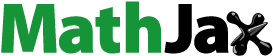
ABSTRACT
In order to control the desertification, large-scale afforestation programs have been attempted worldwide. Among them, China initiated the world’s largest afforestation program, Three-North Afforestation Program (TNAP, 1978–2050), in which the afforestation in sandy land has been questioned during the first 40 years. In fact, the contribution of the TNAP to vegetation establishment and its effectiveness in desertification control still remain unclear, which limited the further construction of the program. To answer the questions, we detected the dynamics of vegetation distribution (forest, shrubland, and grassland) and desertification status (slight, moderate, severe, and extremely severe) during 1978–2017 in the sandy land (45.5 million ha), by visual interpretation of 5-period remote sensing images with validation based on 3,100 sample plots from field surveys and 15,175 sample plots from the National Forest Resource Inventory. Vegetation degradation was identified by analysis combining the trends of net primary productivity and precipitation use efficiency. By Geographical Detector model, the driving forces of vegetation degradation (climate change, human activities, vegetation type, and sandy land type) were ranked and the contributions of the influential factors (climate change, human activities, and vegetation dynamics) to desertification changes were estimated. The results showed that for the 40 years, vegetation coverage increased by 0.5%, with increasing 113.8% and 338.8% of forest and shrubland, but decreasing 9.0% of grassland. Desertification area had little change while the overall intensity decreased. The TNAP contributed to desertification dynamics by 10.3%, which is lower than expected. Vegetation type was the dominant factor of vegetation degradation in general. Forest is less suitable for afforestation in sandy land than shrubland and grassland because of its lower stand establishment rate, higher degradation rate, and less contribution to desertification control. Thus, adjusting vegetation type to match local conditions (e.g., use shrub-land, grassland, and native species) and improving the vegetation resistance (e.g., transform monoculture forests into mixed forests, and make proper proportion for forest, shrubland, and grassland) was suggested. Our study provided specific and feasible strategies for further planning and implementation of TNAP, and references for vegetation restoration of sandy lands worldwide.
Introduction
Desertification is the most severe land degradation in drylands (including arid, semi-arid, and dry sub-humid regions) resulting from various factors, including climatic variations and human activities (UNCCD Citation1994). According to the report of The Millennium Ecosystem Assessment (Reid et al. Citation2005), desertification is one of the major environmental issues of the 21st century in drylands because it would cause environmental and socio-economic-political problems at different scales, such as soil erosion, famine, and large-scale human migrations (Geist and Lambin Citation2004). Furthermore, the world’s drylands have significantly expanded over the past 60 years (Cherlet et al. Citation2018) due to the amplification of global warming (FAO Citation2019; Landman Citation2010). Since 1980, Asia has the world’s largest increases in dryland areas, of which, China alone accounts for about one-third of the increases exposed to aridity worldwide (Prăvălie et al. Citation2019). Vegetation degradation was the most direct distinguishing feature of land degradation and desertification (Bunning et al. Citation2016). Thus, to reverse the process of desertification, large-scale ecological restoration programs have been attempted worldwide (Gnacadja Citation2015; Chazdon Citation2008; Uzuner and Dengiz Citation2020).
China has a vast area of drylands in the Three North Regions (Northwest, North, and Northeast), in which sandified land is dominant, accounting for 172.1 million ha (17.9% of China’s territory area) (National Forestry and Grassland Administration Citation2014). Sandification is one form of desertification that characterized by the land surface being covered with sand and gravel, which often occurs in arid and semi-arid regions with fragile ecological ecosystems, or in areas around the deserts. The mobile sand driven by the wind encroach arable lands and pastures, ruin residents, and cause dust storms. This problem has been exacerbated by the increasing intensity of socio-economic human activities in the recent century (Zhang and Huisingh Citation2018). In order to control the expanding of desertification, the Chinese government launched the Three North Afforestation Program (TNAP), the world’s largest ecological engineering Program in 1978 and ongoing until 2050. The TNAP covered more than 42% of China’s land territory (over 4 million km2), including most of the arid and semi-arid zones of China, in which 96.5% of the sandy land of China (119.9 million ha) is located. During the past 40 years (1978–2017), the TNAP has made significant progresses in increasing local forest coverage and improving ecological conditions of the TNAP region (Wang Citation2014; Zhu and Zheng Citation2019). However, due to the water shortage, severe climatic conditions, and infertile soils, concerns have been raised about the effectiveness of afforestation in the TNAP (vegetation establishment), especially, in the sandy land of the TNAP region (Liu Citation2008; Cao et al. Citation2011; Zhang and Huisingh Citation2018). In fact, little is known about the contribution of the TNAP to vegetation establishment, and its effectiveness on desertification control in the first 40 years, which limits our understanding of how to manage the vegetation in sandy land, and the construction of the TNAP in the next three decades.
The estimation of vegetation establishment, i.e., the existence and status of vegetation, is hard to achieve for large ecological programs in drylands, due to the dynamic growth determined by inter-annual variations in meteorological factors, heterogeneity in geographical conditions, and difficulties in sampling for large areas in long time period (Verbesselt et al. Citation2010; He et al. Citation2019). Similarly, the process of desertification is the issue that should be studied on a regional or even national scale (Geist and Lambin Citation2004; Albalawi and Kumar Citation2013). The earth observation, i.e., satellite remote sensing, provided scientifically acceptable and powerful tools in monitoring vegetation dynamics and desertification process by recording changes in ecosystems on a long time and large space scale (Lanfredi et al. Citation2015; Smith et al. Citation2019). In landscape scale, the coverage area of the existing vegetation, i.e., the vegetation quantity, is the direct indicator in assessing the outcome of afforestation, which could be obtained from the interpretation and classification of vegetation from remote sensing images (Zheng et al. Citation2012; Zheng and Zhu Citation2017; Gerlein-Safdi et al. Citation2020). When assessing the vegetation status, i.e., the vegetation quality, the ecosystem structure, and function are the two aspects most concerned about (Smith et al. Citation2019). Based on remote sensing methodology, the vegetation index of net primary productivity (NPP) was considered to be more suitable for estimation of biomass (i.e., ecosystem structure) for sparse vegetation than other indices, such as normalized difference vegetation index (NDVI) (Anyamba and Tucker Citation2005; Sun et al. Citation2019). Since water is the limited condition in arid and semi-arid ecosystems, the ability to use available precipitation (PUE, i.e., precipitation use efficiency) is an apt feature in ecosystem functional assessment (Houerou Citation1984; Bai et al. Citation2008). Thus, as an integral method for estimating vegetation status (Li, Wei, and Zhou Citation2015), the combined analysis of NPP and PUE is especially suitable for identifying vegetation degradation in drylands.
As a process with distinct ground surface features, desertification could be identified through visual interpretation of remote sensing images with acceptable accuracy (Yan et al. Citation2009; Yan et al. Citation2015; Albalawi and Kumar Citation2013). However, the explanation for decades of desertification in spatial is a challenge. Over the years, previous studies have suggested that desertification is caused by several human factors (e.g., human activities, population dynamics, and agricultural policies) and natural factors (e.g., climatic change, soil type, and vegetation cover) (Du et al. Citation2017; Feng et al. Citation2015; Jiang et al. Citation2019). On that base, the contributions and the causal pattern of the factors are found to be specific to each locality (Geist and Lambin Citation2004; Yan et al. Citation2015; Sayl et al. Citation2021). Except for the irreducible complexity of desertification process, this finding also results from the differences in the methods of attribution (e.g., linear regression analysis and path analysis) and the difficulties in analyzing mixed types of data representing various factors (e.g., categorical data and continuous data). The model of Geographical Detector has been designed for quantifying the relative importance of several factors to response variables, free of the assumption of linear relationship of variables (Du et al. Citation2016; Wang et al. Citation2010). Based on the measurement of spatially stratified heterogeneity, the model can analyze mixed data types, including categorical data and continuous data (Cao, Ge, and Wang Citation2013; Wang, Zhang, and Fu Citation2016; Wang and Hu Citation2012). Having been successfully applied in the attribution analysis of vegetation dynamics at various scales (Zheng et al. Citation2021; Zhao et al. Citation2020; Zhang et al. Citation2021; Ran et al. Citation2019; Du et al. Citation2017), Geographical Detector is considered suitable for detecting the relative importance of vegetation to desertification dynamics among the other natural and human factors, and dominant drivers of vegetation degradation in sandy land of TNAP.
In this study, the study area was determined by extracting the sandy land from the dataset of Distribution of Desert (Sandy Land) in China (Wang et al. Citation2005) by excluding the desert (i.e., contiguous mobile sandy land and semi-mobile sandy land, referring to the high-resolution images provided by Google Earth) in the TNAP region, which is considered as the potential land for afforestation or vegetation restoration (45.5 million ha). Vegetation dynamics and desertification status were monitored and assessed by the interpretation of five periods of remote sensing images from 1978 to 2017 with the plot data of national inventory (National Forestry Administration Citation2000; National Forestry and Grassland Administration Citation2014, Citation2019, Citation2000; Xiao Citation2005; Jia Citation2009; Li et al. Citation2019) and field survey. Time-series data of vegetation biomass, climate, and human activities were used for studying the potential problems of existing vegetation and their driving forces. The specific objectives of this study were: (1) to display the vegetation dynamics both quantitatively and qualitatively; (2) to find the problems in present vegetation establishment and their driving forces; (3) to study the desertification processes and their relationship with the vegetation dynamics, climate changes and human activities, particularly the contribution of the TNAP to control the desertification processes. By achieving these objectives, specific and feasible strategies for further planning and implementation of TNAP are provided. References to vegetation restoration of sandy lands worldwide, especially in large scale and long term, are put forward.
Materials and methods
Study area
The study area was extracted by overlaying the distribution regions of sandy land and desertified land within the TNAP region (). The sandy land was obtained from the Dataset of the Distribution of Desert (Sandy Land) in China (1:100,000) (Wang et al. Citation2005). The sandy land and deserts are mainly located in the northern provincial administrative units, including Xinjiang, Qinghai, Tibet, Ningxia, Inner Mongolia, Gansu, Shaanxi, Shanxi, Hebei, Beijing, Henan, Jilin, and Liaoning. The four main sandy lands (i.e., Hulunbuir Sandy Land, Otindag Sandy Land, Horqin Sandy Land, and Mu Us Sandy Land) of China are distributed in the east of Northern China. In the northwest, there are the eight main deserts of China (i.e., Taklimakan Desert, Gurbantünggüt Desert, Qaidam Basin Desert, Tengger Desert, Badain Jaran Desert, Kubuqi Desert, Ulan Buh Desert, Kumtag Desert) that consist of semi-mobile and mobile sandy land (Zhang and Huisingh Citation2018) (). The TNAP region is located in the Northeast, North, and Northwest of China, including the majority of sandy land and desertified land in China, in which the vegetation restoration is implemented for desertification control ().
Figure 1. Spatial distribution of study area. (a) shows the study area, consisted of sandy land and desertification area around the deserts in the TNAP; (b) shows all the sandy land and desert in the TNAP region (the four major sandy lands of Horqin Sandy Land, Otindag Sandy Land, Hulunbuir Sandy Land, and Mu Us Sandy Land are marked as I~Ⅳ, and the eight major deserts of Taklimakan Desert, Gurbantünggüt Desert, Kumtag Desert, Tengger Desert, Ulan Buh Desert, Kubuqi Desert, Qaidam Basin Desert, and Badain Jaran Desert are marked as Ⅴ~VII); and (c) shows the distribution of desertified land in the TNAP region.

The construction scope of the TNAP generally varied in different construction periods, and in the current period the TNAP has a total area of 435.8 million ha. To examine the interaction between vegetation restoration and desertification processes, the core area of deserts was excluded from the study area because the area is covered by stable desert. As a result, our study area covered 45.5 million ha, accounting for 4.7% of China’s land territory and 36.6% of total sandy land (124.2 million ha) of China (). According to the China’s Meteorological Background Dataset (Xu and Zhang Citation2017), the mean value of annual average temperature of the study area was 4.5°C (ranged between −6.6°C and 15.7°C), and the mean value of annual precipitation was 234 mm (ranged from less than 50 mm to around 470 mm). The original main natural vegetation types include desert steppe, typical steppe, meadow steppe, shrubland, and forest (Hou Citation2001; Fang et al. Citation2002; Su et al. Citation2020).
Data collection
The TNAP started in 1978. Remote sensing images of five time-points (1978, 1990, 2000, 2010, and 2017) were used to detect the quantity and spatial dispersion of vegetation and the status of desertification, representing the initial situation of vegetation and desertification before the TNAP, and the situation after 10, 20, 30, and 40 years’ implementation of the TNAP, respectively. Then the vegetation dynamics and desertification were analyzed in four periods (1978–1990, 1990–2000, Citation2000–2010, and 2010–2017). All the datasets used in this study are listed in .
Table 1. Overview of the datasets used in the study.
Remote sensing images
Five sets of remote-sensing images from 1978 to 2017 were selected, with Landsat TM images (30-m spatial resolution) acquired in 1990, 2000, 2010, and 2017, and Landsat MSS images (80-m spatial resolution) acquired in 1978, totaling 785 scenes (157 for each time point). The high-resolution images, i.e., SPOT 5 images in 2008 (2.5-m spatial resolution) and GF-1 images in 2017 (2-m spatial resolution), were used to improve the accuracy of vegetation monitoring and provide complementary information for the Landsat images, as in the previous studies of the TNAP (Yan et al. Citation2011; Zheng et al. Citation2012; Zheng and Zhu Citation2017), totaling 279 scenes and 1094 scenes, respectively. All the images were acquired between the end of June and mid-September, for the time period is the growing season of the local vegetation, and the images during the time period are apt for extracting the vegetation information (Zheng and Zhu Citation2017). To ensure the use of high-quality images (i.e., cloud free, good radiometric quality, low contents of aerosols, and high solar elevation angles), this study used the images of the previous year or the next year if the images of a specific year did not meet the requirements.
Satellite-based vegetation dataset
The spatial annual Net Primary Productivity (NPP) data from 1981 to 2017 were extracted from the Global 5 km 8-day Total and Net Primary Productivity Products (Cui et al. Citation2016; Yu et al. Citation2018; Wang et al. Citation2020). The dataset was produced by the light energy utilization model of MuSyQ-NPP based on 5-km resolution GLASS (Global Land Surface Satellite) LAI and FPAR products, and ERA-Interim meteorological data products. The model was improved by introducing a sky index, which could provide more accurate calculation of light use efficiency. The dataset was produced under the funding of the National Key R&D Program Project “Stereoscopic Observation and Inversion of Key Parameters of Global Ecosystem Carbon Cycle” and was verified by data from FLUXNET sites (http://www.resdc.cn).
Meteorological dataset
The meteorological data included moisture index, annual precipitation, and annual average temperature. The data of moisture index were obtained from the China’s Meteorological Background Dataset (Xu and Zhang Citation2017). The annual precipitation and annual average temperature from 1981 to 2017 were extracted from the 1 km monthly temperature and precipitation dataset (Peng et al. Citation2019). This dataset is downscaled in China through the Delta space downscaling scheme based on the global 0.5°Climate dataset provided by the Climatic Research Unit (https://crudata.uea.ac.uk/cru/data/hrg/) and the global high-resolution climate dataset released by the WorldClim (http://www.worldclim.org/), with verification from 496 independent meteorological observation stations throughout China.
Other dataset
The dataset of Distribution of Desert (Sandy Land) in China (1:100,000) (Wang et al. Citation2005) were used to extract the spatial range of sandy land. The local population and gross domestic product (GDP) were used as socioeconomic indicators, extracting from the China’s population and GDP spatial distribution dataset (1 km grid) (Xu Citation2017b, Citation2017a). The spatial distribution data of terrestrial ecosystem types, derived from the China’s Multi-Period Land Use Land Cover Remote Sensing Monitoring Dataset (CNLUCC) (1 km grid) (Xu et al. Citation2018), were used as a reference for the interpretation of remote sensing images. Data including National Forest Resource Inventory (the 5th to 9th) (National Forestry Administration Citation2000; National Forestry and Grassland Administration Citation2014, Citation2019, Xiao Citation2005; Jia Citation2009), Bulletin of National Desertification and Sandification Monitoring (the 1st to 5th) (National Forestry Administration Citation2000; National Forestry and Grassland Administration Citation2006, Citation2009, Citation2014; Li et al. Citation2019), national statistical data (construction/assessment reports of the TNAP and the Second National Land and Resources Survey Data), national permanent sample plot data (monitoring of ecosystem status and desertification, updated every five years), and field survey, were used to verify the interpretation results.
Field survey
The field surveys were carried out in the study area from June to September during 2007–2018 for investigation of vegetation and desertification status (Zheng et al. Citation2012; Zhu, Zheng, and Yan Citation2016; Zheng and Zhu Citation2017). 36033 Global Navigation Satellite System (GNSS) points were marked for interpretation and validation in the 375,165 km long survey route, with the minimum interval distance of 1 km. Land cover information, including vegetation type, vegetation coverage, and desertification intensity, were obtained by 3,100 sample plots, with each sample covering 900 m2 of land area. The long-term monitoring data of the National Forest Resource Inventory (updated every five years) from 15,075 sample plots within the study area were used for the supplementary information of vegetation type, vegetation coverage, and vegetation health status, with each sample covering 667 m2 of land area.
Classification of woody-grass vegetation and desertification
Image preprocessing
The 1:100,000 scaled digital topographic maps and other thematic maps (e.g., regional land-use, desertification, and vegetation maps) were used for geometric correction of the satellite images. All images were projected in Albers equal area conic projection, with geo-referenced and orthorectified by 70 to 80 uniformly distributed ground control points (GCPs) for each image. The root-mean-square error (RMSE) for the geometrical rectification using image-to-image matching method was at 1 to 2 pixels (i.e., <60 and <160 m for TM images and MSS images, respectively). All image data were processed by using an ERDAS 9.1 image processing system.
Visual interpretation and classification
The spatial distribution of vegetation and desertification at each time point were derived by the visual interactive interpretation method from the standard false-color images were composited by near-infrared band, red band, and green band (R, G, B) for Landsat MSS and TM remote sensing images (Yan et al. Citation2009; Zheng et al. Citation2012). Following the classification scheme developed in the previous studies of the TNAP (Zheng et al. Citation2012; Zheng and Zhu Citation2017), the vegetation of the study area was classified into five vegetation types at each time point (i.e., 1978, 1990, 2000, 2010, and 2017): coniferous forest, broadleaved forest, mixed conifer-broadleaved forest, shrubland, and grassland (Appendix 1). Among the vegetation types, the three arbor forest types and shrubland make up the planted forests in sandy land. Four intensity levels of desertification were identified from the false-color images, including slight desertification, moderate desertification, severe desertification, and extremely severe desertification (Yan et al. Citation2009; Zhu, Zheng, and Yan Citation2016), according to the classification system in . The land cover types (i.e., orchard, farmland, residential land, and waters) that were not considered in this study were included into a type named other land for statistical convenience. The vegetation types and desertification status were allocated manually according to the visual texture, color, and location of the patches in the composed images referring the natural vegetation maps and CNLUCC dataset (Zheng et al. Citation2012; Yan et al. Citation2009).
In the process of visual interpretation, the adopted mapping principles were listed as follows. First, the minimum map patch size was 6 pixels × 6 pixels, equivalent to 180 m × 180 m on the ground. Second, the positioning error was one pixel on the screen, equivalent to 2 m, 2.5 m, 30 m, and 80 m on the ground in the GF-1 images, the SPOT 5 images, the TM image, and the MSS images, respectively. Third, the classification results of vegetation type and desertification status were validated by the field investigation data, the National Forest Resource Inventory data, and other materials (e.g., regional land-use, desertification maps, and high-resolution maps from Google Earth), of which the overall accuracy was higher than 95% (Zhu, Zheng, and Yan Citation2016).
Calibration of vegetation quantity
Since the resolution of Landsat TM image is 30 m, the vegetation patch with areas less than 32,400 m2 (by the mapping requirement at 1:100,000) was unable to be extracted. In order to improve the accuracy in estimation of vegetation quantity based on Landsat TM images, an area calibration method was put forward. Nine SPOT 5 images were randomly selected, of which the overlapped area with Landsat TM was split by plots with the size of 1 km × 1 km. In each plot, the vegetation area obtained from Landsat TM (i.e., medium-resolution) and by SPOT 5 (i.e., high-resolution) were extracted. Considering the variations in natural conditions across the study area, the plots were divided into two groups, i.e., annual average precipitation larger than 303 mm versus that lower than 303 mm (Zhu, Zheng, and Yan Citation2016). In each group, 80% of plots were used for the linear regression between the vegetation area obtained from Landsat TM and that by SPOT 5, and the other 20% were used for the verification. The accuracy of calibration was estimated as:
where Pr (%) is the accuracy, xe is the calibrated value of vegetation area obtained from Landsat TM (m2), x0 is the vegetation area obtained from SPOT 5 (m2), and n is the number of plots. The calibration relationship between vegetation area values extracted from SPOT 5 and Landsat TM used in this study is shown in .
Estimation of woody-grass vegetation quality
Calculation of precipitation use efficiency
Precipitation use efficiency (PUE) is a key indicator to assess vegetation status of dryland ecosystems, and has been widely used in studies on vegetation degradation and desertification (Houerou Citation1984; Hein and De Ridder Citation2006; Zhao et al. Citation2019). PUE is defined as the ratio between annual net primary production and annual precipitation, representing the ability of a vegetation system to convert precipitation water into vegetation (Prince, Brown De Colstoun, and Kravitz Citation1998). So, a decline in PUE means reduction in the capacity of the vegetation to transform water and nutrients to biomass, which indicates the vegetation degradation occurrence (Houerou Citation1984; Snyman Citation1998; Li, Wei, and Zhou Citation2015). In this study, the value of PUE for each time point was calculated as:
where PUE is the rain-use efficiency (g/m2 mm) for each pixel, NPP (g/m2) is the average annual net primary productivity, and P (mm) is the annual precipitation.
Detection of the trends of NPP and PUE
The trend analysis was conducted for NPP and PUE in the four periods by acquiring the slope coefficient of linear regression at each pixel (1 km × 1 km). The slope coefficient is calculated as:
where i is the number of the year in the period, and n is the range of the period, and represents the value of NPP or PUE at the ith year.
The significance of the slope was tested by the t-statistic. According to the outcome of the t-statistic, the pixels were classified into three categories: significantly increasing group (Slope >0, p < 0.25), significantly decreasing group (Slope <0, p < 0.25) and not significant group (p ≥ 0.25), referring to the method of Li et al. (Li, Wu, and Huang Citation2012). The trend of NPP and PUE was detected by four periods (i.e., 1978–1990, 1990–2000, Citation2000–2010, and 2010–2017).
Identification of vegetation quality types
Both NPP and PUE are considered to be effective factors to illustrate the vegetation degradation of arid and semi-arid ecosystem from different aspects (Prince, Brown De Colstoun, and Kravitz Citation1998; Hein and De Ridder Citation2006, Citation2006), and could be combined to improve the assessment of vegetation situation (Li, Wei, and Zhou Citation2015). In this study, degradation was detected by combining temporal change trends of NPP and PUE for the four periods, including 1978–1990, 1990–2000, Citation2000–2010, and 2010–2017. Based on the changes in both NPP and PUE, the existing vegetation was categorized into four quality types: severe degradation, potential degradation A, potential degradation B, and no degradation (). For the two time points of Time 1 and Time 2, during the period between Time 1 and Time 2, significant decreases in both NPP and PUE indicated the vegetation degradation in both structure and function, which can be considered to be in a situation of severe degradation (). If NPP decreased while PUE increased or did not significantly change, the situation is considered as potential degradation A (). Under this scenario, total vegetation biomass decreased, but the vegetation self-recovery is possible because the function of the ecosystem did not decrease. The scenario that NPP increased or had not significant change but PUE decreased significantly is categorized into potential degradation B because the function of the ecosystem was actually deteriorating (). The situation that neither NPP nor PUE has a significant trend was divided into potential degradation B or no degradation. All the other situations, i.e., neither PUE nor NPP showing a significant decrease trend, are categorized into no degradation. showed one typical situation in which the structure and function of vegetation was improved. The outcome of the vegetation quality type identification was assessed by the National Forest Resource Inventory data, of which the overall consistency was around 80% ().
Figure 2. Four vegetation quality types identified based on the trends of NPP (net primary productivity) and PUE (precipitation use efficiency). The four vegetation quality types are (a) severe degradation, (b) potential degradation A, (c) potential degradation B, and (d) no degradation. The figure was adapted from the figure of Li, Wei, and Zhou (Citation2015).

Statistical analysis
Spatiotemporal change of woody-grass vegetation
The land cover transition matrix () was used to measure the spatiotemporal dynamics of vegetation during the four periods, i.e., 1978–1990, 1990–2000, Citation2000–2010, and 2010–2017. Time 1 and Time 2 refer to the starting and end points, respectively. is the area of the land belonging to Type i in Time 1, but transfers into Type j in Time 2. If i equals to j, the land cover type remained unchanged from Time 1 to Time 2.
and
are the total area of the Type n in Time 1 and Time 2. During the period of Time 1 to Time 2, the decrease area of Type n equals to
, and the increase area of Type n is
.
Table 2. Land cover transition matrix during period of Time 1 - Time 2. This table was adapted from the table of Wang et al. (Citation2019).
Based on the transition matrix, net change area (NC), swap change area (SC), and total change area (TC) were calculated for the vegetation types. NC is the difference between the increase area and decrease area during the starting and end time points (Equation (4)).SC is the area of land that transferring into and out of one vegetation type (Equation (5)), which reflects the real change area during the process. TC is the sum of change area of one vegetation type during the period (Equation (6)). For vegetation type n, NC, SC, and TC were calculated as (Pontius, Shusas, and McEachern Citation2004; Wang et al. Citation2019):
Geographical detector model
Geographical Detector Model (GeoDetector) was applied in the attribution analysis. GeoDetector is a method developed for exploring the relationships between landscape spatial patterns and their impact factors. It is a statistical tool for spatial variance analysis based on the measurement of spatial stratified heterogeneity (SSH), i.e., the phenomena that are within strata are more similar than are between strata (Wang et al. Citation2010; Wang and Hu Citation2012; Guo et al. Citation2022). Except that the assumption of linear relationship of variables is not required, GeoDetector is especially apt for analyzing mixed data types including categorical data and continuous data (Du et al. Citation2016; Liang and Yang Citation2016). The power of determinant (PD) of a certain factor is defined as:
where is the power determinant of the explanatory variable to target variable,
is the strata number of the target variable stratified by the explanatory variable,
and
are the total number of samples and the variance of target variable, respectively,
and
denote the number of samples and the variance of target variable in the same stratum, respectively.
ranges from 0 to 1, representing that the explanatory power of an explanatory variable to the target variable goes from weak to strong. When
= 1, the target variable is completely dependent on the explanatory variable.
In this study, six factors were taken as potential explanatory variables into the driving factor analysis of vegetation degradation, including the trend of annual precipitation, the trend of annual average temperature, vegetation type, sandy land type, the change of local population and GDP, representing natural conditions and human activities. The six potential explanatory variables were calculated for the 10-year periods (i.e., 1990–2000, Citation2000–2010, and 2010–2017), in order to correspond to the results of vegetation quality types. In analyzing the driving forces for desertification process, area change for each vegetation type, the trend of annual precipitation and annual average temperature, the change of local population and GDP between 1978 and 2017 were included as explaining factors, with area change of each desertification intensity level as the target variable.
All the continuous factors (i.e., the trend of annual precipitation and annual average temperature, the change of local population and GDP, and the changes in area of vegetation and desertification) were discretized into nine levels by the quantile method in ArcGIS 10.4 for Desktop before putting into GeoDetector, because only discrete format explanatory variable data were applicable in the GeoDetector algorithm (Cao, Ge, and Wang Citation2013; Wang, Zhang, and Fu Citation2016; Liang and Yang Citation2016). The sample grid size for discretization in the driving factor analysis of vegetation degradation was set to be 1 km2 to meet the resolution of majority of data. While 10 km2 was taken as the sample grid size in analyzing the driving factors to desertification process, mainly considering that vegetation can affect the desertification process in a certain range around it. The GeoDetector software was downloaded freely from the website of http://www.geodetector.cn. The discretization and format transformation of the factor data were done by ArcGIS 10.4.
Results
Vegetation dynamics in sandy land during 1978 to 2017
The total area of vegetation in the study area increased from 30.9 million ha in 1978 to 31.2 million ha in 2017, with the coverage rate changing from 68.0% to 68.5% ( and ). Due to the afforestation programs (TNAP), forest, and shrubland increased by 113.8% (from 0.3 million ha to 0.7 million ha) and 338.8% (from 0.8 million ha to 3.3 million ha), respectively, during 1978 –2017. By contrast, grassland decreased by 9.0% (from 29.9 million ha to 27.2 million ha). Grassland was the dominant vegetation type in the study area at all time points, followed by shrubland and forest. Of the three forest types, broadleaved forests had the largest area, while conifer-broadleaved mixed forests had the smallest area.
Figure 3. Spatial distribution and statistic results of each vegetation type during 1978 to 2017. The spatial distribution of forest, shrubland and grassland in (a) 1978, (b) 1990, (c) 2000, (d) 2010, and (e) 2017 are shown. Each bar in (f) represents the area of the specific vegetation type of the year.

Vegetation type changes during the four periods of 1978–1990, 1990–2000, Citation2000–2010, and 2010–2017 were detected by the transition matrix (). For all vegetation types, the increase area was generally greater than the decrease area in the first three periods (1978–1990, 1990–2000, and 2000–2010), but contrary pattern was found during 2010–2017. Broadleaved forests had the largest positive net change area in the past 40 years among the three forest types, while mixed conifer-broadleaved forest was the only forest type that had a negative net change area. Shrub-land had the largest positive net change area during 2000–2010, but the largest negative net change area occurred during 2010–2017, resulting the positive net change area in the 40 years was much less than the total change area. Compared with the other three periods, the swap change area during 2010–2017 was the largest, indicating frequent and wide changes happened in vegetation during this period.
The distribution and proportion of the four vegetation quality types showed significant difference among the time periods ( and Appendix 7). The overall degradation rate of forest and shrubland was 17.4% during 1978–1990, peaked at 40.5% during 2000–2010, and reduced to 25.3% during 2010–2017. For the forests, the degradation rate increased from 18.1% during 1978–1990 to 76.1% during 2000–2010, and reduced to 49.7% during 2010–2017. During 1990–2000, potential degradation A (degradation in structure) was the major degradation type, accounting for 23.7% of the total forest area (including 7.0 × 104 ha of coniferous forests, 4.4 × 104 ha of broadleaved forests, and 0.6 × 104 ha of mixed conifer-broadleaved forests). In the next period, i.e., 2000–2010, potential degradation B (degradation in function) and severe degradation (degradation in both structure and function) were the two degradation types that happened most to the forests, accounting for 48.7% (including 8.3 × 104 ha of coniferous forests, 16.6 × 104 ha of broadleaved forests, and 1.2 × 104 ha of mixed conifer-broadleaved forests), and 16.3% (including 5.6 × 104 ha of coniferous forests, 2.8 × 104 ha of broadleaved forests, and 0.1 × 104 ha of mixed conifer-broadleaved forests) of total forest area, respectively ( and Appendix 7).
Figure 4. Spatial distribution and statistic results of vegetation quality types for each vegetation type in four periods during 1978 to 2017. The spatial distribution of vegetation quality types for forest, shrubland and grassland during (a) 1978 to 1990, (b) 1990 to 2000, (c) 2000 to 2010, and (d) 2010 to 2017 are shown. Four vegetation quality types were identified, including severe degradation, potential degradation A, potential degradation B and no degradation. Each bar in (e) represents the proportion of area of the specific vegetation quality type of the period for each vegetation type.

The degradation rate of shrub-land fluctuated in the 40 years, changed from 17.1% during 1978–1990 to 24.6% during 1990–2000, peaked at 35.0% during 2000–2010, and reduced to 20.5% during 2010–2017 ( and Appendix 7). Similar to those of forests, the dominant degradation type during 1990–2000 was potential degradation A, accounting for 10.8% of the total shrub-land area. During 2000–2010, the proportion of severe degradation shrubland did not increase significantly like the forests. Potential degradation A and potential degradation B were the two major degradation types during the time, accounting for 9.7% and 21.4% of the total shrub-land area, respectively. The results showed that for forest and shrubland, a high proportion of potential degradation B would happen following a high proportion of potential degradation A happened, indicating that the degradation in structure could result in the degradation in function in the following years ( and Appendix 7).
Driving forces of vegetation degradation
The six potential driving forces affecting vegetation degradation were ranked according to their PD values (the power of determinant, ). Vegetation type was the most important factor in the periods of 1990–2000 and 2000–2010, and the second most important in the period of 2010–2017. The highest rank of human activities factors, represented by the change of GDP (gross domestic product) and population, was 2nd, 2nd, and 3rd during the three periods, respectively. Although the PD of temperature change was ranked as 1st during 2010–2017, the climate change factors were less influential than human activities in general (0.068 + 0.067 < 0.097 + 0.033 during 1990–2017). Sandy land type, i.e., the site conditions, had significant impacts on the vegetation degradation, although it was not ranked high.
Table 3. PD values and ranking of potential driving factors to vegetation degradation for period of 1990–2000, Citation2000–2010, and 2010–2017. PD values represent the percentage that each factor could explain the distribution of vegetation quality types in spatial in each time period.
Desertification dynamics in sandy land during 1978 to 2017
Changes in desertification area and intensity
The total area of desertification was 24.9 million ha in 1978, peaked at 29.2 million ha in 2000 with an increase rate of 17.2% during 1978–2000, and reduced to 28.7 million ha in 2017 with a decrease rate of 1.5% during 2000–2017 ( and Appendix 8). From 1978 to 2017, the area of all intensity levels of desertification generally increased with the time, except for extremely severe desertification, whose area decreased continuously after 2000. The proportion of slight desertification and moderate desertification to that of severe desertification and extremely severe desertification was 0.495: 0.505 in 1978, changed into 0.461: 0.539 in 2000, and became 0.551: 0.449 in 2017.
Figure 5. Spatial distribution and statistic results of desertification with intensity levels during 1978 to 2017. The spatial distribution of each desertification intensity levels in (a) 1978, (b) 1990, (c) 2000, (d) 2010, and (e) 2017 are shown. Each bar in (f) represents the desertification area of the specific intensity level of the year.

Driving forces of desertification dynamics
Seven factors were examined for their contribution to the desertification dynamics by PD values (). Change of vegetation area could explain 36.7% of the desertification dynamics during 1978–2017, while climate change and human activities explained by 12.5% and 25.3%, respectively. Of the vegetation factors, forest, shrubland and grassland explained 3.1%, 7.2%, and 26.4% of the desertification dynamics, respectively. Area changes at all intensity levels of desertification could be significantly explained by changes in forest, shrubland, and grassland areas. Grassland had the largest PD in all vegetation types for all intensity levels of desertification. For slight and moderate desertification, forest had similar explanation power than shrub-land (1.6% versus 1.5%). However, for severe and extremely severe desertification, the explanation power of forest was less than shrubland (2.7% versus 4.6%). Climate change and human activities had different explanation power in the desertification area changes for each intensity level (). Precipitation change explained the changes in slight and moderate desertification more than in the other two desertification intensity levels (4.5% versus 1.4%), while temperature change had the similar explanation power for all intensity levels of desertification (3.4% versus 3.3%). Human activities explained the area changes of desertification in slight and extremely severe levels (7.7% and 9.9%) more than the other two intensity levels (4.1% and 3.7%).
Table 4. PD values (%) of vegetation area change, climate change, and human activities to desertification dynamics during 1978 to 2017. PD values represent the percentage that each factor could explain the distribution of vegetation quality types in spatial in each time period.
Discussion
The impact of TNAP on vegetation restoration in sandy land of China during 1978 to 2017
Desertification is a combined consequence of multiple driving forces (Geist and Lambin Citation2004). The harsh natural conditions and the complex interactions between ecosystems and human activities in the sandy land of northern China present a challenge in the vegetation restoration through large-scale ecological programs (Zhang and Huisingh Citation2018). Our findings implied that vegetation restoration was not as effective as expected in the sandy land where the TNAP is implemented, since the vegetation coverage did not increase during the past 40 years (68.0% in 1978 and 68.5% in 2017). This is because the increase in forest and shrub-land by the TNAP is almost offset by the reduction of grassland. As reported by many previous studies, the low survival rate of the afforestation in the sandy land was believed to be one of the major causes for the unsatisfactory outcome of vegetation restoration (Wang et al. Citation2010; Wang et al. Citation2010; Cao et al. Citation2011). Stand establishment rate is another commonly used index in assessing the effectiveness of afforestation, which is the ratio of the actual increase area of forest or shrubland to the area of the newly planted forest or shrub-land that survived within 3–5 years. It was found that the establishment rates of the TNAP in sandy land was about 21.8% and 49.2% within 10 years, and decreased to 11.5% and 33.7% during the 40 years, respectively, for forest and shrubland. This indicates that only 1/5 of the survived forests became stands within 10 years of afforestation, and only 1/10 of the forests are still alive by 2017. In contrast, for the shrubs survived after afforestation, nearly half of them successfully became shrubland after 10 years, and more than 1/3 of the shrubland still exists by 2017. This suggests that the forest and shrubland suffered serious degradation consistently, but the shrub-land showed better adaptation than forest.
The increase of forest and shrubland area was limited not only due to the low establishment rate, but also due to the high degradation rate. It is obvious that the low quality or the degradation problem of the survived planted forests limited the functions of the ecological engineering projects (Li Citation2004; Zheng et al. Citation2012). It was found that the problem of vegetation degradation has always existed in sandy land during the construction of the TNAP. The degradation rate for forest and shrubland generally increased in the first 30 years, but decreased suddenly during 2010–2017. The possible reasons for this result might include: (1) the degraded forest and shrubland in 2000–2010 possibly died out during 2010–2017, as the decrease in area of both forest and shrub-land, during 2010–2017 was largest in all the periods. (2) There are likely some uncertainties in the assessment of degradation rates during 2010–2017, for the precipitation fluctuated more seriously in this period and the length of time for statistics was shorter than the other 3 periods. Therefore, the actual degradation rates during 2010–2017 may be larger than the calculated degradation rates in this study.
Among the factors that potentially caused the vegetation degradation in the study area, besides the mismatch between vegetation type and local site conditions, the socioeconomic factors were the dominant ones, except for the period when climate changed drastically (i.e., 2010–2017). According to Feng et al.’s study (Citation2015), socioeconomic factors influenced more than environmental factors in NDVI decreasing and desertification increasing in China from 1983 to 2012. In previous studies conducted within the TNAP region, e.g., Altay Prefecture and Inner Mongolia, human disturbance was also proved to be the main factor responsible for vegetation degradation in the recent decades (Zhang et al. Citation2019; Sun et al. Citation2019). However, climate change became more influential than human activities in period with severe climate changes. With the dryer and warmer trend of climatic conditions in the northern China (Li, Chen, and Li Citation2019; Prăvălie et al. Citation2019), the meteorological factors would trigger more serious vegetation degradation in the future.
The contribution of TNAP in desertification changes in sandy land of China during 1978 to 2017
Through the construction of the TNAP, the desertification process during 1978–2017 in the sandy land was controlled to some extent, but the impact of the TNAP was very limited. Although the desertification area showed a decreasing trend after 2000, there was a net increase in the past 40 years. The overall intensity of desertification decreased, with slight and moderate desertification became dominant. The change trend of desertification area observed in this study was consistent with the conclusion from the 5th Bulletin of National Desertification and Sandification Monitoring (National Forestry and Grassland Administration Citation2014).
The limited impact of the TNAP to desertification control was mainly due to the lower than preserved rate of forest and shrub-land (i.e., low survival rate and low stand establishment rate), and decrease in the area of grassland. The major determinants of the desertification dynamics in the study area during 1978–2017 was vegetation area change. The area changes of forest and shrubland explained 10.3% (3.1% and 7.2%, respectively) of the desertification dynamics, which was not as effective as expected. Grassland has more than double contribution to changes in desertification than forest and shrubland. Considering the fact that the area of grassland reduced by almost 9.0% in the study area, this may be one of the major reasons for the net increase in desertification area during 1978 to 2017. In addition, different vegetation types were found to have apparently different explanation of desertification area changes in each intensity level in this study. Of all the vegetation types, grassland worked well for dealing with all intensity levels of desertification. In controlling of slight and moderate desertification, forest worked as well as shrubland, while shrub-land worked much better than forest in controlling severe and extremely severe desertification. This is consistent with the findings in other studies that the establishment of shrub-land was an important step in the reversal of desertification processes (Maestre et al. Citation2009). The 40-year stand establishment rate of forest was only as 1/3 as that of shrubland and the forest had double degradation rate compared with the shrubland in the period with the most serious degradation, indicating that forest is less adaptive than shrub-land to the sandy land conditions. It was also found that the contribution of shrubland to desertification dynamics was more than twice of forest. These results suggest that shrub-land was more suitable than forest for large-scale afforestation in sandy land. These findings confirm that the future afforestation or vegetation restoration should fully consider the proportions of different vegetation types.
Human activities and climate change also weakened the impact of the TNAP in desertification control. This study reveals that the human activities were more crucial to desertification dynamics during the past 40 years than climate change. This result is consistent with the report that the socioeconomic factors were dominant in desertification development of China in recent decades (Li, Wu, and Huang Citation2012; Yan et al. Citation2015). The factors of human activities explained 25.3% of the desertification dynamics, took up 70.0% of the sum of the significant explanatory power by human activities and climate change (25.3% + 12.5%). In previous studies, this proportion was 65.8% to 68.6% (Zhou et al. Citation2014; Sun et al. Citation2019), which is similar to our results. Jiang et al. (Jiang et al. Citation2019) reported that the desertification process in grassland was more vulnerable to human activities than forest and sparse-forest-grass vegetation in Central Asia. In our study, it was clear that human activities not only resulted in the limited vegetation restoration, but also diminished the effect of the TNAP in desertification control in the sandy land. In most studies, the vegetation dynamics were used as a metric for indirectly identifying desertification rather than a driving force (Piao Citation2005; Verón et al. Citation2018; Jiang et al. Citation2019). Thus, our results provided a direct comparison between the effects of biological elements (i.e., vegetation dynamics) and non-biological elements (i.e., human activities and climate change) to desertification, which was an important supplement for understanding desertification. However, the intensity of human activities was difficult to describe adequately (Du et al. Citation2016). Thus the proxies used in this study, i.e., the change of population and GDP, may not be sufficient for detecting the full explanation power of human activities to desertification dynamics. According to Geist and Lambin’s study (Geist and Lambin Citation2004), desertification is driven by a limited set of variables but the pattern of synergies among causal factors has specific pathways for each region and period. The mixing of causal patterns caused by large space-time span of this study could be another reason for that the desertification dynamics was not fully significantly explained.
Suggestions and strategies
Here suggestions were proposed for the TNAP based on the findings in this study. For the future construction of the TNAP, the most important issue is to improve the survival rate and the stand establishment rate of afforestation, and minimize the degradation of surviving vegetation. According to our findings, the appropriate choice of vegetation type matching the local site conditions (particularly, water condition) could be the most effective way. With the originally harsh natural environment of the sandy land, the afforestation quantity and tree species should be determined according to scientific assessment of the site conditions, e.g., applying the annual precipitation, aridity index, or vegetation carrying capacity in planning (Zheng and Zhu Citation2017; Zhang et al. Citation2020). Besides the local species, shrub-land was demonstrated to be more adaptive to the natural conditions in sandy land than forest in this study. Thus, it is suggested to use shrubland, grassland, and native species to replace the forests in the areas of precipitation below 250 mm (Zheng and Zhu Citation2017). It is necessary to control human disturbances because the human activities were one of the major drivers for vegetation degradation and desertification. Grassland was found to be the most effective vegetation type in desertification control, the further disturbance of grassland should be urgently prohibited. According to the fact that the human disturbances, e.g., unsustainable grazing and cultivation practices, are related to the economic need of the local people, appropriate socio-economic strategies should be made based on “the interactions among the mountains, rivers, forests, farmland, lakes, grassland, sandy land, and the local people” (Yang Citation2004; Ge et al. Citation2015). Thus, rural poverty alleviation and the cost – benefit balance of the local community should be considered in the further construction of the TNAP (Cao, Suo, and Xia Citation2020; Suo and Cao Citation2021). Although climate change was less important than human activities in driving vegetation degradation and desertification, our study provides evidence that climate change could be a crucial factor of vegetation restoration in the future. With the stress from accelerated climate change (Huang et al. Citation2020), it is necessary to improve the resistance and resilience of the forest and shrubland under the conditions of climate change. For example, to establish the mixed conifer-broadleaved forests, because the mixed conifer-broadleaved forests were found to be able to keep lower degradation in all the four periods when other forest types suffered degradation. Similarly, the richness of plant species was also proved to be helpful in the functioning of restored vegetation (Li et al. Citation2021). According to the report of De Keersmaecker et al. (Citation2015), the resistance and resilience of vegetation is also associated with the proportion of forest and non-tree vegetation (i.e., shrub-land and grassland). Thus, it is suggested that transforming monoculture forests into mixed forests in areas of precipitation more than 350 mm, and making proper proportion of forest, shrubland, and grassland in areas of precipitation more than 200 mm (Zheng and Zhu Citation2017).
There are uncertainties in the estimation of vegetation area in sandy land by remote sensing images in this study. First, errors were inevitable from the interpretation of remote sensing images. Even for the high-resolution images, e.g., SPOT 5 and GF-1, the vegetation patches with area less than 400 m2 are difficult to be extracted (according to the pixel size of the images and 1:10,000 mapping requirement). Second, errors exist in the process of vegetation area calibration. Although the significant linear relationships were found in the vegetation area obtained from both Landsat TM and SPOT 5 (Appendix 3), there are still errors in the calibrated vegetation areas extracted from medium-resolution images compared with that from high-resolution images. Another limitation of this study is the method used for the identification of vegetation degradation by the combined trend analysis of NPP and PUE. In this study 10 years were used as the time period to detect the changing trend of NPP and PUE, because it is the minimum construction period of the TNAP. However, the 10-year period may not be the optimal time scale, as in other research the fluctuation period of NPP of vegetation in drylands was found to be 6 years, or even shorter (Jia, Liu, and Lin Citation2019). What’s more, the variations in NPP and PUE could be more complicated at the time scale of decades (Li, Wei, and Zhou Citation2015), which may not be able to be detected by linear regression. In future study, the trend analysis and degradation identification will be improved by combining the construction time point with the natural trend break point achieved by the Mann-Kendall method. In addition, to detect the spatial and temporal characteristics of NPP and PUE more precisely, multi time-scale analysis or multiple-regression will be applied. Besides, for research focused on large space-time scale like this study, the improvement of the quality of interpretation results is essential. In cloud platforms such as Google Earth engine, time-series composite remote sensing images could be managed, optimized, and compared, by which the seasonal effects and year-to-year change could be observed and captured better (Praticò et al. Citation2021). Thus, in future study, Google Earth engine will be applied for the improvement of vegetation classification.
Conclusions
This study revealed the vegetation dynamics and desertification process in the past 40 years in the sandy land with the construction of the TNAP. Our data showed that vegetation coverage increased by only 0.5%, with increasing 113.8% and 338.8% of forest and shrubland, but decreasing 9.0% of grassland during 1978–2017. Desertification had little change in area, but the proportion of slight-moderate intensity increased and outweighed that of the severe-extremely severe intensity. The TNAP contributed to desertification process by 10.3% in the 40 years, which is lower than expected. It was found that compared with shrubland, forest is less suitable for large-scale afforestation in sandy land, for it has relatively lower stand establishment rate, higher degradation rate, and less contribution to desertification reduction. In order to achieve the successful afforestation and more efficient desertification control, specific suggestions based on our research were provided as follows. (1) It is necessary to adjust the vegetation types to match the local conditions, e.g., using shrub-land, grassland, and native species to replace the forests in the areas of precipitation below 250 mm. (2) The measures to improve the vegetation resistance as well as the total impacts of vegetation on control of desertification should be implemented, e.g., transforming monoculture forests into mixed forests in areas of precipitation more than 350 mm, and making proper proportions for forest, shrubland, and grassland in areas of precipitation more than 200 mm. (3) The human disturbances should be controlled with appropriate socio-economic strategies by considering “the interactions among the mountains, rivers, forests, farmland, lakes, grassland, sandy land and the local people.” Our study provided a scientific support for planning and implementation of TNAP in the following 30 years and for the sustainable development of ecological restoration programs in sandy land worldwide. This study also provided an example for remote sensing research of sandy land vegetation on a large time-space scale, and further updates in methodologies combined with cloud platforms are suggested correspondingly.
Acknowledgement
This work was supported by the Consultation Project supported by Divisions of the Chinese Academy of Sciences [2019-ZW09-A-032]; and the National Nature Science Foundation of China [No. 31770758 and No. 32071837]. We acknowledge for the data support from “National Earth System Science Data Center, National Science & Technology Infrastructure of China (http://www.geodata.cn)”, “Resource and Environmental Science and Data Center, Institute of Geographic Sciences and Natural Resources Research (http://www.resdc.cn)” and “Cold and Arid Regions Sciences Data Center at Lanzhou (http://westdc.westgis.ac.cn)”.
Disclosure statement
No potential conflict of interest was reported by the authors.
Data availability statement
The data that support the findings of this study are available from the corresponding author upon reasonable request (http://www.iae.cas.cn/cslst/), except for the data from National Forest Resource Inventory and National Desertification and Sandification Monitoring.
Additional information
Funding
References
- Albalawi, E. K., and L. Kumar. 2013. “Using Remote Sensing Technology to Detect, Model and Map Desertification: A Review.” Journal of Food, Agriculture & Environment 11 (2): 791–26.
- Anyamba, A., and C. J. Tucker. 2005. “Analysis of Sahelian Vegetation Dynamics Using NOAA-AVHRR NDVI Data from 1981–2003.” Journal of Arid Environments 63 (3): 596–614. doi:10.1016/j.jaridenv.2005.03.007.
- Bai, Y., J. Wu, Q. Xing, Q. Pan, J. Huang, D. Yang, and X. Han. 2008. “Primary Production and Rain Use Efficiency Across a Precipitation Gradient on the Mongolia Plateau.” Ecology 89 (8): 2140–2153. doi:10.1890/07-0992.1.
- Bunning, S., J. Mcdonagh, J. Rioux, F. Nachtergaele, R. Biancalani, and A. C. Woodfine. 2016. Land Degradation Assessment in Drylands (LADA Project). FAO. http://www.fao.org/fileadmin/templates/nr/kagera/Documents/LADA_manuals
- Cao, S., L. Chen, D. Shankman, C. Wang, X. Wang, and H. Zhang. 2011. “Excessive Reliance on Afforestation in China’s Arid and Semi-Arid Regions: Lessons in Ecological Restoration.” Earth-Science Reviews 104 (4): 240–245. doi:10.1016/j.earscirev.2010.11.002.
- Cao, F., Y. Ge, and J. F. Wang. 2013. “Optimal Discretization for Geographical Detectors-Based Risk Assessment.” GIScience & Remote Sensing 50 (1): 78–92. doi:10.1080/15481603.2013.778562.
- Cao, S., X. Suo, and C. Xia. 2020. “Payoff from Afforestation Under the Three-North Shelter Forest Program.” Journal of Cleaner Production 256 (May): 120461. doi:10.1016/j.jclepro.2020.120461.
- National Forestroy and Grassland Administration. 2006. “The Main Results of the 3rd National Desertification and Sandification Monitoring.” http://www.forestry.gov.cn/main/65/20060924/758164.html.
- National Forestry and Grasssland Administration. 2014. The 5th Bulletin of National Desertification and Sandification Monitoring. http://www.forestry.gov.cn/main/69/content-831684.html.
- Chazdon, R. L. 2008. “Beyond Deforestation: Restoring Forests and Ecosystem Services on Degraded Lands.” Science 320 (5882): 1458–1460. doi:10.1126/science.1155365.
- Cherlet, M., C. Hutchinson, J. Reynolds, J. Hill, S. Sommer, and G. von Maltitz. 2018. World Atlas of Desertification. Luxembourg: Publication Office of the European Union.
- China Centre For Resources Satellite Data and Application. 2015. “GF–1.” http://www.cresda.com/EN/satellite/7155.shtml.
- Cui, T. X., Y. J. Wang, R. Sun, C. Qiao, W. Fan, G. Jiang, L. Hao, L. Zhang, and W. Yuan. 2016. “Estimating Vegetation Primary Production in the Heihe River Basin of China with Multi-Source and Multi-Scale Data.” Plos One 11 (4): e0153971. doi:10.1371/journal.pone.0153971.
- Du, Z., X. Xu, H. Zhang, Z. Wu, Y. Liu, and V. Magar. 2016. “Geographical Detector-Based Identification of the Impact of Major Determinants on Aeolian Desertification Risk Edited by Vanesa Magar.” Plos One 11 (3): e0151331. doi:10.1371/journal.pone.0151331.
- Du, Z., X. Zhang, X. Xu, H. Zhang, Z. Wu, and J. Pang. 2017. “Quantifying Influences of Physiographic Factors on Temperate Dryland Vegetation, Northwest China.” Scientific reports 7 (1): 40092. doi:10.1038/srep40092.
- Fang, J. Y., Y. C. Song, H. Y. Liu, and S. L. Piao. 2002. “Vegetation-Climate Relationship and Its Application in the Division of Vegetation Zone in China.” Acta Botanica Sinica 44 (9): 1105–1122.
- FAO. 2019. “Trees, Forests and Land Use in Drylands: The First Global Assessment-Full Report”. FAO Forestry Paper No.184, Rome.
- Feng, Q., H. Ma, X. Jiang, X. Wang, and S. Cao. 2015. “What Has Caused Desertification in China?” Scientific Reports 5 (1): 15998. doi:10.1038/srep15998.
- Geist, H. J., and E. F. Lambin. 2004. “Dynamic Causal Patterns of Desertification.” Bioscience 54 (9): 817–829. doi:10.1641/0006-3568(2004)054[0817:DCPOD]2.0.CO;2.
- Ge, X., Y. Li, A. E. Luloff, K. Dong, and J. Xiao. 2015. “Effect of Agricultural Economic Growth on Sandy Desertification in Horqin Sandy Land.” Ecological Economics 119 (November): 53–63. doi:10.1016/j.ecolecon.2015.08.006.
- Gerlein-Safdi, C., G. Keppel-Aleks, F. Wang, S. Frolking, and D. L. Mauzerall. 2020. “Satellite Monitoring of Natural Reforestation Efforts in China’s Drylands.” One Earth 2 (1): 98–108. doi:10.1016/j.oneear.2019.12.015.
- Gnacadja, L. 2015. “New Challenges in Science and Policies to Combat Desertification.” Journal of Arid Environments 112 (January): 1–4. doi:10.1016/j.jaridenv.2014.10.010.
- Guo, J., J. Wang, C. Xu, and Y. Song. 2022. “Modeling of Spatial Stratified Heterogeneity.” GIScience & Remote Sensing 59 (1): 1660–1677. doi:10.1080/15481603.2022.2126375.
- Hein, L., and N. De Ridder. 2006. “Desertification in the Sahel: A Reinterpretation.” Global Change Biology 12 (5): 751–758. doi:10.1111/j.1365-2486.2006.01135.x.
- He, B., S. Wang, L. Guo, and X. Wu. 2019. “Aridity Change and Its Correlation with Greening Over Drylands.” Agricultural and Forest Meteorology 278 (November): 107663. doi:10.1016/j.agrformet.2019.107663.
- Hou, X. Y. 2001. Vegetation Atlas of China (1:1000 000). Beijing: Science Press.
- Houerou, H. N. L. 1984. “Rain Use Efficiency: A Unifying Concept in Arid-Land Ecology.” Journal of Arid Environments 7 (3): 213–247. doi:10.1016/S0140-1963(18)31362-4.
- Huang, J., H. Yu, D. Han, G. Zhang, Y. Wei, J. Huang, L. An, X. Liu, and Y. Ren. 2020. “Declines in Global Ecological Security Under Climate Change.” Ecological indicators 117 (October): 106651. doi:10.1016/j.ecolind.2020.106651.
- Jia, Z. 2009. Forest Resources Report of China (The 7th National Forest Resources Inventory). Beijing: China Forestry Publishing House.
- Jia, J., H. Liu, and Z. Lin. 2019. “Multi-Time Scale Changes of Vegetation NPP in Six Provinces of Northwest China and Their Responses to Climate Change.” Acta Ecologica Sinica 39: 14.
- Jiang, L., G. Jiapaer, A. Bao, A. Kurban, H. Guo, G. Zheng, and P. De Maeyer. 2019. “Monitoring the Long-Term Desertification Process and Assessing the Relative Roles of Its Drivers in Central Asia.” Ecological indicators 104 (September): 195–208. doi:10.1016/j.ecolind.2019.04.067.
- Keersmaecker, W., S. L. Lhermitte, L. Tits, O. Honnay, B. Somers, and P. Coppin. 2015. “A Model Quantifying Global Vegetation Resistance and Resilience to Short-Term Climate Anomalies and Their Relationship with Vegetation Cover: Global Vegetation Resistance and Resilience.” Global Ecology and Biogeography 24 (5): 539–548. doi:10.1111/geb.12279.
- Landman, W. 2010. “Climate Change 2007: The Physical Science Basis.” South African Geographical Journal 92 (1): 86–87. doi:10.1080/03736245.2010.480842.
- Lanfredi, M., R. Coppola, T. Simoniello, R. Coluzzi, M. D’Emilio, V. Imbrenda, and M. Macchiato. 2015. “Early Identification of Land Degradation Hotspots in Complex Bio-Geographic Regions.” Remote Sensing 7 (6): 8154–8179. doi:10.3390/rs70608154.
- Latry, C., and B. Rouge. 1998. “SPOT5 THR Mode.“ In Earth Observing Systems III, 480–491. San Diego: SPIE. doi:10.1117/12.325654.
- Liang, P., and X. Yang. 2016. “Landscape Spatial Patterns in the Maowusu (Mu Us) Sandy Land, Northern China and Their Impact Factors.” CATENA 145 (October): 321–333. doi:10.1016/j.catena.2016.06.023.
- Li, Y., Y. Chen, and Z. Li. 2019. “Dry/Wet Pattern Changes in Global Dryland Areas Over the Past Six Decades.” Global and Planetary Change 178 (July): 184–192. doi:10.1016/j.gloplacha.2019.04.017.
- Li, C., B. Fu, S. Wang, L. C. Stringer, Y. Wang, Z. Li, Y. Liu, and W. Zhou. 2021. “Drivers and Impacts of Changes in China’s Drylands.” Nature Reviews Earth & Environment 2 (12, November): 858–873. doi:10.1038/s43017-021-00226-z.
- Liu, X. 2008. “Comment on “Why Large-Scale Afforestation Efforts in China Have Failed to Solve the Desertification Problem”.” Environmental Science & Technology 42 (21): 8165–8165. doi:10.1021/es801718q
- Li, H., X. Wei, and H. Zhou. 2015. “Rain-Use Efficiency and NDVI-Based Assessment of Karst Ecosystem Degradation or Recovery: A Case Study in Guangxi, China.” Environmental Earth Sciences 74 (2): 977–984. doi:10.1007/s12665-014-3679-6.
- Li, A., J. Wu, and J. Huang. 2012. “Distinguishing Between Human-Induced and Climate-Driven Vegetation Changes: A Critical Application of RESTREND in Inner Mongolia.” Landscape Ecology 27 (7): 969–982. doi:10.1007/s10980-012-9751-2.
- Loveland, T. R., and D. M. Shaw. 1996. “Multiresolution Land Characterization: Building Collaborative Partnerships.” In Gap Analysis: A Landscape Approach to Biodiversity Planning, Proceedings of the ASPRS/GAP Symposium, edited by J. M. Scott, T. Tear, and F. Davis, 83–89. Bethesda, Maryland: American Society for Photogrammetry and Remote Sensing.
- National Forestry and Grassland Administration. 2019. Forest Resources Report of China (2014-2018). Beijing: China Forestry Publishing House.
- Maestre, F. T., M. A. Bowker, M. D. Puche, M. Belén Hinojosa, I. Martínez, P. García-Palacios, A. P. Castillo, et al. 2009. “Shrub Encroachment Can Reverse Desertification in Semi-Arid Mediterranean Grasslands.” Ecology letters 12 (9): 930–941. doi:10.1111/j.1461-0248.2009.01352.x.
- National Forestry Administration. 2000. “The Results of the 2nd National Desertification and Sandification Monitoring.” http://www.scio.gov.cn/m/ztk/xwfb/18/10/Document/838878/838878.htm.
- National Forestry Administration. 2000. “Main Results of the 5th National Forest Resources Inventory (1994-1998).” http://www.forestry.gov.cn/portal/main/s/65/content-554361.html.
- National Forestry and Grassland Administration. 2014. Forest Resources Report of China (2009-2013). Beijing: China Forestry Publishing House.
- National Forestry and Grasssland Administration. 2009. The 4th Bulletin of National Desertification and Sandification Monitoring.
- Peng, S., Y. Ding, W. Liu, and Z. Li. 2019. “1 Km Monthly Temperature and Precipitation Dataset for China from 1901 to 2017.” Earth System Science Data 11 (4): 1931–1946. doi:10.5194/essd-11-1931-2019.
- Piao, S. 2005. “NDVI-Indicated Decline in Desertification in China in the Past Two Decades.” Geophysical Research Letters 32 (6): 6. doi:10.1029/2004GL021764.
- Pontius, R. G., E. Shusas, and M. McEachern. 2004. “Detecting Important Categorical Land Changes While Accounting for Persistence.” Agriculture, Ecosystems & Environment 101 (2–3): 251–268. doi:10.1016/j.agee.2003.09.008.
- Praticò, S., F. Solano, S. Di Fazio, and G. Modica. 2021. “Machine Learning Classification of Mediterranean Forest Habitats in Google Earth Engine Based on Seasonal Sentinel-2 Time-Series and Input Image Composition Optimisation.” Remote Sensing 13 (4): 586. doi:10.3390/rs13040586.
- Prăvălie, R., G. Bandoc, C. Patriche, and T. Sternberg. 2019. “Recent Changes in Global Drylands: Evidences from Two Major Aridity Databases.” CATENA 178 (July): 209–231. doi:10.1016/j.catena.2019.03.016.
- Prince, S. D., E. Brown De Colstoun, and L. L. Kravitz. 1998. “Evidence from Rain‐use Efficiencies Does Not Indicate Extensive Sahelian Desertification.” Global Change Biology 4 (4): 359–374. doi:10.1046/j.1365-2486.1998.00158.x.
- Li, Q., F. Yu, T. Sha, and X. Mao. 2019. China Ecological Governance Development Report. Beijing: Social Sciences Literature Press.
- Ran, Q., Y. Hao, A. Xia, W. Liu, R. Hu, X. Cui, K. Xue, et al. 2019. “Quantitative Assessment of the Impact of Physical and Anthropogenic Factors on Vegetation Spatial-Temporal Variation in Northern Tibet.” Remote Sensing 11 (10): 1183. doi:10.3390/rs11101183.
- Reid, W. V., R. T. Watson, T. Rosswall, A. Steiner, K. Töpfer, S. Arico, and P. Bridgewater. 2005. “Millennium Ecosystem Assessment: Ecosystems and Human Well Being - Synthesis Report.”
- Sayl, K. N., S. O. Oleiwi Sulaiman, A. H. Hatem Kamel, N. S. Shazwani Muhammad, J. Abdullah, N. Al-Ansari, and Y. Qian. 2021. “Minimizing the Impacts of Desertification in an Arid Region: A Case Study of the West Desert of Iraq.” Advances in Civil Engineering 2021 (June): 5580286. doi:10.1155/2021/5580286.
- Smith, W. K., M. P. Dannenberg, D. Yan, S. Herrmann, M. L. Barnes, G. A. Barron-Gafford, J. A. Biederman, et al. 2019. “Remote Sensing of Dryland Ecosystem Structure and Function: Progress, Challenges, and Opportunities.” Remote Sensing of Environment 233 (November): 111401. doi:10.1016/j.rse.2019.111401.
- Snyman, H. A. 1998. “Dynamics and Sustainable Utilization of Rangeland Ecosystems in Arid and Semi-Arid Climates of Southern Africa.” Journal of Arid Environments 39 (4): 645–666. doi:10.1006/jare.1998.0387.
- Su, Y., Q. Guo, T. Hu, H. Guan, S. Jin, S. An, X. Chen, et al. 2020. “An Updated Vegetation Map of China (1:1000000).” Science Bulletin 65(13April): S2095927320302152. 10.1016/j.scib.2020.04.004
- Sun, B., Z. Li, W. Gao, Y. Zhang, Z. Gao, Z. Song, P. Qin, and X. Tian. 2019. “Identification and Assessment of the Factors Driving Vegetation Degradation/Regeneration in Drylands Using Synthetic High Spatiotemporal Remote Sensing Data—a Case Study in Zhenglanqi, Inner Mongolia, China.” Ecological indicators 107 (December): 105614. doi:10.1016/j.ecolind.2019.105614.
- Suo, X., and S. Cao. 2021. “China’s Three North Shelter Forest Program: Cost–Benefit Analysis and Policy Implications.” Environment, Development and Sustainability 23 (10): 14605–14618. doi:10.1007/s10668-021-01260-z.
- Wang, T. 2014. “Aeolian Desertification and Its Control in Northern China.” International Soil and Water Conservation Research 2 (4): 34–41. doi:10.1016/S2095-6339(15)30056-3.
- UNCCD. 1994. “‘United Nations Convention to Combat Desertification, Elaboration of an International Convention to Combat Desertification in Countries Experiencing Serious Drought And/Or Desertification, Particularly in Africa’(u.N. Doc. A/AC.241/27, 33 I.L.M. 1328, United Nations, 1994).”
- Uzuner, Ç., and O. Dengiz. 2020. “Desertification Risk Assessment in Turkey Based on Environmentally Sensitive Areas.” Ecological indicators 114: 106295. doi:10.1016/j.ecolind.2020.106295.
- Verbesselt, J., R. Hyndman, G. Newnham, and D. Culvenor. 2010. “Detecting Trend and Seasonal Changes in Satellite Image Time Series.” Remote Sensing of Environment 114 (1): 10. doi:10.1016/j.rse.2009.08.014.
- Verón, S. R., L. J. Blanco, M. A. Texeira, J. G. N. Irisarri, and J. M. Paruelo. 2018. “Desertification and Ecosystem Services Supply: The Case of the Arid Chaco of South America.” Journal of Arid Environments 159 (December): 66–74. doi:10.1016/j.jaridenv.2017.11.001.
- Wang, J., K. Cheng, Q. Liu, J. Zhu, A. Ochir, D. Davaasuren, G. Li, et al. 2019. “Land Cover Patterns in Mongolia and Their Spatiotemporal Changes from 1990 to 2010.” Arabian Journal of Geosciences 12 (24): 778. doi:10.1007/s12517-019-4893-z.
- Wang, J. F., and Y. Hu. 2012. “Environmental Health Risk Detection with GeogDetector.” Environmental Modelling & Software 33: 114–115. doi:10.1016/j.envsoft.2012.01.015.
- Wang, J. -F., X. -H. Li, G. Christakos, Y. -L. Liao, T. Zhang, X. Gu, and X. -Y. Zheng. 2010. “Geographical Detectors-Based Health Risk Assessment and Its Application in the Neural Tube Defects Study of the Heshun Region, China.” International Journal of Geographical Information Science 24 (1): 107–127. doi:10.1080/13658810802443457.
- Wang, M. J., R. Sun, A. R. Zhu, and Z. Q. Xiao. 2020. “Evaluation and Comparison of Light Use Efficiency and Gross Primary Productivity Using Three Different Approaches.” Remote Sensing 12 (6): 1003. doi:10.3390/rs12061003.
- Wang, Y., J. Wang, Q. Yuan, and C. Yan. 2005. The Dataset of the Distribution of Desert (Sandy Land) in China (1:100,000). Cold and Arid Regions Sciences Data Center. doi:10.3972/westdc.006.2013.db.
- Wang, J. F., T. L. Zhang, and B. J. Fu. 2016. “A Measure of Spatial Stratified Heterogeneity.” Ecological indicators 67 (August): 250–256. doi:10.1016/j.ecolind.2016.02.052.
- Wang, X. M., C. X. Zhang, E. Hasi, and Z. B. Dong. 2010. “Has the Three Norths Forest Shelterbelt Program Solved the Desertification and Dust Storm Problems in Arid and Semiarid China?” Journal of Arid Environments 74 (1): 13–22. doi:10.1016/j.jaridenv.2009.08.001.
- Li, W. 2004. “Degradation and Restoration of Forest Ecosystems in China.” Forest Ecology and Management 201 (1): 33–41. doi:10.1016/j.foreco.2004.06.010.
- Xiao, X. 2005. Inventory of Forest Resources of China. Beijing: China Forestry Publishing House.
- Xu, X. L. 2017a. “China’s GDP Spatial Distribution Dataset (1 Kilometer Grid).” Data Registration and Publishing System of the Resource and Environmental Science Data Center of the Chinese Academy of Sciences. doi:10.12078/2017121102.
- Xu, X. L. 2017b. “China’s Population Spatial Distribution Dataset (1 Kilometer Grid).” Data Registration and Publishing System of the Resource and Environmental Science Data Center of the Chinese Academy of Sciences. doi:10.12078/2017121101.
- Xu, X. L., J. Y. Liu, S. W. Zhang, R. D. Li, C. Z. Yan, and S. X. Wu. 2018. “China’s Multi-Period Land Use Land Cover Remote Sensing Monitoring Dataset (CNLUCC).” Data Registration and Publishing System of the Resource and Environmental Science Data Center of the Chinese Academy of Sciences. doi:10.12078/2018070201.
- Xu, X. L., and Y. Q. Zhang. 2017. “China’s Meteorological Background Dataset.” Data Registration and Publishing System of the Resource and Environmental Science Data Center of the Chinese Academy of Sciences. doi:10.12078/2017121301.
- Yang, H. 2004. “Land Conservation Campaign in China: Integrated Management, Local Participation and Food Supply Option.” Geoforum 35 (4): 507–518. doi:10.1016/j.geoforum.2003.10.002.
- Yan, C. Z., X. Song, Y. M. Zhou, H. C. Duan, and S. Li. 2009. “Assessment of Aeolian Desertification Trends from 1975’s to 2005’s in the Watershed of the Longyangxia Reservoir in the Upper Reaches of China’s Yellow River.” Geomorphology 112 (3–4): 205–211. doi:10.1016/j.geomorph.2009.06.003.
- Yan, Q. L., J. J. Zhu, Z. B. Hu, and O. J. Sun. 2011. “Environmental Impacts of the Shelter Forests in Horqin Sandy Land, Northeast China.” Journal of Environmental Quality 40 (3): 815. doi:10.2134/jeq2010.0137.
- Yan, Q., J. Zhu, X. Zheng, and C. Jin. 2015. “Causal Effects of Shelter Forests and Water Factors on Desertification Control During 2000–2010 at the Horqin Sandy Land Region, China.” Journal of Forestry Research 26 (1): 33–45. doi:10.1007/s11676-014-0012-x.
- Yu, T., R. Sun, Z. Xiao, Q. Zhang, G. Liu, T. Cui, and J. Wang. 2018. “Estimation of Global Vegetation Productivity from Global LAnd Surface Satellite Data.” Remote Sensing 10 (2): 327. doi:10.3390/rs10020327.
- Zhang, Z., and D. Huisingh. 2018. “Combating Desertification in China: Monitoring, Control, Management and Revegetation.” Journal of Cleaner Production 182 (May): 765–775. doi:10.1016/j.jclepro.2018.01.233.
- Zhang, X., J. Wang, Y. Gao, and L. Wang. 2021. “Variations and Controlling Factors of Vegetation Dynamics on the Qingzang Plateau of China Over the Recent 20 Years.” Geography and Sustainability 2 (1): 74–85. doi:10.1016/j.geosus.2021.02.001.
- Zhang, G., J. Yan, X. Zhu, H. Ling, and H. Xu. 2019. “Spatio-Temporal Variation in Grassland Degradation and Its Main Drivers, Based on Biomass: Case Study in the Altay Prefecture, China.” Global Ecology and Conservation 20 (October): e00723. doi:10.1016/j.gecco.2019.e00723.
- Zhang, J., Y. Zhang, S. Qin, B. Wu, G. Ding, X. Wu, Y. Gao, and Y. Zhu. 2020. “Carrying Capacity for Vegetation Across Northern China Drylands.” The Science of the Total Environment 710 (March): 136391. doi:10.1016/j.scitotenv.2019.136391.
- Zhao, W., Z. Hu, Q. Guo, G. Wu, R. Chen, and S. Li. 2020. “Contributions of Climatic Factors to Interannual Variability of the Vegetation Index in Northern China Grasslands.” Journal of Climate 33 (1): 175–183. doi:10.1175/JCLI-D-18-0587.1.
- Zhao, G., M. Liu, P. Shi, N. Zong, J. Wang, J. Wu, and X. Zhang. 2019. “Spatial–Temporal Variation of ANPP and Rain-Use Efficiency Along a Precipitation Gradient on Changtang Plateau, Tibet.” Remote Sensing 11 (3): 325. doi:10.3390/rs11030325.
- Zheng, K., L. Tan, Y. Sun, Y. Wu, Z. Duan, Y. Xu, and C. Gao. 2021. “Impacts of Climate Change and Anthropogenic Activities on Vegetation Change: Evidence from Typical Areas in China.” Ecological indicators 126 (July): 107648. doi:10.1016/j.ecolind.2021.107648.
- Zheng, X., and J. J. Zhu. 2017. “A New Climatic Classification of Afforestation in Three-North Regions of China with Multi-Source Remote Sensing Data.” Theoretical and Applied Climatology 127 (1–2): 465–480. doi:10.1007/s00704-015-1646-0.
- Zheng, X., J. J. Zhu, Q. L. Yan, and L. N. Song. 2012. “Effects of Land Use Changes on the Groundwater Table and the Decline of Pinus Sylvestris Var. Mongolica Plantations in Southern Horqin Sandy Land, Northeast China.” Agricultural Water Management 109 (June): 94–106. doi:10.1016/j.agwat.2012.02.010.
- Zhou, W., C. Gang, L. Zhou, Y. Chen, J. Li, W. Ju, and I. Odeh. 2014. “Dynamic of Grassland Vegetation Degradation and Its Quantitative Assessment in the Northwest China.” Acta Oecologica 55 (February): 86–96. doi:10.1016/j.actao.2013.12.006.
- Zhu, J. J., and X. Zheng. 2019. “The Prospects of Development of the Three-North Afforestation Program (TNAP) : On the Basis of the Results of the 40-Year Construction General Assessment of the TNAP.” Chinese Journal of Ecology 38 (05): 1600–1610.
- Zhu, J., X. Zheng, and Q. Yan. 2016. Assessment of Impacts of the Three-North Protective Forest Program on Ecological Environments by Remote Sensing Technology—launched After 30 Years (1978-2008). Beijing: Science Press.
Appendices
Appendix 1. Vegetation type classification system. This table was adapted from the tables of Zhu et al. (Citation2016) and Zheng and Zhu (Citation2017).
Appendix 2. Desertification classification system and landscape features of each intensity level. This table was adapted from the table of Yan et al. (Citation2009).
Appendix 3. Calibration relationship between vegetation area values extracted from SPOT 5 and Landsat TM and comparison of the vegetation area value before calibration and the value after calibration. The calibration relationship was built up separately for regions where annual average precipitation higher than 303 mm (a) and annual average precipitation lower than 303 mm (b). The comparison between the calibrated vegetation area extracted from Landsat TM and that extracted from SPOT 5 were checked for regions where annual average precipitation higher than 303 mm (c) and annual average precipitation lower than 303 mm (d). This figure was adapted from the figures of Zhu et al. (Citation2016).

Appendix 4. Assessment of the outcome of the vegetation quality type identified in this study with plot data from National Forest Resource Inventory. Consistency was the ratio of the number of the plots that were identified as healthy vegetation and no degradation, or identified as unhealthy vegetation and severe/potential degradation at the same time to the number of all plots. The assessment was for the outcome of vegetation quality type identification during 2010–2017, for the healthy status data from National Forest Resource Inventory are only available for 2017.
Appendix 5.
Area of vegetation types of study area in each time point (million ha).
Appendix 6.
TC, NC, and SC of different vegetation types for period of 1978-1990, 1990-2000, Citation2000-2010, and 2010-2017 (104 ha). TC = total change area; NC = net change area; SC = swap change area.
Appendix 7.
Spatial distribution of each category of vegetation quality types for all vegetation types in four periods during 1978 to 2017.

Appendix 8.
Area of desertification by intensity level during 1978-2017 (million ha).
Appendix 9. Title and scene ID of the remote sensing images used. For the scene ID, for Landsat images is the “path number and row number”, for SPOT 5 images is the number of “K and J”, and for GF-1 is the “Scene Number”.