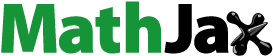
ABSTRACT
Forest aboveground biomass (AGB) estimation is crucial for carbon cycle studies and climate change mitigation actions. However, because of limitations in timely and reliable forestry surveys and high-resolution remote sensing data, producing a fine resolution and spatial continuous forest AGB map of China is challenging. Here, we combined 4789 ground-truth AGB measurements and multisource remote sensing data such as a recently released forest canopy-height product, optical spectral indexes, topographic data, climatological data, and soil properties to train a random forest regression model for forest AGB estimation of China at 30-m resolution. The accuracy of the estimated AGB can yield R2 = 0.67 and RMSE = 70.71 Mg/ha. The nationwide estimates show that the average forest AGB and total forest carbon storage were 97.57 ± 23.85 Mg/ha and 11.06 Pg C for the year 2019, respectively. The value of AGB uncertainty ranges from 0.68 Mg/ha to 37.80 Mg/ha, and the average AGB uncertainty was 4.32 ± 1.75 Mg/ha. The forest AGB estimates of China in this study correspond reasonably well with the AGB estimates derived from the forestry and grassland statistical yearbook at the provincial level (R2 = 0.61, RMSE = 30.15 Mg/ha). In addition, we found that previous AGB products generally underestimate the forest AGB compared with our estimated AGB at the pixel-level and ground-truth AGB measurements. The high-resolution forest AGB map provides an important alternative data source for forest carbon cycle studies and can be used as a baseline map for forest management and conservation practices.
1. Introduction
Forests are important carbon pools and maintain the carbon balance (Pan et al. Citation2011). Forest aboveground biomass (AGB) can be used to measure forest carbon stocks (Zheng et al. Citation2004). Accurately quantifying the spatial patterns and magnitude of forest AGB is essential to understanding carbon cycle processes from regional to global scales, managing biodiversity, and developing carbon offset and mitigation strategies (Piao et al. Citation2022; Fang et al. Citation2018).
Recent research also documented that China’s forest ecosystem was a carbon sink (Fang et al. Citation2014) and they are critical to the global carbon balance (Santoro et al. Citation2021; FAO Citation2020). China focuses on forest carbon stocks and plans to achieve the goal of “Carbon Neutrality” by 2060. China’s implementation of key ecological restoration and afforestation projects has nearly doubled its forest area from 1973 to 2018. Although China only had a 6.6% vegetated area in the world, it contributes 25% of the net growth in the leaf area, of which the increase in forests contributes 42% (Chen et al. Citation2019). These considerable changes in forest resources led to changes in the spatial patterns of forest AGB (Miaomiao et al. Citation2021). Therefore, developing a forest AGB benchmark with high spatial resolution is necessary for ongoing assessments.
Generally, large-scale forest AGB estimates mainly rely on traditional forest resource inventories and remote sensing inversions. Traditional forest resource inventories are often conducted on a per-wood survey of trees in the plot to calculate forest AGB by allometric equations (Luo et al. Citation2020). This method can obtain reliable forest AGB at local or regional scales. However, obtaining ground-truth measurements is expensive, labor-intensive, and time-consuming, and using ground-truth AGB to estimate spatial continuously forest AGB is impossible at large scales. A remote sensing-based approach can not only achieve efficiently large-scale estimation of forest AGB but also obtain forest AGB in areas where humans have not been able to reach them. Spatial mapping of forest AGB can be achieved by linking dispersed ground measurements with spatially continuous remote sensing datasets using regression models (Avitabile et al. Citation2016). However, the major obstacle to these studies is the lack of ground measurements to link remote sensing-derived metrics across the country (Huang et al. Citation2019). Moreover, passive optical, active microwave, and active light detection and ranging (lidar) has become an essential data source for deriving forest AGB. However, due to the limited penetration ability of optical or microwave electromagnetic signals through the forest canopy, the retrieved AGB from optical or microwave imagery usually suffers from the saturation effect that the received signal no longer increases if the AGB is greater than a specific value (Mitchard et al. Citation2012). Compared with traditional optical images and radar backscatter, lidar is not fraught with saturation effects in forests with complex structures and high AGB values (Baccini et al. Citation2008). However, it is difficult for both drone and airborne platforms to obtain large-scale lidar data (Guo et al. Citation2020. Although spaceborne lidar can provide nearly global coverage of land surface elevation data, its footprints are discretely distributed. Typically, large-scale forest AGB estimation uses the advantages of optical or microwave continuity to extrapolate the footprints parameter. Therefore, integrating multisource remote sensing data for achieving large-scale and spatial continuous forest AGB mapping is still a research bottleneck.
Many large-scale forest AGB products have been generated through fusing multisource remote-sensing data (Shen et al. Citation2018; Chi et al. Citation2017; Saatchi et al. Citation2011). A large proportion of these studies are global-scale forest AGB inversion studies, which do not only have a low spatial resolution but also use a limited number of samples in China (Santoro et al. Citation2021; Saatchi et al. Citation2011). Among them, some studies mapped spatial patterns of AGB specifically for Chinese forests (Chang et al. Citation2021; Huang et al. Citation2019; Su et al. Citation2016), but these studies either had a low spatial resolution or were based on data collected before 2010, which cannot reflect the current status of forest AGB in China. In addition, due to the different sources of ground survey data, remote sensing data, and forest mask data used in these studies, there are large differences among the existing forest AGB products (Zhang, Liang, and Yang Citation2019). For example, Huang et al. (Citation2019) and Su et al. (Citation2016) collected field AGB from literature, while Chang et al. (Citation2021) used in situ measurements data for inverting forest AGB. Moreover, Huang et al. (Citation2019) fused spaceborne lidar, microwave data, and optical images to estimate forest AGB, while Santoro et al. (Citation2021) retrieved forest AGB by integrating multiple observation data from microwave remote sensing. Furthermore, various land cover products were used at regional as well as global scales for different forest AGB estimations (Chen et al. Citation2015; Hansen et al. Citation2013; Congcong et al. Citation2014; Liu et al. Citation2014). Hence, a systematic comparative analysis of AGB products can not only point out the existing problems of previous products but also provide a baseline for future research on forest AGB inversion.
Considering the unique advantages of lidar data for inversing forest canopy height and forest AGB, a variety of studies commonly used spaceborne lidar to upscale the estimated forest AGB (Coops et al. Citation2021). However, the most commonly used lidar data in these studies were the Geoscience Laser Altimeter System (GLAS), which spanned from 2003 to 2009. Since the diameter of the footprint is large (~70 m) and the footprint density is low, the AGB maps derived from this dataset often have a coarse resolution of about 1 km. Since China’s forests are complex and diverse, and often consist of intact and non-intact stands, the AGB values in coarse resolution maps are often underestimated due to the spatial averaging of heterogeneous forest areas within a pixel (Liu et al. Citation2015). In addition, such a coarse-resolution map with limited spatial variability is insufficient to support national and regional studies and practices for forest management and conservation (Englund et al. Citation2017). Therefore, fine-resolution wall-to-wall forest AGB products are urgently needed. The recently launched spaceborne lidar, namely the Ice, Cloud and land Elevation Satellite (ICESat-2) mission, and the Global Ecosystem Dynamics Investigation (GEDI), have a higher footprint density and a more appropriate footprint size of about 30 m (Duncanson et al. Citation2022). Studies have documented that they can retrieve high-resolution canopy heights (Mulverhill et al. Citation2022; Liu et al. Citation2021) and further improve the accuracy of forest AGB inversion.
Based on the above background, we combined 4789 in situ measurements with the canopy height map from Liu et al. (Citation2022) and variables extracted from vegetation, topography, meteorology, and soil datasets to produce a high spatial resolution forest AGB product. We focused on answering the following three questions. (1) What is the current spatial pattern and magnitude of forest AGB in China? (2) Which variables contribute more to the AGB inversion model? (3) Compared with previous studies, whether our study can better reflect the current status of forest aboveground carbon stock in China? Our forest AGB map of China may provide an important alternative data source for forest carbon cycle studies and can be used as a baseline map for forest management and conservation practices.
2. Data and methods
2.1 Overview
We generated a new forest AGB product with a 30-m resolution for China based on random forest regression trained on a large in situ measurements dataset distributed evenly in seven vegetation zones (). We used 16 predictors covering 5 categories: vegetation data, topographic data, climatological data, soil data, and ancillary data. Among them, ancillary data include vegetation zones and GlobeLand30. The former was used as a categorical variable for forest AGB inversion and the latter was used to mask the estimated AGB. We comprehensively assessed the accuracy of the inversion forest AGB using three approaches. First, we used 30% of the in situ measurements to evaluate the estimates of AGB in our product. Second, we collected forest stock volume from the forestry and grassland statistical yearbook and converted them into forest AGB to verify the accuracy of our forest AGB product at the provincial level. Third, comparing with other AGB products in the spatial pattern at pixel-level and verifying other AGB products with in situ measurement.
2.2 Forestry survey
A total of 7517 in situ measurements were obtained nationwide, covering eight major vegetation zones in China (). Each measurement included geographical locations, measurement time, measurement methods, forest types, and forest AGB. These in situ measurements came from two field campaigns. Among them, 1986 plots were investigated between 2015 and 2019, spanning a latitude range from 21°N to 50°N and covering various forest types including conifer, broadleaf, and conifer-broadleaf mixed forest. These plots have decimeter-level positioning accuracy, and the area of these plots was greater than 400 m2. The AGB of the tree level in these plots (DBH>5 cm) was calculated using allometric equations from Zhou et al. (Citation2018) and Luo et al. (Citation2019). The other 5531 plots were collected between 2011 and 2015 following systematic sampling approaches and a consistent field investigation protocol. The area of these plots was 1000 m2, and covered forests with different types and different ages. The forest AGB of these plots was calculated using the allometric equations, which were constructed by locally employing harvesting methods (Tang et al. Citation2018). Considering the large time span of in situ measurements, we used two criteria to ensure data quality: (1) should have complete field records and (2) should still be forests in 2019, which was determined by Google Earth images for 2019 and the GlobeLand30 in 2020 (Chen et al. Citation2015). Finally, 4789 plots were retained, of which 1613 plots and 3176 plots were obtained in 2015–2019 and 2011–2015, respectively. We then divided the remaining 4789 plots into training samples and validation samples by vegetation zones at a ratio of 7:3 (). Considering that we needed enough samples from each vegetation zone and that the dominant vegetation types of Qinghai-Tibet Plateau alpine (QT) and temperate steppe (TS) were similar, we considered QT and TS as one vegetation zone in subsequent data processing and AGB analysis. The distribution of all plots in each vegetation zone shows that the sample size of training and testing samples used for model inversion varies greatly in different vegetation zones (). However, the sample size in each vegetation zone is consistent with the forest area of each vegetation zone and meets the statistical modeling requirements ( and Fig. S1).
Figure 2. The spatial distribution of validation samples and training samples in seven vegetation zones with a corresponding photo (All photos come from the Internet).

Table 1. Count of training and validation ground measurements by vegetation zone.
2.3 Variables required for biomass inversion
2.3.1 Vegetation data
Forest canopy height (FCH) is critical for large-scale retrieval of forest AGB. The canopy height product was collected from Liu et al. (Citation2022), which was developed by a neural network-guided interpolation method using Sentinel-2 images, ICESat-2 ATLAS, and GEDI. It has a 30-m spatial resolution across China for 2019. The accuracy of this canopy height product was verified by three independent datasets, and the root mean square error (RMSE) between the derived canopy heights and the reference height values is less than 5.32 m. In addition, as the tree height increases, there is almost no saturation effect on this canopy height product, confirming that the accuracy of this product is reliable and further ensuring the accuracy of the AGB estimation.
The normalized difference vegetation index (NDVI) is a typical index to reflect vegetation status, which has often been used to quantify vegetation growth, vegetation cover, and vegetation biomass. Sentinel-2 (A and B) is a satellite imaging mission with high revisit frequency, multi-spectral, and high-resolution, which provides excellent data for extracting vegetation indices at fine scale. To reduce the impact of the cloud on calculating NDVI, we used Google Earth Engine to filter Sentinel-2 Level-2A images (preprocessed by radiation correction and atmospheric correction) with cloud covers greater than 20% and used the maximum-value composite method to generate NDVI based on all imagery from 2019 (Maselli et al. Citation2020; Drusch et al. Citation2012). Then, an average interpolation was used to resample the NDVI image to 30-m resolution.
2.3.2 Topographic data
Topographic features are directly associated with light and water availability and soil condition, and hence are also essential to predict forest AGB (Silveira et al. Citation2019). The Shuttle Radar Topography Mission (SRTM) covers almost the entire Earth’s land surface and provides a 30-m resolution DEM. Its designed accuracies of horizontal and vertical are 20 m and 16 m, respectively. However, SRTM has a greater bias in forest areas. To reduce the impact of DEM on subsequent analyses, a corrected SRTM dataset was released by Zhao et al. (Citation2018). The bias and RMSE of the corrected SRTM were close to 0 and 1 m, respectively. In this study, we adopted the corrected SRTM DEM as the elevation variable to calculate the slope and aspect features for building the model in the forest area.
2.3.3 Climatological data
Climatological conditions, which determine the distribution of species and influence vegetation growth, have been demonstrated to be important variables for predicting forest AGB (Zhang, Liang, and Yang Citation2019). We used a monthly climate dataset, named as TerraClimate, which has a high resolution of about 4 km and is available from 1958 to 2021 (Abatzoglou et al. Citation2018). Based on this dataset, we calculated six climate surfaces: average temperature per year (Tmean), temperature seasonality (Tseason), average precipitation per year (Pmean), precipitation seasonality (Pseason), mean annual potential evapotranspiration (PET), and maximum annual vapor pressure deficit (VPD) (). Then, the climate surface datasets were resampled to 30-m resolution by bilinear interpolation. Among them, Tseason and Pseason were calculated using Equationequation (1)(1)
(1) , which was proposed in Citation2011:
Table 2. Statistics for 17 variables used in this study.
where and
are the standard deviation and average of temperature or precipitation per month, respectively.
2.3.4 Soil data
Soil properties can impact on the spatial pattern of forest AGB by affecting the growth of each tree and modulating forest ecosystem processes (Bennett et al. Citation2020; Paoli, Curran, and Slik Citation2008). It has been shown that soil properties can explain one-third or more of the variation in forest AGB (Laurance et al. Citation1999). In this study, we adopted three soil properties, including soil total nitrogen density (STN), soil organic carbon (SOC), and soil C: N mass ratios (C/N) in the upper layer (30 cm), which had a 1-km resolution and produced by Zhao et al. (Citation2019). In addition, a soil moisture (SM) with about 4-km resolution was also adopted from the TerraClimate dataset. We then resampled the soil properties as well, with the same interpolation method as the meteorological data.
2.3.5 Ancillary data
Vegetation zone and land cover were included as auxiliary datasets for AGB mapping. The vegetation zone was used as a categorical variable to represent the vegetation classification unit. Based on the dominant vegetation type and 1:1,000,000 vegetation map, eight vegetation zones were identified (Hou Citation2001). We then resampled the vegetation zone data to 30-m resolution using nearest neighbor interpolation. Due to QT and TS being merged into one zone, we split the sample plots into seven vegetation zones and constructed the forest AGB model.
The land cover from GlobeLand30 was developed by Chen et al. (Citation2015), which was classified using pixel and object-based approaches and prior knowledge of over 10,000 satellite images. Tests across different landscapes show that the overall classification accuracy of this map can achieve over 80%. In this study, we obtained the forest area of the latest GlobeLand30 (revised version 2020) to mask the estimated forest AGB.
2.4 Forest AGB estimation
Random forest is a commonly used machine learning model. The advantage of handling high-dimensional features and large samples makes its application in eco-geographic big data analysis increasingly widespread over the past 50 years (Belgiu and Drăguţ Citation2016). In this study, we construct an inversion model by implementing the R package randomForest between observed AGB and prediction variables, including three terrain features, six climate features, four soil properties, two vegetation features, and a vegetation zone (). The importance of the predictor variables was investigated by ranking them based on %IncMSE and IncNodePurity (Belgiu and Drăguţ Citation2016; Liaw and Wiener Citation2002). Greater values of %IncMSE and IncNodePurity indicate higher importance of a variable. The random forest was trained by 70% of in situ measurements, and its performance was tested by the remaining samples. The ntree represents the number of decision trees and mtry represents the variables adopted in the decision tree, both of them are important parameters of the random forest. In this study, we took a grid search method to find the optimal combination of mtry and ntree that makes the highest average accuracy of three replicate experiments. Among them, ntree varies from 100 to 1000 with an interval of 100, and mtry varies from 2 to 16 with an interval of 1. To improve the computation efficiency, the best prediction accuracy of the inversion model was used as the criterion, and finally ntree = 500 and mtry = 2 were adopted as the optimal parameters for the random forest model.
In addition, to provide reliable estimations of forest AGB and its uncertainty, we randomly sampled the in situ measurement (70% of all measurements) 30 times using a Monte Carlo simulation and constructed 30 random forest models using the optimal mtry and ntree parameters (Zhu et al. Citation2017). Then, a robust AGB map was produced by averaging the 30 AGB estimates, and its uncertainty was calculated by the standard deviation (SD) of the 30 AGB estimates.
2.5 Accuracy assessment
Model performances were assessed with the remaining 30% of the in situ measurements using the coefficient of determination (R2), RMSE, SD, and mean bias (Bias) shown in Equationequations (2)(2)
(2) -(Equation5
(5)
(5) ) (Silva et al. Citation2021; Koch and Link Citation2002), respectively:
where n represents the sample size, ,
,
represent the estimated AGB, observed AGB, and the average of observed AGB, respectively.
Forest survey data from the statistical yearbook for the year 2018 reported by the State Forestry and Grassland Administration were also adopted to verify the accuracy of biomass estimation. The statistical report includes the forest area and stock volume of each province, Guo et al. (Citation2013) documented the conversion method between forest AGB and stock volume density at the province level as Equationequation (6)(6)
(6) . Using this equation, we can convert the forest stock volume to forest AGB in each province, and then the total forest AGB can be calculated by multiplying the value by the area:
where and
are forest AGB (Mg/ha) and stock volume density (m3/ha), respectively.
2.6 Comparison with other forest AGB maps
To implement a more comprehensive assessment of the spatial patterns of predicted AGB values, we further compared our estimated forest AGB with previous forest AGB products at the pixel level. Since previous forest AGB products with the same time and spatial resolution as this study were hard to collect, we chose the AGB products that were closer in time and had the highest resolution (≥1 km) to compare our estimated AGB. Four typical forest AGB products were Huang et al. (Citation2019), Chang et al. (Citation2021), Saatchi et al. (Citation2011), and Santoro et al. (Citation2021) (). Among them, Huang et al. (Citation2019) estimated a 30-m resolution forest AGB in 2006 for all of China, using 1607 field AGB plots by fusing ICESat GLAS, PALSAR, and Landsat data. Chang et al. (Citation2021) used an optimal weighting technique by fusing five forest AGB maps to produce China’s 1-km resolution forest AGB from 2011 to 2015. Saatchi et al. (Citation2011) combined 4079 in situ measurements and ICESat GLAS to map the global forest AGB and underground biomass with a 1-km resolution. Currently, this map was updated to version 3 with a 100-m resolution for 2018. Santoro et al. (Citation2021) generated a 1-km resolution AGB map for 2010 at a global scale by a big dataset of 110,897 in situ measurements and combining multiple synthetic aperture radar observations. Considering the spatial resolution of three of the products was lower than our AGB map, we resampled all AGB products to 1-km resolution using average interpolation to ensure comparability between different products. As for the temporal mismatch, we used the accumulation of net primary production (NPP), an annual product with 500-m resolution, to characterize the amount of forest AGB accumulated by forest growth as Equationequation (7)(7)
(7) (Running and Zhao Citation2019). Using the average interpolation method, we resampled the NPP product to 1-km resolution. Then, AGB difference of our AGB map and other AGB maps was calculated at the pixel level. We also used in situ measurements to assist the difference analysis of our estimated AGB and other products, i.e. comparing AGB measurements with the corresponding pixels of these forest AGB products directly.
Table 3. Major characteristics of forest AGB maps for comparison.
where Map represents the AGB value from Huang map, Chang map, Saatchi Map, or Santoro Map and i is its production year.
3. Results
3.1 Distribution of field AGB in different vegetation zones
The histograms of observed forest AGB values of the seven vegetation zones in China exhibited large variations with AGB values from 11.14 to 750 Mg/ha; the observed AGB values of each zone generally followed a normal distribution (). The average observed AGB value was highest in TMFRF (250.7 Mg/ha), followed by TNBMF (213.3 Mg/ha) and TD (154.2 Mg/ha); the lowest AGB value was in WTDBF (64.3 Mg/ha). However, the standard deviation of observed AGB was lowest for WTDBF (34.7 Mg/ha) and highest for TMFRF (177.6 Mg/ha). The wide distribution and diversity of field AGB indicated that the collected ground measurements were representative and could be used to reliably train the random forest model for a high-resolution forest AGB mapping.
3.2 Forest AGB patterns and regional overview
A 30-m resolution and spatial continuously forest AGB product of China was shown in . The high values of forest AGB are principally distributed in the southwest, northeast, and northwest of China. The value of forest AGB was from 34.61 Mg/ha to 335.44 Mg/ha. The average of forest AGB was 97.57 ± 23.85 Mg/ha. In addition, the total forest carbon storage was 11.06 Pg C, which was calculated by a commonly used conversion coefficient between AGB and carbon storage (Piao et al. Citation2005). The pixel-level statistics of the nationwide forest AGB show that the estimated AGB values are principally distributed in 75–100 Mg/ha and 100–150 Mg/ha (), and the corresponding forest area accounts for about 70% of the total forest area in China.
Figure 4. (a) Spatial pattern of forest AGB of China. (b) the percentage of forest AGB at different levels.

By quantitatively analyzing the uncertainty introduced by sampling differences of in situ measurements, we found that the value of AGB uncertainty was 0.68–7.80 Mg/ha. The average AGB uncertainty were 4.32 ± 1.75 Mg/ha. In addition, forest AGB uncertainty is basically distributed within 0–10 Mg/ha, and the regions with greater uncertainty are principally distributed in the southwest of China and the Populus euphratica forest in the Tarim River Basin in Xinjiang ().
Figure 5. The spatial pattern of AGB uncertainty of China. AGB uncertainty is the standard deviation of the 30 AGB estimates.

The statistics of estimated AGB by province and vegetation zones in the form of boxplots and violin plots further quantify the spatial patterns and magnitudes of China’s forest AGB (). TD (119.95 Mg/ha) and TMFRF (118.06 Mg/ha) have a higher forest AGB than other vegetation zones, followed by TNBMF (102.49 Mg/ha) ( and Table S2). In addition, WTDBF has relatively lower forest AGB than other vegetation zones. Among all provinces, the results showed that forest AGB in Tibet and Yunnan were relatively high with a value of AGB was 128.61 Mg/ha and 124.78 Mg/ha, respectively, followed by Sichuan (123.80 Mg/ha), Taiwan (123.61 Mg/ha), and Xinjiang (123.34 Mg/ha) ( and Table S3). Furthermore, eight provinces have an average forest AGB greater than 100 Mg/ha.
Figure 6. The statistics of AGB estimates by (a) administrative divisions (i.e. provinces, autonomous regions, or special administrative districts) and (b) vegetation zones in China.

By sorting the total AGB of all provinces, the top three provinces are Yunnan, Sichuan, and Heilongjiang, with a total AGB of 2.72 Pg, 2.57 Pg, and 1.84 Pg, respectively (Table S1). This can be partly attributed to their large forest areas, which are also the top three in China.
3.3 Accuracy assessment
In this study, the prediction model yielded an accuracy of R2 = 0.67 and RMSE = 70.71 Mg/ha by using 30% of the in situ measurements for model validation (). Then, the mean bias (Bias) and SD were −2.08 Mg/ha and 122.91 Mg/ha, respectively. In addition, by ranking %IncMSE and IncNodePurity, we found that FCH and Elevation and soil variables (C/N, SOC) contributed significantly to the prediction model ().
Figure 7. (a) Predicted AGB versus observed AGB; (b) Rank the importance of variables by %IncMSE and IncNodePurity. Note that R2, RMSE, Bias, SD represent the coefficient of determination, root mean square error, mean bias, and standard deviation. FCH, NDVI, C/N, SOC, SM, STN, Pmean, Pseason, PET, Tmean, Tseason, and VPD represent forest canopy height, normalized difference vegetation index, soil C: N mass ratios, soil organic carbon, soil moisture, soil total nitrogen density, average precipitation per year, precipitation seasonality, mean annual potential evapotranspiration, average temperature per year (Tmean), temperature seasonality (Tseason), and maximum annual vapor pressure deficit.

The estimated AGB in this study correlated well with the AGB statistics at the provincial level in 2018 (). Although the estimated average forest AGB values of three provinces (Qinghai, Xinjiang, and Ningxia) were divergent from the corresponding values in the statistical yearbook (R2 = 0.35, RMSE = 36.75 Mg/ha; , our estimated AGB was generally consistent with the statistical AGB in 28 provinces of mainland China. After removing the average AGB values of these three provinces, the estimated AGB and statistical AGB matched well in the remaining 28 provinces, and the R2 increased to 0.61, while the RMSE decreased to 30.15 Mg/ha (). In terms of total AGB, there was a slight difference in all provinces, with an average difference of about 0.25 Pg and the largest difference in Yunnan of about 0.91 Pg (Table S1). However, in comparison, the estimated total AGB with the statistical total AGB, the R2 and RMSE of them were 0.79 and 0.35 Pg, respectively ().
3.4 Comparison with other AGB map
For each pixel, the AGB differences between our AGB estimates and the AGB estimates from the Huang map, Saatchi map, Chang map, and Santoro map were calculated and compared (). The average AGB differences (μ) were all greater than 0 ()), indicating that previous AGB products underestimated the forest AGB and were unable to reflect the current status of forest AGB in China. The AGB differences from were the lowest with μ = 17.80 Mg/ha and σ = 15.05 Mg/ha, followed by the AGB values from the Santoro map. In addition, the AGB values of the Saatchi map differed greatly from our estimated AGB with the average of the AGB difference was 63.52 ± 31.24 Mg/ha, respectively.
Figure 9. Forest AGB differences between our map and other maps for the year 2019. μ: average of the AGB difference; σ: standard deviation of the AGB difference.

The histogram of AGB difference between our estimated AGB and other AGB maps was mainly between −50 and 150 Mg/ha but the spatial pattern of them were not consistent (). The high values of AGB differences between our estimated AGB and the Huang map and Santoro map were located in southwest China, while the regions with greater AGB differences from the Saatchi map were mainly distributed in southern China, including the vegetation zones of SEBF and TMFRF. The AGB differences between our map and the maps of Chang ( (c)) were relatively uniform and exhibited no outstanding spatial patterns. However, our AGB estimates were slightly lower than the AGB estimates of Chang map in southwestern of China. Then, we randomly selected three of the regions with large differences and represent different forest types in . The details of the enlarged view show that differences in the forest definition of different products may be the cause of discrepancies in the total of forest AGB ().
We selected and evaluated the four AGB products using in situ measurements (). The AGB of the Chang map correlated well with the observed AGB, with R2 of 0.28 and RMSE of 65.05 Mg/ha, followed by forest AGB estimates of the Santoro and Saatchi maps. In addition, by spatially overlaying in situ measurements onto Chang map, we found inconsistencies in the land cover between the Chang map and our AGB. Only 2085 samples out of our 4789 in situ measurements overlapped with the forest pixels in the Chang map, which may be one of the reasons why the AGB estimates of the Chang map had a poor correlation with observed AGB values (). Overall, the four AGB maps tend to underestimate forest AGB with the AGB values mostly below the 1:1 line and less than 150 Mg/ha ()).
4. Discussion
A large-scale and spatial continuous forest AGB map is essential for quantifying forest carbon stocks on a global scale. However, because of the lack of large scale and consistent ground measurements and high-resolution remote sensing data, there are almost no maps at a 30-m resolution that reflect the current state of forest AGB in China (Huang et al. Citation2019; Chang et al. Citation2021). To achieve the target of large-scale and high-resolution forest AGB mapping, 4789 in situ measurements from 2011 to 2019, and a 30-m resolution canopy height map were collected. By combining vegetation index, topographic features, climate surfaces, and soil properties and using a classic random forest model, the predicted model in this study explained 67% of the observed variance and RMSE was only 70.71 Mg/ha. In addition, the analysis of AGB uncertainty shows that the average AGB uncertainty is about 4.32 Mg/ha, which is about 4% of the average AGB. This demonstrates that the retrieved forest AGB in this study is reliable and robust.
The number and representativeness of training samples are one of the most important factors influencing forest AGB inversion accuracy (Ploton et al. Citation2020). Evenly distributed ground samples in each vegetation zone were considered to be beneficial to improve forest AGB inversion accuracy (Chen et al. Citation2022). In this study, although there was a disparity issue in the number of ground samples within different vegetation zones (), the ratio of the number of ground samples to area was close in each vegetation zone (Figure S1 (a) and (b)). To further examine the potential influence of the disparity issue, we further conducted a training sample balancing analysis. The vegetation zone with the smallest ratio of the number of ground samples to area was used as the reference, and the training samples in all other vegetation zones were randomly stratified based on its area so that the ratio of sample size of training samples to area in each vegetation zone was the same. Then, the same random forest-based forest AGB inversion process as described above was used to generate forest AGB product of China. The accuracy of estimated forest AGB using the stratified ground samples was slightly lower to that using all ground samples (Fig. S2 (a) and (b)), which further demonstrated the representativeness of the collected ground samples in this study.
To evaluate the accuracy of our estimated AGB, the statistical AGB at the province level was collected from the statistical yearbook to validate our estimated AGB. The results showed that our estimated AGB strongly correlates with the statistical AGB and the RMSE was less than 30.15 Mg/ha. However, the estimated AGB in this study was significantly higher than the statistical AGB in the three provinces of Qinghai, Xinjiang, and Ningxia (Table S1). There were no in situ measurements in Qinghai and Ningxia provinces. To explain this overestimation, we calculated the average in situ measurement for Xinjiang Province (157.26 Mg/ha), which was closer to our estimated AGB (123.10 Mg/ha) than the statistical AGB (54.37 Mg/ha), showing that our estimated AGB was reliable. In addition, the total AGB in this study correlated well with the statistical AGB at the province level and R2 was greater than 0.7, which once again demonstrated the reliability of our estimated AGB. Disparities in the total AGB may be caused by two reasons: differences in forest area and differences in the conversion from forest stock volume to AGB (Guo et al. Citation2013). It was demonstrated that differences in forest area usually result from inconsistent forest definitions across studies (Sexton et al. Citation2016) and can lead to a difference of up to 10.7% in the total forest area, which indirectly affects the total AGB (Li et al. Citation2017).
To further assess the performance of AGB estimation, the comparison of our AGB map and the other AGB maps at the pixel level and with in situ measurements were carried out. At the pixel level, the average AGB differences were all greater than 0, which means that previous studies generally underestimated the forest AGB ()). This is mainly due to the fact that the observed AGB was obtained after 2010 and the multisource remote sensing data were also collected around 2019 (). To some degree, our estimated AGB better reflects the current status of forest AGB in China (). The AGB differences between our estimated AGB and the Chang map were the lowest, with μ = 17.80 Mg/ha and σ = 15.05 Mg/ha (), this can be attributed to the fact that Chang map also used a large amount of ground-truth AGB and the acquisition time of these data was much closer to our in situ measurements (both after 2010). However, the R2 between these two maps was 0.28 (). This may be caused by the difference in forest definitions (Li et al. Citation2017). Since the forest regions used in our AGB and Chang map are from Chen et al. (Citation2015) and Liu et al. (Citation2014), respectively, only 2085 samples out of all our in situ samples fell within the forest regions of Chang’s map. The spatial details of the forest AGB map in can also support this point well. The forest AGB of different products has large differences, and the forests areas of different products are also different (). In addition, compared to the observed AGB, the AGB values of other maps were mostly below the 1:1 line and seriously underestimated, which also confirmed that the existing forest AGB could not reflect the current situation of forest AGB (). Concerning China’s carbon neutrality plan by 2060, this study will hopefully provide a base map to reflect the magnitude and spatial pattern of the current status of China’s carbon stocks.
By ranking the importance of variables in random forests, we found that forest canopy height is the best variable for the prediction of forest AGB (). There are three explanations for this result. Firstly, the AGB of tree level was calculated using tree height and DBH as independent variables (de Almeida et al. Citation2019). Secondly, canopy height product was obtained using the newly launched spaceborne lidars (ICESat2 ATLAS and GEDI) and adopting a deep learning-guided spatial interpolation model (Liu et al. Citation2022). Benefiting from small and dense lidar footprints and the interpolation-based derived method, the canopy height map was hardly affected by the saturation effect in canopy-crowded and multi-layered forest areas and the RMSE of canopy height was lower than 5.32 m. Third, canopy height product had a 30-m resolution, which was more suitable for the sample size of the in situ measurement. Moreover, topographic (Elevation) and soil variables (C/N and SOC) also contribute significantly to the prediction model, which was supported by the results of Laurance et al. (Citation1999).
However, there are also some limitations in this study. First, the in situ measurements covered a large time span, but we did not consider the effect of forest growth on the AGB mapping. Additionally, the spatial mismatch between in situ measurements (0.04 ha for 34% of samples and 0.1 ha for 66% of samples) and multisource remote sensing data (~0.09 ha) adds uncertainty to the forest AGB estimation. Moreover, a more systematic assessment of the various uncertainties that may exist in the biomass mapping process is required. In the future, it will be necessary to collect more ground measurements in a standardized and consistent manner, combine the advantages of multisource data, and develop robust and efficient AGB estimation methods to achieve high-precision and high-resolution wall-to-wall forest AGB estimates. Moreover, remote sensing technology has undergone rapid development, and a large amount of remote sensing data has been accumulated, which has laid the foundation for forest AGB estimation in time series. In particular, spaceborne lidars (GEDI and ICESat-2) and the upcoming spaceborne NISAR and BIOMASS missions is expected to revolutionize the current AGB estimation approaches at large scale.
5. Conclusion
In this study, we generated a 30-m resolution and wall-to-wall forest AGB of China through the combination of multisource remote sensing data. In total 4789 ground-truth AGB measurements were collected from 2011 to 2019. Moreover, to minimize the influence of the saturation effect of optical imagery on the estimation accuracy, the latest canopy height product with 30-m resolution and high precision was collected for model building. The average value and standard deviation of China’s forest AGB were 97.57 Mg/ha and 23.85 Mg/ha, respectively. The total forest carbon storage was 11.06 Pg C. The AGB estimates were generally high in the southwest, northeast, and northwest of China. The average of AGB uncertainty was 4.32 ± 1.75 Mg/ha. In addition, Yunnan, Sichuan, and Heilongjiang ranked as the top three provinces with a total AGB of 2.72 Pg, 2.57 Pg, and 1.84 Pg, respectively. Our estimated forest AGB correlated well with the forest AGB converted from the forestry and grassland statistical yearbook (R2 = 0.61, RMSE = 30.15 Mg/ha). Moreover, we found that forest canopy height, elevation, and soil C/N are the critical variables for the AGB prediction model. By comparison with our AGB map as well as in situ measurements, we also found that previous studies underestimated forest AGB and do not reflect the current state of forest carbon stock of China. We estimated AGB can be used as a base map for forest management and conservation and carbon cycle research.
Supplemental Material
Download (254.7 KB)Acknowledgments
<change class=“ins iAUser-1 CurrentChange” data-cid=“24” data-userid=“1” data-username=“Author” data-time=“1681887275000” id=“5y7i068xavs3421” aid=“271xyi830v46as5” user_name=“Author” title=“Inserted by Author - 04/19/2023 2:54 pm”> </change>
This study appreciated anonymous reviewers for their comments and suggestions, which helped improve the quality of our manuscript.
Disclosure statement
The authors declare that they have no known competing financial interests or personal relationships that could have appeared to influence the work reported in this paper.
Data availability statement
The generated high-resolution forest AGB map will be made available through the web (www.3decology.org) upon the decision of acceptance for publication.
Supplementary material
Supplemental data for this article can be accessed online at https://doi.org/10.1080/15481603.2023.2203303.
Additional information
Funding
References
- Abatzoglou, J. T., S. Z. Dobrowski, S. A. Parks, and K. C. Hegewisch. 2018. “TerraClimate, a High-Resolution Global Dataset of Monthly Climate and Climatic Water Balance from 1958–2015.” Scientific Data 5 (1): 1–19. doi:10.1038/sdata.2017.191.
- Avitabile, V., M. Herold, G. B. Heuvelink, S. L. Lewis, O. L. Phillips, G. P. Asner, J. Armston, et al. 2016. “An Integrated Pan-Tropical Biomass Map Using Multiple Reference Datasets.” Global Change Biology 22 (4): 1406–1420. doi:10.1111/gcb.13139.
- Baccini, A., N. Laporte, S. J. Goetz, M. Sun, and H. Dong. 2008. “A First Map of Tropical Africa’s Above-Ground Biomass Derived from Satellite Imagery.” Environmental Research Letters 3 (4): 045011. doi:10.1088/1748-9326/3/4/045011.
- Belgiu, M., and L. Drăguţ. 2016. “Random Forest in Remote Sensing: A Review of Applications and Future Directions.” ISPRS Journal of Photogrammetry and Remote Sensing 114: 24–31. doi:10.1016/j.isprsjprs.2016.01.011.
- Bennett, A. C., T. D. Penman, S. K. Arndt, S. H. Roxburgh, and L. T. Bennett. 2020. “Climate More Important Than Soils for Predicting Forest Biomass at the Continental Scale.” Ecography 43 (11): 1692–1705. doi:10.1111/ecog.05180.
- Chang, Z., S. Hobeichi, Y.P. Wang, X. Tang, G. Abramowitz, Y. Chen, N. Cao, et al. 2021. “New Forest Aboveground Biomass Maps of China Integrating Multiple Datasets.” Remote Sensing 13 (15): 2892. doi:10.3390/rs13152892.
- Chen, J., J. Chen, A. Liao, X. Cao, L. Chen, X. Chen, H. Chaoying, et al. 2015. “Global Land Cover Mapping at 30 M Resolution: A POK-Based Operational Approach.” ISPRS Journal of Photogrammetry and Remote Sensing 103: 7–27. doi:10.1016/j.isprsjprs.2014.09.002.
- Chen, C., Y. Ma, G. Ren, and J. Wang. 2022. “Aboveground Biomass of Salt-Marsh Vegetation in Coastal Wetlands: Sample Expansion of in situ Hyperspectral and Sentinel-2 Data Using a Generative Adversarial Network.” Remote Sensing of Environment 270: 112885. doi:10.1016/j.rse.2021.112885.
- Chen, C., T. Park, X. Wang, S. Piao, B. Xu, R. K. Chaturvedi, R. Fuchs, et al. 2019. “China and India Lead in Greening of the World Through Land-Use Management.” Nature Sustainability 2: 122–129. doi:10.1038/s41893-019-0220-7.
- Chi, H., G. Sun, J. Huang, L. Rendong, X. Ren, N. Wenjian, and F. Anmin. 2017. “Estimation of Forest Aboveground Biomass in Changbai Mountain Region Using ICESat/GLAS and Landsat/TM Data.” Remote Sensing 9 (7): 707. doi:10.3390/rs9070707.
- Congcong, L., J. Wang, H. Luanyun, L. Yu, N. Clinton, H. Huang, J. Yang, and P. Gong. 2014. “A Circa 2010 Thirty Meter Resolution Forest Map for China.” Remote Sensing 6 (6): 5325–5343. doi:10.3390/rs6065325.
- Coops, N. C., P. Tompalski, T. R. H. Goodbody, M. Queinnec, J. E. Luther, D. K. Bolton, J. C. White, M. A. Wulder, O. R. van Lier, and T. Hermosilla. 2021. “Modelling Lidar-Derived Estimates of Forest Attributes Over Space and Time: A Review of Approaches and Future Trends.” Remote Sensing of Environment 260: 112477. doi:10.1016/j.rse.2021.112477.
- de Almeida, C. T., L. S. Galvão, L. E. de Oliveira Cruz e Aragão, J. P. H. B. Ometto, A. D. Jacon, F. R. de Souza Pereira, L. Y. Sato, et al. 2019. “Combining LiDar and Hyperspectral Data for Aboveground Biomass Modeling in the Brazilian Amazon Using Different Regression Algorithms.” Remote Sensing of Environment 232: 111323. doi:10.1016/j.rse.2019.111323.
- Drusch, M., U. Del Bello, S. Carlier, O. Colin, V. Fernandez, F. Gascon, B. Hoersch, et al. 2012. “Sentinel-2: Esa’s Optical High-Resolution Mission for GMES Operational Services.” Remote Sensing of Environment 120: 25–36. doi:10.1016/j.rse.2011.11.026.
- Duncanson, L., J. R. Kellner, J. Armston, R. Dubayah, D. M. Minor, S. S. Hancock P. Healey, P. L. Patterson, S. Saarela and S. Marselis, S. Healey. 2022. ”Aboveground Biomass Density Models for Nasa’s Global Ecosystem Dynamics Investigation (GEDI) Lidar Mission.” Remote Sensing of Environment 270: 112845. doi:10.6067/XCV85B05BG.
- Englund, O., G. Sparovek, G. Berndes, F. Freitas, J. P. Ometto, P. V. D. C. E. Oliveira, C. Costa Jr, and D. Lapola. 2017. “A New High‐resolution Nationwide Aboveground Carbon Map for Brazil.” Geo: Geography and Environment 4 (2): e00045. doi:10.1002/geo2.45.
- Fang, J., Z. Guo, H. Hu, T. Kato, H. Muraoka, and Y. Son. 2014. “Forest Biomass Carbon Sinks in East Asia, with Special Reference to the Relative Contributions of Forest Expansion and Forest Growth.” Global Change Biology 20 (6): 2019–2030. doi:10.1111/gcb.12512.
- Fang, J., G. Yu, L. Liu, S. Hu, and F.S. Chapin III. 2018. “Climate Change, Human Impacts, and Carbon Sequestration in China”. Proceedings of the National Academy of Sciences 115(16). 4015-20 %@ 0027-8424. doi:10.1073/pnas.1700304115.
- FAO. 2020. “Global Forest Resources Assessment 2020: Main Report. Rome
- Guo, Z., H. Hu, P. Li, N. Li, and J. Fang. 2013. “Spatio-Temporal Changes in Biomass Carbon Sinks in China’s Forests from 1977 to 2008.” Science China: Life Sciences 56 (7): 661–671. doi:10.1007/s11427-013-4492-2.
- Guo, Q., S. Yanjun, H. Tianyu, H. Guan, S. Jin, J. Zhang, X. Zhao, X. Kexin, D. Wei, and M. Kelly. 2020. “Lidar Boosts 3D Ecological Observations and Modelings: A Review and Perspective.” IEEE Geoscience and Remote Sensing Magazine. doi: 10.1109/MGRS.2020.3032713.
- Hansen, M. C., P. V. Potapov, R. Moore, M. Hancher, S. A. Turubanova, A. Tyukavina, D. Thau, S. V. Stehman, S. J. Goetz, and T. R. Loveland. 2013. “High-Resolution Global Maps of 21st-Century Forest Cover Change.” Science 342 (6160): 850–853. doi:10.1126/science.1244693.
- Hou, X. 2001. “Vegetation Atlas of China.” Chinese Academy of Science, the Editorial Board of Vegetation Map of China 113–124.
- Huang, H., C. Liu, X. Wang, X. Zhou, and P. Gong. 2019. “Integration of Multi-Resource Remotely Sensed Data and Allometric Models for Forest Aboveground Biomass Estimation in China.” Remote Sensing of Environment 221: 225–234. doi:10.1016/j.rse.2018.11.017.
- Koch, G. S., and R. F. Link. 2002. Statistical Analysis of Geological Data. Massachusetts, U.S.A: Courier Corporation.
- Laurance, W. F., P. M. Fearnside, S. G. Laurance, P. Delamonica, T. E. Lovejoy, J. M. Rankin-de Merona, J. Q. Chambers, and C. Gascon. 1999. “Relationship Between Soils and Amazon Forest Biomass: A Landscape-Scale Study.” Forest Ecology and Management 118 (1–3): 127–138. doi:10.1016/S0378-1127(98)00494-0.
- Liaw, A., and M. Wiener. 2002. “Classification and Regression by randomForest.” R News.” 2 (3): 18–22.
- Li, Y., Sulla-Menashe, D., Motesharrei, S., Song, X.P., Kalnay, E., Ying, Q., Li, S. and Ma, Z. 2017. “Inconsistent estimates of forest cover change in China between 2000 and 2013 from multiple datasets: Differences in parameters, spatial resolution, and definitions.” Scientific reports 7 (1): 1–12. doi:10.1038/s41598-017-07732-5.
- Liu, A., X. Cheng, and Z. Chen. 2021. “Performance Evaluation of GEDI and ICESat-2 Laser Altimeter Data for Terrain and Canopy Height Retrievals.” Remote Sensing of Environment 264. doi:10.1016/j.rse.2021.112571.
- Liu, J., W. Kuang, Z. Zhang, X. Xinliang, Y. Qin, J. Ning, W. Zhou, S. Zhang, L. Rendong, and C. Yan. 2014. “Spatiotemporal Characteristics, Patterns, and Causes of Land-Use Changes in China Since the Late 1980s.” Journal of Geographical Sciences 24 (2): 195–210. doi:10.1007/s11442-014-1082-6.
- Liu, Y. Y., A. I. Van Dijk, R. A. De Jeu, J. G. Canadell, M. F. McCabe, J. P. Evans, and G. Wang. 2015. “Recent Reversal in Loss of Global Terrestrial Biomass.” Nature Climate Change 5 (5): 470–474. doi:10.1038/nclimate2581.
- Liu, X., S. Yanjun, H. Tianyu, Q. Yang, B. Liu, Y. Deng, H. Tang, Z. Tang, J. Fang, and Q. Guo. 2022. “Neural Network Guided Interpolation for Mapping Canopy Height of China’s Forests by Integrating GEDI and ICESat-2 Data.” Remote Sensing of Environment 269: 112844. doi:10.1016/j.rse.2021.112844.
- Luo, Y., X. Wang, Z. Ouyang, L. Fei, L. Feng, and J. Tao. 2019. “ChinAllometree 1.0: China’s Normalized Tree Biomass Equation Dataset.” Earth System Science Data Discussions 1–75. doi:10.5194/essd-2019-1.
- Luo, Y., X. Wang, Z. Ouyang, L. Fei, L. Feng, and J. Tao. 2020. “A Review of Biomass Equations for China’s Tree Species.” Earth System Science Data 12 (1): 21–40. doi:10.5194/essd-12-21-2020.
- Maselli, F., P. Battista, M. Chiesi, B. Rapi, L. Angeli, L. Fibbi, R. Magno, and B. Gozzini. 2020. “Use of Sentinel-2 MSI Data to Monitor Crop Irrigation in Mediterranean Areas.” International Journal of Applied Earth Observation and Geoinformation 93: 102216. doi:10.1016/j.jag.2020.102216.
- Miaomiao, Z., J. Yang, N. Zhao, L. Liu, D. Ling, X. Xiao, T. Yue, and J. P. Wilson. 2021. “Spatially Explicit Changes in Forest Biomass Carbon of China Over the Past 4 Decades: Coupling Long-Term Inventory and Remote Sensing Data.” Journal of Cleaner Production 316: 128274. doi:10.1016/j.jclepro.2021.128274.
- Mitchard, E. T., S. S. Saatchi, L. J. White, K. Anne Abernethy, K. Jane Jeffery, S. L. Lewis, M. Collins, et al. 2012. “Mapping Tropical Forest Biomass with Radar and Spaceborne LiDar in Lopé National Park, Gabon: Overcoming Problems of High Biomass and Persistent Cloud.” Biogeosciences 9 (1): 179–191. doi:10.5194/bg-9-179-2012.
- Mulverhill, C., N. C. Coops, T. Hermosilla, J. C. White, and M. A. Wulder. 2022. “Evaluating ICESat-2 for Monitoring, Modeling, and Update of Large Area Forest Canopy Height Products.” Remote Sensing of Environment 271: 112919. doi:10.1016/j.rse.2022.112919.
- Pan, Y., R. A. Birdsey, J. Fang, R. Houghton, P. E. Kauppi, W. A. Kurz, O. L. Phillips, et al. 2011. “A Large and Persistent Carbon Sink in the World’s Forests.” Science 333 (6045): 988–993. doi:10.1126/science.1201609.
- Paoli, G. D., L. M. Curran, and J. W. F. Slik. 2008. “Soil Nutrients Affect Spatial Patterns of Aboveground Biomass and Emergent Tree Density in Southwestern Borneo.” Oecologia 155 (2): 287–299. doi:10.1007/s00442-007-0906-9.
- Piao, S., J. Fang, B. Zhu, and K. Tan. 2005. “Forest Biomass Carbon Stocks in China Over the Past 2 Decades: Estimation Based on Integrated Inventory and Satellite Data.” Journal of Geophysical Research: Biogeosciences 110 (G1). doi:10.1029/2005JG000014.
- Piao, S., H. Yue, X. Wang, and F. Chen. 2022. “Estimation of Carbon Sinks in Terrestrial Ecosystems in China: Methods, Progress and Prospects.” Science China Earth Sciences 2021–0197. doi: 10.1360/SSTe-2021-0197.
- Ploton, P., F. Mortier, M. Rejou-Mechain, N. Barbier, N. Picard, V. Rossi, C. Dormann, et al. 2020. “Spatial Validation Reveals Poor Predictive Performance of Large-Scale Ecological Mapping Models.” Nature Communications 11 (1): 4540. doi:10.1038/s41467-020-18321-y.
- Running, S., and M. Zhao. 2019. MYD17A3HGF MODIS/Aqua Net Primary Production Gap-Filled Yearly L4 Global 500 M SIN Grid V006. 2019, Distributed by NASA EOSDIS Land Processes DAAC, 10.5067/MODIS/MYD17A3HGF.006.
- Saatchi, S. S., N. L. Harris, S. Brown, M. Lefsky, E. T. A. Mitchard, W. Salas, B. R. Zutta, et al. 2011. “Benchmark Map of Forest Carbon Stocks in Tropical Regions Across Three Continents.” Proceedings of the National Academy of Sciences 108 (24): 9899–9904. doi:10.1073/pnas.1019576108.
- Santoro, M., O. Cartus, N. Carvalhais, D. M. A. Rozendaal, V. Avitabile, A. Araza, S. de Bruin, et al. 2021. “The Global Forest Above-Ground Biomass Pool for 2010 Estimated from High-Resolution Satellite Observations.” Earth System Science Data 13 (8): 3927–3950. doi:10.5194/essd-13-3927-2021.
- Sexton, J. O., P. Noojipady, X.P. Song, M. Feng, D.X. Song, D.H. Kim, A. Anand, et al. 2016. “Conservation Policy and the Measurement of Forests.” Nature Climate Change 6 (2): 192–196. doi:10.1038/nclimate2816.
- Shen, W., L. Mingshi, C. Huang, X. Tao, and A. Wei. 2018. “Annual Forest Aboveground Biomass Changes Mapped Using ICESat/GLAS Measurements, Historical Inventory Data, and Time-Series Optical and Radar Imagery for Guangdong Province, China.” Agricultural and Forest Meteorology 259: 23–38. doi:10.1016/j.agrformet.2018.04.005.
- Silva, C. A., L. Duncanson, S. Hancock, A. Neuenschwander, N. Thomas, M. Hofton, L. Fatoyinbo, et al. 2021. “Fusing Simulated GEDI, ICESat-2 and NISAR Data for Regional Aboveground Biomass Mapping.” Remote Sensing of Environment 253: 112234. doi:10.1016/j.rse.2020.112234.
- Silveira, E. M., S. Henrique G Silva, F. W. Acerbi-Junior, M. C. Carvalho, L. Marcelo T Carvalho, J. Roberto S Scolforo, and M. A. Wulder. 2019. “Object-Based Random Forest Modelling of Aboveground Forest Biomass Outperforms a Pixel-Based Approach in a Heterogeneous and Mountain Tropical Environment.” International Journal of Applied Earth Observation and Geoinformation 78: 175–188. doi:10.1016/j.jag.2019.02.004.
- Su, Y., Q. Guo, B. Xue, T. Hu, O. Alvarez, S. Tao, and J. Fang. 2016. “Spatial Distribution of Forest Aboveground Biomass in China: Estimation Through Combination of Spaceborne Lidar, Optical Imagery, and Forest Inventory Data.” Remote Sensing of Environment 173: 187–199. doi:10.1016/j.rse.2015.12.002.
- Tang, X., X. Zhao, Y. Bai, Z. Tang, W. Wang, Y. Zhao, H. Wan, et al. 2018. “Carbon Pools in China’s Terrestrial Ecosystems: New Estimates Based on an Intensive Field Survey.” Proceedings of the National Academy of Sciences 115 (16): 4021–4026. doi:10.1073/pnas.1700291115.
- Tingbao, X., and M. Hutchinson. 2011. ANUCLIM Version 6.1 User Guide. Canberra: The Australian National University, Fenner School of Environment and Society.
- Zhang, Y., S. Liang, and L. Yang. 2019. “A Review of Regional and Global Gridded Forest Biomass Datasets.” Remote Sensing 11 (23). doi:10.3390/rs11232744.
- Zhao, X., Y. Yang, H. Shen, X. Geng, and J. Fang. 2019. “Global Soil–Climate–Biome Diagram: Linking Surface Soil Properties to Climate and Biota.” Biogeosciences 16 (14): 2857–2871. doi:10.5194/bg-16-2857-2019.
- Zhao, X., S. Yanjun, H. Tianyu, L. Chen, S. Gao, R. Wang, S. Jin, and Q. Guo. 2018. “A Global Corrected SRTM DEM Product for Vegetated Areas.” Remote Sensing Letters 9 (4): 393–402. doi:10.1080/2150704x.2018.1425560.
- Zheng, D., J. Rademacher, J. Chen, T. Crow, M. Bresee, J. Le Moine, and S.R. Ryu. 2004. “Estimating Aboveground Biomass Using Landsat 7 ETM+ Data Across a Managed Landscape in Northern Wisconsin, USA.” Remote Sensing of Environment 93 (3): 402–411.
- Zhou, G., G. Yin, X. Tang, D. Wen, C. Liu, Y. Kuang, and W. Wang. 2018. Carbon Storage of Forest Ecosystem in China—Biomass Equation, 40–80. Ch: Science Press.
- Zhu, J., H. Huifeng, S. Tao, X. Chi, L. Peng, L. Jiang, J. Chengjun, et al. 2017. “Carbon Stocks and Changes of Dead Organic Matter in China’s Forests.” Nature Communications 8 (1): 1–10. doi:10.1038/s41467-017-00207-1.