC (e.g. 30 m, 10 m) should be used to analyze the impacts of anthropogenic activities on drought conditions. It is also important that future research considers other physical and socio-economic factors in addition to LULC such as topography, irrigation systems, soil type, household income, population density, and agricultural practices to fully explain the drought variations.
Acronyms
DSI | = | Drought Severity Index |
EDSI | = | Enhanced Drought Severity Index |
ESI | = | Evaporative Stress Index |
ET | = | Actual evapotranspiration |
iMDI | = | integrated Multivariate Drought Index |
LST | = | Land Surface Temperature |
LULC | = | Land Use Land Cover |
MODIS | = | MODerate-resolution Imaging Spectroradiometer |
NDVI | = | Normalized Different Vegetation Index |
PET | = | Potential EvapoTranspiration |
RDI | = | Reconnaissance Drought Index |
SPI | = | Standardized Precipitation Index |
TCI | = | Temperature Condition Index |
UNDRR | = | United Nations Office for Disaster Risk Reduction |
VCI | = | Vegetation Condition Index |
VHI | = | Vegetation Health Index |
Author Contributions
T.V.T., D.X.T. conceived the idea of the study; D.B.N., T.V.T. obtained and processed data from GEE for measurement; T.V.T., D.X.T. analyzed the data. T.V.T., D.B, C.H., and S.W.M. wrote manuscript. All authors contributed to the manuscript.
Disclosure statement
No potential conflict of interest was reported by the authors.
Data availability statement
The data that support the findings of this study (i.e. normalized difference vegetation index, land surface temperature, evapotranspiration, land use and land cover, drought indices, and probability maps, respectively) are obtained from the Google Earth Engine (https://earthengine.google.com/) and available from the corresponding author, TVT, upon reasonable request.
Additional information
Funding
Notes
1. The rainfall that is intercepted by the tree canopy and successively evaporates from the leaves.
References
- Aghabozorgi, S., A. S. Shirkhorshidi, and T. Y. Wah. 2015. “Time-Series Clustering–A Decade Review.” Information Systems 53: 16–23. doi:10.1016/j.is.2015.04.007.
- Amarasinghe, U. A., C. T. Hoanh, D. D’Haeze, and T. Q. Hung. 2015. “Toward Sustainders, A., Tian, Y., Varadi, F.Able Coffee Production in Vietnam: More Coffee with Less Water.” Agricultural systems 136: 96–105. doi:10.1016/j.agsy.2015.02.008.
- Anderson, M. C., C. Hain, B. Wardlow, A. Pimstein, J. R. Mecikalski, and W. P. Kustas. 2011. “Evaluation of Drought Indices Based on Thermal Remote Sensing of Evapotranspiration Over the Continental United States.” Journal of Climate 24 (8): 2025–2044. doi:10.1175/2010JCLI3812.1.
- Anderson, M. C., J. M. Norman, J. R. Mecikalski, J. A. Otkin, and W. P. Kustas. 2007a. “A Climatological Study of Evapotranspiration and Moisture Stress Across the Continental United States Based on Thermal Remote Sensing: 1. Model Formulation.” Journal of Geophysical Research Atmospheres 112 (D10). doi:10.1029/2006JD007506.
- Anderson, M. C., J. M. Norman, J. R. Mecikalski, J. A. Otkin, and W. P. Kustas. 2007b. “A Climatological Study of Evapotranspiration and Moisture Stress Across the Continental United States Based on Thermal Remote Sensing: 1. Model Formulation.” Journal of Geophysical Research Atmospheres 112 (D10). doi:10.1029/2006JD007506.
- Aniruddha, B. 2020. Feature Scaling | Standardization Vs Normalization. Analytics Vidhya. https://www.analyticsvidhya.com/blog/2020/04/feature-scaling-machine-learning-normalization-standardization/.
- Bento, V. A., C. M. Gouveia, C. C. DaCamara, R. Libonati, and I. F. Trigo. 2020. “The Roles of NDVI and Land Surface Temperature When Using the Vegetation Health Index Over Dry Regions.” Global and Planetary Change 190: 103198. doi:10.1016/j.gloplacha.2020.103198.
- Bradford, J. B., D. R. Schlaepfer, W. K. Lauenroth, and K. A. Palmquist. 2020. “Robust Ecological Drought Projections for Drylands in the 21st Century.” Global Change Biology 26 (7): 3906–3919. doi:10.1111/gcb.15075.
- Brust, C., J. S. Kimball, M. P. Maneta, K. Jencso, M. He, and R. H. Reichle. 2021. “Using SMAP Level-4 Soil Moisture to Constrain MOD16 Evapotranspiration Over the Contiguous USA.” Remote Sensing of Environment 255: 112277. doi:10.1016/j.rse.2020.112277.
- Bushra, N., R. V. Rohli, N. S. N. Lam, L. Zou, R. B. Mostafiz, and V. Mihunov. 2019. “The Relationship Between the Normalized Difference Vegetation Index and Drought Indices in the South Central United States.” Natural Hazards 96 (2): 791–808. doi:10.1007/s11069-019-03569-5.
- Cao, Y., S. Chen, L. Wang, B. Zhu, T. Lu, and Y. Yu. 2019. “An Agricultural Drought Index for Assessing Droughts Using a Water Balance Method: A Case Study in Jilin Province, Northeast China.” Remote Sensing 11 (9): 1066. doi:10.3390/rs11091066.
- Christian, J. I., J. B. Basara, J. A. Otkin, E. D. Hunt, R. A. Wakefield, P. X. Flanagan, and X. Xiao. 2019. “A Methodology for Flash Drought Identification: Application of Flash Drought Frequency Across the United States.” Journal of Hydrometeorology 20 (5): 833–846. doi:10.1175/JHM-D-18-0198.1.
- Chuyen, T., 2020. Giảm diện tích rừng ở Tây nguyên [ online]. Thanh niên. Available from: https://thanhnien.vn/thoi-su/giam-dien-tich-rung-o-tay-nguyen-1242144.html [Accessed 13 Mar 2021].
- Crausbay, S. D., A. R. Ramirez, S. L. Carter, M. S. Cross, K. R. Hall, D. J. Bathke, J. L. Betancourt, et al. 2017. “Defining Ecological Drought for the Twenty-First Century.” Bulletin of the American Meteorological Society 98 (12): 2543–2550. doi:10.1175/BAMS-D-16-0292.1.
- de Carvalho Júnior, O. A., R. F. Guimarães, C. R. Silva, and R. A. T. Gomes. 2015. “Standardized Time-Series and Interannual Phenological Deviation: New Techniques for Burned-Area Detection Using Long-Term MODIS-NBR Dataset.” Remote Sensing 7 (6): 6950–6985. doi:10.3390/rs70606950.
- Dinh, N. T., N. T. T. Ha, N. T. P. Thao, and N. T. Linh, 2017. 30 Years Monitoring Spatial - Temporal Dynamics of Agricultural Drought in the Central Highlands Using Landsat Data. In: Geo-spatial Technologies and Earth Resources. Presented at the The International Conference on Geo-Spatial Technologies and Earth Resources, Hanoi City, Vietnam: Publishing House for Science and Technology, 181–188.
- Dorjsuren, M., Y. -A. Liou, and C. -H. Cheng. 2016. “Time Series MODIS and in situ Data Analysis for Mongolia Drought.” Remote Sensing 8 (6): 509. doi:10.3390/rs8060509.
- Dzierżak, R. 2019. “Comparison of the Influence of Standardization and Normalization of Data on the Effectiveness of Spongy Tissue Texture Classification.” Informatyka, Automatyka, Pomiary w Gospodarce i Ochronie Środowiska 9 (3): 66–69. doi:10.35784/iapgos.62.
- Elhag, K., and W. Zhang. 2018. “Monitoring and Assessment of Drought Focused on Its Impact on Sorghum Yield Over Sudan by Using Meteorological Drought Indices for the Period 2001–2011.” Remote Sensing 10 (8): 1231. doi:10.3390/rs10081231.
- Erickson, T., 2014. Multi-Source Geospatial Data Analysis with Google Earth Engine. In: AGU Fall Meeting Abstracts 2014: IN53E-05, San Francisco, US.
- FAO. 2021. The Impact of Disasters and Crises on Agriculture and Food Security. Rome, Italy: Food and Agriculture Organization of the United Nations.
- Frazier, R. J., N. C. Coops, M. A. Wulder, T. Hermosilla, and J. C. White. 2018. “Analyzing Spatial and Temporal Variability in Short-Term Rates of Post-Fire Vegetation Return from Landsat Time Series.” Remote Sensing of Environment 205: 32–45. doi:10.1016/j.rse.2017.11.007.
- General Assembly of the UN. 2015. “Sustainable Development Goals.” SDGs, Transforming Our World: The 2030, 338–350. New York: UN.
- Ghil, M., M. R. Allen, M. D. Dettinger, K. Ide, D. Kondrashov, M. E. Mann, A. W. Robertson, et al. 2002. “Advanced Spectral MetMapping Droughts Over the Central Highland of Vietnam in El Niño Years Using Landsat Imagerieshods for Climatic Time Series.” Reviews of Geophysics 40 (1): 3-3–41. doi:10.1029/2000RG000092
- Gorelick, N., M. Hancher, M. Dixon, S. Ilyushchenko, D. Thau, and R. Moore. 2017. “Google Earth Engine: Planetary-Scale Geospatial Analysis for Everyone.” Remote Sensing of Environment 202: 18–27. doi:10.1016/j.rse.2017.06.031.
- Grabusts, P., and A. Borisov. 2009. “Clustering Methodology for Time Series Mining.” Computer Science 40 (1): 40. doi:10.2478/v10143-010-0011-0.
- GSO. 2021. Viet Nam Statistical Yearbook of 2020. General Statistics Office. Hanoi City, Vietnam: Statistical Publishing House.
- Guerschman, J. P., T. R. McVicar, J. Vleeshower, T. G. Van Niel, J. L. Peña-Arancibia, and Y. Chen. 2022. “Estimating Actual Evapotranspiration at Field-to-Continent Scales by Calibrating the CMRSET Algorithm with MODIS, VIIRS, Landsat and Sentinel-2 Data.” Journal of Hydrology 605: 127318. doi:10.1016/j.jhydrol.2021.127318.
- Guijo-Rubio, D., A. M. Durán-Rosal, P. A. Gutiérrez, A. Troncoso, and C. Hervás-Martínez. 2020. “Time-Series Clustering Based on the Characterization of Segment Typologies.” IEEE Transactions on Cybernetics 51 (11): 5409–5422. doi:10.1109/TCYB.2019.2962584.
- Guttman, N. B. 1998. “Comparing the Palmer Drought Index and the Standardized Precipitation Index 1.” JAWRA Journal of the American Water Resources Association 34 (1): 113–121. doi:10.1111/j.1752-1688.1998.tb05964.x.
- Guyet, T., and H. Nicolas. 2016. “Long Term Analysis of Time Series of Satellite Images.” Pattern recognition letters 70: 17–23. doi:10.1016/j.patrec.2015.11.005.
- Ha, T. V., J. Huth, F. Bachofer, and C. Kuenzer. 2022. “A Review of Earth Observation-Based Drought Studies in Southeast Asia.” Remote Sensing 14 (15): 3763. doi:10.3390/rs14153763.
- Hao, Z., and A. AghaKouchak. 2013. “Multivariate Standardized Drought Index: A Parametric Multi-Index Model.” Advances in Water Resources 57: 12–18. doi:10.1016/j.advwatres.2013.03.009.
- Hao, C., J. Zhang, and F. Yao. 2015. “Combination of Multi-Sensor Remote Sensing Data for Drought Monitoring Over Southwest China.” International Journal of Applied Earth Observation and Geoinformation 35: 270–283. doi:10.1016/j.jag.2014.09.011.
- Heo, J., and T. W. FitzHugh. 2000. “A Standardized Radiometric Normalization Method for Change Detection Using Remotely Sensed Imagery.” Photogrammetric Engineering and Remote Sensing 66 (2): 173–181.
- Hieu, B., and L. Hao. 2000. Irrigation techniques for crops. Hanoi City, Vietnam: Agriculture Publishing House.
- Honeck, E., R. Castello, B. Chatenoux, J. -P. Richard, A. Lehmann, and G. Giuliani. 2018. “From a Vegetation Index to a Sustainable Development Goal Indicator: Forest Trend Monitoring Using Three Decades of Earth Observations Across Switzerland.” ISPRS International Journal of Geo-Information 7 (12): 455. doi:10.3390/ijgi7120455.
- Hosking, J. R. M., and J. R. Wallis. 1997. Regional Frequency Analysis: An Approach Based on L-Moments. Cambridge, UK: Cambridge University Press.
- Hu, C., J. Xia, D. She, L. Li, Z. Song, and S. Hong. 2021. “A New Framework for the Identification of Flash Drought: Multivariable and Probabilistic Statistic Perspectives: Identification of Flash Drought.” International Journal of Climatology 41 (13): 5862–5878. doi:10.1002/joc.7157.
- Jiao, W., L. Wang, and M. F. McCabe. 2021. “Multi-Sensor Remote Sensing for Drought Characterization: Current Status, Opportunities and a Roadmap for the Future.” Remote sensing of environment 256: 112313. doi:10.1016/j.rse.2021.112313.
- Jönsson, P., Z. Cai, E. Melaas, M. A. Friedl, and L. Eklundh. 2018. “A Method for Robust Estimation of Vegetation Seasonality from Landsat and Sentinel-2 Time Series Data.” Remote Sensing 10 (4): 635. doi:10.3390/rs10040635.
- Jung, M., M. Reichstein, P. Ciais, S. I. Seneviratne, J. Sheffield, M. L. Goulden, G. Bonan, et al. 2010. “Recent Decline in the Global Land Evapotranspiration Trend Due to Limited Moisture Supply.” Nature 467 (7318): 951–954. doi:10.1038/nature09396.
- Karnieli, A., M. Bayasgalan, Y. Bayarjargal, N. Agam, S. Khudulmur, and C. J. Tucker. 2006. “Comments on the Use of the Vegetation Health Index Over Mongolia.” International Journal of Remote Sensing 27 (10): 2017–2024. doi:10.1080/01431160500121727.
- Kerr, Y. H., J. -P. Wigneron, A. Al Bitar, A. Mialon, and P. K. Srivastava. 2016. “Soil moisture from space: Techniques and limitations.” In Satellite Soil Moisture Retrieval, 3–27. Amsterdam, Netherlands: Elsevier.
- Kogan, F. N. 1995. “Application of Vegetation Index and Brightness Temperature for Drought Detection.” Advances in Space Research 15 (11): 91–100. doi:10.1016/0273-1177(95)00079-T.
- Kogan, F., and J. Sullivan. 1993. “Development of Global Drought-Watch System Using NOAA/AVHRR Data.” Advances in Space Research 13 (5): 219–222. doi:10.1016/0273-1177(93)90548-P.
- Le, T., C. Sun, S. Choy, and Y. Kuleshov. 2021. “Regional Drought Risk Assessment in the Central Highlands and the South of Vietnam.” Geomatics, Natural Hazards and Risk 12 (1): 3140–3159. doi:10.1080/19475705.2021.1998232.
- Lokman, S. -F., A. T. Othman, M. H. A. Bakar, and S. Musa, 2019. “The Impact of Different Feature Scaling Methods on Intrusion Detection for In-Vehicle Controller Area Network (CAN)”. In: International Conference on Advances in Cyber Security, pp. 195–205. Singapore: Springer.
- MARD, M. of A. and R.D., 2021. Quyết định số 1558/QĐ-BNN-TCLN về công bố hiện trạng rừng năm 2020.
- McKee, T. B., N. J. Doesken, and J. Kleist, 1993. The Relationship of Drought Frequency and Duration to Time Scales. In: Proceedings of the 8th Conference on Applied Climatology. American Meteorological Society Boston, MA, 179–183.
- McVicar, T., J. Vleeshouwer, T. Van Niel, and J. Guerschman. 2022. “Actual Evapotranspiration for Australia Using CMRSET Algorithm.” Terrestrial Ecosystem Research Network. doi:10.25901/gg27-ck96.
- Mu, Q., F. A. Heinsch, M. Zhao, and S. W. Running. 2007. “Development of a Global Evapotranspiration Algorithm Based on MODIS and Global Meteorology Data.” Remote Sensing of Environment 111 (4): 519–536. doi:10.1016/j.rse.2007.04.015.
- Mu, Q., M. Zhao, J. S. Kimball, N. G. McDowell, and S. W. Running. 2013. “A Remotely Sensed Global Terrestrial Drought Severity Index.” Bulletin of the American Meteorological Society 94 (1): 83–98. doi:10.1175/BAMS-D-11-00213.1.
- Mu, Q., M. Zhao, and S. W. Running. 2011. “Improvements to a MODIS Global Terrestrial Evapotranspiration Algorithm.” Remote sensing of environment 115 (8): 1781–1800. doi:10.1016/j.rse.2011.02.019.
- Myronidis, D., K. Ioannou, D. Fotakis, and G. Dörflinger. 2018. “Streamflow and Hydrological Drought Trend Analysis and Forecasting in Cyprus.” Water Resources Management 32 (5): 1759–1776. doi:10.1007/s11269-018-1902-z.
- NDMC. 2022. How Does Drought Affect Our Lives? [Online]. National Drought Mitigation Center. Available from: https://drought.unl.edu/Education/DroughtforKids/DroughtEffects.aspx.
- Nguyen, H. T. T., N. T. Mai, C. D. Bui, and T. T. P. Nguyen. 2016. ”Mapping Droughts Over the Central Highland of Vietnam in El Niño Years Using Landsat Imageries.” VNU Journal of Science: Social Sciences and Humanities 32 (1S): 255–263.
- Nguyen, Q. T. N., L. D. Nguyen, N. D. Nguyen, T. Nguyen, L. T. Bui, and L. K. Nguyen. 2017. “Phân vùng hạn hán dựa trên chỉ số hạn và mô phỏng chế độ thủy văn trên lưu vực Srepok vùng Tây Nguyên.” VNU Journal of Science: Earth and Environmental Sciences 33 (1): 65–81.
- Ontel, I., A. Irimescu, G. Boldeanu, D. Mihailescu, C. -V. Angearu, A. Nertan, V. Craciunescu, and S. Negreanu. 2021. “Assessment of Soil Moisture Anomaly Sensitivity to Detect Drought Spatio-Temporal Variability in Romania.” Sensors 21 (24): 8371. doi:10.3390/s21248371.
- Orvos, P. I., V. Homonnai, A. Várai, Z. Bozóki, and I. M. Jánosi. 2015. “Global Trend Analysis of the MODIS Drought Severity Index.” Geoscientific Instrumentation, Methods and Data Systems 4 (2): 189–196. doi:10.5194/gi-4-189-2015.
- Paulo, A. A., and L. S. Pereira. 2006. “Drought Concepts and Characterization.” Water International 31 (1): 37–49. doi:10.1080/02508060608691913.
- Peng, J., A. Loew, O. Merlin, and N. E. C. Verhoest. 2017. “A Review of Spatial Downscaling of Satellite Remotely Sensed Soil Moisture.” Reviews of Geophysics 55 (2): 341–366. doi:10.1002/2016RG000543.
- Rey, D., I. P. Holman, and J. W. Knox. 2017. “Developing Drought Resilience in Irrigated Agriculture in the Face of Increasing Water Scarcity.” Regional Environmental Change 17 (5): 1527–1540. doi:10.1007/s10113-017-1116-6.
- Rhee, J., J. Im, and G. J. Carbone. 2010. “Monitoring Agricultural Drought for Arid and Humid Regions Using Multi-Sensor Remote Sensing Data.” Remote sensing of environment 114 (12): 2875–2887. doi:10.1016/j.rse.2010.07.005.
- Running, S. W., Q. Mu, and M. Zhao. 2019. MOD16A2 MODIS/Terra Net Evapotranspiration 8-Day L4 Global 500m SIN Grid V006 [Data set]. Distributed Active Archive Center: NASA DAAC.
- Seiler, R. A., F. Kogan, and J. Sullivan. 1998. “AVHRR-Based Vegetation and Temperature Condition Indices for Drought Detection in Argentina.” Advances in Space Research 21 (3): 481–484. doi:10.1016/S0273-1177(97)00884-3.
- Shah, D., and V. Mishra. 2020. “Integrated Drought Index (IDI) for Drought Monitoring and Assessment in India.” Water Resources Research 56 (2): e2019WR026284. doi:10.1029/2019WR026284.
- Sherval, M., L. E. Askew, and P. M. McGuirk. 2014. ”Manifestations of Drought.” In Encyclopedia of Quality of Life and Well-Being Research, A. C. Michalos ed., 3756–3761. Dordrecht: Springer Netherlands
- Shumway, R. H., and D. S. Stoffer. 2017. “Characteristics of Time Series.” In Time Series Analysis and Its Applications: With R Examples, edited by R. H. Shumway and D. S. Stoffer, 1–44. Cham: Springer International Publishing.
- Singh, R. P., S. Roy, and F. Kogan. 2003. “Vegetation and Temperature Condition Indices from NOAA AVHRR Data for Drought Monitoring Over India.” International Journal of Remote Sensing 24 (22): 4393–4402. doi:10.1080/0143116031000084323.
- Son, B., S. Park, J. Im, S. Park, Y. Ke, and L. J. Quackenbush. 2021. “A New Drought Monitoring Approach: Vector Projection Analysis (VPA).” Remote Sensing of Environment 252: 112145. doi:10.1016/j.rse.2020.112145.
- Spinoni, J., P. Barbosa, A. De Jager, N. McCormick, G. Naumann, J. V. Vogt, D. Magni, D. Masante, and M. Mazzeschi. 2019. “A New Global Database of Meteorological Drought Events from 1951 to 2016.” Regional Studies 22: 100593. doi:10.1016/j.ejrh.2019.100593.
- Sulla-Menashe, D., and M. A. Friedl. 2018. User Guide to Collection 6 MODIS Land Cover (MCD12Q1 and MCD12C1) Product, 1–18. Reston, VA, USA: USGS.
- Sun, X., M. Wang, G. Li, and Y. Wang. 2020. “Regional-Scale Drought Monitor Using Synthesized Index Based on Remote Sensing in Northeast China.” Open Geosciences 12 (1): 163–173. doi:10.1515/geo-2020-0037.
- Tan, N. V., N. V. Chien, D. Hong, and N. Le. 2018. Modular Textbook: Caring for Coffee Plants - Coffee Farming. Hanoi City, Vietnam: Agriculture Publishing House.
- Thornthwaite, C. W. 1947. “Climate and Moisture Conservation.” Annals of the Association of American Geographers 37 (2): 87–100. doi:10.1080/00045604709351946.
- Thuong, T., C. Xuan, N. Hieu, P. Tuan, and D. Mon. 2022. “Examininging Drought Intensity Inentral Highlands During the 2001–2020 Period Using Daily MODIS Time–Series Imagery.” Vietnam Journal of Hydrometeorology 733: 1–14.
- Tigkas, D., H. Vangelis, and G. Tsakiris. 2013. “The RDI as a Composite Climatic Index.” Europe Water 41: 17–22.
- Tigkas, D., H. Vangelis, and G. Tsakiris. 2015. “DrinC: A Software for Drought Analysis Based on Drought Indices.” Earth Science Informatics 8 (3): 697–709. doi:10.1007/s12145-014-0178-y.
- Toan, P. N., and P. T. Dac. 1975. “Climatic Conditions of Vietnam.” Science and Technics Publishing House. Hanoi, Vietnam.
- Tran, H. T., J. B. Campbell, T. D. Tran, and H. T. Tran. 2017. “Monitoring Drought Vulnerability Using Multispectral Indices Observed from Sequential Remote Sensing (Case Study: Tuy Phong, Binh Thuan, Vietnam).” GIScience & Remote Sensing 54 (2): 167–184. doi:10.1080/15481603.2017.1287838.
- Tran, T. V., D. X. Tran, S. W. Myint, P. Latorre-Carmona, D. D. Ho, P. H. Tran, and H. N. Dao. 2019. “Assessing Spatiotemporal Drought Dynamics and Its Related Environmental Issues in the Mekong River Delta.” Remote Sensing 11 (23): 2742. doi:10.3390/rs11232742.
- Trieu, V., T. Pham, and T. Dao. 2021. Kết quả thực hiện Chiến lược phát triển Lâm nghiệp Việt Nam giai đoạn 2006–2020 và đề xuất nội dung Chiến lược phát triển Lâm nghiệp Việt Nam giai đoạn 2021–2030, tầm nhìn đến năm 2050. Bogor, Indonesia: Tổ chức Nghiên cứu Lâm nghiệp Quốc tế (CIFOR).
- Tsakiris, G., D. Pangalou, and H. Vangelis. 2007. “Regional Drought Assessment Based on the Reconnaissance Drought Index (RDI).” Water Resources Management 21 (5): 821–833. doi:10.1007/s11269-006-9105-4.
- Tsakiris, G., and H. Vangelis. 2005. “Establishing a Drought Index Incorporating Evapotranspiration.” European Water 9 (10): 3–11.
- Um, M. -J., Y. Kim, and D. Park. 2018. “Evaluation and Modification of the Drought Severity Index (DSI) in East Asia.” Remote sensing of environment 209: 66–76. doi:10.1016/j.rse.2018.02.044.
- UNDRR, 2021. GAR Special Report on Drought 2021. United Nations Office for Disaster Risk Reduction. Geneva, Switzerland.
- Vu, A. T., T. H. Vu, and H. D. Trinh. 2019. “The Characteristics and Trends of Meteorological Drought in Central Highlands.” Vietnam Journal of Hydrometeorology 3: 50–58.
- Wan, X. 2019. “Influence of Feature Scaling on Convergence of Gradient Iterative Algorithm.” Journal of Physics Conference Series 1213 (3): 032021. doi:10.1088/1742-6596/1213/3/032021.
- West, H., N. Quinn, and M. Horswell. 2019. “Remote sensing for drought monitoring & impact assessment: Progress, past challenges and future opportunities.” Remote sensing of environment 232: 111291. doi:10.1016/j.rse.2019.111291.
- Wilhite, D. A., and M. H. Glantz. 1985. “Understanding: The Drought Phenomenon: The Role of Definitions.” Water International 10 (3): 111–120. doi:10.1080/02508068508686328.
- WMO, 2016. Drought. World Meteorological Organization. URL: https://public.wmo.int/en/our-mandate/water/drought [accessed on 20/10/2022].
- WMO, 2022. Droughts Threaten Sustainable Development. World Meteorological Organization. URL: https://public.wmo.int/en/media/news/droughts-threaten-sustainable-development [accessed on 20/10/2022].
- WMO and GWP. 2016. Handbook of Drought Indicators and Indices. (M. Svoboda and B.A. Fuchs). Integrated Drought Management Programme (IDMP), Integrated Drought Management Tools and Guidelines Series 2. Geneva: World Meteorological Organization and Global Water Partnership.
- Wu, B., Z. Ma, and N. Yan. 2020. “Agricultural Drought Mitigating Indices Derived from the Changes in Drought Characteristics.” Remote sensing of environment 244: 111813. doi:10.1016/j.rse.2020.111813.
- Yao, Y., S. Liang, Q. Qin, and K. Wang. 2010. “Monitoring Drought Over the Conterminous United States Using MODIS and NCEP Reanalysis-2 Data.” Journal of Applied Meteorology and Climatology 49 (8): 1665–1680. doi:10.1175/2010JAMC2328.1.
- Yoo, J., H. -H. Kwon, T. -W. Kim, and J. -H. Ahn. 2012. “Drought Frequency Analysis Using Cluster Analysis and Bivariate Probability Distribution.” Journal of Hydrology 420-421: 102–111. doi:10.1016/j.jhydrol.2011.11.046.
- Yu, H., Q. Zhang, C. -Y. Xu, J. Du, P. Sun, and P. Hu. 2019. “Modified Palmer Drought Severity Index: Model Improvement and Application.” Environment international 130: 104951. doi:10.1016/j.envint.2019.104951.
- Zakaria, J., 2019. When and Why to Standardize Your Data? [ online]. Built In. Available from: https://builtin.com/data-science/when-and-why-standardize-your-data [Accessed 2 Sep 2021].
- Zeng, J., R. Zhang, Y. Qu, V. A. Bento, T. Zhou, Y. Lin, X. Wu, J. Qi, W. Shui, and Q. Wang. 2022. “Improving the Drought Monitoring Capability of VHI at the Global Scale via Ensemble Indices for Various Vegetation Types from 2001 to 2018.” Weather and Climate Extremes 35: 100412. doi:10.1016/j.wace.2022.100412.
- Zhang, Z., P. Tang, L. Huo, and Z. Zhou. 2014. “MODIS NDVI Time Series Clustering Under Dynamic Time Warping.” International Journal of Wavelets, Multiresolution and Information Processing 12 (05): 1461011. doi:10.1142/S0219691314610116.