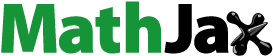
ABSTRACT
Lava flows from volcanoes can significantly destroy infrastructure, although they rarely threaten human life. Mapping lava flows is essential to managing the volcanic hazard during eruptions and understanding significant changes in geomorphology after eruptions. In 2018, the eruption of Kilauea, Hawaii’s youngest and most active volcano, destroyed more than 700 homes, farmlands, and roads. Active lava flow tracking is crucial for providing timely warnings to evacuate residents and protect infrastructure. We constructed a map of the lava flow in the East Rift Zone (ERZ) during the Kilauea volcanic eruption in 2018 using remotely sensed satellite synthetic aperture radar observations. We processed 23 C-band Sentinel-1 A/B interferometric wide mode acquisitions captured from May 1 to 17 September 2018 and applied a coherent change detection (CCD) technique to detect minute changes in the target structure. A time series of coherence maps provided detailed spatial information on the movement of the lava flow fields in the ERZ. The overall lava flow map had an area of approximately 33.3 km2 and was verified with a lava thickness map provided by the United States Geological Survey that was generated by two digital elevation models using airborne light detection and ranging observations. Excellent agreement (>90%) among the lava flow observations suggests that the CCD technique is effective in monitoring lava flows during a volcanic eruption.
1. Introduction
Volcanoes are unique geological features created by the extrusion of magma through the earth’s crust that reaches the surface. Volcanic eruptions can generate numerous phenomena, including lava flows, ashfalls, pyroclastic density currents, and volcanic gases, which can create casualties and destroy the surrounding natural environment and man-made infrastructure (Vallance et al. Citation2001). Hot spot volcanoes (e.g. Kilauea in Hawaii), which are caused by a long-lasting magma supply from the Earth’s mantle, emit a large amount of flowable lava that encompasses large areas during eruptions (Tilling et al. Citation1994). In general, volcanic eruption are localized hazards and the emitted lava flows slowly (Harris Citation2015); thus, it rarely threatens human life (Arnold et al. Citation2019). However, lava flows can dramatically transform the original topography and destroy all ecosystems and environments along their paths, resulting in major societal and economic disruption (Barberi et al. Citation1993; Harris Citation2015; Brown et al. Citation2017). Additional lava flows due to subsequent volcanic activity after short intervals may be affected by changes in the terrain due to preexisting lava and result in complex directions of lava flows and cooling mechanisms (Kauahikaua Citation2007; Romero et al. Citation2022). Hence, the construction of a detailed lava flow map is essential to mitigate catastrophic geohazards and assess the risk of lava flow by predicting or simulating the path of lava flow (Richter et al. Citation2016; Favalli et al. Citation2009). Comprehensive information regarding the lava flow can help understand its emplacement processes, which can be estimated using numerical flow models (Turner, Perroy, and Hon Citation2017).
Although traditional terrestrial field surveys such as the Global Navigation Satellite System and ground-based terrestrial laser scanners can provide the most accurate information of near-real-time lava flows (Richter et al. Citation2016), these methods have limitations in terms of the possible danger to access during volcanic activity and instantaneous measurement over broad areas. Remotely sensed multispectral observations, such as light detection and ranging (LiDAR) and photogrammetry using spaceborne, airborne, and unmanned aerial vehicles (UAVs), including drone platforms, can provide a very high spatial resolution map of the lava flow (Fornaciai et al. Citation2022; Di et al. Citation2020; Gracchi et al. Citation2022). However, the cost and logistics of airborne and UAV imaging prevent frequent (e.g. daily, or hourly) observations, and multi-spectral optical observations rely on weather or day and night conditions.
Observations from space-based synthetic aperture radar (SAR), a coherent imaging radar, can provide periodic and detailed surface information because of its capability to obtain images in any weather condition (Hanssen Citation2001; Bamler and Hartl Citation1998). Generally, a SAR image provides two types of information like amplitude and phase that depend on the physical and electrical properties (Moreira et al. Citation2013). As the amplitude reflects the backscattered intensity according to ground surface characteristics, such as thickness, roughness, or wetness, the amount of the backscattered intensity from a newly deposited lava region can vary (Wadge et al. Citation2011; Arnold et al. Citation2018, Citation2017). Thus, a comparison of muti-temporal amplitude data acquired before and after eruption can be utilized to detect the new lava flow area using the difference of scattering properties in terms of both vegetation and the lava flow field (Casalbore et al. Citation2021; Nolesini et al. Citation2018; Saepuloh et al. Citation2010, Citation2015). However, a new lava flow over existing lava emplacement fields from past eruptions might prohibit the differentiation of the lava flow mechanism using the SAR amplitude image (Poland Citation2022). Poland (Citation2022) utilized a dataset comprising two cross-polarized amplitude images and a coherence value to construct a volcanic flow map. The color composite image can help discern the lava flow area between vegetated and unvegetated areas. Although the use of the amplitude image with coherence can detect the lava flow, setting appropriate thresholds is often challenging. This is because the amplitude image usually has a larger dynamic range value than the coherence differences. The SAR interferometry (InSAR) technique can estimate the topographic height using phase differences between two radar observations. A multi-temporal topographic elevation obtained using the InSAR technique can be used to monitor the change in volcanic deposits (Arnold et al. Citation2016). Differential interferometry (DInSAR) can be used to observe the displacement by removing the phase resulting from deformation during the time of acquisition (Moreira et al. Citation2013). In particular, time-series analysis using differential interferograms has been widely used to estimate surface displacements caused by earthquakes, volcanoes, ground subsidence, and glacier movements with a high accuracy ranging from millimeter to centimeter scales (Bui et al. Citation2021; De Luca et al. Citation2021; Grassi et al. Citation2022; Lyu et al. Citation2020; Wang et al. Citation2022). However, monitoring lava flows using the DInSAR technique is challenging because of the severe degradation of coherence, which is used to evaluate the quantitative similarity of the backscattered phase signals between two interferometric SAR observations (Touzi et al. Citation1999). The coherence ranges from zero, implying that the scattering target has undergone deformation, to one, implying that no surface change has occurred (Yanjie and Prinet Citation2004; Bouaraba, Belhadj-Aissa, and Closson Citation2016). Thus, coherence images can easily represent surface changes caused by lava flow, which cannot be easily retrieved by the interferometric phase (Lê et al. Citation2019). The coherent change detection (CCD) technique compares the difference between two coherence images that have a simple range (0 to 1), providing surface change information after an event (Bouaraba et al. Citation2012). Washaya et al. (Citation2018) used the CCD method for rapid natural disaster monitoring and management in urban areas during an earthquake in September 2017 in San Juan, the capital city of Puerto Rico, with Sentinel-1 SAR data. They adopted coherence threshold criteria to discriminate between surface changes. Jung et al. (Citation2016) detected a volcanic ash deposit after an eruption using CCD analysis with Advanced Land Observing Satellite Phased Array type L-band Synthetic Aperture Radar (ALOS PALSAR) observations from the Shinmoedake volcanic eruption in Japan in 2011. A new temporal correlation model was introduced that considered the ground-to-volume ratio.
New lava flows significantly alter the scattering behavior of the surface, resulting in coherence degradation of the coherence image (Dietterich et al. Citation2012). Rapid cooling of the lava flow enables the maintenance of coherent scatterers during radar acquisition after the eruption (Zebker et al. Citation1996). These different surface characteristics of the lava flow can be used to discriminate the surface change from lava flow fields through CCD analysis. Dietterich et al. (Citation2012) tracked lava flow emplacement over the East Rift Zone (ERZ) of Kilauea, Hawaii using coherence decorrelation in sea water and vegetated areas. In this study, we construct the lava flow map using CCD analysis that can calculate the difference in coherence values and extract the quantitative lava flow area of the Kilauea eruption in 2018.
Kilauea is one of the most active volcanoes globally and is a shield volcano located in the ERZ, the southeastern part of the Big Island of Hawaii (), and has erupted continuously since the 1980s (Turner, Perroy, and Hon Citation2017; Klein Citation1982). Most areas of Kilauea comprise vast lava fields created by historical eruptions that have been protected to preserve the natural flora and fauna in the wild ecosystem.
Figure 1. Map of the study area. (a) amplitude synthetic aperture radar (SAR) image of the sentinel-1 ground range detected (GRD) product collected on November 17, 2016 depicting hawaii’s big island. The red dotted rectangle denotes the area of interest located in the eastern part of the east rift zone (ERZ). (b) the optical image of multispectral Landsat-8 operational land imager (OLI) in February 2019 shows the lava flow area (courtesy of United States geological survey; USGS). The yellow dotted polygons indicate previously erupted lava, and the other darker areas (red arrow) were created by the lava flow during the volcanic eruption in 2018.

Lava flows could destroy the natural forest environment, triggering increased methane discharge into the atmosphere (James et al. Citation2009). Our study focused on the lava flow field in eastern part of the ERZ, created by effusion from fissure 8 near the Leilani Estates for approximately three months beginning in May 2018 (Patrick et al. Citation2019). Soule et al. (Citation2021) measured the new lava delta area formed by the eruption in 2018 by investigating the deposit grain size, which affected the shape and stability of the delta. They reported that a large lava delta with an area of approximately 35.5 km2 had been created toward the southeastern coastal direction. The erupted lava transformed the original topography or geography, destroyed the geological topography near the volcano, and created a large lava delta in the direction of the southeastern sea (Mosbrucker, Zoeller, and Ramsey Citation2020.). Laverdiere et al. (Citation2020) utilized convolutional neural network techniques to monitor the structural destruction caused by the lava flow during the eruption in 2018. Their approach is based on a machine learning method with remotely sensed imagery that detects the region damaged by fast-moving lava flows. DeGraffenried et al. (Citation2021) applied a lava flow propagation model to predict the precise rate change of a lava flow from the eruption using captured still shots and thermal orthomosaics from helicopter overflight imagery. In this study, we constructed a lava flow map from May to August 2018 using CCD analysis. Our main objective was to evaluate the capability of interferometric coherent change detection and map the lava flow using space-based SAR observations. We first describe the dataset and methodology used for the lava flow map construction in the following section and then present the time-series CCD analysis results to track the lava flow. Finally, we discuss the advantages and limitations of the CCD technique for mapping the lava flow.
2. Data and methodology
2.1 Data
We collected a total of 23 sets of Sentinel-1 A/B interferometric wide mode single look complex (SLC) SAR data, operated by the European Space Agency (ESA), from May 2 to 17 September 2018 to calculate a time series of coherence maps (). They have a spatial resolution of 5 × 20 m in the range and azimuth direction, with a swath width of 250 km and revisit cycle of 12 days (González et al. Citation2015). As the Sentinel-1 mission comprises a constellation of two satellites, the revisit cycle can be reduced to only 6 days. A shorter temporal time span between two consecutive observations is a more favorable condition for ensuring coherence because of the lack of considerable variation in the surface conditions (Hong, Wdowinski, and Kim Citation2009; Hong and Wdowinski Citation2013).
Table 1. The list of data used in this study.
We used a lava thickness map produced by the United States Geological Survey (USGS) to validate the constructed lava flow map using CCD analysis. The thickness map was derived by a topographic field survey using a helicopter-mounted LiDAR sensor to estimate the variation in elevation pre- and post-eruption (Hawaiian-Volcano-Observatory Citation2019). We also utilized two types of digital elevation model (DEM) products to create another lava thickness map to verify our results. One was the LiDAR DEM with a spatial resolution of 1/3 arc seconds (~10 m) and vertical accuracy of less than 5 m, provided by USGS 3D Elevation Program (3DEP) that was called the National Elevation Dataset (NED) up to 2015 (Tighe and Chamberlain Citation2009); the other product was the airborne LiDAR DEM data provided by the USGS in July 2019. An airborne LiDAR survey was rigorously conducted around the Kilauea volcano summit, ERZ, and Puʻu ʻŌʻō lava flow field after the eruption in 2018 (Mosbrucker, Zoeller, and Ramsey Citation2020.). We collected two operational land imager (OLI) and thermal infrared sensor (TIRS) products of Landsat 8 from July 28 to 20 August 2018 to retrieve the land surface temperature (LST). The OLI collects data with a spatial resolution of 30 m for bands 1–9, except band 8 that has a spatial resolution of 15 m. The TIRS comprised two thermal bands (10 and 11) with a spatial resolution of 100 m. Data from spectral band 10 (10.6–11.9 µm) are useful in providing accurate surface temperatures (Blackett Citation2014). Therefore, we investigated the relationship between LST and coherence during the lava cooling process.
2.2 Methodologies
2.2.1 Lava flow mapping using CCD analysis
We processed the Sentinel-1 dataset to produce coherence maps using the Sentinel Application Platform (SNAP), which was developed by the ESA as common software to support Sentinel missions (Hogoiu Citation2022). SNAP is an open-source toolbox that includes various SAR image processing capabilities for interferometric and polarimetric applications (Zuhlke et al. Citation2015).
shows the flowchart of the CCD analysis used in this study. Sentinel-1 adopts the terrain observation with progressive scan SAR (TOPSAR) technique, comprising three sub-swaths and multiple bursts (González et al. Citation2015). We applied orbit correction to refine the accurate satellite positions and reduce the phase noise. Subsequently, all secondary SLC images were co-registered to a chosen reference image using the orbit ephemeris and DEM data from the Shuttle Radar Topography Mission (SRTM) with a resolution of three arc seconds (~90 m).
Figure 2. The data processing scheme used for constructing the lava flow map. Flow chart of the (a) coherent change detection (CCD) analysis using Sentinel-1 interferometric wide mode terrain observation with progressive scan (TOPS) SAR single look complex (SLC) observations, (b) land surface temperature (LST) conversion using landsat 8 OLI and thermal infrared sensor (TIRS) measurements, and (c) comparison between the coherence and LST value. For the conversion of brightness temperature (BT), we used top-of-atmosphere (TOA) information of thermal band 10 and the normal difference vegetation index (NDVI), proportion of vegetation (Pv), and surface emissivity (ε) of bands 4 and 5.

We utilized only consecutive SAR observations with a revisit cycle of 6 days to consider the temporal decorrelation. After conducting burst overlap co-registration to remove the phase discontinuities between bursts, Goldstein phase filtering was applied to suppress the phase noise (Goldstein and Werner Citation1998). A coherence map was constructed using 10 × 10 pixels of the sub-window. All coherence maps were geocoded using the SRTM DEM data. Finally, a time series of CCD maps was generated by subtracting the former coherence image from the latter. To eliminate the area of previously erupted lava flows, the final CCD map was calculated by subtracting the two coherence maps that corresponded to the periods before and after the eruption (). Consequently, the CCD map ranges from -1 to 1.
Figure 3. A time series of coherence maps showing lava flow from periods before and after the eruption. The data in each inset indicate the interferometric pair used for generating the coherence map. The lava flow area over time is clearly detected by high coherence values. The yellow dotted polygons in (a) indicate the previously erupted lava area. The red dotted polygon in (q) indicates partial coherence degradation despite the termination of volcanic activity. The cyan dotted polygon in (v) shows the entire lava flow area, including the past lava flow area.

2.2.2 Lava flow mapping using DEM subtraction
A lava thickness map was derived by subtracting the 3DEP LiDAR DEM data published in September 2013 from the LiDAR DEM acquired in July 2019 to validate the lava flow map using CCD analysis. We utilized QGIS, an open-source geographic information system (GIS) tool, to support various geospatial data formats and offer valuable GIS functions (Meyer and Riechert Citation2019). The eruption in 2018 was the only one that occurred between the creation of the collected two DEMs in the study area (Hawaiian-Volcano-Observatory, Citation2018). Thus, a lava thickness map can be generated by subtracting the two DEMs (Lu et al. Citation2003). The masking process was applied to the initial lava thickness map if the newly created lava flow field from the 2018 eruption had a positive topographic height change (>0 m).
2.2.3 Estimation of land surface temperature (LST)
To compare the coherence with LST over lava flow fields, as CCD analysis relies on coherence, we converted the brightness temperature (BT) value of thermal band 10 in the Landsat 8 TIRS dataset into the LST (). The top-of-atmosphere (TOA) spectral radiance was calculated using the values of the band-specific multiplicative rescaling factor and additive factor. Two thermal conversion constants, K1 (774.8853) and K2 (1321.0789), found in the metadata, were used to calculate the BT. Normal difference vegetation index (NDVI) values were used to analyze temporal changes in temperature radiance concentration over time. The surface emissivity (ε) is related to the radiation, convection, or conduction, and the proportion of vegetation (Pv) is affected by the LST. NDVI, Pv, and emissivity were estimated using Landsat OLI bands 4 and 5. Finally, LST was converted using the following equation (Avdan and Jovanovska Citation2016):
We constructed two LST maps using the Landsat-8 dataset collected on August 4 and 13, 2018, which were close to the acquisition date of the Sentinel-1 dataset (August 6 and 12, 2018) for coherence estimation. The two LST maps were merged into an LST image and compared with the coherence map.
3. Results
CCD analysis using frequent observations of the Sentinel-1 dataset enabled the extraction of the lava flow field. We successfully identified the process of the lava flow field over five months, including the periods before and after eruption through time-series coherence maps (). The coherence level could depend on interferometric decorrelation factors, such as the geometric and temporal baseline and volume scattering. The results show the developments in the lava flow area by increasing the bright areas (high coherence). The time-series coherence maps could provide useful information on surface changes due to the lava flow. The coherence map from May 2 to 8 clearly shows a lava flow field created by volcanic activity during the eruption in the 1960s (yellow polygons in ). In early August (), a higher coherence map, depicted as an area brighter than that in , indicates a newly exposed lava flow field from the eruption in 2018. However, partial coherence degradation could still be detected, although the volcanic activity had practically stopped (red dotted polygon in . The coherence map generated using the datasets acquired on September 11 and 17 can detect the overall surface change owing to new and past lava flow fields, as shown in the cyan dotted polygon in .
The CCD technique was used to discriminate between the two lava flows. The lava flow map was generated using the CCD analysis by subtracting the coherence map in the middle of September () from the coherence image in early May () in the range of -1 to 1. A negative CCD value ranging from 0 to -1 indicates a new lava flow field from the eruption in 2018, as shown in the dark region of . We observed that the CCD technique could effectively remove past lava flow fields.
Figure 4. (a) CCD estimation and (b) DEM subtraction results for the lava flow maps. The newly created lava areas from the eruption in 2018 were 33.3 and 34.8 km2 using the CCD estimation approach and DEM subtraction method, respectively. Thresholds to discriminate the lava flow field from other surface features are zero coherence change and zero meters in DEM. The A-A’ transection line was used to evaluate the height differences due to eruptions.

Through the subtraction of DEMs, the lava thickness map can provide useful information regarding the lava flow field (). The lava thickness ranged from 0 to 30 The total area of each estimated lava flow field (km2), as shown in , and the percentage of overlap area with respect to the USGS lava thickness map (%).
Figure 5. The final lava flow maps from the (a) CCD analysis, (b) subtraction of DEMs, and (c) USGS lava thickness map (Hawaiian-Volcano-Observatory Citation2019). The overall patterns of the constructed lava flow field are similar. The CCD analysis result is in good agreement with the reference lava flow map obtained from the USGS at the inland area, whereas the DEM subtraction result represents a newly formed land area along the coastline (the red dotted polygon area in ).

The lava flow was mapped by masking areas where the lava did not flow in 2018. Although negative values were selected in the CCD analysis (yellow polygon in )), the difference in the topographic heights, which was greater than zero, remained in the lava thickness map (cyan polygon in )). The USGS provided another lava thickness map that was used to validate our results (magenta polygon in )). The pattern or distribution of the lava flow field of the constructed lava flow is similar to that of the reference map of the USGS. Notably, the results from the CCD analysis have an almost identical lava flow field. The total areas of the lava flow field of the CCD analysis, the lava thickness map of our results, and that of the USGS are 33.3, 34.8, and 36.9 km2, respectively (). The percentages of overlap with respect to the USGS lava thickness map were 90.2 and 94.3% in the CCD analysis and our map, respectively. This indicates that CCD analysis is a useful approach for estimating the lava flow field.
Table 2. The total area of each estimated lava flow field (km2), as shown in , and the percentage of overlap area with respect to the USGS lava thickness map (%).
shows the topographic height change map due to the emplaced lava flow area by subtracting the elevations before and after eruption using LiDAR DEMs. The height difference could be utilized to estimate volume changes from the lava eruption using an annual or biannual time-series of DEM. The transection profiles () indicate that the height variations along the A-A’ line over the lava flow area shown in are increased by supplied lava in the thick delta area, whereas the high elevation area does not change significantly. Notably, the northern summit in the Kapoho crater collapsed, although it might have resulted in a height error in the provided DEM. The topographic slope is a critical parameter to estimate lava flows in future. The flow direction analyses, assuming that liquid lava flows from high to low elevations starting at the opening fissure, indicate that an abrupt change in the lava flow direction could be detected; however, in general, future lava flows would flow toward the eastern direction (). This implies that the newly formed lava area affected the roughness, slope, and height of the original topographic features.
Figure 6. (a) the profile shows the topographic heights of two LiDAR DEMs before (blue line) and after (red line) eruption along the A-A’ line to indicate the height change resulting from the lava flow. (b) flow direction analysis using slope information of the provided DEMs before (blue arrow) and after (red arrow) eruption. The arrow shows the flow direction and the size of the arrow indicates the flow velocity. The yellow symbol indicates the opening fissure 8 of eruptions in 2018.

4. Discussion
Monitoring the lava flow using the CCD analysis cannot provide real-time observations, such as LiDAR or UAV; however, it can help predict lava flow behaviors to ensure protection from future lava flows. Although we successfully mapped the lava flow field by CCD analysis using time-series coherence maps, there is a potential limitation in calculating the CCD image. As CCD analysis relies on a pair of coherence, the coherence should be maintained over the area of interest to detect surface changes. Thus, lava flow detection using CCD requires a short revisit cycle to prevent coherence degradation from the temperature and temporal baselines. Shorter temporal baselines, which are the time spans between two SAR observations, provide a more favorable condition for maintaining the coherence level. Accurately extracting the lava flow requires many time-series SAR images, including those before and after the volcanic eruption. Sentinel-1, a constellation mission comprising two twin satellites, can provide relatively short temporal baselines with a revisit cycle of 6 days, and this is sufficient to monitor lava flows using CCD. Additionally, interferometric phase noise caused by other decorrelation factors deteriorates the quality of the CCD map. The salt and pepper noises in could be explained as phase noise due to decorrelation factors, such as geometric and temporal baselines and volume scattering.
Another insight into the coherence level can be obtained from the scattering behavior of the radar signal according to the residual heat over lava areas. We noticed that the coherence map acquired in September () showed the entire lava flow area, whereas the map from the dataset in August () could not detect the lava flow field, even though the volcanic activity was almost completed. The omission of the lava flow field might be explained by the degree of solidification during the lava cooling process and residual heat retention by some heat source. The lava in September was cold enough to ensure a high coherence. However, coherence decorrelation occurred in August because the partial lava flow surfaces may ultimately be unsolidified by remnant heat, which prevents radar signal scattering. We generated a CCD map using coherence maps obtained on August 6–12 and September 11–17 that reflected different surface conditions based on the degree of lava cooling (). These two independent observations might represent each scattering condition based on the partially unsolidified status owing to some remnant heat or local surface displacement from the re-adjustment of the lava surface. The LST map obtained from the Landsat-8 TIRS optical sensor on August 4 and 11 was used to investigate the relationship between the coherence and temperature of the surface (). Random point sampling (1,500 points) inside the lava flow field boundary (red polygon in was selected for comparison. A scatter plot between coherence and LST, which has an interval of 1°C, is shown in . High coherence (>0.8) was observed at a relatively lower temperature (10–20°C). However, we could not find high coherence at temperatures greater than 20°C. The linear regression model suggests that coherence and surface temperature are inversely proportional with good agreement (coefficients of determination of>0.85). This implies that the surface temperature may be an interferometric decorrelation factor in lava flow monitoring. The lava lake in the crater was hot and the lava surface did not solidify until 4 September, although the volcanic eruption almost ended in August 2018 (Staff Citation2021). Additionally, thick water vapor owing to the high temperature of the lava might result in the degradation of local coherence. Alternatively, local displacement due to rapid surface re-adjustment could also lead to a loss of coherence.
Figure 7. (A) CCD analysis using coherence maps obtained on August 6 and 12, which reflect the surface condition after the volcanic activity, and those obtained on September 11 and 17, which correspond to lava flow characteristics after approximately one month of volcanic activity. The different color schemes from black to white in the lava field indicate that the coherence level may differ with respect to the lava flow status. (b) the estimated LST map using the Landsat-8 dataset acquired on August 4 and 13. The random point sampling (1,500 points) inside the lava flow field boundary (red polygon) was selected to examine the correlation between the coherence level and LST. (c) a scatter plot between the coherence and LST has an interval of 1°C. The linear regression model suggests that both observations are inversely proportional with good agreement (coefficients of determination were>0.85).

5. Conclusions
In this study, we applied CCD analysis to map the lava flow field caused by the Kilauea eruption in Hawaii in 2018 using Sentinel-1 SAR observations acquired from May 2 to 17 September 2018. The eruption created a lava flow field with an area of 33.3 km2, and this value was in good agreement (the percentage of overlap is approximately 90.2%) with that of the lava thickness map provided by the USGS. The mapping accuracy increased, except for the newly created lava flow area along the coastline. A time series of coherence maps, an intermediate product in CCD analysis, can be used to monitor changes in the lava flow field over time. This has important implications for mitigating future geohazards arising from lava flows.
The mapping of the lava flow using space-based SAR observations has the advantages of working with limited optical remote sensing data and mitigating the high logistic costs of field or airborne surveys, including UAV or drone remote sensing. The short revisit cycle of the satellite could provide more reliable information on lava flow. This proposed approach can also help to quantitatively extract new lava flow areas compared to existing approaches, such as those using amplitude data. Unfortunately, a satellite anomaly of Sentinel-1B occurred on 23 December 2021, and has been reported by the ESA. This implies that the coherence might be degraded because of an extended temporal baseline in the Sentinel-1 SAR observations. Several constellation SAR missions have been developed and used to observe the Earth surface. Using these satellite observations, CCD analysis can generate and update existing lava flow maps with frequent observations from space. If global SAR observation missions such as Sentinel-1 are available for interferometric purposes, CCD analysis could be utilized to monitor surface changes, such as any other lava flows, pyroclastic deposits, and agricultural crops. Updated lava flow maps can provide useful information for geologists, resulting in a better understanding of the lava flow model, and for government authorities to propose national land development measures.
Acknowledgments
The authors thank the European Space Agency (ESA) for providing Sentinel-1 data and the United States Geological Survey (USGS) for the SRTM DEM and Landsat 8 OLI/TIRS data.
Disclosure statement
No potential conflict of interest was reported by the authors.
Data availability statement
The data that support the findings of this study (i.e. the land cover and probability maps, respectively) are available from the corresponding author, SHH, upon reasonable request.
Additional information
Funding
References
- Arnold, D. W. D., J. Biggs, K. Anderson, S. Vallejo Vargas, G. Wadge, S. K. Ebmeier, M. F. Naranjo, and P. Mothes. 2017. “Decaying Lava Extrusion Rate at El Reventador Volcano, Ecuador, Measured Using High‐resolution Satellite Radar.” Journal of Geophysical Research: Solid Earth 122 (12): 9966–12. doi:10.1002/2017JB014580.
- Arnold, D. W. D., J. Biggs, H. R. Dietterich, S. Vallejo Vargas, G. Wadge, and P. Mothes. 2019. “Lava Flow Morphology at an Erupting Andesitic Stratovolcano: A Satellite Perspective on El Reventador, Ecuador.” Journal of Volcanology and Geothermal Research 372: 34–47.
- Arnold, D. W. D., J. Biggs, S. E. Geoff Wadge, H. M. Odbert, and M. P. Poland. 2016. “Dome Growth, Collapse, and Valley Fill at Soufrière Hills Volcano, Montserrat, from 1995 to 2013: Contributions from Satellite Radar Measurements of Topographic Change.” Geosphere 12 (4): 1300–1315.
- Arnold, D. W. D., J. Biggs, G. Wadge, and P. Mothes. 2018. “Using Satellite Radar Amplitude Imaging for Monitoring Syn-Eruptive Changes in Surface Morphology at an Ice-Capped Stratovolcano.” Remote Sensing of Environment 209: 480–488. doi:10.1016/j.rse.2018.02.040.
- Avdan, U., and G. Jovanovska. 2016. “Algorithm for Automated Mapping of Land Surface Temperature Using LANDSAT 8 Satellite Data.” Journal of Sensors 2016: 1–8.
- Bamler, R., and P. Hartl. 1998. “Synthetic Aperture Radar Interferometry.” Inverse Problems 14 (4): R1. doi:10.1088/0266-5611/14/4/001.
- Barberi, F., M. L. Carapezza, M. Valenza, and L. Villari. 1993. “The Control of Lava Flow During the 1991–1992 Eruption of Mt. Etna.” Journal of Volcanology and Geothermal Research 56 (1–2): 1–34.
- Blackett, M. 2014. “Early Analysis of Landsat-8 Thermal Infrared Sensor Imagery of Volcanic Activity.” Remote Sensing 6 (3): 2282–2295. doi:10.3390/rs6032282.
- Bouaraba, A., A. Belhadj-Aissa, and D. Closson. 2016. “Man-Made Change Detection Using High-Resolution Cosmo-Skymed SAR Interferometry.” Arabian Journal for Science and Engineering 41 (1): 201–208. doi:10.1007/s13369-015-1736-4.
- Bouaraba, A., A. Younsi, A. Belhadj Aissa, M. Acheroy, N. Milisavljevic, and D. Closson. 2012. “Robust Techniques for Coherent Change Detection Using COSMO-SkyMed SAR Images.” Progress in Electromagnetics Research M 22: 219–232. doi:10.2528/PIERM11110707.
- Brown, S. K., S. F. Jenkins, R. Sparks, H. Odbert, and M. R. Auker. 2017. “Volcanic Fatalities Database: Analysis of Volcanic Threat with Distance and Victim Classification.” Journal of Applied Volcanology 6 (1): 1–20.
- Bui, L. K., P. V. Le, P. D. Dao, N. Q. Long, H. V. Pham, H. H. Tran, and L. Xie. 2021. “Recent Land Deformation Detected by Sentinel-1A InSar Data (2016–2020) Over Hanoi, Vietnam, and the Relationship with Groundwater Level Change.” GIScience & Remote Sensing 58 (2): 161–179.
- Casalbore, D., F. Di Traglia, A. Bosman, C. Romagnoli, N. Casagli, and F. L. Chiocci. 2021. “Submarine and Subaerial Morphological Changes Associated with the 2014 Eruption at Stromboli Island.” Remote Sensing 13 (11): 2043.
- deGraffenried R, J. Hammer, H. Dietterich, R. Perroy, M. Patrick and T. Shea. 2021.“Evaluating lava flow propagation models with a case study from the 2018 eruption of Kīlauea Volcano, Hawaiʻi.“ Bull Volcanol 83(11). doi:10.1007/s00445-021-01492-x
- De Luca, D. F., C. Luca, M. Manzo, T. Nolesini, N. Casagli, R. Lanari, and F. Casu. 2021. “Joint Exploitation of Space-Borne and Ground-Based Multitemporal InSar Measurements for Volcano Monitoring: The Stromboli Volcano Case Study.” Remote Sensing of Environment 260: 112441.
- Dietterich, H. R., M. P. Poland, D. A. Schmidt, K. V. Cashman, D. R. Sherrod, and A. T. Espinosa. 2012. “Tracking Lava Flow Emplacement on the East Rift Zone of Kīlauea, Hawai ‘I, with Synthetic Aperture Radar Coherence.” Geochemistry, Geophysics, Geosystems 13: 5.
- Di, T., A. F. Federico, M. Favalli, T. Nolesini, and N. Casagli. 2020. “Catching Geomorphological Response to Volcanic Activity on Steep Slope Volcanoes Using Multi-Platform Remote Sensing.” Remote Sensing 12 (3): 438.
- Favalli, M., S. Tarquini, A. Fornaciai, and E. Boschi. 2009. “A New Approach to Risk Assessment of Lava Flow at Mount Etna.” Geology 37 (12): 1111–1114. doi:10.1130/G30187A.1.
- Fornaciai, D. F., A. F. Federico, D. Casalbore, M. Favalli, I. Manzella, C. Romagnoli, F. L. Latino Chiocci, P. Cole, T. Nolesini, and N. Casagli. 2022. “Subaerial-Submarine Morphological Changes at Stromboli Volcano (Italy) Induced by the 2019–2020 Eruptive Activity.” Geomorphology 400: 108093.
- Goldstein, R. M., and C. L. Werner. 1998. “Radar Interferogram Filtering for Geophysical Applications.” Geophysical Research Letters 25 (21): 4035–4038.
- González, P. J., M. Bagnardi, A. J. Hooper, Y. Larsen, P. Marinkovic, S. V. Samsonov, and T. J. Wright. 2015. “The 2014–2015 Eruption of Fogo Volcano: Geodetic Modeling of Sentinel‐1 TOPS Interferometry.” Geophysical Research Letters 42 (21): 9239–9246.
- Gracchi, T., C. Tacconi Stefanelli, G. Rossi, F. Di Traglia, T. Nolesini, L. Tanteri, and N. Casagli. 2022. “UAV-Based Multitemporal Remote Sensing Surveys of Volcano Unstable Flanks: A Case Study from Stromboli.” Remote Sensing 14 (10): 2489. doi:10.3390/rs14102489.
- Grassi, F., F. Mancini, E. Bassoli, and L. Vincenzi. 2022. “Contribution of Anthropogenic Consolidation Processes to Subsidence Phenomena from Multi-Temporal DInSar: A GIS-Based Approach.” GIScience & Remote Sensing 59 (1): 1901–1917. doi:10.1080/15481603.2022.2143683.
- Hanssen, R. F. 2001. Radar Interferometry: Data Interpretation and Error Analysis. Vol. 2. Dordrecht, The Netherlands: Kluwer Academic Publishers.
- Harris, A. J. 2015. “Basaltic lava flow hazard.” In Volcanic Hazards, Risks and Disasters, edited by J. F. Shroder, P. Papale,17–46. Amsterdam: Elsevier.
- Hawaiian-Volcano-Observatory. 2018. “Time Series of Kīlauea Volcano’s Lower East Rift Zone Lava-Flow Maps.” https://www.usgs.gov/media/videos/time-series-k-lauea-volcanos-lower-east-rift-zone-lava-flow-maps
- Hawaiian-Volcano-Observatory. 2019. “Kīlauea 2018 Lower East Rift Zone Lava Flow Thicknesses.” Accessed 31 July. https://www.usgs.gov/media/images/kilauea-2018-lower-east-rift-zone-lava-flow-thicknesses
- Hogoiu, O. 2022. “Science Toolbox Exploitation Platform.” Accessed 22 April. https://step.esa.int/
- Hong, S. H., and S. Wdowinski. 2013. “Multitemporal Multitrack Monitoring of Wetland Water Levels in the Florida Everglades Using ALOS PALSAR Data with Interferometric Processing.” IEEE Geoscience and Remote Sensing Letters 11 (8): 1355–1359.
- Hong, S. H., S. Wdowinski, and S. W. Kim. 2009. “Evaluation of TerraSar-X Observations for Wetland InSar Application.” IEEE Transactions on Geoscience and Remote Sensing 48 (2): 864–873.
- James, M. R., S. J. Lane, L. Wilson, and S. B. Corder. 2009. “Degassing at Low Magma-Viscosity Volcanoes: Quantifying the Transition Between Passive Bubble-Burst and Strombolian Eruption.” Journal of Volcanology and Geothermal Research 180 (2–4): 81–88.
- Jung J, D. Kim, M. Lavalle and S. Yun. Coherent Change Detection Using InSAR Temporal Decorrelation Model: A Case Study for Volcanic Ash Detection. IEEE Trans. Geosci. Remote Sensing 54 (10): 5765–5775. doi:10.1109/TGRS.2016.2572166
- Kauahikaua, J. 2007. “Lava Flow Hazard Assessment, as of August 2007, for Kīlauea East Rift Zone Eruptions, Hawai ‘I Island.” US Geological Survey Open-File Report 9: 1264.
- Klein, F. W. 1982. “Patterns of Historical Eruptions at Hawaiian Volcanoes.” Journal of Volcanology and Geothermal Research 12 (1–2): 1–35.
- Laverdiere, M., L. Yang, M. Tuttle, and C. Vaughan. 2020. ”Rapid Structure Detection in Support of Disaster Response: A Case Study of the 2018 Kilauea Volcano Eruption.” IGARSS 2020 - 2020 IEEE International Geoscience and Remote Sensing Symposium, Waikoloa, HI, USA. 26 Sept.-2 Oct. 2020, 6826–6829. doi:10.1109/IGARSS39084.2020.9324160
- Lê, T. T., J. L. Froger, A. Hrysiewicz, and H. Thai Pham. 2019. Multitemporal Insar Coherence Change Analysis: Application to Volcanic Eruption Monitoring. Paper presented at the 2019 10th International Workshop on the Analysis of Multitemporal Remote Sensing Images (MultiTemp), 05-07 August 2019, Shanghai, China. doi:10.1109/Multi-Temp.2019.8866922
- Lu, Z., E. Fielding, M. R. Patrick, and C. M. Trautwein. 2003. “Estimating Lava Volume by Precision Combination of Multiple Baseline Spaceborne and Airborne Interferometric Synthetic Aperture Radar: The 1997 Eruption of Okmok Volcano, Alaska.” IEEE Transactions on Geoscience and Remote Sensing 41 (6): 1428–1436.
- Lyu, M., Y. Ke, L. Guo, X. Li, L. Zhu, H. Gong, and C. Constantinos. 2020. “Change in Regional Land Subsidence in Beijing After South-To-North Water Diversion Project Observed Using Satellite Radar Interferometry.” GIScience & Remote Sensing 57 (1): 140–156.
- Meyer, D., and M. Riechert. 2019. “Open Source QGIS Toolkit for the Advanced Research WRF Modelling System.” Environmental Modelling & Software 112: 166–178. doi:10.1016/j.envsoft.2018.10.018.
- Moreira, A., P. Prats-Iraola, M. Younis, G. Krieger, I. Hajnsek, and K. P. Papathanassiou. 2013. “A Tutorial on Synthetic Aperture Radar.” IEEE Geoscience and Remote Sensing Magazine 1 (1): 6–43.
- Mosbrucker, A. R., M. H. Zoeller, and D. W. Ramsey. 2020. “Digital elevation model of Kīlauea Volcano, Hawaiʻi, based on July 2019 airborne lidar surveys.“ US Geological Survey Data Release. doi:10.5066/P9F1ZU8O.
- Nolesini, D. F., T. N. Federico, A. Ciampalini, L. Solari, W. Frodella, F. Bellotti, A. Fumagalli, G. De Rosa, and N. Casagli. 2018. “Tracking Morphological Changes and Slope Instability Using Spaceborne and Ground-Based SAR Data.” Geomorphology 300: 95–112.
- Patrick, M. R., H. R. Dietterich, J. J. Lyons, A. K. Diefenbach, C. Parcheta, K. R. Anderson, A. Namiki, I. Sumita, B. Shiro, and J. P. Kauahikaua. 2019. “Cyclic Lava Effusion During the 2018 Eruption of Kīlauea Volcano.” Science 366 (eaay9070).
- Poland, M. P. 2022. “Synthetic Aperture Radar Volcanic Flow Maps (SAR VFMs): A Simple Method for Rapid Identification and Mapping of Volcanic Mass Flows.” Bulletin of Volcanology 84 (3): 1–11.
- Richter, N., M. Favalli, E. de Zeeuw-van Dalfsen, A. Fornaciai, R. M. da Silva Fernandes, N. M. Pérez, J. Levy, S. S. Silva Victória, and T. R. Walter. 2016. “Lava Flow Hazard at Fogo Volcano, Cabo Verde, Before and After the 2014–2015 Eruption.” Natural Hazards and Earth System Sciences 16 (8): 1925–1951.
- Romero, J. E., M. Burton, F. Cáceres, J. Taddeucci, R. Civico, T. Ricci, M. J. Pankhurst, et al. 2022. “The Initial Phase of the 2021 Cumbre Vieja Ridge Eruption (Canary Islands): Products and Dynamics Controlling Edifice Growth and Collapse.” Journal of Volcanology and Geothermal Research 431: 107642.
- Saepuloh, A., K. Koike, M. Omura, M. Iguchi, and A. Setiawan. 2010. “SAR-And Gravity Change-Based Characterization of the Distribution Pattern of Pyroclastic Flow Deposits at Mt. Merapi During the Past 10 Years.” Bulletin of Volcanology 72 (2): 221–232.
- Saepuloh, A., K. Koike, M. Urai, and J. Tetuko Sri Sumantyo. 2015. “Identifying Surface Materials on an Active Volcano by Deriving Dielectric Permittivity from Polarimetric SAR Data.” IEEE Geoscience and Remote Sensing Letters 12 (8): 1620–1624. doi:10.1109/LGRS.2015.2415871.
- Soule S. A., M. Zoeller and C. Parcheta. 2021. Submarine lava deltas of the 2018 eruption of Kīlauea volcano. Bull Volcanol 83 (4). doi:10.1007/s00445-020-01424-1
- Staff, H. V. O. 2021 . “Kīlauea Volcano–2018 Summit and Lower East Rift Zone (LERZ), Brief Overview of Events April 17 to October 5, 2018.” https://volcanoes.usgs.gov/vsc/file_mngr/file-179/Chronology%20of%20events%202018.pdf
- Tighe, M. L., and D. Chamberlain. 2009. Accuracy Comparison of the SRTM, ASTER, NED, NEXTMAP® USA Digital Terrain Model Over Several USA Study Sites. Paper presented at the ASPRS/MAPPS 2009 Fall Conference, San Antonia, Texas.
- Tilling, R. I., P. R. Vogt, H. Spall, and P. Mathieux. 2nd ed. 1994. “This Dynamic Planet World Map of Volcanoes, Earthquakes, Impact Craters, and Plate Tectonics.“ U.S. Geological Survey map .
- Touzi, R., A. Lopes, J. Bruniquel, and P. W. Vachon. 1999. “Coherence Estimation for SAR Imagery.” IEEE Transactions on Geoscience and Remote Sensing 37 (1): 135–149.
- Turner, N. R., R. L. Perroy, and K. Hon. 2017. “Lava Flow Hazard Prediction and Monitoring with UAS: A Case Study from the 2014–2015 Pāhoa Lava Flow Crisis, Hawai ‘I.” Journal of Applied Volcanology 6 (1): 1–11.
- Vallance, J. W., S. P. Schilling, O. Matías, W. I. Rose, and M. M. Howell. 2001. “Volcano Hazards at Fuego and Acatenango, Guatemala.” US Geological Survey Open-File Report 01–431, 24 pp. 4 plates.
- Wadge, G., P. Cole, A. Stinton, J. C. Komorowski, R. Stewart, A. C. Toombs, and Y. Legendre. 2011. “Rapid Topographic Change Measured by High-Resolution Satellite Radar at Soufriere Hills Volcano, Montserrat, 2008–2010.” Journal of Volcanology and Geothermal Research 199 (1–2): 142–152. doi:10.1016/j.jvolgeores.2010.10.011.
- Wang, S., G. Zhang, Z. Chen, H. Cui, Y. Zheng, Z. Xu, and Q. Li. 2022. “Surface Deformation Extraction from Small Baseline Subset Synthetic Aperture Radar Interferometry (SBAS-InSar) Using Coherence-Optimized Baseline Combinations.” GIScience & Remote Sensing 59 (1): 295–309.
- Washaya P., T. Balz and B. Mohamadi. 2018.“Coherence Change-Detection with Sentinel-1 for Natural and Anthropogenic Disaster Monitoring in Urban Areas.“ Remote Sensing 10 (7): 1026. doi:10.3390/rs10071026
- Yanjie, Z., and V. Prinet. 2004. InSar Coherence Estimation. Paper presented at the IGARSS 2004. 2004 IEEE International Geoscience and Remote Sensing Symposium, Anchorage, AK.
- Zebker, H. A., P. Rosen, S. Hensley, and P. J. Mouginis-Mark. 1996. “Analysis of Active Lava Flows on Kilauea Volcano, Hawaii, Using SIR-C Radar Correlation Measurements.” Geology 24 (6): 495–498.
- Zuhlke, M., N. Fomferra, C. Brockmann, M. Peters, L. Veci, J. Malik, and P. Regner. 2015. “SNAP (Sentinel Application Platform) and the ESA Sentinel 3 Toolbox.” Sentinel-3 for Science Workshop 734: 21.