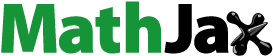
ABSTRACT
Spatially explicit information on the distribution of dominant tree species groups and aboveground biomass (AGB) in forested areas is essential for developing targeted forest management and biodiversity conservation measures, as well as assessing forest carbon sequestration capacity. There is a shortage of continuously updated 30-m spatial resolution products for mapping dominant tree species groups. The vast majority of remote sensing-based AGB estimation approaches have relatively low accuracy for dominant tree species groups or forest types and are unsuitable for AGB modeling. Therefore, this study aims to develop an integrated framework that considers the phenological characteristics of different tree species to improve the mapping accuracies of forest dominant tree groups and corresponding AGB estimates. Thirty-meter resolution maps of dominant tree species groups were created using machine learning algorithms and phenological parameters. Features extracted from optical and radar images and phenological characteristics were used to construct AGB estimation models in a temporally consistent manner to improve the AGB estimation accuracy and perform dynamic AGB monitoring. The proposed method accurately characterized the dynamic distribution of the dominant tree species groups in the study area. The traditional AGB model that does not consider different forest types or species had an R2 value of 0.52, whereas the proposed model that considers phenology and forest types had an R2 value of 0.67. This result indicates that incorporating information on phenology and dominant species improves the accuracy of AGB estimations. The AGB in most regions was 30–55 t/ha, showing that the majority of the forests were young or middle-aged stands, and the areal percentage of AGB greater than 30 t/ha increased during the study period, suggesting an improvement in forest quality. Furthermore, the oak AGB was the highest, indicating that oak afforestation should be encouraged to enhance the carbon sequestration capacity of future forest ecosystems. The results provide new insights for researchers and managers to understand the trends of forest development and forest health, as well as technical information and a database for formulating more rational forest management strategies.
1 Introduction
Spatially explicit information on the distribution of dominant tree species groups in forested areas is critical for numerous scientific applications, such as biodiversity protection, biomass estimation, and tree species management (Boschetti et al. Citation2007; Fassnacht et al. Citation2016; Shang and Chisholm Citation2014). Extensive field surveys conducted by forestry professionals provide highly accurate information. However, this approach is time-consuming, labor-intensive, and cost-ineffective, and areas with steep slopes can often not be accessed (Cao Citation2020). The use of modern remote sensing techniques coupled with well-tuned algorithms to derive dominant tree species distributions is a reliable alternative because of the wide availability of multi- and hyper-spectral, multi-temporal, and multi-scale resolution imagery. These approaches facilitate the extraction of forest information primarily based on spectral variables and textural features (Linderman et al. Citation2004; Zhu and Liu Citation2014; Araza et al. Citation2022; Fassnacht et al. Citation2016). Due to the high temporal revisit frequency of satellites such as Landsat, long-term observations have been widely used for large-scale dominant tree species classifications at 30 m spatial resolution. However, most studies have only distinguished broad classes of forest types, e.g. coniferous and broad-leaved forests, or have extracted single tree species (Linderman et al. Citation2004; Yang, Xu, and Zhai Citation2021). Detailed mapping of dominant tree species is generally performed using high spatial resolution or hyperspectral data (Cho, Malahlela, and Ramoelo Citation2015; Lin et al. Citation2015). Thus, there are insufficient mapping products for dominant tree species groups at medium spatial resolution.
The appropriate selection of variables or image features for dominant tree species group classifications is critical to improving the classification accuracy (Lu and Weng Citation2007). Phenological parameters are a promising feature for dominant tree species classification because the phenology of dominant tree species changes with the seasons. Some phenological events, such as leaves turning green in spring and yellow in autumn, and the timing of these events, are generally species-specific. Thus, tree species exhibit different spectral characteristics in multi-temporal remote sensing images, enabling the differentiation of dominant tree species in forestlands (Fassnacht et al. Citation2016; Yu et al. Citation2004). For example, Yu et al. (Citation2004) used MODIS time-series data to classify forest types and found that phenological parameters improved the separability of forest types. Yang, Xu, and Zhai (Citation2021) also confirmed that incorporating phenology into random forest (RF) modeling increased the mapping accuracy of dominant tree species. In addition to phenology, different spectral characteristics in different periods can be exploited to improve classification accuracy (Fassnacht et al. Citation2016). It has been suggested to avoid using late autumn images because the forest background signals make the separation of broadleaf species more difficult (Voss and Sugumaran Citation2008). Other studies have shown that the combination of images acquired in different seasons (one in spring and the other in autumn) can result in higher classification accuracy (Hill et al. Citation2010). In summary, we should consider phenological characteristics and the image acquisition time to classify dominant tree species.
Forest aboveground biomass (AGB) is critical to the global biogeochemical cycle and biodiversity conservation (Shen et al. Citation2016). Field measurement is the most accurate method for estimating forest AGB; however, this method lacks sufficient temporal and spatial coverage (Huiyi et al. Citation2020). As an alternative, Landsat imagery can be used to estimate forest AGB in large areas due to its adequate spatial and spectral resolution and wide coverage. In recent years, Landsat’s free and open policy has made long-term AGB assessments very popular. Many studies have shown that forest AGB and Landsat spectral data are highly correlated (Shen et al. Citation2016; Li, Li, and Li Citation2019; Huiyi et al. Citation2020). However, existing remote sensing-based AGB estimation models often have low accuracy due to an inadequate characterization of the forest structure. The distribution of tree groups is one of multiple horizontal stand structure characteristics and affects the accuracy of AGB modeling (Li et al. Citation2020; Li, Li, and Li Citation2019). Due to the lack of medium-resolution products suitable for characterizing dominant tree species groups in a changing landscape, most studies used a unified model for AGB estimation, regardless of the dominant tree species group or forest type, resulting in the overestimation (underestimation) of sparse (dense) forests (Singh et al. Citation2014). In addition, the selection of model variables is also highly critical for improving model accuracy. The most commonly used variables are spectral features extracted from optical remote sensing images, such as vegetation indices, and texture measures (Zhang et al. Citation2020; Bao et al. Citation2022; Pötzschner et al. Citation2022). However, these variables are limited because they lack temporal scalability and do not reflect the changing physiological characteristics of forests. New features are expected to improve AGB estimation. Research has demonstrated correlations between phenological characteristics and AGB (Ganjurjav et al. Citation2021; Keenan et al. Citation2014). For example, global warming prolongs the growing season and further increases forest carbon storage (Keenan et al. Citation2014). However, Ganjurjav et al. (Citation2021) also found that the shortening of the growing season increased the biomass in an alpine meadow on the Tibetan Plateau. Therefore, capturing these seasonal changes via remote sensing can reveal physical changes in forests.
Developing reliable methods to extract phenological characteristics and dominant tree species groups is a promising option to improve the accuracy of AGB estimation models. The major contribution of this work to forestry remote sensing is the proposed progressive and time-consistent strategy for forest AGB estimation. Phenological characteristics are extracted to map the distribution of dominant tree species groups with high accuracy. Subsequently, features from optical and radar images are combined with the phenological features to construct a time-consistent AGB estimation model based on tree species groups instead of having to model AGB at multiple time points.
2. Materials and methods
2.1 Study area
The study area is located in the east of the Dali Bai Autonomous Prefecture (99°57 ”−101 °03‘ E, 25°14 ’−26 °13” N) of Yunnan Province, southwest China. It includes Dali City, Xiangyun County, Binchuan County, parts of Midu, Eryuan, and Heqing Counties, and the Yangbi and Weishan minority autonomous counties. The total area is 11,405 km2 (). The highest altitude in the study area is 4295.8 m, and the lowest is 730 m. The dry and wet seasons are distinct in this study area. Dry-season rainfall (from November to early April) accounts for 5–15% of the annual rainfall, whereas wet-season rainfall (from May to October) accounts for 85–95% of the annual rainfall. The average annual precipitation is approximately 1000 mm, and the annual average temperature is around 15°C (Ding and Peng Citation2018). Pines (Pinus yunnanensis, Pinus armandii Franch.), oak (Quercus pannosa), and walnut (Juglans sigillata Dode.) are the dominant tree species in the study area (). The pine species are dominant in the evergreen coniferous forests, which are the most widely distributed forest type in this study area. These pine species have strong adaptability and can withstand cold, drought, and barren soil. Most oaks grow in evergreen broad-leaved forests and are adapted to the cold temperature at high altitudes. Walnut trees are a deciduous species with high economic value. They like sunlight and are suitable for rich and moist sandy soil. Although Dali prefecture has numerous vegetation species, the quality of some tree species is low, such as thin Pinus yunnanensis trunks and low density of walnut trees (). Existing timber forests include natural and middle-aged and young plantation forests. Due to an imbalance between wood supply and demand, it is difficult to support the large-scale development of the forestry industry.
Figure 1. Study area; (a-c) show the geographical location of the study area and the phenological observation sites; (d) is a Landsat 8 OLI false-color composite (R: NIR band, G: red band, B: green band) acquired on 15 December 2019.

2.2 Datasets
We used Landsat and Sentinel-2 imagery, Phased Array L-band SAR (PALSAR) data, and forest field survey datasets. The Landsat surface reflectance (SR) data included blue, green, red, near-infrared (NIR), shortwave infrared 1 (SWIR1), and SWIR2 bands with less than 80% cloud cover ranging from 1999 to 2019. They were principally used to extract forest phenological parameters, including the start of the growing season (SOS), end of the growing season (EOS), and length of the growing season (LOS). These data were also used to map dominant tree species groups and AGB. Sentinel-2 top-of-atmosphere (TOA) reflectance images from 2017 to 2019 were used as supplementary data for Landsat imagery to increase the observation frequency and improve the extraction accuracy of the forest phenological parameters. The Sentinel-2 bands corresponded to those of Landsat. A total of 610 scenes were collected, including 514 scenes from Landsat Path/Row number: 131/042 (152 Landsat 5 TM images, 243 Landsat 7 ETM+ images, and 119 Landsat 8 OLI images) and 96 scenes from Sentinel-2 orbital number: T47RPJ (58 Sentinel-2A images and 38 Sentinel-2B images).
Five PALSAR images from 2007, 2010, 2015, 2016, and 2019 were used to extract features for the model input to characterize the vertical structure of the forest canopy and improve the AGB prediction accuracy.
Forest survey data were employed for the training and validation of the classifications of the dominant tree species groups and the forest AGB estimation. We collected data from the national forest inventory (NFI) plots in 2011 and forest management planning inventory (FMPI) in 2007 and 2016 (Lei et al. Citation2009; Xie et al. Citation2011). The NFI records various forest attributes (tree height, diameter at breast height, stand volume, canopy density, dominant species, etc.) using systematic sampling. The permanent plots with a size of 28.28 m × 28.28 m are revisited by local forest investigators every 5 years in China. A total of 78 permanent sample plots in the study area were measured in 2011. The FMPI divides the forest areas into multiple irregular sub-compartments that cover the entire research area. Local forest investigators record the average attributes of each sub-compartment nearly every ten years (Lei et al. Citation2009; Xie et al. Citation2011). A total of 2000 forest samples (0.1–2 ha) were randomly selected from the 2007 and 2016 data. It is worth noting that pure forest areas were selected as samples. CongDe et al. (Citation2008) established a regression model between AGB and stock volume in Sichuan Province, southwest China, using extensive field measurements of Pinus yunnanensis, other pines, and oak. Thus, the species-specific biomass conversion factor was used to convert the stocking volume into forest AGB due to the geographic proximity (Appendix I) (CongDe et al. Citation2008) For dominant tree species without existing allometric biomass equations, the biomass equations of similar dominant tree species (same genus) were used to approximate their biomass. The field biomass data from 2007, 2011, and 2016 were combined to facilitate the establishment of the time-consistent estimation models.
2.3 Method
In our previous study (Zhang and Mingshi Citation2021), we integrated Landsat and Sentinel-2 to develop the modified continuous change detection and classification (MCCDC) model to extract phenological parameters. In this study, a similar method was again used to extract phenological parameters to further characterize the spatial distribution of forest tree species groups and AGB. Specifically, a series of multi-source data integration techniques were performed to match the Landsat and Sentinel 2 images after atmospheric correction and cloud mask (Zhang and Mingshi Citation2021). Subsequently, MCCDC model was adopted to synthesize cloud-free daily images and extract the MCCDC coefficients. Logistic regression models were used to extract the phenological parameters from the daily Landsat images (Zhang and Mingshi Citation2021). Based on MCCDC coefficients and phenological parameters, a map of dominant tree species groups was generated using a support vector machine (SVM). After the appropriate important variables were selected, a model with optical and PALSAR image features and phenological characteristics was constructed for different dominant tree species groups to estimate AGB in the entire study area. shows the flowchart of this study.
2.3.1 Image pre-processing
2.3.1.1 Pre-processing of Landsat and sentinel-2 data
Due to the large data volume, the atmospherically corrected Landsat SR data were ordered from the United States Geological Survey (USGS) Earth Resources Observation and Science Center (https://espa.cr.usgs.gov/ordering/new). The SR was multiplied by 10,000 to convert the data into an integer format for easy storage and handling. The USGS uses the Landsat Ecosystem Disturbance Adaptive Processing System (LEDAPS) algorithm for the atmospheric correction of TM and ETM+ images (Masek et al. Citation2006), whereas the Landsat 8 Surface Reflectance Code (LaSRC) algorithm is used for the atmospheric correction of the OLI images (Vermote et al. Citation2016). These two techniques are very mature and routinely implemented to generate highly accurate and consistent long-term (from 1982 to present) Landsat Analysis Ready Data (ARD) for surface change monitoring (Dwyer et al. Citation2018).
In addition, Sentinel-2 TOA reflectance data with less than 80% cloud cover from March to November 2017–2019 were obtained from the European Space Agency (ESA) as a supplement to Landsat data to increase the observation frequency. The bands were spectrally consistent with those of Landsat. The Sentinel-2 TOA reflectance data were atmospherically corrected to SR data using the Sen2cor radiative transfer atmospheric correction code (version 2.8, released 21 February 2019; http://step.esa.int/main/third-party-plugins-2/sen2cor/). The function of mask (Fmask) 4.0 software was used for batch cloud removal from all Landsat and Sentinel-2 data (Zhu and Woodcock Citation2014).
In addition to atmospheric correction and cloud masking, we also performed registration, resampling, BRDF correction, terrain correction, and bandpass adjustment to integrate the Landsat and Sentinel-2 data. Finally, Sentinel-2 was spatially and spectrally consistent with Landsat. The pre-processing parameters and results have been described by Zhang and Mingshi (Citation2021).
2.3.1.2 PALSAR data pre-processing
The PALSAR images with a spatial resolution of 25 m acquired in 2007, 2010, 2015, 2016, and 2019 were used for the AGB estimation. The product contains horizontal-transmit, vertical receive (HV), and horizontal transmit, horizontal receive (HH) polarization images that have been geometrically and radiometrically corrected and terrain-normalized. The digital number (DN) was converted into the dB backscattering coefficients of the HH and HV polarization components as follows:
where CF is the calibration factor. The CF for HH and HV were −83.2 and −80.2, respectively, before 2009 and −83.0 after 2009 (Shimada et al. Citation2009). An enhanced Lee filter with a window size of 5 × 5 was used to reduce the speckle noise (Jong-Sen et al. Citation2009). For most SAR data, the pixels in the 3 × 3 window may not be statistically independent due to oversampling to maintain the spatial resolution (Jong-Sen et al. Citation2009). Therefore, a 5 × 5 window size was used here. The related variables, including the HH and HV polarization values and the ratio and difference between HH and HV, were derived. All images were resampled to 30 m resolution using the nearest neighbor sampling method to be consistent with the Landsat images. All steps were implemented in the professional software The Environment for Visualizing Images (ENVI).
2.3.2 Extraction of forest phenological parameters
Zhang and Mingshi (Citation2021) developed a new method to extract the SOS from multi-source remote sensing data (Landsat and Sentinel-2) using the MCCDC model. The MCCDC produces synthetic daily cloud-free Landsat images. The MCCDC model assumes that the SR value of pixels in areas with a stable land cover changes periodically over time. Thus, the MCCDC model first determines when a land cover change has occurred in a time series. It then simulates the periodic changes before and after the land cover changes and creates daily remote sensing images from the fitted curves. The synthesized SR images do not contain clouds, shadows, and scan lines.
Zhang and Mingshi (Citation2021) also found that the land surface water index (LSWI) had a highest accuracy for extracting the SOS in this study area. Thus, a logistic regression model was used to extract the annual phenological parameters (SOS, EOS, LOS) using the LSWI in this study. When the rate of change of curvature of the logistic regression model reaches the first peak, the corresponding date (day of year, DOY) is the SOS, and when the rate of change of curvature reaches the last valley, the corresponding date is the EOS. The difference between the EOS and SOS is the LOS (Zhang et al., Citation2003). The MCCDC model was established, and the phenological parameters were extracted using the Interactive Data Language software.
2.3.3 Forest dominant tree group mapping based on the MCCDC coefficient and phenological parameters
2.3.3.1 Principles of forest dominant tree group mapping
We used cloud-free Landsat data (30 m resolution) to classify only the dominant tree species groups rather than specific tree species. After interpreting the 2016 FMPI data, we found that the dominant tree species in the study area were pine (53.52%), oak (30.20%), and walnut (8.5%). Thus, the forest was classified into four categories: pine, oak, walnut, and other dominant tree species. The spectral characteristics differed for different dominant tree species and were characterized by the MCCDC model coefficients (). Specifically, the NIR reflectance of pine was substantially different from that of the oak and walnut species (). The maximum SR of pine was generally lower than 3000, but the maximum SR of oak and walnut generally exceeded 3000. The spectral curves of walnut were different from those of the other two species in the red, SWIR1, and SWIR2 bands. Phenology can be used for dominant tree species classification because different tree species have different phenological characteristics. As shown in , walnut has an earlier SOS than pine and oak, pine has a later EOS than oak and walnut, and walnut has a longer LOS than pine and oak. We divided the study area into forest and non-forest to facilitate the classification of the dominant tree species groups. We fed the regions of interest (ROIs) and MCCDC coefficients into an SVM to classify forest and non-forest areas (Burges Citation1998). Subsequently, the forest masks from the previous step were further subdivided into different dominant tree species groups. In this study, the ROIs of the dominant tree species group samples were randomly selected from the FMPI in 2007 and 2016. Due to the lack of FMPI data in the other years, persistent forest regions were extracted from the annual forest cover map from 1999 to 2019. We assumed no change in the dominant tree species groups in these persistent forest regions. The spatial intersections of the persistent forest areas and the samples from 2007 and 2016 were used to extract ROIs for the remaining years.
Figure 4. Spectral characteristics of different dominant tree species over time characterized by the MCCDC coefficients.

The MCCDC coefficients and phenological parameters were used as input into the SVM to obtain a map of the dominant tree species groups. Finally, the classification of the dominant tree species groups was performed in the kernlab program of RStudio.
2.3.3.2 Accuracy assessment of dominant tree species groups
Here, a ten-fold cross-validation strategy was used to evaluate the classification accuracy of the model (Fushiki Citation2011). All ROIs were divided into 10 parts; nine parts were used as training data, and one part was used as validation data. This process was repeated 10 times, and majority voting was implemented to generate the final classification result and calculate the accuracy statistics of the algorithm. The accuracy was evaluated using the user’s accuracy, producer’s accuracy, and overall accuracy. The user’s accuracy refers to the ratio of correctly classified samples to the classified samples of a certain category. The producer’s accuracy represents the ratio of correctly classified samples to the number of reference samples of a certain category. The overall accuracy refers to the ratio of correctly classified samples to the samples of all categories. Additionally, the binomial proportion confidence interval, which is based on the Bernoulli trial, was used to express the uncertainty of the classification accuracy (Eq. 2) (Orawo Citation2021).
Where is the confidence interval, P refers to the estimated accuracies, including the user’s accuracy, producer’s accuracy, and overall accuracy, n is the number of validation samples. Z equals 1.96 when the confidence level is 95%.
2.3.4 Establishment of forest AGB estimation model
2.3.4.1 AGB prediction variables
The model variables included optical remote sensing and topographic variables, including SR, normalized difference vegetation index (NDVI), enhanced vegetation index (EVI), soil-adjusted vegetation index (SAVI), tasseled cap transformation, principal component analysis (PCA), texture information, elevation, slope, and aspect (Byrne, Crapper, and Mayo Citation1980; Crist, Eric, and Richard Citation1984; Haralick, Shanmugam, and Dinstein Citation1973; Huete et al. Citation2002; Huete Citation1988; Schell Citation1973). The tasseled cap transformation creates a new set of features using a linear combination of six Landsat SR bands and fixed coefficients. The first three features are brightness, wetness, and greenness, which have physical meanings in vegetation analysis (Crist, Eric, and Richard Citation1984). PCA is an orthogonal linear transformation to remove redundant information and recombine the variance contributions into newly generated components (Byrne, Crapper, and Mayo Citation1980). We also used the gray-level co-occurrence matrix and six SR bands to calculate the mean, variance, homogeneity, contrast, dissimilarity, entropy, second moment, and correlation (Haralick, Shanmugam, and Dinstein Citation1973). These operations were implemented in ENVI. Elevation data with a spatial resolution of 90 m were obtained from Shuttle Radar Topography Mission data from the geospatial data cloud website (http://www.gscloud.cn/). The elevation data were resampled to 30 m spatial resolution using the nearest neighbor resampling method. The slope and aspect tools of ArcGIS were used to obtain the slope and aspect data layers. We incorporated the MCCDC coefficients and phenological parameters (SOS, EOS, LOS) into the AGB model to improve the AGB estimation accuracy. The PALSAR data and its derivatives (HH, HV, and their difference and ratio) were also included in the AGB model ().
Table 1. Predictor variables for AGB modeling.
2.3.4.2 Selection of important variables and AGB modeling
Using redundant variables in an AGB model reduces its prediction ability (Shen et al. Citation2016). Therefore, the variable importance function of the RF package was used to rank the importance of the variables using two indicators: the percent increase in the mean square error (PercentIncMSE) and the increase in node purity (IncNodePurity) (Breiman Citation2001). The higher the PercentIncMSE and IncNodePurity are, the more important the variables are.
The important predictor variables and the matching measured AGB data were used to establish the time-consistent AGB prediction models at three-year interval in the RF package of R software. Three parameters were set in this model: 1) the ntree is the number of decision trees; it was set to 500; 2) the mtry is the number of variables at each node; it was set to one-third of the input variables; 3) the node size is the minimum number of nodes in the decision tree; we used the default value of 1.
2.3.4.3 Evaluation of forest AGB model
Similar to the evaluation of the dominant tree species groups classification, we used ten-fold cross-validation to evaluate the prediction accuracy of the AGB prediction model (Fushiki Citation2011). The results from ten outputs were averaged. The R2, root mean square error (RMSE), mean absolute error (MAE), and RMSE percentage (RMSE%) between the actual and predicted values were selected to evaluate the model performance (Eq. 3–6). In general, the larger the R2, and the smaller the RMSE, MAE, and RMSE%, the better the predictive ability of the model.
where is the measured AGB,
is the estimated AGB,
is the mean of the observed AGB values, and n is the number of samples.
3 Results
3.1 Classification accuracy and distribution of forest dominant tree species groups
The accuracy of the forest phenology estimation is shown in Appendix II. indicate that the classification accuracy of the model with the MCCDC coefficients and phenological parameters was higher than that of the spectral classification algorithm that used SR, texture information, EVI, and NDVI. A t-test was used to determine the statistically significant difference in the model prediction capability of the two methods. The differences between the left and right endpoints of the confidence intervals did not exceed 3% for all accuracies (). Thus, the MCCDC coefficients and phenological parameters were used as the optimal input variables to create the maps of the forest dominant tree species groups in the study area.
Table 2. Accuracy and confidence interval of the dominant tree species groups obtained from the model with only spectral variables in 2007 and 2016 (confidence level = 95%).
Table 3. Accuracy and confidence interval of the dominant tree species groups obtained from the model with the MCCDC coefficients and phenological parameters in 2007 and 2016 (confidence level = 95%).
shows the spatial distribution of the dominant tree species groups from 2000 to 2019. Pines were more common on the right side of the western mountains and the edge of the other mountains. Oaks occurred predominantly on the left side of the western mountain and the upper right and middle upper part of the study area. Walnuts were mainly located at the left edge of the study area. In the upper right corner of the study area, oaks were interspersed with walnuts, and other forest species were scattered at the edges and inside other forest stands.
Figure 6. Spatiotemporal distribution of the dominant tree species groups obtained from the model with the MCCDC coefficients and phenological parameters.

shows the percentage of different dominant tree species groups in different years. The area of pine forest decreased over time, except for a temp orary increase from 2007 to 2010. However, the trend of the oak forest was the opposite of that of the pine forest. The percentage of walnut forest remained unchanged at about 12%, and the percentage of other dominant tree species groups showed a downward trend.
3.2 Forest AGB validation accuracy
3.2.1 Effects of different variable combinations on AGB estimation accuracy
A total of 16 variables were selected for this study according to the order of the two indicators (Red_3, NIR, NIR_1, NIR_3, SWIR1, SWIR1_3, SWIR2_5, PCA1, PCA3, HV, HHHV, SOS, EOS, LOS, DEM, Wetness) (). Note that NIR_1 refers to the first coefficient of the MCCDC for the NIR band, and so on.
Different variable combinations were evaluated to demonstrate the influence of the MCCDC coefficients and phenological parameters on the AGB model. The RF model with only the spectral and PALSAR variables obtained an R2 of 0.52 (). In contrast, the model that included the MCCDC coefficients and phenological parameters had an R2 0.56. The RMSE was 18.62 t/ha for the first model and 14.05 t/ha for the proposed model. The MAE was slightly higher for the proposed model, and the RMSE% was 52.49% for the first model and 32.91% for the proposed model (). Therefore, incorporating the MCCDC coefficients and phenological parameters resulted in a higher accuracy of the AGB model.
Table 4. Evaluation indices of models with different variable combinations.
3.2.2 Influence of dominant tree species on AGB estimation accuracy
The results revealed the validation accuracy of the AGB model when separating tree species groups for AGB modeling. Walnut had the highest validation R2 (0.78), with relatively small RMSE, MAE, and RMSE%. The R2 values of the pine, oak, and other dominant tree species were 0.61, 0.62, and 0.65, respectively. Although the R2 of the other dominant tree species was slightly higher than those of pine and oak, the RMSE, MAE, and RMSE% of this group were the highest (24.45 t/ha, 18.62 t/ha, and 56.67%, respectively) ().
Table 5. AGB accuracy of the proposed AGB model for different dominant tree species.
presents the scatter plots of the observed and predicted AGB for all samples before and after considering the dominant tree species groups. There were significant differences in the predicted AGB between the two methods (). The R2 and RMSE were 0.56 and 14.05 t/ha in the former case and 0.67 and 12.13 t/ha in the latter case. Some low (high) AGB values were overestimated (underestimated) prior to considering the tree species, particularly when the AGB <15 t/ha and >60 t/ha. However, the differences were less pronounced when the dominant tree species were considered because the y=x line of the AGB model was closer to the trend line. This result demonstrates that each dominant tree or forest type is required for AGB model estimation.
3.3 Temporal and spatial dynamics of AGB
We used the proposed models for different dominant tree species to map the spatial distribution of AGB from 2000 to 2019. The AGB in the lower left region of the study was less than 30 t/ha, and walnut was the dominant tree species in this area () In the southeast corner of the study area, the AGB was generally lower than 30 t/ha, and pine was dominant. The AGB was relatively high (greater than 55 t/ha) on the left side, the northeast corner, and the upper middle area of the study area, and oak was the main species. In the northwest corner and the lower central region, the AGB increased from 30–55 t/ha to more than 55 t/ha from 2000 to 2019. The AGB in the southeast corner did not change substantially over time and was less than 30 t/ha ().
presents the AGB statistics for different years. From 2000 to 2019, the area percentage of AGB less than 30 t/ha exhibited a downward trend. From 2000 to 2013, the area percentage of AGB of 30–55 t/ha increased, reaching 45.83%. The area percentage of AGB of 55–80 t/ha increased from 12.54% in 2000 to 18.40% in 2019, and the area percentage of AGB of 80–105 t/ha increased from 4.19% in 2007 to 9.76% in 2016. The area percentage of AGB greater than 105 t/ha remained less than 0.8%. The area percentage of AGB of 30–105 t/ha increased, whereas that with an AGB of less than 30 t/ha decreased. These results indicate an increase in forest quality in the study area from 2000 to 2019.
shows the total AGB of different dominant tree species in different years. The total AGB of pines remained unchanged from 2000 to 2007 and decreased after 2010 to 9.57E + 06 ton in 2019. The total AGB of oak increased to 1.20E + 07 ton, except for a decline in 2010. The total AGB of walnut was lower than that of pine and oak. The total AGB of other forest species decreased from 2.93E + 06 ton to 2.39E + 06 ton from 2000 to 2007 and then remained at 2.90E + 06 ton.
4 Discussion
4.1 Effects of phenological characteristics on dominant tree species group classification
The phenological characteristics differed for different species. The SOS was generally later for pine and oak than for walnut, pine had a later EOS than oak and walnut, and walnut had a longer LOS than the other dominant tree species (). Besides, different dominant tree species exhibited different spectral response curves over time. Therefore, incorporating the MCCDC coefficients and phenological parameters into the model improved the classification accuracy of the dominant tree species groups.
As shown in , pines were the most common tree species in the study area, followed by oaks, although the area of the oak forest increased over time. Pinus yunnanensis is a native dominant tree species in southwest China, with good adaptability and drought resistance (Cha Citation2018); therefore, pines were the most widely distributed tree species. However, a single-species coniferous forest does not have high biodiversity and is easily threatened by forest insects and disease; thus, it is necessary to increase the proportion of broad-leaved trees to create more stable mixed forest ecosystems. The afforestation project has increased the proportion of oaks in recent years (https://www.docin.com/p-1263061185.html).
4.2 Advantages of estimating forest AGB based on dominant tree species and seasonal characteristics
Our results showed that the validation R2 of the AGB model was higher (0.56 compared to 0.52 for the other model) after the phenological parameters were added. Some studies have shown that global warming advances the SOS and delays the EOS, lengthening the LOS and increasing AGB (Chu et al. Citation2016; Jin et al. Citation2019). However, a delay in the SOS in our study area also increased AGB. The reason may be that the growing season was shortened, which decreased the drought duration, leading to an increase in AGB ()
Different dominant tree species have different AGB values. Our results indicated that oak species had higher AGB, while walnut species had lower AGB, even though they are both broad-leaved tree species. In addition, pine had lower AGB than oak (). Thus, separate AGB models for different forest types are better able to capture the differences in canopy characteristics between different coniferous and broadleaved forests. The validation of the AGB estimation accuracy showed that when one AGB model was used for all tree species, low AGB values (less than 15 t/ha) were overestimated, and high AGB values (more than 60 t/ha) were underestimated. The AGB model that considered different dominant tree species had a higher R2 and a lower RMSE, and the trend line approached y=x (). Thus, it is necessary to construct AGB models for different tree species.
4.3 Comparison and drivers of AGB dynamics
(Han Citation2014) estimated forest AGB using one-year Landsat TM images and found that most AGB estimates were in the range of 35–55 t/ha. In contrast, Huang et al. (Citation2013) obtained AGB estimates of 0–25 t/ha in the same area in 2005. The AGB values in our study area were 30–55 t/ha, suggesting low AGB. In addition, Cha (Citation2018) estimated that the average forest carbon stock in the Erhai basin was 39.63 t/ha and found that the order of carbon density was oak > pine > other broad-leaved trees, which is consistent with our results.
In general, the AGB in this study area was low (), which can be attributed to the wide distribution of young and middle-aged forests, poor site conditions, and ineffective forest management practices. For example, the main tree species is Pinus yunnanensis, which is managed for timber production with a short rotation cycle. Thus, frequent logging and regeneration events result in a positively skewed distribution of forest age (Cha Citation2018). Additionally, the walnut AGB was also low. The trunks are small, and the tree crowns are pruned during cultivation. In addition, the row spacing of the walnut trees is wide, resulting in a low number of walnuts per unit area. These factors are responsible for the low walnut AGB. However, the area percentage of AGB greater than 55 t/ha for oak increased from 2000 to 2019 () because of afforestation (), leading to an increase in overall forest AGB. Besides, some young oak forests shifted to middle-aged and mature forests, increasing AGB. This information can be used to learn from past operational practices and improve forest management plans to achieve sustainable development in the near future. For example, afforestation is critical for increasing forest carbon sinks. Since oak has the highest AGB per unit area, it is suggested to prioritize oak afforestation in the study area to change the forest structure, which is currently dominated by coniferous forests, and achieve a transformation from single-species to multi-species forests. Mature forests generally have a larger carbon sequestration capacity. Thus, it is necessary to promote forest succession, prevent reverse succession, and focus on cultivating mature forests. Lastly, the greater the forest volume per unit area, the greater its carbon sequestration capacity. The most effective strategy to increase forest stock per unit area is to breed more long-lived, large-diameter trees (Yan et al. Citation2016).
4.4. Research limitations and future work
First, the Sentinel-2 data were resampled to 30 m resolution to match the Landsat resolution. In this process, some non-forest pixels might be mixed with forest pixels at the edge of forest and non-forest areas, decreasing the purity of the Sentinel-2 pixels. Therefore, some mixed pixels may have resulted in spectral information that did not accurately reflect the seasonal changes of the tree species. Second, cloud-free observations are required to establish the MCCDC model. In regions with cloudy conditions in the growing season, there may be an insufficient number of cloud-free observation points, potentially reducing the fitting accuracy of the MCCDC model. Thus, these MCCDC coefficients will affect the accuracy of tree species classification and AGB estimation. For example, we obtained a relatively low producer’s accuracy of about 74% for the other dominant tree species (). There was a considerable amount of misclassification of this class. The current availability of Landsat 9 and the upcoming launch of Sentinel-2C will provide more cloud-free data, improving the applicability and accuracy of the proposed method. Third, some high AGB values (>60 t/ha) were underestimated, and low AGB values (<15 t/ha) were overestimated (), which was caused by the inadequate characterization of the forest structure, particularly the vertical structure. Using forest canopy height data obtained from lidar data to model the vertical stand structure may minimize this problem.
5 Conclusion
Incorporating phenological parameters and dominant tree species groups is considered an effective method to improve the estimation accuracy of AGB models. Therefore, this study created maps of dominant tree species groups and established a model that included features extracted from optical and radar images and phenological characteristics to improve the accuracy of forest AGB estimation. The results indicated that incorporating phenological parameters and dominant tree species data significantly improved the accuracy of estimating forest AGB. The area percentage with AGB greater than 55 t/ha increased from 2000 to 2019, and the oak AGB was the highest in the study area. The findings indicate that the forest quality level is improving. Oak afforestation should be encouraged. Another contribution of this work is that the proposed time-consistent AGB modeling strategy can be used to interpolate or extrapolate AGB estimates.
Acknowledgments
Special thanks are extended to the USGS EROS Data Center and ESA for providing the Landsat and Sentinel-2 images used in this work.
Disclosure statement
The authors declare that they have no known competing financial interests or personal relationships that could have appeared to influence the work reported in this paper
Data availability statement
The data that support the findings of this study (i.e. the land cover and probability maps) are available from the corresponding author and USGS (https://glovis.usgs.gov/).
Additional information
Funding
References
- Araza, A., S. de Bruin, M. Herold, S. Quegan, N. Labriere, P. Rodriguez-Veiga, V. Avitabile, et al. 2022. “A Comprehensive Framework for Assessing the Accuracy and Uncertainty of Global Above-Ground Biomass Maps.” Remote Sensing of Environment 272: 112917. doi:10.1016/j.rse.2022.112917.
- Bao, H., N. Quy Truong, N. Quy Khiem, K. P. Poudel, and H. Temesgen. 2022. “Deep Learning Models for Improved Reliability of Tree Aboveground Biomass Prediction in the Tropical Evergreen Broadleaf Forests.” Forest Ecology and Management 508: 120031. doi:10.1016/j.foreco.2022.120031.
- Boschetti, M., L. Boschetti, S. Oliveri, L. Casati, and I. Canova. 2007. “Tree Species Mapping with Airborne Hyper-Spectral MIVIS Data: The Ticino Park Study Case.” International Journal of Remote Sensing 28 (6): 1251–19. doi:10.1080/01431160600928542.
- Breiman, L. 2001. “Random Forests.” Machine Learning 45 (1): 5–32. doi:10.1023/a:1010933404324.
- Burges, C. 1998. “A Tutorial on Support Vector Machines for Pattern Recognition.” Data Mining Knowledge Discovery 2 (2): 121–167. doi:10.1023/A:1009715923555.
- Byrne, G. F., P. F. Crapper, and K. K. Mayo. 1980. “Monitoring Land-Cover Change by Principal Component Analysis of Multitemporal Landsat Data.” Remote Sensing of Environment 10 (3): 175–184. doi:10.1016/0034-4257(80)90021-8.
- Cao, G. 2020. “Application of Remote Sensing Technology in Forest Resources Investigation.” Remote Sensing 9: 46. doi:10.18282/rs.v9i2.1373.
- Cha, Z. 2018. “Estimation of Forest Vegetation Carbon Storage and Density in Erhai Basin of Yunnan Province.” Journal of Fujian Forestry Science and Technology 45 (4): 33–42.
- Cho, M. A., O. Malahlela, and A. Ramoelo. 2015. “Assessing the Utility WorldView-2 Imagery for Tree Species Mapping in South African Subtropical Humid Forest and the Conservation Implications: Dukuduku Forest Patch as Case Study.” International Journal of Applied Earth Observation and Geoinformation 38: 349–357. doi:10.1016/j.jag.2015.01.015.
- Chu, C., M. Bartlett, Y. Wang, F. He, J. Weiner, J. Chave, and L. Sack. 2016. “Does Climate Directly Influence NPP Globally?” Global Change Biology 22 (1): 12–24.
- CongDe, H., J. Zhang, W. Yang, X. Tang, and A. Zhao. 2008. “Dynamics on Forest Carbon Stock in Sichuan Province and Chongqing City.” Acta Ecologica Sinica 28 (3): 85–94.
- Crist, P., C. Eric, and C. Richard. 1984. “A Physically-Based Transformation of Thematic Mapper Data—the TM Tasseled Cap.” IEEE Transactions on Geoscience and Remote Sensing 3: 256–263. doi:10.1109/TGRS.1984.350619.
- Ding, Y., and J. Peng. 2018. “Impacts of Urbanization of Mountainous Areas on Resources and Environment: Based on Ecological Footprint Model.” Sustainability (Basel, Switzerland) 10 (3): 765. doi:10.3390/su10030765.
- Dwyer, J. L., D. P. Roy, B. Sauer, C. B. Jenkerson, H. K. Zhang, and L. Lymburner. 2018. “Analysis Ready Data: Enabling Analysis of the Landsat Archive.” Remote Sensing 10 (9): 1363. doi:10.3390/rs10091363.
- Fassnacht, F. E., H. Latifi, K. Sterenczak, A. Modzelewska, M. Lefsky, L. T. Waser, C. Straub, and A. Ghosh. 2016. “Review of Studies on Tree Species Classification from Remotely Sensed Data.” Remote Sensing of Environment 186: 64–87. doi:10.1016/j.rse.2016.08.013.
- Fushiki, T. 2011. “Estimation of Prediction Error by Using K-Fold Cross-Validation.” Statistics & Computing 21 (2): 137–146. doi:10.1007/s11222-009-9153-8.
- Ganjurjav, H., E. Gornish, G. Z. Hu, J. S. Wu, Y. F. Wan, Y. Li, Q. Z. Gao, and E. Lamb. 2021. “Phenological Changes Offset the Warming Effects on Biomass Production in an Alpine Meadow on the Qinghai–Tibetan Plateau.” The Journal of Ecology 109 (2): 1014–1025. doi:10.1111/1365-2745.13531.
- Han, T. 2014. Forest Biomass Estimation by Using Remote Sensing in Yunnan Province. Inner Mongolia Normal University.
- Haralick, R. M., K. Shanmugam, and I. Dinstein. 1973. “Textural Features for Image Classification.” SMC 3 (6): 610–621. doi:10.1109/TSMC.1973.4309314.
- Hill, R. A., A. K. Wilson, M. George, and S. A. Hinsley. 2010. “Mapping Tree Species in Temperate Deciduous Woodland Using Time-Series Multi-Spectral Data.” Applied Vegetation Science 13 (1): 86–99. doi:10.1111/j.1654-109X.2009.01053.x.
- Huang, K., Y. Pang, Q. Shu, and F. Tian. 2013. “Aboveground Forest Biomass Estimation Using ICESat GLAS in Yunnan,china.” Journal of Remote Sensing 01: 169–183.
- Huete, A. R. 1988. “A Soil-Adjusted Vegetation Index (SAVI).” Remote Sensing of Environment 25: 295–3009. doi:10.1016/0034-4257(88)90106-X.
- Huete, A., K. Didan, T. Miura, E. P. Rodriguez, X. Gao, and L. G. Ferreira. 2002. “Overview of the Radiometric and Biophysical Performance of the MODIS Vegetation Indices.” Remote Sensing of Environment 83 (1–2): 195–213. doi:10.1016/s0034-4257(02)00096-2.
- Huiyi, S., W. Shen, J. Wang, A. Ali, and L. Mingshi. 2020. “Machine Learning and Geostatistical Approaches for Estimating Aboveground Biomass in Chinese Subtropical Forests.” Forest Ecosystems 7 (1): 64. doi:10.1186/s40663-020-00276-7.
- Jin, J. X., X. L. Ma, H. Chen, H. Wang, X. M. Kang, X. F. Wang, Y. Wang, B. Yong, and F. S. Guo. 2019. “Grassland Production in Response to Changes in Biological Metrics Over the Tibetan Plateau.” The Science of the Total Environment 666: 641–651. doi:10.1016/j.scitotenv.2019.02.293.
- Jong-Sen, L., W. Jen-Hung, T. L. Ainsworth, C. Kun-Shan, and A. J. Chen. 2009. “Improved Sigma Filter for Speckle Filtering of SAR Imagery.” IEEE Transactions on Geoscience and Remote Sensing 47 (1): 202–213. doi:10.1109/TGRS.2008.2002881.
- Keenan, T. F., J. Gray, M. A. Friedl, M. Toomey, G. Bohrer, D. Y. Hollinger, J. W. Munger, et al. 2014. “Net Carbon Uptake Has Increased Through Warming-Induced Changes in Temperate Forest Phenology.” Nature Climate Change 4 (7): 598–604. doi:10.1038/nclimate2253.
- Lei, X. D., M. P. Tang, Y. C. Lu, L. X. Hong, and D. L. Tian. 2009. “Forest Inventory in China: Status and Challenges.” The International Forestry Review 11 (1): 52–63. doi:10.1505/ifor.11.1.52.
- Li, C., Y. C. Li, and M. Y. Li. 2019. “Improving Forest Aboveground Biomass (AGB) Estimation by Incorporating Crown Density and Using Landsat 8 OLI Images of a Subtropical Forest in Western Hunan in Central China.” Forests 10 (2). doi:10.3390/f10020104.
- Li, C., M. Y. Li, J. Liu, Y. C. Li, and Q. S. Dai. 2020. “Comparative Analysis of Seasonal Landsat 8 Images for Forest Aboveground Biomass Estimation in a Subtropical Forest.” Forests 11 (1): 45. doi:10.3390/f11010045.
- Linderman, M., J. Liu, J. Qi, L. An, Z. Ouyang, J. Yang, and Y. Tan. 2004. “Using Artificial Neural Networks to Map the Spatial Distribution of Understorey Bamboo from Remote Sensing Data.” International Journal of Remote Sensing 25 (9): 1685–1700. doi:10.1080/01431160310001598971.
- Lin, C. S., S. C. Popescu, G. Thomson, K. Tsogt, C. I. Chang, and K. P. Vadrevu. 2015. “Classification of Tree Species in Overstorey Canopy of Subtropical Forest Using QuickBird Images.” Plos One 10 (5). doi:10.1371/journal.pone.0125554.
- Lu, D., and Q. Weng. 2007. “A Survey of Image Classification Methods and Techniques for Improving Classification Performance.” International Journal of Remote Sensing 28 (5): 823–870. doi:10.1080/01431160600746456.
- Masek, J. G., E. F. Vermote, N. E. Saleous, R. Wolfe, F. G. Hall, K. F. Huemmrich, F. Gao, J. Kutler, and T. K. Lim. 2006. “A Landsat Surface Reflectance Dataset for North America, 1990-2000.” IEEE Geoscience and Remote Sensing Letters 3 (1): 68–72. doi:10.1109/LGRS.2005.857030.
- Orawo, L. A. 2021. “Confidence Intervals for the Binomial Proportion: A Comparison of Four Methods.” Open Journal of Statistics 11 (5): 11. doi:10.4236/ojs.2021.115047.
- Pötzschner, F., M. Baumann, N. Ignacio Gasparri, G. Conti, D. Loto, M. Piquer-Rodríguez, and T. Kuemmerle. 2022. “Ecoregion-Wide, Multi-Sensor Biomass Mapping Highlights a Major Underestimation of Dry Forests Carbon Stocks.” Remote sensing of environment 269: 112849. doi:10.1016/j.rse.2021.112849.
- Schell, J. A. 1973. “Monitoring Vegetation Systems in the Great Plains with ERTS.” Nasa Special Publication 351: 309.
- Shang, X., and L. A. Chisholm. 2014. “Classification of Australian Native Forest Species Using Hyperspectral Remote Sensing and Machine-Learning Classification Algorithms.” IEEE Journal of Selected Topics in Applied Earth Observations and Remote Sensing 7 (6): 2481–2489. doi:10.1109/jstars.2013.2282166.
- Shen, W. J., M. S. Li, C. Q. Huang, and A. S. Wei. 2016. “Quantifying Live Aboveground Biomass and Forest Disturbance of Mountainous Natural and Plantation Forests in Northern Guangdong, China, Based on Multi-Temporal Landsat, PALSAR and Field Plot Data.” Remote Sensing 8 (7). doi:10.3390/rs8070595.
- Shimada, M., O. Isoguchi, T. Tadono, and K. Isono. 2009. “PALSAR Radiometric and Geometric Calibration.” IEEE Transactions on Geoscience and Remote Sensing 47 (12): 3915–3932. doi:10.1109/tgrs.2009.2023909.
- Singh, M., Malhi Y., Bhagwat S. 2014. “Biomass Estimation of Mixed Forest Landscape Using a Fourier Transform Texture-Based Approach on Very-High-Resolution Optical Satellite Imagery.” International Journal of Remote Sensing 35 (9): 3331–3349. doi:10.1080/01431161.2014.903441.
- Vermote, E., C. Justice, M. Claverie, and B. Franch. 2016. “Preliminary Analysis of the Performance of the Landsat 8/OLI Land Surface Reflectance Product.” Remote Sensing of Environment 185 (Iss 2): 46–56. doi:10.1016/j.rse.2016.04.008.
- Voss, M., and R. Sugumaran. 2008. “Seasonal Effect on Tree Species Classification in an Urban Environment Using Hyperspectral Data, LiDar, and an Object-Oriented Approach.” Sensors 8 (5): 3020–3036. doi:10.3390/s8053020.
- Xie, X., Q. Wang, L. Dai, S. Dongkai, X. Wang, Q. Guang, and Y. Yujing. 2011. “Application of China’s National Forest Continuous Inventory Database.” Environmental Management (New York) 48 (6): 1095–1106. doi:10.1007/s00267-011-9716-2.
- Yang, J. B., J. C. Xu, and D. L. Zhai. 2021. “Integrating Phenological and Geographical Information with Artificial Intelligence Algorithm to Map Rubber Plantations in Xishuangbanna.” Remote Sensing 13 (14): 2793. doi:10.3390/rs13142793.
- Yan, T., Y. Peng, X. Wang, S. Kaiwen, L. Chen, W. Zheng, and H. Gong. 2016. “Forest Carbon Storage in Yunnan During 2008-2013.” Journal of Zhejiang Forestry Science and Technology 36 (2): 15–19.
- Yu, X. F., D. F. Zhuang, H. Chen, and X. Y. Hou. 2004. “Forest Classification Based on MODIS Time Series and Vegetation Phenology.” Igarss 2004: Ieee International Geoscience and Remote Sensing Symposium Proceedings, Vols 1-7, Science for Society: Exploring and Managing a Changing Planet, Anchorage, Alaska.
- Zhang X. Y., M. A. Friedl, C. B. Schaaf, A. H. Strahler, J. C. F. Hodges, F. Gao, B. C. Reed and A. Huete. 2003. “Monitoring vegetation phenology using MODIS.“ Remote Sensing of Environment 84 (3): 471–475. doi:10.1016/S0034-4257(02)00135-9.
- Zhang, Y., and L. Mingshi. 2021. “A New Method for Monitoring Start of Season (SOS) of Forest Based on Multisource Remote Sensing.” International Journal of Applied Earth Observation and Geoinformation 104: 102556. doi:10.1016/j.jag.2021.102556.
- Zhang, Y. L., W. J. Shen, M. S. Li, and Y. Y. Lv. 2020. “Integrating Landsat Time Series Observations and Corona Images to Characterize Forest Change Patterns in a Mining Region of Nanjing, Eastern China from 1967 to 2019.” Remote Sensing 12 (19). doi:10.3390/rs12193191.
- Zhu, Z., and C. E. Woodcock. 2014. “Automated Cloud, Cloud Shadow, and Snow Detection in Multitemporal Landsat Data: An Algorithm Designed Specifically for Monitoring Land Cover Change.” Remote Sensing of Environment 152: 217–234. doi:10.1016/j.rse.2014.06.012.
- Zhu, X. L., and D. S. Liu. 2014. “Accurate Mapping of Forest Types Using Dense Seasonal Landsat Time-Series.” Isprs Journal of Photogrammetry and Remote Sensing 96: 1–11. doi:10.1016/j.isprsjprs.2014.06.012.
Appendix Appendix I
Relationship between biomass and tree volume (Huang et al. 2008)
Appendix II
Scatter plots of observed and predicted SOS and EOS extracted from the EVI, NDVI, and LSWI