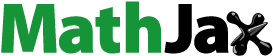
ABSTRACT
Mangrove ecosystems are one of the most diverse and productive marine ecosystems around the world, although losses of global mangrove area have been occurring over the past decades. Therefore, tracking spatio-temporal changes and assessing the current state are essential for mangroves conservation. To solve the issues of inaccurate detection results of single algorithms and those limited to historical change detection, this study proposes the detect–monitor–predict (DMP) framework of mangroves for detecting time-series historical changes, monitoring abrupt near-real-time events, and predicting future trends in Beibu Gulf, China, through the synergetic use of multiple detection change algorithms. This study further developed a method for extracting mangroves using multi-source inter-annual time-series spectral indices images, and evaluated the performance of twenty-one spectral indices for capturing expansion events of mangroves. Finally, this study reveals the spatio-temporal dynamics of mangroves in Beibu Gulf from 1986 to 2021. In this study, we found that our method could extract mangrove growth regions from 1986 to 2021, and achieved 0.887 overall accuracy, which proved that this method is able to rapidly extract large-scale mangroves without field-based samples. We confirmed that the normalized difference vegetation index and tasseled cap angle outperform other spectral indexes in capturing mangrove expansion changes, while enhanced vegetation index and soil-adjusted vegetation index capture the change events with a time delay. This study revealed that mangrove changes displayed historical changes in the hierarchical gradient from land to sea with an average annual expansion of 239.822 ha in the Beibu Gulf during 1986–2021, detected slight improvements and deteriorations of some contemporary mangroves, and predicted 72.778% of mangroves with good growth conditions in the future.
1. Introduction
Mangroves are one of the most productive natural ecosystems globally, and are considered to be most efficient in carbon sequestration and climate change mitigation (Murdiyarso et al. Citation2015). Mangrove forests play an important role in the global C cycle, accounting for 10–15% of all coastal C storage despite covering less than 0.5% of the global coastal areas (Atwood et al. Citation2017). Mangrove conservation and restoration are closely related to the implementation of the Sustainable Development Goals (SDGs) (Weise et al. Citation2020). Recent studies have reported that global mangrove ecosystems have suffered degradation under the dual impacts of climate change and human activities (Pirasteh et al. Citation2021; Li et al. Citation2020). Globally, mangroves have declined by 30–50% during the last century, and it is estimated that these ecosystems may disappear within 100 years (Murdiyarso et al. Citation2015). Therefore, accurate and repeatable monitoring of historical changes, assessing current status, and abrupt changes, and predicting future trends are crucial to achieve the protection and restoration, sustainable management, and development of mangrove ecosystems.
Remote sensing techniques have become an important tool for monitoring dynamic changes of mangroves (Zhu Citation2017; Younes Cárdenas, Joyce, and Maier Citation2017; Thomas et al. Citation2018), including multi-temporal image classifications and time-series trajectory analysis methods (Halabisky et al. Citation2016). The former analyzes the spatio-temporal change patterns in mangroves using the change information about the conversion of land cover types from the classification results of multi-temporal remote sensing images (Navarro et al. Citation2021; Zhang et al. Citation2021; Jia et al. Citation2018; Ma et al. Citation2019). However, this method not only requires a large number of training samples from different years (Navarro et al. Citation2021), but also cannot track the succession process and obtain the magnitude of changes (Woodcock et al. Citation2020). Trajectory analysis methods could accurately detect the minor and long-term changes in mangroves without training samples and numerous classifiers (Baloloy et al. Citation2020). However, the rapid extraction of mangroves using time-series spectral index images and change detection approaches still faces great challenges.
Spectral indices have different capabilities for capturing change events of mangroves, such as growth, disturbance, loss, restoration, etc., due to the different spectral sensitivities. The normalized difference vegetation index (NDVI) is a widely used spectral index for detecting dynamic changes in mangroves (Awty-Carroll et al. Citation2019; de Jong et al. Citation2021; Otero et al. Citation2017); some popular spectral indices have demonstrated strong performance in monitoring mangrove dynamics, such as the enhanced vegetation index (EVI) (Zhu, Liao, and Shen Citation2021), normalized difference moisture index (NDMI) (Aljahdali, Munawar, and Khan Citation2021), etc. Moreover, some scholars have proposed several novel spectral indices, which are more sensitive to mangroves than traditional vegetation indices, for the classification and extraction of mangroves. For example, the mangrove vegetation index (MVI) and enhanced mangrove vegetation index (EMVI) can distinguish terrestrial vegetation from mangroves (Baloloy et al. Citation2020; Yang et al. Citation2022). However, the performance of different spectral indices for capturing mangrove time-series change trajectories still lacks a systematic evaluation under the eliminating the effect of tidal submergence, especially those vegetation indices sensitive to mangroves.
Currently, different change detection algorithms with the time-series remote sensing images have been applied to detect gradual and abrupt changes in hydrology (Liang et al. Citation2020; Xia et al. Citation2021), vegetation (Fu et al. Citation2022), and climate (Yoo et al. Citation2018). Several studies have demonstrated that the LandTrendr algorithm represents an important tool for monitoring dynamic changes in other wetland types (de Jong et al. Citation2021; Zhu et al. Citation2019; Fu et al. Citation2022). Continuous change detection and classification (CCDC) algorithms (Zhu and Woodcock Citation2014) and breaks for the additive season and trend (BFAST) algorithms (Verbesselt et al. Citation2010, Citation2010) have been used to detect change periods of mangrove cutting, and the trends in regeneration rates (Awty-Carroll et al. Citation2019; Otero et al. Citation2017). Mann – Kendall significance tests (MKs) and Mann – Kendall mutation tests (MKm) have been used to assess the significance of the trends and mutation detection in hydrological and vegetation time series analysis, respectively (Hamed Citation2008; Figueira Branco et al. Citation2019; Wu et al. Citation2022). However, the different algorithms have their own advantages and disadvantages in capturing and representing the time series change characteristic information. They may only obtain specific change information or be limited by the length of the time series (Verbesselt, Zeileis, and Herold Citation2012). In addition, previous studies have reported that a single optimal algorithm may not comprehensively display the spatio-temporal changes in mangrove forests, and a more reasonable approach should be the advantageous trade-off between multiple algorithms (Cohen et al. Citation2017; Bianco, Ciocca, and Schettini Citation2017). Inspired by these ideas, we aim to develop a new method that could track dynamic historical changes, assess the current status, and predict future trends of mangroves by combining the advantages of multiple change-detection algorithms.
The essential objectives of this study were as follows: (1) develop a new method based on multi-source inter-annual time-series spectral indices to extract historical large-scale mangroves; (2) evaluate the performance of twenty-one spectral indices for monitoring change periods and intensity, and spatial expansion changes in mangroves; (3) propose a detect–monitor–predict (DMP) framework of mangroves to track historical changes, monitor the current state and abrupt events, and predict future developments; (4) reveal spatio-temporal trajectories of mangroves from 1986 to 2021 in Beibu Gulf, and quantitatively evaluate the area changes in different regions and at different phases.
2. Study area and data source
2.1 Study area
The Beibu Gulf is located in tropical and subtropical regions northwest of the South China Sea (), which is the largest remaining mangrove distribution area in China (Gan et al. Citation2013). The mangrove communities in the study area are representative and typical of the Pacific West Coast. There are two National Nature Reserves (NNR), one Provincial Nature Reserves (PNR), and three Local Nature Reserves (LNR) in the coastal areas of Beibu Gulf. A large amount of estuarine sediment deposition provides suitable conditions for mangrove growth; the Chinese government has been carrying out a range of protection and restoration actions of mangroves since 1980. However, mangroves in the study area have still been suffering from varying degrees of damage under the influence of human activities, such as land reclamation, mariculture, deforestation, etc. (Long et al. Citation2022). Therefore, the study area represents great research value in terms of monitoring mangrove expansion and disturbance, which could provide implications for global mangrove conservation and management.
2.2 Data acquisition and processing
In this study, we used atmospherically corrected multi-source remote sensing images of the Landsat 5 TM, Landsat 7 ETM+, and Landsat 8 OLI surface reflectance from 1986 to 2021 and the Sentinel-2 multispectral instrument (MSI) from 2018 to 2021 on the Google Earth Engine platform (Gorelick et al. Citation2017) () and coordinated the surface reflectivity of Landsat TM and ETM +. In order to preserve good quality observations, QA60 and CFmask pixel QA band were used to mask the cloud and cloud shadow in the Sentinel-2 and Landsat images, respectively. We counted the number of good quality observations in each location of an individual pixel and their percentage frequency (). In addition, The SRTM DEM acts as an assistant to extract mangrove wetlands.
Table 1. Summary of time-series remote sensing images from different platforms.
We conducted field surveys and UAV images collection of mangroves in the study area on 19–25 November, 2020, 8–14 January and 4–16 April, 2021, 17–24 August, 2021, and 17–28 May, 2022. There are two main methods: (1) the longitude and latitude coordinates of mangrove plots were recorded using a handheld centimeter-level positioning accuracy RTK; (2) a multi-spectral camera mounted on a DJI Phantom 4 Pro was used for sample aerial photography, with a flight height of 30-60 m. The UAV images were interpreted to determine mangrove vegetation. Meanwhile, the following classification products were used to validate the extracted mangrove regions: (A) Jia et al. (Citation2018) mapped Chinese mangrove classification products from 1990, 2000, 2010, and 2015 with 30 m spatial resolution and 87% to 92% overall accuracy (OA); (B) Zhao and Qin (Citation2020) extracted the 2017 Chinese mangrove region (resolution 10 m, OA 92.4%); (C) 10 m global mangrove classification products of 2018–2020 (OA 92.4%) were obtained from Scientific Data Bank (https://www.scidb.cn).
3. Methods
The technical workflow of this study is shown in , including four primary steps. First, Landsat and Sentinel-2 satellite images were processed, and intra-annual and inter-annual time-series spectral indices images from 1986 to 2021 were reconstructed. Second, mangrove growth regions (MGRs) in the study area were extracted by threshold segmentation using the time-series reconstructed spectral indices images. Meanwhile, we evaluated the performance of twenty-one spectral indices for monitoring mangrove changes in Beibu Gulf, and selected suitable spectral indices to analyze the expansion dynamics of mangrove forests. Finally, this study proposes a DMP framework to detect historical changes, monitor the current state and abrupt events, and predict the future development trends of mangroves. We extracted change information using MKm, LandTrendr, BFAST, and BFAST Monitor algorithms to track the historical change process of mangroves. Then, the BFAST Monitor algorithm with stable period of history (SPH) observations was used to monitor structural changes in mangroves, including minor and near-real-time changes. MKs, Sen, and Hurst algorithms were used to assess the significance of trends, slope, and persistence of the time series, and predict future trends.
3.1 Reconstruction of spectral index time-series datasets
This study reconstructed time-series spectral index () datasets using Landsat and Sentinel-2 images from 1986 to 2021. To weaken the effect of tidal intrusions into the coastal zone on the spectral reflection variation of the mangrove canopy, three inter-annual time-series images (EquationEquation (1)(1)
(1) ) were created for each spectral index based on the GEE platform using maximum/mean/median spectral index composition (Jia et al. Citation2021). Moreover, we used the data correction method (EquationEquation (2)
(2)
(2) ) and maximum spectral index value within one year to remove tide-influenced observations from the intra-annual time series. The inter-annual and intra-annual time series images were resampled to 30 m on the GEE platform.
where SIi and SIA(i) are the time series original spectral index and inter-annual spectral index composition images, respectively.
where SIIA(i) is the intra-annual time series spectral index in the i-th year, k is the correction coefficient, and the value range of k was determined to be 0.6–0.8 by many repeated experiments. The higher k values indicate that EquationEquation (2)(2)
(2) removes more tide-influenced observations, and fewer high-quality observations remain; a is the spectral index correction constant, with the specific range shown in ; and f(x, y) represents the processing of data correction for the intra-annual time series spectral index images using the maximum spectral value in a year.
3.2 Rapid extraction of mangrove growth regions using threshold segmentation method
This study proposes a threshold segmentation method based on the GEE platform using multi-source inter-annual time-series spectral indices images to implement mangrove region extraction. The process is divided into three main steps: (1) MVI time-series images were reconstructed using EquationEquation (1)(1)
(1) from Landsat and Sentinel-2 images; (2) the potential mangrove regions were extracted each year using the MVI time-series images based on EquationEquation (3)
(3)
(3) , then we further used the NDWI and DEM data to remove the inland marshes in the potential mangrove regions; (3)We stacked potential mangrove regions without inland marshes from 1986 to 2021, counted the frequency of each pixel identified as mangroves in all regions, and extracted the final mangrove growth regions (MGRs) using EquationEquation (4)
(4)
(4) .
where MVIA(i) and NDWIA(i) are the spectral values of MVI and NDWI in the i-th year through maximum/mean/median spectral index composition, respectively; T is the threshold value; F(·) represents the filtering of the pixels that satisfy the condition; PMRsensor, i is the potential mangrove regions extracted in the i-th year using Landsat and Sentinel-2 images; and MGRs is the regions where had existed mangroves or have been existing mangroves.
3.3 Monitoring the spatio-temporal dynamics of mangroves
To obtain more accurate spatial and temporal information on mangroves and to monitor mangrove dynamics, this study proposes a DMP framework (EquationEquation (5)(5)
(5) ) through the combination of LandTrendr (Kennedy, Yang, and Cohen Citation2010), MKs (Hamed Citation2008), MKm (Wu et al. Citation2022), BFAST (Verbesselt et al. Citation2010), BFAST Monitor (Verbesselt, Zeileis, and Herold Citation2012), Sen (Jiang et al. Citation2015), and Hurst (Zhu, Liao, and Shen Citation2021) algorithms to detect historical changes, monitoring near-real-time status, and predicting future trends in mangrove areas in Beibu Gulf. This study summarizes the algorithm components and change information in the DMP framework () and provides the final parameter values of the LandTrendr algorithm ().
where S is the detection results of the DMP framework in the spatial domain; SD represents the detection of historical changes in the spatial domain; SM represents the near-real-time monitoring in the spatial domain; SP represents a future prediction in the spatial domain; t represents the time series of the pixel in the entire region; t1 and t2 represent the time series of pixels in the detection results of the LandTrendr algorithm that change drastically and have large detection errors, respectively; t3 represents the time series of the prediction period; t1, t2, and t3∈t. y denote the change information in the time domain obtained using the LandTrendr algorithm; c1 represents the comparison of change information between different algorithms in the time domain; and c2 represents the correction of detection results with large errors.
The DMP-based dynamics of mangroves were mainly covered in three parts in this study ():
The LandTrendr algorithm and MKm were used to determine the expansion years (mutations) (y) and clarify the spatial trends (c1) of mangroves. Then, the BFAST algorithm was further used to correct the error change information detected by the LandTrendr algorithm, and analyze the change characteristics (c2) of the time series to track the historical spatio-temporal gradient changes in mangroves (SD).
BFAST Monitor was used for detecting structural changes and monitoring near-real-time changes in the mangrove forest (SM) based on the intra-annual monitoring periods provided by the BFAST algorithm.
A combination of MKs, Sen, and Hurst methods was used to obtain the time series change information (significance, slope, and sustainability) of mangroves, and further predict the spatial distribution (SP) and percentage of the different trends in mangroves in the future.
3.5 Accuracy assessment
Expansion Analysis. This study applied the urban expansion index to quantitatively assess the rate and intensity of mangrove expansion from 1986 to 2021. Annual expansion (AE) represents the yearly area of mangrove expansion in a certain period and enables the comparison of different periods of expansion in a region (Terfa et al. Citation2019). The intensity index of urban expansion (UERi) could indicate the stage change rate of the mangrove area of each spatial unit (Wang et al. Citation2020). AE and UERi are calculated using EquationEquation (6)(6)
(6) and EquationEquation (7)
(7)
(7) .
where At1 and At2 are the mangrove areas at times t1 and t2, respectively.
where UERi is the intensity index of mangrove expansion, and
are the mangrove area of the spatial unit, i, at times t1 and t2, respectively. Δt is the duration of the research.
To perform an accurate assessment of extracting mangroves, this study evaluated the accuracy of extracting MGRs from 1986 to 2021 using validation points. Meanwhile, we conducted accuracy validation for the potential mangrove regions by interval of 5 year. The validation points were derived from multiple datasets: (1) field measurements and manual selection from UAV images during 2020–2021; (2) randomly producing from mangrove classification products and removing misclassified sample points; (3) visual interpretation and manual selection from Landsat and Sentinel-2 historical images. We established a validation dataset, including 1523 mangrove points and 1037 non-mangrove points ().
4. Results
4.1 Accuracy assessment of mangrove extraction
This study verified the extraction accuracy of mangroves in the ten typical regions of Beibu Gulf. As detailed in , our method finely extracted the MGRs of Beibu Gulf from 1986 to 2021 and produced an overall accuracy of 0.887. The MGRs of Zhenzhu Bay presented the highest overall accuracy of 0.953, whereas Beihai Bay obtained the lowest accuracy of 0.857. The overall accuracy of the potential mangrove areas extracted by the interval of 5 years was above 0.86, with the highest accuracy in 2021, indicating that the addition of Sentinel 2 images allowed our method to identify potential mangroves more accurately. Moreover, the area of potential mangroves extracted by our method was slightly larger than the previous classification products in the same year (). However, the area of the potential mangrove, consistent with the area change of classification products, showed a trend in the significant increase from 1990 to 2015 and a slowdown in expansion from 2015 to 2020 in mangroves. Two datasets had similar area changes for each typical region at the same interval of time. In addition, our method could quickly identify and map the mangrove regions without training samples, which was easy to implement on the GEE platform. The MGRs obtained by our method could be further used to detect the long-term dynamics of mangroves.
Figure 5. The potential mangrove area obtained by the threshold segmentation method in comparison with Jia et al. and Yang et al. classifications from 1990 to 2020. (a) Changes in mangrove area in ten typical regions: I-i, mangrove area in the i-th year based on classification products; II-i, potential mangrove area in the i-th year based on the method; A, Zhenzhu Bay; B, Fangcheng Bay; C, Maowei Sea; D, Dafeng River; E, Beihai Bay; F, Tieshan Port; G, Dandou Sea; H, Yingluo Bay; I, Anpu Port; J, Danzhou. (b) Changes in mangrove area in Beibu Gulf.

Table 2. The Overall accuracy and kappa coefficient of extracting MGRs in ten typical regions of Beibu Gulf from 1986 to 2021.
4.2 Evaluation of the effect of spectral indices on monitoring mangrove changes
As shown in , the mangroves displayed a prominent characteristic of hierarchical spatial expansion. The detection results of the traditional spectral indices were generally better than the sensitive spectral indices for mangroves. In the former, NIR could not accurately identify young mangroves expanding in 2016–2021. The detection results of NDVI, EVI, TCA, WAVI, SAVI, TCG, and WFI exhibited minor differences in their detection results over time. However, they could obtain accurate years and trends of mangrove expansion. Among the latter, CMRI, MVI, and MI were the more effective in identifying mangrove expansion, and other spectral indices could not significantly map the dynamic of mangroves. We filtered the best indices for further comparison.
Figure 6. The change detection results of twenty-one spectral indices based on the LandTrendr algorithm in the major mangrove area of Golden Bay Mangrove Reserves of Beihai Bay.

In this study, we randomly selected 845 mangrove points based on historical images and previous classification products of the study area, and visually interpreted their expansion years. As shown in , we found that the annual expansion curves of mangroves from the ten spectral indices displayed similar trends to the reference curves. MI, TCG, WAVI, and WFI detected mangrove expansion less frequently than the reference in 1990–1998 and produced higher peaks in 2012 or 2018, indicating a significant bias in accurately detecting expansion events. From , it can be seen that the deviations in the NDVI and TCA were within five years of each other, which could accurately map the spatial expansion of mangroves. In contrast, deviations in the other four spectral indices in 10–20 years had a higher frequency.
Figure 7. The detection change results of mangroves using ten spectral indices. (a) Frequency of expansion years. The reference is the frequency of expansion years based on visual interpretation. (b) Deviation distribution of the expansion years detected by the six spectral indices (normalized difference vegetation index, enhanced vegetation index, tasseled cap angle, soil-adjusted vegetation index, combined mangrove recognition index, and mangrove vegetation index) and visual interpretation.

We selected four typical areas to examine the accuracy of detecting mangrove expansion between the NDVI and TCA. As can be seen from the frequency percentage curves in , trends in the frequency percentage of expansion (mutation) events detected by the TCA and NDVI were similar, demonstrating high confidence in the results. However, the TCA detected a much lower frequency of expansion events in 1987, with a peak during 1986–1990. This indicated that the TCA failed to accurately differentiate between mangroves before 1987 and those that expanded in 1987–1990. Therefore, the NDVI is more suitable for monitoring the dynamics of mangroves than the TCA.
4.3 DMP-based spatio-temporal dynamics of mangroves
4.3.1 Tracking historical changes
Quantitative assessment. , and indicate a general increasing trend in the inter-annual gain of mangroves from 1988 to 2020. The expansion of mangroves could be divided into two periods. From 1988 to 2009, the expansion was slow, while from 2010 to 2020, the expansion was more rapid. The AE exhibited an “up – down” trend, with an AE of 239.822 ha/year from 1988 to 2020. The overall trend of UERi was similar to that of AE, but its magnitude was lower. (1) There were significant differences in the expansion rate between the fastest and slowest regions during the study period. The Maowei Sea was the fastest expanding region with an AE of 47.808 ha/year and UERi of 0.046, whereas the AE in Dandou Sea was the lowest with 10.413 ha/year. (2) The fastest period of expansion was from 2011 to 2015, with an AE of 499.893 ha/year. During this period, The gain area of 136.573 in Maoweihai and 66.869 ha/year in Beihai was much higher than in other regions.
Figure 9. The inter-annual gain area from 1988–2020 (a); annual expansion (AE) (b); and the expansion index (UERi) (c) for all typical regions from 1988 to 2020.

Table 3. Annual expansion (AE) of mangroves for ten typical regions during 1988–2020 (ha/year).
Table 4. Evaluating the intensity of change in mangroves for all typical regions from 1988 to 2020 using the expansion index (UERi).
Temporal and spatial mangrove expansion. The distribution of expansion years displayed a pattern of hierarchical change, showing the process of mangrove forests formation from nonexistence to existence. The mangroves in Zhenzhu Bay () and Beihai Bay () showed a radial spread, with the existing mangroves as the center. Initially, small patches emerged and subsequently merged with other patches, forming larger patches that continued to expand outward. The narrowly distributed mangroves in Maowei Sea () and Tieshan Port () were expanding from land to sea. We found that the spatial change in mangroves was influenced by mudflats and seawater height, which caused changes in expansion direction and a decline in the expansion rate.
Figure 10. Spatio-temporal characteristics of mangrove forests in Beibu Gulf: (a) Beibu Gulf; (b) Zhenzhu Bay; (c) Maowei Sea; (d) Tieshan Port; and (e) Beihai Sea.

As shown in , we used the DMP framework to analyze the spatial expansion process of mangroves and selected points P1, P2, and P3 to reflect the temporal profiles from 1986 to 2021. In the spatial domain, the result of the LandTrendr algorithm () showed a hierarchical change in mangroves in Anpu Port, which was consistent with that of MKm (). However, deviations were observed in the results using the LandTrendr algorithm in , and after correction, the expansion which occurred in this region from 1991 to 2000 presented a more evident pattern of hierarchical change without any noise. In the time domain, the year of expansion detected by the LandTrendr algorithm at P1 exhibited a delay deviation, whereas that identified by the BFAST and BFAST Monitor algorithms identified the actual change year. The consistent expansion years were detected at P2 and P3 by LandTrendr, BFAST, and BFAST Monitor algorithms. The above results showed that MKm could corroborate the expansion trend in space, and the BFAST and BFAST Monitor algorithms effectively confirmed and corrected the change information (years, magnitude, and duration) with deviations from the results of the LandTrendr algorithm.
Figure 11. Historical change process of mangroves detected based on MKm, LandTrendr, BFAST, and BFAST Monitor algorithms: (a) the expansion years of mangroves gained by the LandTrendr algorithm in Anpu Port; (b) information for the year corresponding to the intersections of UFk and UBk; (A1) region with deviated expansion years obtained by LandTrendr algorithm; (A2) region after correction of the expansion year by BFAST algorithm. PI, point of intersection; LT, LandTrendr; BF, BFAST; BFM, BFAST Monitor; BFM, BFAST Monitor; AF, algorithm fitting; SI, significant improvement; CL, confidence level; PI, point of intersection.

The trend component fitted by the BFAST algorithm showed that the expansion process exhibited obvious features in the time series. Before the expansion, the spectral index values of the time series were consistently low, and the region was a mudflat at this time; after the expansion, the values increased rapidly, indicating that the mangroves started to grow; finally, the values fluctuated and stabilized, indicating a steady growth of the mangroves. Moreover, after the apparent improvements in P1, P2, and P3 (UFk>1.96), UFk showed a monotonic upward trend, indicating that the mangroves were still maintaining their growth during the study period.
4.4.2 Monitoring the current changes
Knowledge of the study area was provided based on historical detection; therefore, this study selected regions B () and C () for near-real-time monitoring. These regions had earlier expansion years and longer SPH. As shown in , we determined the start of the monitoring period for regions B and C based on the expansion years and magnitudes obtained by the LandTrendr and BFAST algorithms. indicates that the magnitudes monitored by the BFAST Monitor algorithm at B and C ranged from −0.32 to 0.19 and −0.15 to 0.14, respectively, much smaller than those during growth, showing that the BFAST Monitor algorithm could detect the drastic changes in magnitude after mangrove expansion and the minor changes in adult mangroves. In the time domain, the monitoring results for the BFAST Monitor algorithm showed that the time series of P1 started to stabilize earlier and had a long SPH, and it monitored a structural change on day 305 of 2018; the time series of P2 had a short SPH due to instability before 2018, but the BFAST Monitor algorithm still exhibited a slight recession. Therefore, when the time series was sufficient and stable, the start of the monitoring period could be shifted back close to when new observations were provided for near-real-time monitoring. The above results show that slight improvements and deteriorations of some of the mangroves were currently occurring in Beibu Gulf.
4.4.3 Predicting future trends
MKs, Sen, Hurst, and time series data from 2013 to 2021 were used for predicting the future trends of mangroves in Beibu Gulf, which were classified into nine types (), and the proportion of each type in the typical regions was counted ( and ).
Figure 13. Predicted results using MKs, Sen, Hurst, and 2013–2021 time series data in each typical region: (a) Beibu Gulf; (b) Zhenzhu Bay; (c) Fangcheng Bay; (d) Yingluo Bay; (e) Anpu Port; (f) Tieshan Port; (g) Dandou Sea; (h) Danzhou Port; (i) Maowei Sea; (j) Dafeng River; and (k) Beihai Bay.

Table 5. Classification of spatio-temporal trends in mangrove areas.
Table 6. Summary of area percentages of each trend type in ten typical regions, and Beibu Gulf overall.
According to and , we found that 72.778% of areas in Beibu Gulf showed sustainable improvements in mangroves, while only 7.464% of areas demonstrated sustainable deterioration. This indicates that mangroves in Beibu Gulf would sustainably improve in the future. The Maowei Sea, Dafeng River, and Beibu Bay, which are adjacent and distributed in the northern part of the Beibu Gulf, had the highest percentages of sustainable and significant improvements in mangroves, with 75.349%, 63.482%, and 59.340%, respectively. The distribution of different trend types had some notable features. The unsustained slight improvement and degradation mainly occurred in areas inside the mangroves (B1, B2, C2, D2, E1, E2, F2, H1, and H2). Sustained and degenerated regions were near land (C1, F1, G1, and H3), which were impacted by human activities; these may continue to experience a decline. The trend types were complex in D1, G2, and H2, but the areas of sustained significant change were small, suggesting that non-obvious changes may occur in these regions.
shows the pixel-based prediction results in different typical regions. (1) During the prediction period, the trend slopes of the time series of the pixels in B1 and C2 were 0.0036 and 0.0162, respectively. This suggests a possible decline in the mangrove population in these regions, whereas mangroves at pixels E2 and H1 would continue to improve. (2) The time series of pixel points in D1 varied to within 0.01 in magnitude during 2013–2021, and the trend slope was close to 0, indicating that the mangroves in the region are likely to remain stable. (3) The mangrove forest at the pixel in G1 showed a significant and sustainable decline trend from 2013 to 2021, indicating that it would continue to degrade or even die out.
5. Discussion
The selection of spectral indices and the reconstruction of their time series are important for detecting dynamic changes in mangroves. This study found that the NDVI was more accurate in detecting mangrove expansion, and the TCA followed. Some scholars have reached similar results (Zhu et al. Citation2019; Nguyen et al. Citation2020), but the TCA has certain noise in detecting changes in wetland dynamics (Fu et al. Citation2022). However, some researchers have demonstrated that the EVI and NDMI are more suitable for analyzing the dynamic changes in mangroves, such as Zhu, Liao, and Shen (Citation2021) and Aljahdali, Munawar, and Khan (Citation2021). Nevertheless, we found that the EVI was inadequate in capturing expansion events, and the NDMI time series did not show a pattern of vegetation growth (). In addition, different spectral composition methods yielded the optimal detection result for each spectral index. The reasons for this may be related to the tide, the outlier values of the spectral index, and cloud pixels. Although the manual selection of high-quality images can weaken the tidal influence (Zhu, Liao, and Shen Citation2021), it may not be practical for study areas with long temporal scales and large spatial scales. Spectral index composition methods have been proven to apply to the study of mangrove change detection (de Jong et al. Citation2021) but may cause outliers. Cloud bands are not always successful in cloud detection, which may affect the inter-annual and intra-annual detection results to some extent. Finally, the results for LandTrendr, BFAST, and BFAST Monitor consistently show that intra-annual time series reconstructed by data correction can be used for BFAST and BFAST Monitor algorithms and are robust. However, cloud-containing pixels and mangrove phenology changes may be factors in the minor changes detected by the BFAST Monitor algorithm.
Figure 15. Time series of the NDVI, EVI, and NDMI reconstructed based on maximum/mean/median spectral index composition methods and their results using the LandTrendr algorithm. P1, P2, and P3 are located in mangroves that expanded during different periods, and P4 is located in mudflats. A/B/C, maximum/mean/median spectral index composition method.

This study proposes a threshold segmentation method for the rapid extraction of mangroves differing from classification. Our method could extract the regions where had historically existed mangroves or have been existing mangroves, rather than mapping mangrove regions at a particular time point. Multi-temporal classifications can accurately map mangroves at multiple time points, but this may lose areas that disappear after mangrove growth during the interval period. Our method extracts mangrove using multi-source spectral indices, and does not require training samples. Compared with other methods, a threshold method of SMRI has been proposed to extract submerged mangroves (Xia et al. Citation2020; Yancho et al. Citation2020), but it cannot distinguish between terrestrial and mangrove vegetation. Yancho et al. (Citation2020) proposed a GEEMMM method with multiple spectral indices that are sensitive to mangroves for classification, but this method requires a lot of training samples. The differences between MGRs and classification product area may be caused by the spatial resolution of remote sensing images and misclassification. Besides, the increasing mangrove areas calculated by the classification products in 2015–2020 were much lower than those in 2000–2015 and our extraction for the same period, which indicated that there are still differences in mangrove regions between different scholars.
Mudflats and water depths influence the hierarchical changes in mangroves. Mudflats provide physical space for mangroves, but the relative height of mudflats to sea level influences the rate of their expansion (Swales et al. Citation2019; Lovelock et al. Citation2015, Citation2017). However, with global sea level rises, mangroves in Beibu Gulf still expand offshore, probably because: (1) these are less affected by sea level rise here; and (2) sediment deposition weakens the effect of sea level rise on the expansion (Long et al. Citation2022). A gradient change pattern has been demonstrated for mangroves in the Nanliu River Delta of Beihai; the expansion rate was highest in 2010–2020 (Long et al. Citation2022). Similarly, de Jong et al. (Citation2021) discovered the pattern that offshore mangroves expanded and the trend that near-land mangroves declined toward the ocean, but no naturally occurring degradation of them was detected in this study. Furthermore, future trends of mangroves may still maintain good growth as predicted by the DMP framework, but they may still be under threat. Since the mangrove forests in Beibu Gulf are close to zones such as fishponds and farmlands, their changes are influenced by natural conditions and human activities.
The DMP framework for mangrove expansion detection is more consistent with actual conditions than a single change detection algorithm. MKm could map the trend in mangrove spatial expansion, but could not obtain the exact mutation. The spectral trajectories segmented based on the LandTrendr algorithm may not be optimal due to large fluctuations in the time series or observed outliers (Zhu et al. Citation2019). The BFAST algorithm requires time series observations with equal time intervals. Despite these limitations, the results obtained by those algorithms could be compared and complemented each other to obtain detailed dynamics of the mangroves. In near-real-time monitoring results, SPH and the start of the monitoring period are usually defined manually based on knowledge of the study area (Schultz et al. Citation2018) or detected automatically by the algorithm. However, due to the need for more knowledge about the study area, some studies can only monitor changes based on a hypothetical SPH (Kanjir, Đurić, and Veljanovski Citation2018; Wanyama, Moore, and Dahlin Citation2020). Therefore, it is a rapid and feasible solution to provide knowledge of the study area for the BFAST Monitor algorithm using other algorithms. In future trend prediction results, MKs, Sen, and Hurst, although they have been used to determine the sustainability of the time series. However, some studies have chosen a predicted period of 20 years or longer (Tran et al. Citation2021; Guan et al. Citation2021; Tong et al. Citation2018). In this study, 2013–2021 was chosen as the predicted period based on the time series of mangroves with stable – growth–stable trends in because this period’s predicted result is more realistic than others. In , the prediction results are different in the three periods. The longer the forecast period, the stronger the sustainability of the time series. The reason is that the longer prediction period includes phases of mangrove expansion and stabilization, and the time series may be monotonically increasing (or decreasing).
6. Conclusions
Tracking spatio-temporal changes and assessing the current state are essential for mangrove conservation. Previous efforts have focused on monitoring the historical changes, and lack the tracking of real-time changes and predicting future trends in mangroves. Moreover, the problem of a change-detection algorithm does not provide enough evidence to evaluate the historical, current, and future changes in mangroves.
To address these issues, we proposed a novel DMP framework for detecting time-series historical changes, monitoring the near-real-time abrupt events, and predicting future trends in mangroves in Beibu Gulf, China, through the synergetic use of multiple detection change algorithms. Then, we further developed a threshold segmentation method for extracting mangroves using time-series spectral indices images and evaluated the performance of twenty-one spectral indices for capturing expansion events of mangroves. As a result, this study reveals the spatio-temporal variation patterns of mangroves in Beibu Gulf from 1986 to 2021.
Our method of mangrove extraction developed based on GEE platform could rapidly map the MGRs in the Beibu Gulf from 1986 to 2021. The overall accuracy reached 0.887. The NDVI and TCA accurately captured the year of mangrove expansion and delineated their dynamic patterns, although the MVI and MI are not suitable for monitoring time-series mangrove expansion. During 1986–2021, mangroves in Beibu Gulf exhibited a hierarchical change pattern from land to sea. The AE of mangroves is 239.822 ha/year and the UERi is 0.031 from 1988 to 2020. The DMP framework provides a comprehensive tool to track the spatio-temporal changes in mangroves, and effectively evaluates the change process and persistent status of mangroves in the past, present, and future. This study can provide guidance for the scientific monitoring and sustainable management of mangroves.
Author’s contributions
Bolin Fu: Conceptualization, Writing – review & editing, Funding acquisition, Supervision. Hang Yao: Conceptualization, Methodology, Software, Writing – original draft, Writing – review & editing. Feiwu Lan: Data curation. Sunzhe Li: Data curation. Yiyin Liang: Data curation. Hongchang He: Funding acquisition, Supervision, Data curation, Investigation. Donglin Fan: Data curation. Mingming Jia: Supervision. Yeqiao Wang: Supervision.
Acknowledgments
This study appreciated anonymous reviewers for their comments and suggestions which helped improve the quality of our manuscript.This study thanked National Earth System Science Data Center (http://www.geodata.cn) and Science Data Bank (https://www.scidb.cn) for providing mangrove product data.
Disclosure statement
No potential conflict of interest was reported by the authors.
Data availability statement
The mangrove products that support the findings of this study are openly available from National Earth System Science Data Center (http://www.geodata.cn) and Science Data Bank (https://www.scidb.cn).
Additional information
Funding
References
- Aljahdali, M. O., S. Munawar, and W. R. Khan. 2021. “Monitoring Mangrove Forest Degradation and Regeneration: Landsat Time Series Analysis of Moisture and Vegetation Indices at Rabigh Lagoon, Red Sea.” Forests 12 (1): 52. doi:10.3390/f12010052.
- Atwood, T. B., R. M. Connolly, H. Almahasheer, P. E. Carnell, C. M. Duarte, C. J. Ewers Lewis, X. Irigoien, et al. 2017. “Global Patterns in Mangrove Soil Carbon Stocks and Losses.” Nature Climate Change 7 (7): 523–24. doi:10.1038/nclimate3326.
- Awty-Carroll, K., P. Bunting, A. Hardy, and G. Bell. 2019. “Using Continuous Change Detection and Classification of Landsat Data to Investigate Long-Term Mangrove Dynamics in the Sundarbans Region.” Remote Sensing 11 (23): 2833. doi:10.3390/rs11232833.
- Baloloy, A. B., A. C. Blanco, R. R. C. S. Ana, and K. Nadaoka. 2020. “Development and Application of a New Mangrove Vegetation Index (MVI) for Rapid and Accurate Mangrove Mapping.” ISPRS Journal of Photogrammetry and Remote Sensing 166 (August): 95–117. doi:10.1016/j.isprsjprs.2020.06.001.
- Bianco, S., G. Ciocca, and R. Schettini. 2017. “How Far Can You Get by Combining Change Detection Algorithms?” In Image Analysis and Processing - ICIAP 2017, 96–107. Cham: Springer International Publishing. 10.1007/978-3-319-68560-1_9.
- Cohen, W., S. Healey, Z. Yang, S. Stehman, C. Brewer, E. Brooks, N. Gorelick, et al. 2017. “How Similar are Forest Disturbance Maps Derived from Different Landsat Time Series Algorithms?” Forests 8 (4): 98. doi:10.3390/f8040098.
- de Jong, S. M., Y. Shen, J. de Vries, G. Bijnaar, B. van Maanen, P. Augustinus, and P. Verweij. 2021. “Mapping Mangrove Dynamics and Colonization Patterns at the Suriname Coast Using Historic Satellite Data and the LandTrendr Algorithm.” International Journal of Applied Earth Observation and Geoinformation 97 (May): 102293. doi:10.1016/j.jag.2020.102293.
- Figueira Branco, E. R., A. R. dos Santos, J. E. M. Pezzopane, A. B. dos Santos, R. S. Alexandre, V. P. Bernardes, R. G. da Silva, K. B. de Souza, and M. Melo Moura. 2019. “Space-Time Analysis of Vegetation Trends and Drought Occurrence in Domain Area of Tropical Forest.” Journal of Environmental Management 246 (September): 384–396. doi:10.1016/j.jenvman.2019.05.097.
- Fu, B., F. Lan, S. Xie, M. Liu, H. He, Y. Li, L. Liu, et al. 2022. “Spatio-Temporal Coupling Coordination Analysis Between Marsh Vegetation and Hydrology Change from 1985 to 2019 Using LandTrendr Algorithm and Google Earth Engine.” Ecological Indicators 137 (April): 108763. doi:10.1016/j.ecolind.2022.108763.
- Fu, B., F. Lan, H. Yao, J. Qin, H. He, L. Liu, L. Huang, D. Fan, and E. Gao. 2022. “Spatio-Temporal Monitoring of Marsh Vegetation Phenology and Its Response to Hydro-Meteorological Factors Using CCDC Algorithm with Optical and SAR Images: In Case of Honghe National Nature Reserve, China.” The Science of the Total Environment 843 (October): 156990. doi:10.1016/j.scitotenv.2022.156990.
- Gan, H., J. Lin, K. Liang, and Z. Xia. 2013. “Selected Trace Metals (As, Cd and Hg) Distribution and Contamination in the Coastal Wetland Sediment of the Northern Beibu Gulf, South China Sea.” Marine Pollution Bulletin 66 (1–2): 252–258. doi:10.1016/j.marpolbul.2012.09.020.
- Gorelick, N., M. Hancher, M. Dixon, S. Ilyushchenko, D. Thau, and R. Moore. 2017. “Google Earth Engine: Planetary-Scale Geospatial Analysis for Everyone.” Remote Sensing of Environment 202 (December): 18–27. doi:10.1016/j.rse.2017.06.031.
- Guan, J., J. Yao, M. Li, and J. Zheng. 2021. “Assessing the Spatiotemporal Evolution of Anthropogenic Impacts on Remotely Sensed Vegetation Dynamics in Xinjiang, China.” Remote Sensing 13 (22): 4651. doi:10.3390/rs13224651.
- Halabisky, M., L. M. Moskal, A. Gillespie, and M. Hannam. 2016. “Reconstructing Semi-Arid Wetland Surface Water Dynamics Through Spectral Mixture Analysis of a Time Series of Landsat Satellite Images (1984–2011).” Remote Sensing of Environment 177 (May): 171–183. doi:10.1016/j.rse.2016.02.040.
- Hamed, K. H. 2008. “Trend Detection in Hydrologic Data: The Mann–Kendall Trend Test Under the Scaling Hypothesis.” Journal of Hydrology 349 (3–4): 350–363. doi:10.1016/j.jhydrol.2007.11.009.
- Jia, M., Z. Wang, D. Mao, C. Ren, C. Wang, and Y. Wang. 2021. “Rapid, Robust, and Automated Mapping of Tidal Flats in China Using Time Series Sentinel-2 Images and Google Earth Engine.” Remote Sensing of Environment 255 (March): 112285. doi:10.1016/j.rse.2021.112285.
- Jia, M., Z. Wang, Y. Zhang, D. Mao, and C. Wang. 2018. “Monitoring Loss and Recovery of Mangrove Forests During 42 Years: The Achievements of Mangrove Conservation in China.” International Journal of Applied Earth Observation and Geoinformation 73 (December): 535–545. doi:10.1016/j.jag.2018.07.025.
- Jiang, W., L. Yuan, W. Wang, R. Cao, Y. Zhang, and W. Shen. 2015. “Spatio-Temporal Analysis of Vegetation Variation in the Yellow River Basin.” Ecological Indicators 51 (April): 117–126. doi:10.1016/j.ecolind.2014.07.031.
- Kanjir, U., N. Đurić, and T. Veljanovski. 2018. “Sentinel-2 Based Temporal Detection of Agricultural Land Use Anomalies in Support of Common Agricultural Policy Monitoring.” ISPRS International Journal of Geo-Information 7 (10): 405. doi:10.3390/ijgi7100405.
- Kennedy, R. E., Z. Yang, and W. B. Cohen. 2010. “Detecting Trends in Forest Disturbance and Recovery Using Yearly Landsat Time Series: 1. LandTrendr — Temporal Segmentation Algorithms.” Remote Sensing of Environment 114 (12): 2897–2910. doi:10.1016/j.rse.2010.07.008.
- Li, R., L. Yu, M. Chai, H. Wu, and X. Zhu. 2020. “The Distribution, Characteristics and Ecological Risks of Microplastics in the Mangroves of Southern China.” The Science of the Total Environment 708 (March): 135025. doi:10.1016/j.scitotenv.2019.135025.
- Liang, D., J. Lu, X. Chen, C. Liu, and J. Lin. 2020. “An Investigation of the Hydrological Influence on the Distribution and Transition of Wetland Cover in a Complex Lake–Floodplain System Using Time-Series Remote Sensing and Hydrodynamic Simulation.” Journal of Hydrology 587 (August): 125038. doi:10.1016/j.jhydrol.2020.125038.
- Long, C., Z. Dai, R. Wang, Y. Lou, X. Zhou, S. Li, and Y. Nie. 2022. “Dynamic Changes in Mangroves of the Largest Delta in Northern Beibu Gulf, China: Reasons and Causes.” Forest Ecology and Management 504 (January): 119855. doi:10.1016/j.foreco.2021.119855.
- Lovelock, C. E., D. R. Cahoon, D. A. Friess, G. R. Guntenspergen, K. W. Krauss, R. Reef, K. Rogers, et al. 2015. “The Vulnerability of Indo-Pacific Mangrove Forests to Sea-Level Rise.” Nature 526 (7574): 559–563. doi:10.1038/nature15538.
- Lovelock, C. E., I. C. Feller, R. Reef, S. Hickey, and M. C. Ball. 2017. “Mangrove Dieback During Fluctuating Sea Levels.” Scientific Reports 7: 1. doi:10.1038/s41598-017-01927-6.
- Ma, C., B. Ai, J. Zhao, X. Xu, and W. Huang. 2019. “Change Detection of Mangrove Forests in Coastal Guangdong During the Past Three Decades Based on Remote Sensing Data.” Remote Sensing 11 (8): 921. doi:10.3390/rs11080921.
- Murdiyarso, D., J. Purbopuspito, J. B. Kauffman, M. W. Warren, S. D. Sasmito, D. C. Donato, S. Manuri, H. Krisnawati, S. Taberima, and S. Kurnianto. 2015. “The Potential of Indonesian Mangrove Forests for Global Climate Change Mitigation.” Nature Climate Change 5 (12): 1089–1092. doi:10.1038/nclimate2734.
- Navarro, A., M. Young, P. I. Macreadie, E. Nicholson, and D. Ierodiaconou. 2021. “Mangrove and Saltmarsh Distribution Mapping and Land Cover Change Assessment for South-Eastern Australia from 1991 to 2015.” Remote Sensing 13 (8): 1450. doi:10.3390/rs13081450.
- Nguyen, H.H., L. T. N. Tran, A. T. Le, N. H. Nghia, L. V. K. Duong, H. T. T. Nguyen, S. Bohm, and C. F. S. Premnath. 2020. “Monitoring Changes in Coastal Mangrove Extents Using Multi-Temporal Satellite Data in Selected Communes, Hai Phong City, Vietnam.” Forest and Society 4 (1): 256. doi:10.24259/fs.v4i1.8486.
- Otero, V., C. Martinez-Espinosa, F. Dahdouh-Guebas, R. Van De Kerchove, B. Satyanarayana, and R. Lucas. 2017. “Variations in Mangrove Regeneration Rates Under Different Management Plans: An Analysis of Landsat Time-Series in the Matang Mangrove Forest Reserve, Peninsular Malaysia.” In 2017 9th International Workshop on the Analysis of Multitemporal Remote Sensing Images (MultiTemp). IEEE. 10.1109/multi-temp.2017.8035238.
- Pirasteh, S., E. K. Zenner, D. Mafi-Gholami, A. Jaafari, A. N. Kamari, G. Liu, Q. Zhu, and J. Li. 2021. “Modeling Mangrove Responses to Multi-Decadal Climate Change and Anthropogenic Impacts Using a Long-Term Time Series of Satellite Imagery.” International Journal of Applied Earth Observation and Geoinformation 102 (October): 102390. doi:10.1016/j.jag.2021.102390.
- Schultz, M., A. Shapiro, J. Clevers, C. Beech, and M. Herold. 2018. “Forest Cover and Vegetation Degradation Detection in the Kavango Zambezi Transfrontier Conservation Area Using BFAST Monitor.” Remote Sensing 10 (11): 1850. doi:10.3390/rs10111850.
- Swales, A., G. Reeve, D. R. Cahoon, and C. E. Lovelock. 2019. “Landscape Evolution of a Fluvial Sediment-Rich Avicennia Marina Mangrove Forest: Insights from Seasonal and Inter-Annual Surface-Elevation Dynamics.” Ecosystems (New York, NY) 22 (6): 1232–1255. doi:10.1007/s10021-018-0330-5.
- Terfa, B. K., N. Chen, D. Liu, X. Zhang, and D. Niyogi. 2019. “Urban Expansion in Ethiopia from 1987 to 2017: Characteristics, Spatial Patterns, and Driving Forces.” Sustainability 11 (10): 2973. doi:10.3390/su11102973.
- Thomas, N., P. Bunting, R. Lucas, A. Hardy, A. Rosenqvist, and T. Fatoyinbo. 2018. “Mapping Mangrove Extent and Change: A Globally Applicable Approach.” Remote Sensing 10 (9): 1466. doi:10.3390/rs10091466.
- Tong, S., J. Zhang, Y. Bao, Q. Lai, X. Lian, N. Li, and Y. Bao. 2018. “Analyzing Vegetation Dynamic Trend on the Mongolian Plateau Based on the Hurst Exponent and Influencing Factors from 1982–2013.” Journal of Geographical Sciences 28 (5): 595–610. doi:10.1007/s11442-018-1493-x.
- Tran, T. V., D. X. Tran, H. Nguyen, P. Latorre‐carmona, and S. W. Myint. 2021. “Characterising Spatiotemporal Vegetation Variations Using LANDSAT Time‐series and Hurst Exponent Index in the Mekong River Delta.” Land Degradation & Development 32 (13): 3507–3523. doi:10.1002/ldr.3934.
- Verbesselt, J., R. Hyndman, G. Newnham, and D. Culvenor. 2010. “Detecting Trend and Seasonal Changes in Satellite Image Time Series.” Remote Sensing of Environment 114 (1): 106–115. doi:10.1016/j.rse.2009.08.014.
- Verbesselt, J., R. Hyndman, A. Zeileis, and D. Culvenor. 2010. “Phenological Change Detection While Accounting for Abrupt and Gradual Trends in Satellite Image Time Series.” Remote Sensing of Environment 114 (12): 2970–2980. doi:10.1016/j.rse.2010.08.003.
- Verbesselt, J., A. Zeileis, and M. Herold. 2012. “Near Real-Time Disturbance Detection Using Satellite Image Time Series.” Remote Sensing of Environment 123 (August): 98–108. doi:10.1016/j.rse.2012.02.022.
- Wang, H., B. Zhang, Y. Liu, Y. Liu, S. Xu, Y. Zhao, Y. Chen, and S. Hong. 2020. “Urban Expansion Patterns and Their Driving Forces Based on the Center of Gravity-GTWR Model: A Case Study of the Beijing-Tianjin-Hebei Urban Agglomeration.” Journal of Geographical Sciences 30 (2): 297–318. doi:10.1007/s11442-020-1729-4.
- Wanyama, D., N. J. Moore, and K. M. Dahlin. 2020. “Persistent Vegetation Greening and Browning Trends Related to Natural and Human Activities in the Mount Elgon Ecosystem.” Remote Sensing 12 (13): 2113. doi:10.3390/rs12132113.
- Weise, K., R. Höfer, J. Franke, A. Guelmami, W. Simonson, J. Muro, B. O’Connor, et al. 2020. “Wetland Extent Tools for SDG 6.6.1 Reporting from the Satellite-Based Wetland Observation Service (SWOS).” Remote Sensing of Environment 247 (September): 111892. doi:10.1016/j.rse.2020.111892.
- Woodcock, C. E., T. R. Loveland, M. Herold, and M. E. Bauer. 2020. “Transitioning from Change Detection to Monitoring with Remote Sensing: A Paradigm Shift.” Remote Sensing of Environment 238 (March): 111558. doi:10.1016/j.rse.2019.111558.
- Wu, J., Y. Cheng, Z. Mu, W. Dong, Y. Zheng, C. Chen, and Y. Wang. 2022. “Temporal Spatial Mutations of Soil Erosion in the Middle and Lower Reaches of the Lancang River Basin and Its Influencing Mechanisms.” Sustainability 14 (9): 5169. doi:10.3390/su14095169.
- Xia, Q., C.Z. Qin, H. Li, C. Huang, F.Z. Su, and M.M. Jia. 2020. “Evaluation of Submerged Mangrove Recognition Index Using Multi-Tidal Remote Sensing Data.” Ecological Indicators 113 (June): 106196. doi:10.1016/j.ecolind.2020.106196.
- Xia, Y., C. Fang, H. Lin, H. Li, and B. Wu. 2021. “Spatiotemporal Evolution of Wetland Eco-Hydrological Connectivity in the Poyang Lake Area Based on Long Time-Series Remote Sensing Images.” Remote Sensing 13 (23): 4812. doi:10.3390/rs13234812.
- Yancho, J., T. Jones, S. Gandhi, C. Ferster, A. Lin, and L. Glass. 2020. “The Google Earth Engine Mangrove Mapping Methodology (GEEMMM).” Remote Sensing 12 (22): 3758. doi:10.3390/rs12223758.
- Yang, G., K. Huang, W. Sun, X. Meng, D. Mao, and Y. Ge. 2022. “Enhanced Mangrove Vegetation Index Based on Hyperspectral Images for Mapping Mangrove.” ISPRS Journal of Photogrammetry and Remote Sensing 189 (July): 236–254. doi:10.1016/j.isprsjprs.2022.05.003.
- Yoo, C., J. Im, S. Park, and L. J. Quackenbush. 2018. “Estimation of Daily Maximum and Minimum Air Temperatures in Urban Landscapes Using MODIS Time Series Satellite Data.” ISPRS Journal of Photogrammetry and Remote Sensing 137 (March): 149–162. doi:10.1016/j.isprsjprs.2018.01.018.
- Younes Cárdenas, N., K. E. Joyce, and S. W. Maier. 2017. “Monitoring Mangrove Forests: Are We Taking Full Advantage of Technology?” International Journal of Applied Earth Observation and Geoinformation 63 (December): 1–14. doi:10.1016/j.jag.2017.07.004.
- Zhang, J., X. Yang, Z. Wang, T. Zhang, and X. Liu. 2021. “Remote Sensing Based Spatial-Temporal Monitoring of the Changes in Coastline Mangrove Forests in China Over the Last 40 Years.” Remote Sensing 13 (10): 1986. doi:10.3390/rs13101986.
- Zhao, C., and C.Z. Qin. 2020. “10-M-Resolution Mangrove Maps of China Derived from Multi-Source and Multi-Temporal Satellite Observations.” ISPRS Journal of Photogrammetry and Remote Sensing 169 (November): 389–405. doi:10.1016/j.isprsjprs.2020.10.001.
- Zhu, B., J. Liao, and G. Shen. 2021. “Combining Time Series and Land Cover Data for Analyzing Spatio-Temporal Changes in Mangrove Forests: A Case Study of Qinglangang Nature Reserve, Hainan, China.” Ecological Indicators 131 (November): 108135. doi:10.1016/j.ecolind.2021.108135.
- Zhu, L., X. Liu, L. Wu, Y. Tang, and Y. Meng. 2019. “Long-Term Monitoring of Cropland Change Near Dongting Lake, China, Using the LandTrendr Algorithm with Landsat Imagery.” Remote Sensing 11 (10): 1234. doi:10.3390/rs11101234.
- Zhu, Z. 2017. “Change Detection Using Landsat Time Series: A Review of Frequencies, Preprocessing, Algorithms, and Applications.” ISPRS Journal of Photogrammetry and Remote Sensing 130 (August): 370–384. doi:10.1016/j.isprsjprs.2017.06.013.
- Zhu, Z., and C. E. Woodcock. 2014. “Continuous Change Detection and Classification of Land Cover Using All Available Landsat Data.” Remote Sensing of Environment 144 (March): 152–171. doi:10.1016/j.rse.2014.01.011.
Appendix A.
Supplementary data
Table A1. Calculation formula for the spectral index in the study.
Table A2. Summary of the DMP framework and its monitoring change information.
Table A3. Parameter values for the LandTrendr algorithm applied in this study.
Table A4. The Overall accuracy extracting the potential mangrove of ten typical regions of Beibu Gulf in 1991,1996,2001,2006,2011,2016 and 2021.