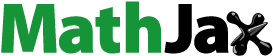
ABSTRACT
Near-real time observations of aerosol properties could have a potential to improve the accuracy of XCO2 retrieval algorithm in operational satellite missions. In this study, we developed a retrieval algorithm of XCO2 (Yonsei Retrieval Algorithm; YCAR) based on the Optimal Estimation (OE) method that used aerosol information at the location of the Orbiting Carbon Observatory-2 (OCO-2) measurement from co-located measurement of the Afternoon constellation (A-train) such as the Cloud-Aerosol Lidar with Orthogonal Polarization (CALIOP) onboard the Cloud-Aerosol Lidar and Infrared Pathfinder Observation (CALIPSO) and the MODerate-resolution Imaging Spectrometer (MODIS) onboard the Aqua. Specifically, we used optical depth, vertical profile, and optical properties of aerosol from MODIS and CALIOP data. We validated retrieval results to the Total Carbon Column Observing Network (TCCON) ground-based measurements and found general consistency. The impact of observed aerosol information and its constraint was examined by retrieval tests using different settings. The effect of using additional aerosol information was analyzed in connection with the bias correction process of the operational retrieval algorithm. YCAR using a priori aerosol loading parameters from co-located satellite measurements and less constraint of aerosol optical properties made comparable results with operational data with the bias correction process in three of the four cases subject to this study. Our work provides evidence supporting the bias correction process of operational algorithms and quantitatively presents the effectiveness of synergic use of multiple satellites (e.g. A-train) and better treatment of aerosol information.
1. Introduction
Carbon dioxide (CO2) is the most important greenhouse gas that causes global warming, and its pre-industrial concentration, which was only 280 ppm, has increased rapidly to nearly 410 ppm today by human activities such as fossil fuel combustion and deforestation. Human activity has been mentioned as a major cause of global warming with very high confidence (Delmotte et al. Citation2021). The surface in-situ observation network of CO2 has provided accurate concentration values, and these observations have allowed us to identify the temporal and spatial changes and the trends in CO2 concentrations. For example, in-situ observations are being conducted by World Meteorological Organization (WMO) Global Atmospheric Watch (GAW) program at about 145 locations worldwide. However, the number of in-situ surface stations is small and the spatial distribution is sparse outside of North America, Europe and East Asia, so there was great uncertainty in estimating the global carbon cycle through in-situ observations (e.g. Hungershoefer et al. Citation2010; Gurney et al. Citation2002). Thus, satellite observations, that provide a larger number of observations with a larger global coverage than in situ measurements, were noted as an alternative.
Over past two decades, satellite payloads such as the Atmosperic InfraRed Sounder (AIRS) onboard the Aqua satellite and the SCanning Imaging Absorption spectrometer for Atmospheric CHartography (SCIAMACHY) onboard the Envisat observed thermal infrared (TIR) and short-wave infrared (SWIR) radiation respectively to measure the concentration of atmospheric CO2 (Crevoisier et al. Citation2004; Reuter et al. Citation2011). However, AIRS observations in the TIR regime were not sensitive to CO2 at surface level, and SCIAMACHY showed~2.5 ppm of single sounding retrieval error because the spectral resolution of SCIAMACHY was not specifically designed for CO2 observation (Reuter et al. Citation2011). However, Houweling et al. (Citation2004) pointed out that combined use of satellite and surface measurement is expected to yield added value in inverse modeling. Japan’s Greenhouse Gas Observing Satellite (GOSAT) launched in 2009 was the first satellite dedicated to greenhouse gas observations, with several years of improvement in the retrieval algorithm showing an average bias of less than 1 ppm in column averaged dry-air mole fraction of atmospheric CO2 (XCO2) with respect to the ground-based measurement (Kulawik et al. Citation2016, Citation2019; Yoshida et al. Citation2011; O’Dell et al. Citation2012). Observations from GOSAT satellites have contributed greatly to the study of CO2 flux estimation as the first satellite to observe CO2 concentrations, but the limitation has been reported in that the distance between satellite observation pixels is approximately 250 km and the density of observations is low by producing approximately only 1,000 pixels per day (Schwandner et al., Citation2017). NASA’s Orbiting Carbon Observatory-2 (OCO-2), which was launched later, is a satellite with spatial resolution and accuracy for estimation of local CO2 flux, producing data of about 100,000 pixels a day, with each pixel size less than 3 km2 in nadir mode measurement (Eldering et al. Citation2017).
In the process of retrieving the atmospheric CO2 concentration from space-based measurements without scatterer in the atmosphere, such as cloud or aerosol, it is reported by Buchwitz, Rozanov, and Burrows (Citation2000) that even simple Differential Optical Absorption Spectroscopy (DOAS) method considering only absorption process can provide retrieval results precise enough to improve flux estimate. But this simple retrieval method could cause large error in scenes with scatterer (Houweling et al. Citation2005; O’Brien and Rayner Citation2002). To overcome these limitations, algorithms based on the Optimal Estimation (OE) were developed by Japan’s NIES and U.S. NASA respectively for XCO2 retrieval from GOSAT satellites (O’Dell et al. Citation2012; Yoshida et al. Citation2011). Bril et al. (Citation2017) developed an algorithm to yield XCO2 values at relatively small computations using linear regression methods for coefficients obtained through empirical orthogonal function (EOF) analysis of the observed radiation spectra. For the OCO-2 satellite, retrieval algorithms have been developed by O’Dell et al. (Citation2018) and Wu et al. (Citation2018) featuring improvements in processes with aerosol, information of gas absorption, and speed-up in radiative transfer model have been developed. Among the retrieval algorithms developed so far, there are some differences in prior information about the atmospheric/surface conditions at the time of observation (also known as “a priori”), or radiative transfer models and inverse models, however, the accuracy and coverage were reduced over pixels with high loading of aerosols. Since anthropogenic activities affect not only emission of CO2, but also emission of aerosols, enhancements of CO2 concentration are often accompanied by high aerosol loading, which might misguide retrieval results if aerosol information used is not realistic.
For aerosol loading and its optical properties affect length of light path to satellite (Mao and Kawa Citation2004), there has been much effort to investigate the impact of realistic aerosol information and its constraint in XCO2 retrieval. Nelson and O’Dell (Citation2019) tested a few aerosol models and constraints for XCO2 retrieval and found less constraint of aerosol loading led to the best results. Sanghavi et al. (Citation2020) also analyzed the theoretical error according to the extent of constraint of the aerosol optical properties, and it was expected that there could be a large retrieval error when too tight constraint was given.
Kim et al. (Citation2016) developed XCO2 retrieval algorithm using GOSAT measurements and used aerosol optical depth information provided by the TANSO-CAI sensor on the same satellite to consider realistic aerosol loading. Jung et al. (Citation2016) improved the accuracy of retrieval results in East Asia by including optical properties of the aerosols in the state variables of the retrieval process, and thus assigning less constraint on aerosol optical properties. This study aims to develop OCO-2 ×CO2 retrieval algorithm based on Kim et al. (Citation2016) and Jung et al. (Citation2016), and to investigate the impact of considering the effect of aerosols in constituting state vector and its a priori. The effect of aerosol information is assessed by two sets of retrieval; retrieval with and without collocated aerosol measurements from the Cloud-Aerosol Lidar with Orthogonal Polarization (CALIOP) and the MODerate Resolution Imaging Spectroradiometer (MODIS). Also, the effect of constraint of aerosol information is assessed by retrieval with aerosol optical properties of fixed value and moderate constraint, respectively. The results of the retrieval algorithm were compared with the operational OCO-2 data and ground-based measurements. Also, the impact of aerosol information and constraint to retrieval was investigated by retrieval test on the cases with heavy aerosol loading.
2. Data
2.1. OCO-2
Launched in July 2014, the OCO-2 satellite produces about 1 million pixels on a daily basis with high spatial resolution of 2.3 km x 1.3 km (in nadir observation) with the aim of detecting regional scale CO2 fluxes between the atmosphere and the surface. The OCO-2 provides hyperspectral observations (Δ v = 0.012 nm to 0.033 nm) at O2 absorption band (O2 A-band; 0.76 μm) and two CO2 absorption bands (1.6 μm, 2.05 μm) as shown in .
Figure 1. An example of L1b measurement data of OCO-2 satellite in O2 A-band (left), weak CO2 absorption band (middle), and strong CO2 absorption band (right).

The observation from the O2 A-band provides information on the total number of dry atmospheric molecules, and the observation of the CO2 absorption wavelengths provides information on the total number of CO2 molecules (Crisp and Johnson Citation2005). This information, with the aid of other ancillary information and retrieval algorithm, finally provides the column-averaged dry air mole fraction of CO2, XCO2. The operational retrieval algorithm includes two post-processes of XCO2; quality filtering and bias correction. Raw XCO2 retrieval values are compared to reference data and the differences are combined with various parameters to make linear relationship between the error and parameters. The XCO2 retrievals are filtered out when unacceptable errors are expected according to this linear relationship. After filtered out, the XCO2 values are combined to reference data to make correction terms based on various parameters in retrieval algorithm, indices on cross track direction, and global offset. Details of quality filtering and bias correction processes are found in Osterman et al. (Citation2022). These post-processes by statistics based on the comparison with reference data greatly improve retrieval performance and are a common way to guarantee the quality of retrieval data. However, using parameters related to aerosol and surface pressure for filtering and correction may not reflect true error and finding physics-based solution would be a preferred way (O’Dell et al. Citation2018; Wunch et al. Citation2011).
Details of the OCO-2 observations are presented in Table S1 in the Supplement. In this study, cloud-free L1b and L2 (B10) pixels on land surface observed in nadir observation mode were used since only observations with nadir mode are allowed to be co-located with CALIOP observations.
2.2 MODIS and CALIOP
OCO-2, Aqua, and CALIPSO satellites shared an orbit called Afternoon Constellation (A-train) and passed over one observation point at very short time intervals until CALIPSO moved to another orbit in September 2018 (Schoeberl Citation2002)). The Aqua satellite passes approximately 360 seconds after the OCO-2 satellite passed first, followed by the CALIPSO satellite approximately 73 seconds after. MODIS onboard the Aqua satellite and CALIOP onboard CALIPSO satellite provide useful information about optical depth, vertical distribution of aerosols and the type of aerosols. The information from both satellites is expected to contribute to improve retrieval quality, as both satellites provide information that cannot be obtained with sensors on the OCO-2.
In this study, we used aerosol optical depth (AOD) from daily mean MODIS data (MYD08). The peak height and full width half maximum (FWHM) of aerosol profile were derived by fitting the extinction coefficient profile (532 nm) of CALIOP data into gaussian shape profile and derived peak height and FWHM are used as a priori (). MODIS AOD and CALIOP aerosol profile were used when criteria for spatial co-location below are met.
Table 1. Aerosol products of the A-train used in this study.
Aerosol optical properties, such as parameters of bimodal log-normal size distribution and refractive index, were chosen for each aerosol types (non-absorbing, dust, black carbon, and mixture) as Table S2 using Aerosol Robotic NETwork (AERONET; Dubovik et al. Citation2002) data from 1999 to 2010 over East Asia based on the method in Lee et al. (Citation2010). Aerosol type used in this retrieval algorithm was determined from aerosol types of CALIOP Vertical Feature Mask (VFM) data. CALIOP data provides six types of aerosols (Mielonen et al. Citation2009), and these types are matched to four types in Lee et al. (Citation2010) by a matching criterion suggested in Song et al. (Citation2015). Details about aerosol type determination using AERONET and CALIOP data in the YCAR are described in the Supplement.
2.3 Ground-based Total Carbon Column Observing Network (TCCON) measurements
Using the Fourier transform spectrometer sensor, TCCON performs observations of high spectral resolution (Δν = 0.02 cm−1) and time resolution (~90 seconds). The absorption of CO2 is measured from observations centered on 6,220 cm−1 and 6,339 cm−1, and the absorption of oxygen from observations centered on 7,885 cm−1 is measured, providing XCO2 by GFIT algorithm by the open-source software GGG2014 package (Wunch et al. Citation2015). As shown in , TCCON observations are distributed across all continents, and the accuracy of the XCO2 observations is known to be approximately 0.4 ppm, generally more accurate than satellite observations (Wunch et al. Citation2010). To compare the TCCON ground observations with the results of retrieval algorithm developed in this study, the results of TCCON observations within a range of ±3° longitude, ±2° latitude, and a time window of ±30 minutes from the OCO-2 observation time were compared around the five sites shown as colored circles in . However, due to the limitations of the computing resource and lack of speed-up scheme for radiative transfer calculation, only maximum of 30 pixels closest to the TCCON observation site were calculated from OCO-2 satellite observations of a given spatiotemporal range.
Figure 2. Locations of TCCON measurement sites (a) worldwide and (b) in East Asia. Measurements at sites with colored circles were used to validate result of retrieval algorithm. The underlying maps were created using the Google Maps.

The results of the TCCON observations were compared by calibrating the vertical sensitivity of the OCO-2 output with the following calculations, as done in Rodgers and Connor (Citation2003).
where is the CO2 vertical distribution of TCCON ground observation with the vertical sensitivity of OCO-2,
is the a priori CO2 vertical distribution used in the OCO-2 retrieval algorithm,
is the averaging kernel of the OCO-2 retrieval algorithm, and
is TCCON retrieval values. In the retrieval process of TCCON ground measurements, the CO2 column density is calculated under the assumption that the shape of the CO2 profile is constant, so the a priori CO2 profile in TCCON retrieval is scaled to have the calculated XCO2 value and this scaled profile is used as
.
2.4 Flowchart of XCO2 retrieval algorithm
Based on Kim et al. (Citation2016) and Jung et al. (Citation2016), an algorithm for retrieval XCO2 using the OCO-2 (Yonsei Carbon Retrieval; YCAR) was developed using the OE method suggested in Rodgers (Citation2000). shows the optimal estimation method schematically. Optimal estimation starts with setting the important variables in calculating the target variable as state variables. From the initial value of the state variables (a priori), the retrieval values are calculated by repeating following two steps: simulating radiance by a radiative transfer model and reflecting the difference of simulated and observed radiance to state variables by the Levenberg-Marquardt equation (L-M equation). When the difference of simulated and observed radiance becomes small enough, the state vector is provided as retrieval value representing the real atmosphere.
Figure 3. A schematic illustration of the optimal estimation method in the retrieval algorithm. The operational retrieval algorithm includes the post process of bias correction (dashed line box), while the YCAR algorithm doesn’t.

In order to achieve good results using the OE method, the variables affecting the
target variables should be selected appropriately, the a priori for the state variables should be similar enough to the actual atmospheric conditions, the information of the absorption/scattering such as the optical properties of the aerosol or the gas absorption coefficient should be accurate, and appropriate inverse model parameter should be selected empirically.
In this study, the set of state variables (state vector) of the retrieval algorithm includes vertical distribution and optical depth of aerosols as well as the concentration of CO2 in 20 layers in the state variables. State vector consists of a total of 48 variables and the overall configuration is shown in . Constitution of the state vector, its a priori, and covariance is mostly based on Jung et al. (Citation2016) except for a priori of CO2 profile from the operational L2 product. CO2 covariance defined on the 20 pressure levels is calculated at each level over East Asia from CT-Asia during 2010–2012 and multiplied by 100 to avoid strong constraint by a priori value, as Jung et al. (Citation2016) did.
Table 2. Constitution of the state vector and its a priori value.
Among the components of the OE method-based calculation algorithm, the a priori concentration of CO2 at each altitude, the composition of the surface albedo, the convergence criteria, and the radiative transfer model were referred to the results of the preceding study, Kim et al. (Citation2016) or Jung et al. (Citation2016) except for a priori of CO2 profile from the operational L2 product. The a priori value of surface albedo is assumed as the ratio of continuum level and solar radiance, namely, top-of-atmosphere albedo. The concentration of CO2 in 20 layers calculated through OE is averaged with weights proportional to the number of molecules in each layer and finally calculated as the XCO2 value. It is noteworthy that the post-processes of quality filtering and bias correction mentioned in Section 2.1. are not included in this work since this work aims to retrieve XCO2 even in high AOD scenes while previous retrieval algorithms often include those post-processes, including the OCO-2 operational algorithm.
3. Results
3.1 Comparison with ground-based measurements of XCO2
shows TCCON ground observations (gray) and the retrieval results from this study (red) and the operational product with bias correction (blue) at 5 TCCON sites. The overpass cases over TCCON which meet spatial criterion of co-location are presented. Note that quality filtering is not applied, as stated previously. The general pattern of temporal variations of XCO2 in ground observations is also seen in the retrieval results.
Figure 4. Time series of different XCO2 datasets of one year period at each site. Symbols with gray,red, and blue color represent the TCCON measurement, the YCAR retrieval, and operational retrieval results, respectively. At the top of each time series are the mean bias and root-mean-square error against TCCON.

The results of satellite retrieval in the Northern Hemisphere show the largest XCO2 value in May, and it was significantly reduced by absorption of CO2 by vegetation at the beginning of July (Randerson et al. Citation1997) as seen in Lamont, Tsukuba, and Anmeyondo sites. Also, in the southern hemisphere where the land area is small, the annual variation of CO2 concentration is expected to be small, which is seen in both satellite retrieval and ground-based observation at Darwin site. The results show general negative bias compared to both TCCON and operational OCO-2 products.
Panel (a) in compares the TCCON XCO2 values with the retrieved XCO2 values in this study for each TCCON observation site. The retrieval results over the analysis period showed consistent seasonal variation with TCCON measurements, with a negative bias of −2.40 ppm overall. Panel (b) in shows retrieval errors against TCCON measurements with different AOD. The distribution of AOD by sites shows that Hefei, Anmeyondo, and Tsukuba are under relatively polluted condition than Lamont and Darwin. The amount of YCAR retrievals co-located with TCCON measurements is small when AOD>0.35. The YCAR retrieval results have negative biases for all AOD ranges, with correlation of errors (and standard deviations) and AODs not clear. Also, retrieval errors with different AOD ranges are similar for both YCAR and operational data, which might imply that the difference between two products has little relation to aerosols.
Figure 5. (a) Comparisons of YCAR retrieval results with TCCON measurements for all 5 sites. Each symbol represents 30-pixel overpass mean. (b) Median error against TCCON (rectangular symbols, left axis) and number of pixels (bars, right axis) in 0.05 co-located AOD bins. Error bars represent 0.5 standard deviation within each bin.

Low bias in retrieved XCO2 could be induced by aerosol in the scene as the aerosol terms in the bias correction of the operational product indicate, or by airmass as in Wunch et al. (Citation2011) indicates. Wunch et al. (Citation2011) suggest spectroscopic or instrumental problems could give rise to the airmass-dependent artifact. However, it seems that the low bias in YCAR results is not clearly dependent on neither AOD () nor solar zenith angle (Figure S1 in Supplement) since the YCAR results show negative bias for most range of AOD and solar zenith angle. Instead, it is found that satellite pixels adjacent to or over clouds induced large bias. YCAR retrieval near Hefei on 3 November 2016 yields error of −13.1 ppm compared to TCCON, which is the maximum bias shown in . The OCO-2 pixels in this case passed the pre-screening process of the operational algorithm and were screened out after the post-screening. The reason those pixels are included in this study is that this study relies on the pre-screening of the operational algorithm to avoid screening out pixels with high AOD in the post-screening.
3.2 Retrieval tests over aerosol-loaded scenes
Most of the TCCON sites, including Anmeyondo, Tsukuba, Lamont, and Darwin, are located in remote or suburban regions, out of urban core. Validation with these observation sites enables for space-borne XCO2 measurements to monitor global increasing CO2 trend and large-scale spatial distribution. Considering a good spatial resolution of the OCO-2, the OCO-2 is also suitable for examining an enhancement of XCO2 (i.e. anomaly from surrounding) within and around cities (Wang et al. Citation2018; Labzovskii, Jeong, and Parazoo Citation2019; Park et al. Citation2021), the retrieval of which is expected to be affected by aerosols emitted with CO2.
To examine the impact of aerosol information and its constraint, XCO2 was retrieved by different settings (Set A~D) with 1) different aerosol a priori, and 2) different constraint of aerosol information (See ). For “fixed AOP” setting, aerosol optical properties in the state vector were excluded, thus giving infinite constraint on those variables. For “without MODIS/CALIOP,” a priori values of aerosol loading were set to arbitrary values (AOD : 0, peak height : 1.2 km, FWHM of profile : 0.5 km) instead of values from MODIS and CALIOP data. Also, the type of aerosol, which is determined by CALIOP VFM data in “with MODIS/CALIOP” setting, is set to “NA” (non-absorbing) type. For example, if a sounding of the OCO-2 is retrieved with “fixed AOP” and “with MODIS/CALIOP” setting, AOD, aerosol height, and aerosol type are determined by MODIS and CALIOP data, and AOP parameters do not change during retrieval process because they are not included in the state vector. Cloud-free scenes over Seoul, Korea (10 May. 2015 10 May 2015 and 17 October 2015; 2 scenes) and Beijing, China (27 Dec. 2014 27 December 2014 and 13 October 2016; 2 scenes) with an enough number of soundings (total 4 scenes; 2 over Beijing and 2 over Seoul) are selected () and XCO2 was retrieved by those four settings for only 1 of 8 cross track footprints due to limited computational resources.
Table 3. Description of retrieval settings for case study in Section 3.2.
Figure S2 to Figure S5 in Supplement show details of retrieved XCO2 and aerosol information used. The co-located MODIS AOD value of most soundings in these selected days are more than 0.3. It is noted that the MODIS AOD is defined at the wavelength of 550 nm, while the AOD in operational retrieval algorithm is defined at 755 nm. Also noted is that any quality filtering was not applied here for both operational and YCAR products and the same a priori XCO2 was used.
As shown in , the enhancement of XCO2 is captured by satellite measurements. Red symbols represent the YCAR data, and blue and green represent the operational data with and without bias correction, respectively. Despite of thick aerosol loading, XCO2 enhancement of about 5 ppm (from bottom to peak level) in a regional scale was detected. The XCO2 enhancement of 5 ppm in this scene provides clear evidence of urban emission of CO2, beyond the uncertainty of XCO2 retrieval reported as around 1 ppm in O’Dell et al. (Citation2018). The retrieval XCO2 are noisy northwards of 40.5 °N because of varying surface pressure assumed due to mountainous terrain. The results of YCAR with “Adaptable AOP” and “with MODIS/CALIOP” setting (Set A) are more comparable to the operational data with bias correction, while the results of YCAR with “fixed AOP” and “without MODIS/CALIOP” (Set D) are more fluctuating and show less enhancement of XCO2.
Figure 7. Retrieval results with setting a and D (see ) over Beijing in 27 Dec. 2014. The YCAR products are represented as red symbols, and the operational products with and without bias correction are indicated as blue and green symbols, respectively.

Table 4. Retrieval settings for case study in Section 3.2.
shows retrieval results in a different scene, together with the change of AOD before and after retrieval. The operational data and YCAR with “Set A” show the enhancement of XCO2 near 37 °N (Panel A). However, this enhancement is not seen in the YCAR with “Set D” and operational data without bias correction (Panel B), instead, the enhancement of retrieved AOD is found, which is not found in a priori AOD (Panel D). This difference in AOD before and after retrieval is not seen in YCAR “Set A” (Panel C). This shows that when the AOPs are tied to their initial values, the signal by CO2 absorption in satellite measurements can be misled to the change of other aerosol parameters. It seems that when the constraint of AOP is too tight, the spectral residual between radiative transfer model and satellite measurements which is caused by unreal AOP in the model can be fitted by other parameters, leading to spurious retrieval values.
Figure 8. (Panel A, B) Same with , but over Seoul in 17 Oct. 2015. (Panel C, D) a priori (yellow) and retrieved (blue) AOD in the YCAR algorithm.

In the case of 13 October 2016 (Figure S3), the retrieval results showed much less sensitivity to additional aerosol information, even though the aerosol optical thickness and altitude were similar compared to the 27 December 2014 case (Figure S2). Given the main aerosol type (black carbon, BC) in the 2016 case unlike the other three cases in , this difference in sensitivity seems to be due to different aerosol type. The emission of BC occurs not only in the combustion of biomass but also in the combustion of oil. According to Ou et al. (Citation2017), BC accounts for about half of all aerosol distribution in Beijing.
In order to quantify the impact of aerosol information, the enhancement of XCO2 in each scene is defined as the difference of between 84%ile and 16%ile of XCO2 (). The enhancement detected by the operational product ranges from 2.60 to 7.47 ppm, with larger value over Beijing than that of Seoul. This pattern is seen in the enhancements derived by other datasets. Significant deviations were found in the differences of enhancement by between “Oper” and other datasets especially in 2016 Beijing case. In that case, “YCAR_A” showed the largest difference, while “YCAR_D” showed least difference. This is counterintuitive since better information put in the retrieval algorithm yielded worse results. Other than 2016 Beijing case, the mean absolute difference of enhancement was calculated (). The enhancements by the operational product without bias correction (Oper_raw) were different as much as 0.54 ppm from those derived with bias correction. With the AOP parameters in the state vector (YCAR_A and YCAR_B), the enhancement of XCO2 derived with ancillary information of aerosol were closer to operational product than the enhancement detected without ancillary information as much as 0.41 ppm. Comparing YCAR_C and YCAR_D, however, the addition of ancillary information did not improve performance. This is probably because YCAR_C and D did not have AOPs in the state vector, so could not resolve the information given.
Table 5. Enhancement of XCO2 by each datasets. The enhancements are the difference of XCO2 between 84%ile and 16%ile in the scene. “Oper_raw” indicates the operational product without bias correction. Numbers in parenthesis indicate the difference from the enhancement by “Oper”.
Table 6. Mean absolute difference from the enhancement by “Oper” in selected scenes other than 13 Oct. 2016, Beijing case.
4. Summary and discussion
We have developed an algorithm for retrieval of XCO2 using the OCO-2 measurements based on the Optimal Estimation method. We took advantages of using datasets from the MODIS and the CALIOP, which are the members of the A-train as well as the OCO-2. As a priori values of aerosol loading parameters in the state vector, we used AOD from the MODIS data, and peak height and FWHM of the aerosol profile from the CALIOP data. Also, optical properties of aerosol (thus, aerosol type) were determined by feature mask data of the CALIOP. Retrieval results in 1-year period at 5 stations of the TCCON showed similar seasonal variation when compared to ground-based TCCON measurement, with a negative bias of −2.40 ppm. Since biases in XCO2 in even a sub-ppm scale can induce large error in flux estimation (Miller et al. Citation2007), further investigation on attribution and correction of biases is needed. Retrieval test over a case scene with thick aerosol loading showed the YCAR retrieval is able to detect urban emission of CO2 despite of presence of scatterer.
In addition to the validation with ground-based measurements, the results of the YCAR algorithm were tested over the scenes of high aerosol loading over Seoul and Beijing with different treatments of aerosol information. Retrieval tests over these scenes with thick aerosol loading showed the YCAR retrieval is able to detect urban emission of CO2 despite of presence of scatterer. The YCAR retrieval with more realistic a priori of aerosol loading and with less constraint of aerosol optical properties best fitted with the operational product with the bias correction, while the YCAR with the other settings fitted more with the operational products without the bias correction. Also, the methods for considering aerosols in retrieval process by either statistical correction (e.g. bias correction in the operational product) or physical approach (e.g. improved aerosol information in the YCAR) were compared in terms of the enhancement of XCO2. This comparison suggests that results from both methods support each other and make “Oper” (and the enhancement of XCO2 derived from it) more reliable. Comparing YCAR results with and without AOP parameters, simply setting less constraint on aerosol parameters, improved performance. This could contribute to carbon community interested in retrieval from remote sensing.
Additionally, more investigation on the exceptional case (16 Oct. 2016 16 October 2016, Beijing) must be followed because of little effect of aerosol information in this case. One possible explanation is that the aerosol layer at lower altitude is so absorbent that it blocks the CO2 signal in the lower layer where most of change in CO2 concentration is expected. In this case, even if the aerosol parameter is changed in the retrieval process, the retrieval result may not be affected. It seems that more retrieval cases are needed for the cause of error in the absorbing aerosol environment.
The improvement from ancillary aerosol information also highlights synergic use of multiple satellites/sensors. As a number of missions for measurements of CO2 and aerosols are operational now or planned in future, this synergic use can be applied to many combinations of satellites (e.g. the OCO-3 with geostationary GEMS/TEMPO/Sentinel-5P, the TANSO-FTS2 with TANSO-CAI2 onboard GOSAT-2).
We compared the YCAR results with reference data being the operational OCO-2 product with bias correction, because for now there is no ground-based network around polluted area, such as East Asia. It is expected that dense ground-based network of both aerosols and CO2 around cities in East Asia can give a sight of spatiotemporal distribution of these species and reveal the impact of aerosol on remote sensing of CO2.
One of a challenge in development of YCAR algorithm was its large computational burden, which mainly arise from the calculation of radiative transfer model. It is expected that the speed-up scheme of radiative transfer model such as PCA-based speed-up method in O’Dell et al. (Citation2018) would allow full retrieval instead of 30 selected pixels at each overpass, and lead to better analysis on the performance of this retrieval algorithm.
Supplemental Material
Download MS Word (1 MB)Acknowledgments
This work was supported by the National Institute of Meteorological Research [Meteorological work support technology development research] “Climate change prediction support and application research(KMA2018-00321)” and the Samsung PM2.5 Strategic Research Program. OCO-2 L1b and L2 data in this study were produced by the OCO-2 project at the Jet Propulsion Laboratory, California Institute of Technology, and obtained from the OCO-2 data archive maintained at the NASA Goddard Earth Science Data and Information Services Center. The CALIOP data are available from https://subset.larc.nasa.gov/calipso/. TCCON data were obtained from the TCCON data archive hosted by CaltechDATA, and are available from https://tccondata.org/ (Anmeyondo: 10.14291/tccon.ggg2014.anmeyondo01.R0/1149284, Darwin: 10.14291/tccon.ggg2014.darwin01.R0/1149290, Hefei: 10.14291/tccon.ggg2014.hefei01.R0, Lamont: 10.14291/tccon.ggg2014.lamont01.R1/1255070, Tsukuba: 10.14291/tccon.ggg2014.tsukuba02.R2).
Disclosure statement
The authors declare that they have no known competing financial interests or personal relationships that could have appeared to influence the work reported in this paper.
Data availability statement
The retrieval results of YCAR algorithm in this research can be found in https://figshare.com/s/c5fcdc5a253d6e23b42b.
Supplementary Material
Supplemental data for this article can be accessed online at https://doi.org/10.1080/15481603.2023.2209968.
Additional information
Funding
References
- Bril, А., S. Maksyutov, D. Belikov, S. Oshchepkov, Y. Yoshida, N. M. Deutscher and Giffith, D. et al. 2017. “EOF-Based Regression Algorithm for the Fast Retrieval of Atmospheric CO2 Total Column Amount from the GOSAT Observations.” Journal of Quantitative Spectroscopy & Radiative Transfer 189: 258–14. doi:10.1016/j.jqsrt.2016.12.005.
- Buchwitz, M., V. V. Rozanov, and J. P. Burrows. 2000. “A Near-Infrared Optimized DOAS Method for the Fast Global Retrieval of Atmospheric CH4, CO, CO2, H2O, and N2O Total Column Amounts from SCIAMACHY Envisat-1 Nadir Radiances.” Journal of Geophysical Research 105 (D12): 15231–15245. doi:10.1029/2000JD900191.
- Crevoisier, C., S. Heilliette, A. Chédin, S. Serrar, R. Armante, and N. A. Scott. 2004. “Midtropospheric CO2 Concentration Retrieval from AIRS Observations in the Tropics.” Geophysical Research Letters 31 (17). doi:10.1029/2004GL020141.
- Crisp, D., and C. Johnson. 2005. “The Orbiting Carbon Observatory Mission.” Acta Astronautica 56 (1–2): 193–197. doi:10.1016/j.actaastro.2004.09.032.
- Dubovik, O., B. Holben, T. F. Eck, A. Smirnov, Y. J. Kaufman, M. D. King and, and T. Didier. et al. 2002. “Variability of Absorption and Optical Properties of Key Aerosol Types Observed in Worldwide Locations.” Journal of the Atmospheric Sciences 59 (3): 590–608. doi:10.1175/1520-0469(2002)059<0590:VOAAOP>2.0.CO;2.
- Eldering, A., P. O. Wennberg, C. Wunch, C. Frankenberg, C. M. Roehl, D. Viatte, C. Avis, et al. 2017. “The Orbiting Carbon Observatory-2: First 18 Months of Science Data Products.” Atmospheric Measurement Techniques 10 (2): 549–563. doi:10.5194/amt-10-549-2017.
- Gurney, K. R., R. M. Law, A. S. Denning, P. J. Rayner, D. Baker, P. Bousquet and Bruhwiler, L. et al. 2002. “Towards Robust Regional Estimates of CO2 Sources and Sinks Using Atmospheric Transport Models.” Nature 415 (6872): 626–630. doi:10.1038/415626a.
- Houweling, S., F. M. Breon, I. Aben, C. Rödenbeck, M. Gloor, M. Heimann, and P. Ciais. 2004. “Inverse Modeling of CO2 Sources and Sinks Using Satellite Data: A Synthetic Inter-Comparison of Measurement Techniques and Their Performance as a Function of Space and Time.” Atmospheric Chemistry and Physics 4 (2): 523–538. doi:10.5194/acp-4-523-2004.
- Houweling, S., W. Hartmann, I. Aben, H. Schrijver, J. Skidmore, G. J. Roelofs, and F. M. Breon. 2005. “Evidence of Systematic Errors in SCIAMACHY-Observed CO2 Due to Aerosols.” Atmospheric Chemistry and Physics 5 (11): 3003–3013. doi:10.5194/acp-5-3003-2005.
- Hungershoefer, K, F. M. Breon, P. Peylin, F. Chevallier, P. Rayner, A. Klonecki , S. Houweling, and J. Marshall. et al. 2010. “Evaluation of Various Observing Systems for the Global Monitoring of CO2 Surface Fluxes.” Atmospheric Chemistry and Physics 10 (21): 10503–10520. doi:10.5194/acp-10-10503-2010.
- IPCC. 2021. Climate Change 2021: The Physical Science Basis. Contribution of Working Group I to the Sixth Assessment Report of the Intergovernmental Panel on Climate Change. Masson-Delmotte, V., P. Zhai, A. Pirani, S. L. Connors, C. Péan, S. Berger, N. Caud, Y. Chen, L. Goldfarb, M. I. Gomis, M. Huang, K. Leitzell, E. Lonnoy, J. B. R. Matthews, T. K. Maycock, T. Waterfield, O. Yelekçi, R. Yu, and B. Zhou, eds. Cambridge University Press: Cambridge, United Kingdom and New York, NY, USA, In press. doi:10.1017/9781009157896.
- Jung, Y., J. Kim, W. Kim, H. Boesch, H. Lee, C. Cho, and T. Y. Goo. 2016. “Impact of Aerosol Property on the Accuracy of a CO2 Retrieval Algorithm from Satellite Remote Sensing.” Remote Sensing 8 (4): 322. doi:10.3390/rs8040322.
- Kim, W, J. Kim, Y. Jung, H. Boesch, H. Lee, S. Lee, and M. L. Ou . et al. 2016. “Retrieving XCO2 from GOSAT FTS Over East Asia Using Simultaneous Aerosol Information from CAI.” Remote Sensing 8 (12): 994. doi:10.3390/rs8120994.
- Kulawik, S. S., S. Crowell, D. Baker, J. Liu, K. McKain, C. Sweeney and D. Wunch. 2019. Characterization of OCO-2 and ACOS-GOSAT Biases and Errors for CO2 Flux Estimates. Atmospheric Measurement Techniques Discussions.
- Kulawik, S., D. Wunch, C. O’Dell, C. Frankenberg, M. Reuter, T. Oda, and C. E. Miller . et al. 2016. “Consistent Evaluation of ACOS-GOSAT, BESD-SCIAMACHY, CarbonTracker, and MACC Through Comparisons to TCCON.” Atmospheric Measurement Techniques 9 (2): 683–709. doi:10.5194/amt-9-683-2016.
- Labzovskii, L. D., S. J. Jeong, and N. C. Parazoo. 2019. “Working Towards Confident Spaceborne Monitoring of Carbon Emissions from Cities Using Orbiting Carbon Observatory-2.” Remote Sensing of Environment 233: 111359. doi:10.1016/j.rse.2019.111359.
- Lee, J., J. Kim, C. H. Song, S. B. Kim, Y. Chun, B. J. Sohn, and B. N. Holben. 2010. “Characteristics of Aerosol Types from AERONET Sunphotometer Measurements.” Atmospheric Environment 44 (26): 3110–3117. doi:10.1016/j.atmosenv.2010.05.035.
- Mao, J., and S. R. Kawa. 2004. “Sensitivity Studies for Space-Based Measurement of Atmospheric Total Column Carbon Dioxide by Reflected Sunlight.” Applied Optics 43 (4): 914–927. doi:10.1364/AO.43.000914.
- Mielonen, T., A. Arola, M. Komppula, J. Kukkonen, J. Koskinen, G. De Leeuw, and K. E. J. Lehtinen. 2009. “Comparison of CALIOP Level 2 Aerosol Subtypes to Aerosol Types Derived from AERONET Inversion Data.” Geophysical Research Letters 36 (18). doi:10.1029/2009GL039609.
- Miller, C. E., D. Crisp, P. L. DeCola, S. C. Olsen, J. T. Randerson, A. M. Michalak, and D. B. A. Jones. et al. 2007. “Precision Requirements for Space‐based Data.” Journal of Geophysical Research Atmospheres 112 (D10). doi:10.1029/2006JD007659.
- Nelson, R. R., and C. W. O’Dell. 2019. “The Impact of Improved Aerosol Priors on Near-Infrared Measurements of Carbon Dioxide.” Atmospheric Measurement Techniques 12 (3): 1495–1512. doi:10.5194/amt-12-1495-2019.
- O’Brien, D. M., and P. J. Rayner. 2002. “Global Observations of the Carbon Budget, 2, CO2 Column from Differential Absorption of Reflected Sunlight in the 1.61 Μm Band of CO2.” Journal of Geophysical Research Atmospheres 107 (D18): ACH-6. doi:10.1029/2001JD000617.
- O’Dell, C. W., B. Connor, H. Bösch, D. O’Brien, C. Frankenberg, R. Castano, and J. McDuffie . et al. 2012. “The ACOS CO2 Retrieval Algorithm – Part 1: Description and Validation Against Synthetic Observations.” Atmospheric Measurement Techniques 5 (1): 99–121. doi:10.5194/amt-5-99-2012.
- O’Dell, C., A. Eldering, P. O. Wennberg, D. Crisp, M. Gunson, B. Fisher, and A. Merrelli. et al. 2018. “Improved Retrievals of Carbon Dioxide from Orbiting Carbon Observatory-2 with the Version 8 ACOS Algorithm.” Atmospheric Measurement Techniques 11 (12): 6539–6576. doi:10.5194/amt-11-6539-2018.
- Osterman, G. B., C. W. O’Dell, A. Eldering, B. Fisher, D. Crisp, C. Cheng, C. Frankenberg, et al. 2020 Orbiting Carbon Observatory-2 & 3 (OCO-2 & OCO-3) Data Product User’s Guide, Operational Level 2 Data Versions 10 and Lite File Version 10 and Vearly, National Aeronautics and Space Administration Jet Propulsion Laboratory California Institute of Technology Pasadena, California. Accessed Dec 29 2022 https://docserver.gesdisc.eosdis.nasa.gov/public/project/OCO/OCO2_OCO3_B10_DUG.pdf
- Ou, Y., W. Zhao, J. Wang, W. Zhao, and B. Zhang. 2017. “Characteristics of Aerosol Types in Beijing and the Associations with Air Pollution from 2004 to 2015.” Remote Sensing 9 (9): 898. doi:10.3390/rs9090898.
- Park, H., S. Jeong, H. Park, L. D. Labzovskii, and K. W. Bowman. 2021. “An Assessment of Emission Characteristics of Northern Hemisphere Cities Using Spaceborne Observations of CO2, CO, and NO2.” Remote Sensing of Environment 254: 112246. doi:10.1016/j.rse.2020.112246.
- Randerson, J. T., M. V. Thompson, T. J. Conway, I. Y. Fung, and C. B. Field. 1997. “The Contribution of Terrestrial Sources and Sinks to Trends in the Seasonal Cycle of Atmospheric Carbon Dioxide.” Global Biogeochemical Cycles 11 (4): 535–560. doi:10.1029/97GB02268.
- Reuter, M., H. Bovensmann, M. Buchwitz, J. P. Burrows, B. J. Connor, N. M. Deutscher, and D. Wunch. et al. 2011. “Retrieval of Atmospheric CO2 with Enhanced Accuracy and Precision from SCIAMACHY: Validation with FTS Measurements and Comparison with Model Results.” Journal of Geophysical Research Atmospheres 116 (D4). doi:10.1029/2010JD015047.
- Rodgers, C. D. 2000. Inverse Methods for Atmospheric Sounding: Theory and Practice. 2: 1–238. Singapore: World scientific.
- Rodgers, C. D., and B. J. Connor. 2003. “Intercomparison of Remote Sounding Instruments.” Journal of Geophysical Research Atmospheres 108 (D3). doi:10.1029/2002JD002299.
- Sanghavi, S., R. Nelson, C. Frankenberg, and M. Gunson. 2020. “Aerosols in OCO-2/GOSAT Retrievals of XCO2: An Information Content and Error Analysis.” Remote Sensing of Environment 251: 112053. doi:10.1016/j.rse.2020.112053.
- Schoeberl, M. R. 2002, June. “The Afternoon Constellation: A Formation of Earth Observing Systems for the Atmosphere and Hydrosphere.” IEEE International Geoscience and Remote Sensing Symposium, Toronto, ON, Canada, IEEE, 1, 354–356.
- Schwandner, F. M., M. R. Gunson, C. E. Miller, S. A. Carn, A. Eldering, T. Krings, K. R. Verhulst et al, 2017. “Spaceborne Detection of Localized Carbon Dioxide Sources.” Science 358 (6360): eaam5782.
- Song, S., J. E. Kim, E. Lim, J. W. Cha, and J. Kim. 2015. “Physical, Chemical and Optical Properties of an Asian Dust and Haze Episodes Observed at Seoul in 2010.” Journal of Korean Society for Atmospheric Environment 31 (2): 131–142. doi:10.5572/KOSAE.2015.31.2.131.
- Wang, S., Y. Zhang, J. Hakkarainen, W. Ju, Y. Liu, F. Jiang, and W. He. 2018. “Distinguishing Anthropogenic CO2 Emissions from Different Energy Intensive Industrial Sources Using OCO‐2 Observations: A Case Study in Northern China.” Journal of Geophysical Research Atmospheres 123 (17): 9462–9473. doi:10.1029/2018JD029005.
- Wu, L., O. Hasekamp, H. Hu, J. Landgraf, A. Butz, I. Hase and F. Aben. et al. 2018. “Carbon Dioxide Retrieval from OCO-2 Satellite Observations Using the RemoTec Algorithm and Validation with TCCON Measurements.” Atmospheric Measurement Techniques 11 (5): 3111–3130. doi:10.5194/amt-11-3111-2018.
- Wunch, D., G. C. Toon, J. F. L. Blavier, R. A. Washenfelder, J. Notholt, B. J. Connor and P. O. Wennberg. et al. 2011. “The Total Carbon Column Observing Network.” Philosophical Transactions of the Royal Society A: Mathematical, Physical & Engineering Sciences 369 (1943): 2087–2112. doi:10.1098/rsta.2010.0240.
- Wunch, D., G. C. Toon, V. Sherlock, N. M. Deutscher, C. Liu, D. G. Feist, and P. O. Wennberg. 2015. “Documentation for the 2014 TCCON Data Release (GGG2014.R0).“ CaltechDATA. https://doi.org/10.14291/TCCON.GGG2014.DOCUMENTATION.R0/1221662
- Wunch, D., G. C. Toon, P. O. Wennberg, S. C. Wofsy, B. B. Stephens, M. L. Fischer and J. F. Blavier. et al. 2010. “Calibration of the Total Carbon Column Observing Network Using Aircraft Profile Data.” Atmospheric Measurement Techniques 3 (5): 1351–1362. doi:10.5194/amt-3-1351-2010.
- Yoshida, Y., Y. Ota, N. Eguchi, N. Kikuchi, K. Nobuta, H. Tran and T. Yokota. et al. 2011. “Retrieval Algorithm for CO2 and CH4 Column Abundances from Short-Wavelength Infrared Spectral Observations by the Greenhouse Gases Observing Satellite.” Atmospheric Measurement Techniques 4 (4): 717–734. doi:10.5194/amt-4-717-2011.